Quantitative diffusion magnetic resonance imaging in head and neck tumors
Biomedical imaging in head and neck tumors (HNT)
Worldwide, head and neck malignancies account for approximately 4% of the cancer patients, with 550,000 new cases and 380,000 deaths annually, making HNT the sixth most common cancer, globally (1,2). In 2016, the global 5-year prevalence rates for cancers of lip and oral cavity, nasopharynx, larynx, and other pharynx were 13.5, 4.4, 8.5, and 6.0 per 100,000, respectively (3). Notably, the highest age-standardized incidence rate for nasopharyngeal HNT are reported in the Asia-Pacific region, particularly in Indonesia, Micronesia and Eastern Asia, especially Southern China (3). Greater than 90% of HNT are squamous cell carcinomas (SCC) followed by lymphomas (4). While radiation therapy and surgical resection can achieve favorable results for early stage head and neck SCC, the odds are less favorable for advanced stages.
Biomedical imaging has a substantial role in diagnosis, and initial staging of HNT, as well as monitoring the treatment response and detection of local recurrence or distant metastasis. Nevertheless, accurate initial risk stratification, and predictive biomarkers of molecular subtypes are needed to improve patient care and pave the road for personalized treatment options in era of precision medicine (5). Noninvasive imaging biomarkers can play a crucial role in assessment of the entire tumor sample and can be repeated longitudinally to monitor treatment response, and guide decision-making process. This review focuses on the promises of quantitative diffusion MRI in assessment of patients with HNT.
Diffusion weighted imaging (DWI)
Diffusion MRI can assess cellular density and cytoarchitecture based on the measurement of water diffusivity. For the purpose of diffusion MRI, two strong opposed gradient pulses are applied along a certain diffusion direction, with the first diffusion-sensitizing gradient dephasing the water molecules, and the second gradient completely rephasing the stationary molecules. In living tissues, the motion of water molecules is “restricted” by interactions with other macromolecules, and cell membranes, which is translated into a signal intensity decrease. Such a signal intensity decrease depends on the degree of molecule movement and respective speed along the diffusion-sensitizing gradient, as well as the strength of the gradient itself, and the duration of the diffusion-encoding gradients (b-value) (6,7). Thus, diffusion abnormalities of water molecules, captured by DWI, can reflect changes of tissue organization and impediments in water molecule motion at a cellular level.
The diffusion-sensitizing effects from the gradients are indicated by the b-value (s/mm2), which represents the duration between gradient pulses that water molecules are allowed to diffuse before the distance is measured (6). The b-values are defined by the gradient strength, duration, and the time interval between the gradient pulses (8). Mono- and biexponential models can be used for quantifying the diffusion (9).
For monoexponential models, the apparent diffusion coefficient (ADC, expressed in mm2/s) is calculated by means of the least-squares fit of signal intensities from images acquired with different b-values, where S(b) and S0 are the signal intensities on images with and without diffusion weighting, respectively, b is the gradient factor in s/mm2, and D is the ADC:
However, while fast-moving water molecules quickly lose their phase coherence and signal intensity, even at low b-values, slow-moving molecules will retain high signal intensities far into the higher ranges of b-values (10). Thus, low b-value (0 up to 200 s/mm2) diffusion images reflect microvascularity and tissue perfusion; whereas, high b-value (800 or 1,000 s/mm2) diffusion images represent tissue cellularity (11). Thus, mono-exponential ADC values cannot separate pure molecular diffusion from motion of water molecules in the capillary network; whereas, multi-exponential models using several b-values are more suitable for accurate quantification of diffusion without perfusion contamination (10,11).
The intravoxel incoherent motion (IVIM) and diffusion kurtosis imaging (DKI) assess the water molecule diffusivity with multiple b-values. The IVIM can distinguish pure molecular diffusion from motion of water molecules in the capillary network base on low b-value (<200 s/mm2) and high b-value (>200 s/mm2) diffusion image acquisitions (12,13). The relationship between signal intensities and multiple b-values can distinguish the real diffusion of water molecules (D) from the contribution of perfusion to the signal decay (D*, pseudodiffusion), and the contribution of perfusion to the diffusion signal (f, vascular volume fraction). For biexponential models, the metrics related to IVIM for each b-value are calculated using (12):
In DKI, multiple b-values are applied to assess the extent to which the diffusion pattern of water molecules deviates from a perfect Gaussian curve that is assumed when calculating monoexponential ADC values (14):
There are limited number of studies examining the optimal combination and number of b-values for IVIM. Lemke et al. suggested that at least 10 b-values should be used for fitting the IVIM signal with assigning more weight to low b-value acquisitions (0 to 100 s/mm2) (15). However, Gurney-Champion et al. found that 7 b-values would be enough for abdominal imaging purposes, and only 3 b-values for imaging of the liver (16). Sasaki et al. compared the traditional least-squares method with 11 b-values versus a geometric approach requiring only 3 b-values for differentiating between HNTs (10), and found that despite higher D values and lower f values, the geometric approach had similar accuracy to the least-squares method in differentiation of lymphomas from SCC, as well as different types of salivary gland tumors from each other (10). Table 1 summarizes the IVIM metrics used in evaluation of HNT (10,17-29). Noij et al. recommend inclusion of at least 4 b-values below 200 s/mm2 for optimal fit estimation of perfusion-related parameters (4).
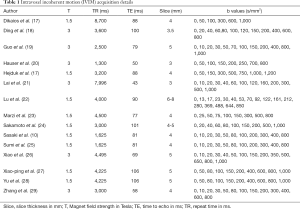
Full table
Regarding the fit model used for estimation of diffusion parameters, the majority of studies listed in Table 1 have applied bi-exponential with Levenberg Marquard algorithm for the IVIM fit—with the exception of Dikaios et al. applying maximum probability model nonlinear regression model (17), Ding et al. applying non-linear least-squares and simplified linear fit (18), and Sasaki et al. applying both traditional least-squares method and geometric approach (10), as mentioned above.
For routine head and neck DWI scan in clinical practice, single shot (SS) echo planar imaging (EPI) technique is most commonly used (30). The SS-EPI is relatively insensitive to motion but prone to susceptibility artifacts, chemical shift, and geometric distortion, with a limited spatial resolution and relatively thick sections (31). Compared to SS-EPI, turbo spin echo technique requires longer echo time but less susceptibility artifacts and better spatial resolution (32). The SS version of turbo spin echo is a diffusion-weighted HASTE sequence with lower sensitivity to motion and susceptibility artifacts as well as geometric distortion compared to the EPI sequence (33). Overall, the non-echo planar diffusion can improve image quality with lower susceptibility artifacts and higher spatial resolution; however, they non-EPI DWI scans take longer to acquire (which can introduce more motions) and have lower signal-to-noise ratio, which requires multiple averages and prolongs scanning time (11). Hence, non-echo planar diffusion is usually reserved for problem solving rather than routine clinical practice.
Differentiation of head and neck cancers
Tissue sampling and pathologic examination remain the gold standard for assessing the malignant nature of a head and neck lesion; however, tissue biopsy is not without risk, and cannot examine the whole lesion. Multiple studies have demonstrated the ability and reliability of quantitative diffusion MRI in distinction of benign from malignant lesions and differentiation of different HNT (34). Although there are many factors affecting the ADC, it is generally accepted that the ADC of a given voxel is inversely proportional to the cellularity of the tissue included in that voxel, and malignant tumors likely demonstrate lower ADC values compared with benign lesions owing to their relatively higher cellularity.
Some authors have focused on distinction of malignant from benign lesions using average ADC values. Using a 1.5 T magnet with split acquisition of fast spin-echo signals (SPLICE) and b-values of 0 and 771 s/mm2, Sakamoto et al. have applied averaged ADC for differentiation of 16 cysts, 32 benign tumors, and 19 malignant tumors in head and neck (35). They found cysts to have higher mean ADC (2.41±0.48 ×10−3 mm2/s), compared to benign (1.48±0.62 ×10−3 mm2/s), and malignant (1.23±0.45 ×10−3 mm2/s) tumors (P<0.001); and an ADC value of >2.10 ×10−3 mm2/s could identify cysts, with 90% accuracy, 94% sensitivity, and 88% specificity (35). However, there was no significant difference between the ADC values of benign and malignant tumors (P=0.246) (35). Notably, when the same group applied (IVIM) technique and half-Fourier single-shot turbo spin-echo (HASTE) diffusion MRI for distinction of 23 malignant and 10 benign head and neck lesions, they found significantly lower average ADC (0.993±0.157 ×10−3 mm2/s) and D values (0.813±0.172 ×10−3 mm2/s) in malignant tumors compared to benign lesions (1.33±0.212 and 1.16±0.238 ×10−3 mm2/s, respectively) (24). Using a 3 T scanner with a single-shot spin-echo EPI and b-values of 0 and 1,000 s/mm2, Srinivasan et al. also found a lower average ADC (1.071±0.293 ×10−3 mm2/s) in 16 malignant HNT compared to 17 benign lesions (1.505±0.487 ×10−3 mm2/s) with a P=0.004 (36).
Quantitative diffusion MRI can also help with differentiation of various histopathologies; for example, differentiation of various salivary gland lesions. Accurate preoperative differentiation of a salivary gland tumor is important in establishing the surgical indication and preoperative planning. Fine needle aspiration cytology, which is currently used for diagnosis of a parotid gland lesion, can achieve 81% to 98% accuracy (37). While the majority of salivary gland tumors are benign (either pleomorphic adenomas or Warthin tumors), some can be malignant (adenoid cystic carcinoma or mucoepidermoid carcinoma). Prior studies suggest that pleomorphic adenomas (which contain myxoid tissue) have the highest ADC; whereas, Warthin tumors (which contain lymphoid tissue) have the lowest ADC values (38).
Using a 1.5 T scanner, with modified Sensitivity Encoding algorithm (SENSE) methods and b-values of 0, 500, and 1,000 s/mm2, Habermann et al. found significantly different average ADC between pleomorphic adenomas (2.14±0.11 ×10–3 mm2/s), Warthin tumors (0.85±0.1 ×10-3 mm2/s), and mucoepidermoid carcinomas (1.04±0.3 ×10-3 mm2/s) in 45 patients with parotid tumor (P<0.001) (39). Ikeda et al. have also shown that mean ADC of Warthin tumors (0.96±0.13 ×10−3 mm2/s) was significantly lower (P<0.01) than that of malignant salivary gland tumors (1.19±0.19 ×10−3 mm2/s) (40). And Kikuchi et al. proposed an average ≥1.5 ×10−3 mm2/s for distinction of parotid pleomorphic adenomas from other tumors (41).
Quantitative diffusion MRI can also help with differentiation between benign and malignant thyroid nodules, with latter showing lower average ADC values (6). Using a 1.5 T scanner with SS EPI acquisition and b-values of 0, 250, and 500 s/mm2, Razek et al. showed that mean ADC value of malignant solitary thyroid nodules (0.73±0.19 ×10−3 mm2/s) was significantly lower than benign nodules (1.8±0.27 ×10−3 mm2/s, P=0.0001) (42). They suggested that an ADC value of <0.98 ×10−3 mm2/s could identify malignant thyroid nodules with an accuracy of 98.9%, sensitivity of 97.5%, and specificity of 91.7%. Using a 1.5 T scanner with SS EPI and b-values of 0 and 1,000 s/mm2, Erdem et al. also found lower average ADC in malignant thyroid nodule (0.695±0.312 ×10−3 mm2/s) compared to benign nodules (2.745±0.601 ×10−3 mm2/s, P<0.001) (43). Similarly, using a 1.5 T scanner with SS EPI and b-values of 100, 200 and 300 s/mm2, Bozgeyik et al. showed that malignant thyroid nodules had lower mean ADC (0.96±0.65, 0.56±0.43 and 0.30±0.20 ×10−3 mm2/s) compared to benign lesions (3.06±0.71, 1.80±0.60, and 1.15±0.43 ×10−3 mm2/s), respectively (44). They reported receiver operatic characteristic (ROC) areas under the curve (AUC) and cutoff values of 0.997 and 1.45 ×10−3 mm2/s; 1.00 and 0.65 ×10−3 mm2/s; and 0.884 and 0.36 ×10−3 mm2/s, for differentiating benign from malignant thyroid nodules at 100, 200 and 300 s/mm2 b-values, respectively (44).
Reactive versus metastatic lymphadenopathy
Nodal metastases herald poor prognosis in patients with head and neck cancer, and their detection is important for treatment planning, extent of radiation treatment field, or surgical neck dissection method. Currently, the differentiation of metastatic lymphadenopathy primarily relies on size criteria; however, nonenlarged nodes may harbor malignancy, and reactive nodes may be prominently enlarged (45). In addition, in patients presenting with suspicious cervical lymphadenopathy, differentiation of lymphoma from metastatic lymph nodes of unknown primary cancer site can be challenging. Distinction of metastatic from lymphomatous lymphadenopathy is particularly crucial since they demand radically different treatment approaches. Quantitative diffusion MRI can help with distinction of these entities.
Table 2 summarizes the results of studies comparing non-malignant, metastatic, and lymphomatous lymph nodes in head and neck (46-49). Except for Sumi et al. who found a lower average ADC in benign lymphadenopathy compared to metastatic lesions (48), other studies reported higher average ADC in benign lymph nodes compared to metastatic lesions (46,47,49); and all studies reported higher average ADC in metastatic lesions compared to lymphoma (Table 2). On the other hand, Hejduk et al. found not significant difference in the mean values of D, D*, and f between metastatic and non-metastatic lymph nodes (50).
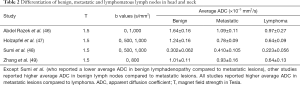
Full table
Abdel Razek et al. suggested an average ADC value of 1.38 ×10−3 mm2/s as a threshold value for differentiating malignant from benign lymph nodes, with an accuracy of 96%, sensitivity of 98%, and specificity of 88% (46). Holzapfel et al. suggested an average ADC value of 1.02 ×10−3 mm2/s as a threshold value for differentiating malignant from benign lymph nodes, with an accuracy of 94.3%, a sensitivity of 100%, and a specificity of 87.0% (47). For distinction of lymphomatous from metastatic lymphadenopathy, Zhang et al. suggested an average ADC value of 0.77 ×10−3 mm2/s as a threshold value, with an AUC of 0.94, a sensitivity of 83%, and a specificity of 89% (49).
Applying IVIM (Table 1), Yu et al. compared the diffusion characteristics of nasopharyngeal carcinoma with head and neck lymphoma (28), and found that the primary lesions in nasopharyngeal carcinoma had higher ADC, D, D*, fD* and f value compared to lymphomas. They proposed an ADC value threshold of 0.761 ×10-3 mm2/s (0.781 AUC, 93.90% sensitivity, and 55.00% specificity); a D value threshold of 0.66 ×10 mm/s (0.802 AUC, 54.88% sensitivity, and 100.00% specificity); D* value threshold of 7.89 ×10 mm/s (0.898 AUC, 82.93% sensitivity, and 85.00% specificity); and f value threshold of 0.29 (0.644 AUC, 41.46% sensitivity, and 95.00 specificity) for distinction of nasopharyngeal carcinoma from lymphoma (28).
Prediction of human papilloma virus (HPV) status
HPV has recently emerged as a major causative risk factor for a subset of oropharyngeal SCC. The HPV-positive oropharyngeal SCC is related to sexual behavior; whereas, HPV-negative cancer is strongly associated with tobacco and alcohol use (51). The HPV-positive oropharyngeal SCC also has a different biology, and is associated with a better prognosis than HPV-negative SCC (52). Thus, in the updated eighth edition of the Cancer Staging Manual of the American Joint Committee on Cancer implemented in January 2018, the HPV-positive oropharyngeal SCC is staged separately from HPV-negative type (51).
The classical description of HPV-positive oropharyngeal cancer histology is non-keratinizing and basaloid differentiated SCC as opposed to keratinizing and poorly differentiated form which is commonly seen with HPV-negative forms (52). This histopathological difference has perhaps lent itself to the difference in ADC characteristics of HPV-positive versus HPV-negative oropharyngeal SCC (53-57). Recent studies have generally reported a lower mean ADC in primary lesion of HPV-positive oropharyngeal SCC compared to the HPV-negative form (Table 3). Chan et al. have also shown an ROC AUC of 0.8467 for the mean ADC values in primary oropharyngeal SCC for distinction of HPV status (53). Nakahira et al. has proposed a mean ADC cut-off value of 1.027 ×10−3 mm2/s for prediction of HPV status in oropharyngeal SCC with 80.77% accuracy, 83.33% sensitivity, and 78.57% specificity (56).
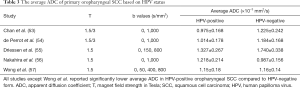
Full table
If validated and standardized, the quantitative ADC analysis can offer a non-invasive imaging biomarker for HPV status of oropharyngeal SCC. This is particularly important since distinction of HPV status dictates the cancer staging and treatment strategy in patients with oropharyngeal SCC (51).
Histopathological and molecular biomarker correlates of ADC
In addition to prediction of HPV status, some studies have shown the application of quantitative diffusion MRI in evaluation of HNT microstructure and histopathological characteristics. Correlation studies have shown the association of quantitative ADC analysis with molecular biomarkers and histopathological findings in patient with SCC of head and neck.
Using a 3 T scanner with EPI DWI sequence and b-values of 0 and 800 s/mm2, Surov et al. reported the associations of combined PET and ADC parameters with histopathological features in head and neck SCC (58). They examined the correlation between ADC and PET standard uptake value (SUV) with expression of Ki-67, epidermal growth factor receptor (EGFR), vascular endothelial growth factor (VEGF), tumor suppressor gene protein p53, hypoxia-inducible factor (HIF)-1α, and cell count (58). There was a significant correlation between cellularity with SUV-max/ADC-min; moreover, in grade 1 and 2 tumors, SUV-max/ADC-min correlated with HIF-1α expression. In separate studies, Surov et al. also examined the ADC histogram correlates of different molecular biomarkers in head and neck SCC (59,60). In grade 1 and 2 tumors, the ADC mode correlated with Ki-67; whereas, in grade 3 tumors, Ki-67 correlated with all ADC parameters except ADC mode. Cellularity correlated with all ADC parameters except ADC-max; and total nucleic had inverse correlation with mean, median, minimum, 25th, and 90th percentile of ADC (59,60).
Using EPI DWI sequence with b-values of 0 and 800 s/mm2 (with undetermined magnet field strength), Meyer et al. also examined the ADC histogram correlates of Hif1-alpha, VEGF, EGFR, p53, p16, and Her 2 expression in patients with head and neck SCC (61). They found significant correlation between ADC-max with p53 expression and ADC mode with Her 2 expression (61). The p16 positive and p16 negative forms showed distinctive correlations between ADC histogram metrics with Hif1-alpha, P53, and VEGF expression (61).
These results promise a new role for quantitative diffusion metrics as imaging biomarkers for prediction of molecular biomarkers in patients with HNT. This is particularly important as we move toward application of personalized treatment strategies with chemotherapy agents targeting specific cellular receptors.
Risk stratification and prognostication
Many authors have examined the application of pretreatment ADC quantification for prediction of outcome in patients with head and neck SCC (62-68). The majority of these studies suggest that higher pretreatment ADC values in the primary SCC lesion are predictive of poor local control and treatment response (Table 4). Ohnishi et al. found that patients with an ADC value >0.79 ×10–3 mm2/s had a significantly lower local control rate (44%) than those with a low ADC value (100%, P=0.0019) (64). Ng et al. suggested an ADC value >1.14 ×10−3 mm2/s (P=0.003) as an independent prognostic factor for 3-year focal recurrence (66). Srinivasan et al. found that an ADC >1.15 ×10-3 mm2/s is associated with poor outcome after chemoradiation at 2-year follow-up (70). Notably, Lambrecht et al. found that primary lesion ADC values obtained with high b-values (500, 750 and 1,000 s/mm2) but not those with low b-values (0, 50 and 100 s/mm2) were predictive of treatment failure (65). On the other hand, Nakajo et al. found that low ADC values of primary lesion are associated with lower rate of 2-year disease free survival (69). Noij et al. also reported that lymph node ADC values at b value of1,000 s/mm2 were predictive of outcome, whereas primary lesion ADC values were not (71).
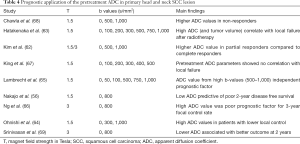
Full table
The IVIM studies also suggest that lower initial ADC and D values are predictive of positive response to treatment. Marzi et al. reported that head and neck SCC patients with regional disease control showed significantly lower pre-treatment D values compared to those with treatment failure (72). In separate studies, Guo et al. and Xiao-ping et al. found lower pretreatment ADC value, and D value in patients with response to therapy (19,27). Similarly, Xiao et al. reported lower baseline D value and higher DeltaD3, DeltaD21, DeltaD3*, DeltaD21*, and Deltaf21 in HNT lesions with positive response to treatment (26). In a cohort of HPV-positive oropharyngeal SCC, Ding et al. also found lower pretreatment ADC value, and D in lesions with complete response (18). However, Hauser et al. found significantly higher initial f value in patients with locoregional failure while the initial diffusion coefficient D was not significantly different between those with locoregional failure versus control (20).
Histopathological and molecular features such as high stromal content, low cellularity (lower proliferation), micronecrosis and negative HPV status are associated with resistance to treatment and poor outcome in patients with head and neck SCC (54,55,73,74). These prognostic characteristics are also associated with higher ADC and likely contribute to association of pretreatment high ADC (and D) values with poor outcome in patients with head and neck SCC. In the future, standardized quantitative diffusion MR metrics may be included in prognostic staging schemes of HNT to improve risk stratification and treatment planning.
Treatment response monitoring
After the start of chemotherapy and/or radiation therapy, it is desirable to monitor treatment response, and tailor further therapy to the individual patient. Quantitative diffusion MRI can evaluate the ADC or D value changes during the treatment, and predict therapy response. Notably, King et al. found no correlation between pretreatment ADC parameters with local failure (Table 4), while primary head and neck SCC with local failure had a lower percentage raise in the mean ADC, higher skewness, and higher kurtosis during treatment, compared with those with local control (67).
The cumulative studies have reported a rise in ADC values of primary head and neck SCC tumors in the first few weeks after the start of treatment (62,67,69,75). These studies suggest that a smaller percentage rise (<14–25%) in the mean ADC values within the first to third weeks after the therapy initiation is predictive of treatment failure (62,67,69,75,76). For example, using a 1.5 T scanner and SS EPI sequence with b-values of 0, 90 and 800 s/mm2, Matoba et al. found that fractional change in primary lesion and nodal ADC at pretreatment and 3 weeks after the start of treatment along with primary tumor volume, nodal volume, nodal stage, and tumor location had significant effect on locoregional failure and locoregional control (75). Primary lesion ADC factional change greater than 0.24 was predictive of locoregional control in their cohort (75). On the other hand, using 1.5 T scanner with spine echo EPI sequence and b-values of 50, 400 and 800 s/mm2, Wong et al. found a tendency toward a larger but statistically nonsignificant increase in ADC values after the first and second rounds of induction chemotherapy (57).
Also in an IVIM study by Xiao-ping et al., the percentage change of D value >25.25% within 20 days of induction treatment had an AUC of 0.859, sensitivity of 94.4%, and specificity of 76.5% to predict response to treatment as opposed to pretreatment ADC threshold of >0.879 ×10−3 mm2/s with an AUC of 0.758, sensitivity of 76.5%, and specificity of 72.2%; and pretreatment D threshold of >0.711 ×10−3 mm2/s with an AUC of 0.765, sensitivity of 64.7%, and specificity of 72.2% (27). Similarly, Paudyal et al. found that changes in D (within 3 weeks of treatment) was significantly different between head and neck SCC patients with complete response compared to incomplete responders (77). They could also identify subcategories of HPV-positive head and neck SCC patients with greater sensitivity to radiotherapy (77). On the other hand, Marzi et al. found that at mid-treatment time point, the patients with regional failure showed significantly higher D values, exhibited larger percent reductions in f, and product D* × f from the baseline (72).
Evaluation of ADC fractional changes in head and neck SCC is promising since the ratio changes could be more reproducible across different scanners and techniques compared to absolute ADC values. However, it should be noted that the optimal timing interval for early intra-treatment assessment of ADC values needs to be established given that development of post treatment mature scar tissues may “falsely” decrease ADC in patients with positive response to treatment (7,78). In the future prospective trials, serial quantitative assessment of diffusion MRI scans can be applied for close monitoring of treatment response in the early therapy phase and to guide personalized treatment decisions in patients with HNT.
Post treatment changes
Distinction of posttreatment fibrosis and granulation tissue from residual or recurrent tumor can be challenging (79). Quantitative analysis of diffusion MRI metrics can help distinguish between residual/recurrent malignant tissue and benign posttreatment changes similar to its application for distinction of primary malignant and benign lesions. Prior studies suggest that residual head and neck SCC tends to have lower average ADC compared to benign posttreatment tissue with optimal average ADC threshold of 1.3 to 1.4 ×10−3 mm2/s for distinction of residual tumor (80-82), yielding 71–95.5% accuracy, 45–94.6% sensitivity, and 90–100% specificity (Table 5). These findings are likely reflective of difference in cellularity of malignant tissue compared to fibrotic posttreatment changes.
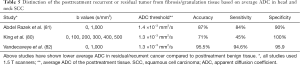
Full table
Applying IVIM, Lai et al. compared newly diagnosed nasopharyngeal carcinoma with biopsy-proven post-chemoradiation fibrosis (83). They found that D and f were significantly lower in nasopharyngeal carcinoma than in posttreatment fibrosis; whereas, D* was significantly higher in tumoral tissue compares to fibrosis (83). Their proposed cut-off values and corresponding accuracy, sensitivity, and specificity were: D of 1.062 ×10-3 mm2/s (100%, 100%, and 100%); f of 0.132 (78.3%, 66.0%, 100%); and D* of 85.283 ×10-3 mm2/s (96.4%, 100%, 90.7%), respectively (83).
Challenges and limitations
Despite the proven value of quantitative diffusion MRI metrics for diagnosis, differentiation, and prognostication of HNT, its integration into clinical practice remains limited. The most obvious limitation in application of quantitative measures is the variety of b-values, pulse sequences, field strength, and imaging protocols that can influence ADC quantification. It will be cumbersome to validate ADC values for clinical use while different machines and imaging protocols yield various results. Lesion segmentation and selection of region of interest for quantification are another source of heterogeneity in reported results; for example, the ADC values of tumor lesion or metastatic lymph node are not easily comparable between different studies, when they consist of both highly cellular malignant component and poorly cellular necrotic portions. Image quality can also be an issue, as Schakel et al. have also reported severe distortions of DWI scans—up to centimeters—that can affect tumor volume measurements (84). Notably, Vidiri et al. suggested that reduced field of view can increase the accuracy of ADC value measurements (85).
Summary
Despite heterogeneity of prior studies in terms of applied b-values, imaging protocols, outcome measurements, and reference standards, the quantitative analysis of diffusion MRI proves to offer far ranging potential applications in patients with head and neck cancer. Quantitative analysis of diffusion metrics can help in distinguishing benign from malignant lesions, predicting tumor response to treatment based on pretreatment characteristics, monitoring and assessing the response to treatment as therapy progresses, and surveilling areas of prior treatment to detect posttreatment and recurrent malignant tissue. Overall, malignant lesions tend to have lower ADC values compared to benign lesions. In addition, ADC values can help with differentiation of potential HNT; for example, among salivary gland neoplasms, Warthin tumors have very low ADC values, and pleomorphic adenomas have very high ADC values, with carcinomas demonstrating midrange ADC values. Similarly, ADC values can distinguish lymphomatous, metastatic, and benign lymphadenopathy from each other (in order of increasing average ADC values). In addition, lower average ADC in primary lesion of patients with oropharyngeal SCC is predictive of positive HPV status. With regards to prediction of treatment response, a low ADC or D values in pretreatment lesions, and early interval increase in ADC and D values during the treatment are harbinger of favorable response to therapy and outcome. Eventually, for distinction of post-treatment changes from residual or recurrent head and neck SCC, the malignant tissue tends to demonstrate lower average ADC. Pending development of more standardized methods for image acquisition, quantitative calculation, and tissue segmentation, the diffusion MR metrics can be applied for HNT patients’ selection, personalized treatment planning, and response monitoring in prospective trials.
Acknowledgements
None.
Footnote
Conflicts of Interest: The author has no conflicts of interest to declare.
References
- Siegel RL, Miller KD, Jemal A. Cancer Statistics, 2017. CA Cancer J Clin 2017;67:7-30. [Crossref] [PubMed]
- Global Burden of Disease Cancer C, Fitzmaurice C, Allen C, Barber RM, Barregard L, Bhutta ZA, Brenner H, Dicker DJ, Chimed-Orchir O, Dandona R, Dandona L, Fleming T, Forouzanfar MH, Hancock J, Hay RJ, Hunter-Merrill R, Huynh C, Hosgood HD, Johnson CO, Jonas JB, Khubchandani J, Kumar GA, Kutz M, Lan Q, Larson HJ, Liang X, Lim SS, Lopez AD, MacIntyre MF, Marczak L, Marquez N, Mokdad AH, Pinho C, Pourmalek F, Salomon JA, Sanabria JR, Sandar L, Sartorius B, Schwartz SM, Shackelford KA, Shibuya K, Stanaway J, Steiner C, Sun J, Takahashi K, Vollset SE, Vos T, Wagner JA, Wang H, Westerman R, Zeeb H, Zoeckler L, Abd-Allah F, Ahmed MB, Alabed S, Alam NK, Aldhahri SF, Alem G, Alemayohu MA, Ali R, Al-Raddadi R, Amare A, Amoako Y, Artaman A, Asayesh H, Atnafu N, Awasthi A, Saleem HB, Barac A, Bedi N, Bensenor I, Berhane A, Bernabe E, Betsu B, Binagwaho A, Boneya D, Campos-Nonato I, Castaneda-Orjuela C, Catala-Lopez F, Chiang P, Chibueze C, Chitheer A, Choi JY, Cowie B, Damtew S, das Neves J, Dey S, Dharmaratne S, Dhillon P, Ding E, Driscoll T, Ekwueme D, Endries AY, Farvid M, Farzadfar F, Fernandes J, Fischer F, TT GH, Gebru A, Gopalani S, Hailu A, Horino M, Horita N, Husseini A, Huybrechts I, Inoue M, Islami F, Jakovljevic M, James S, Javanbakht M, Jee SH, Kasaeian A, Kedir MS, Khader YS, Khang YH, Kim D, Leigh J, Linn S, Lunevicius R, El Razek HMA, Malekzadeh R, Malta DC, Marcenes W, Markos D, Melaku YA, Meles KG, Mendoza W, Mengiste DT, Meretoja TJ, Miller TR, Mohammad KA, Mohammadi A, Mohammed S, Moradi-Lakeh M, Nagel G, Nand D, Le Nguyen Q, Nolte S, Ogbo FA, Oladimeji KE, Oren E, Pa M, Park EK, Pereira DM, Plass D, Qorbani M, Radfar A, Rafay A, Rahman M, Rana SM, Soreide K, Satpathy M, Sawhney M, Sepanlou SG, Shaikh MA, She J, Shiue I, Shore HR, Shrime MG, So S, Soneji S, Stathopoulou V, Stroumpoulis K, Sufiyan MB, Sykes BL, Tabares-Seisdedos R, Tadese F, Tedla BA, Tessema GA, Thakur JS, Tran BX, Ukwaja KN, Uzochukwu BSC, Vlassov VV, Weiderpass E, Wubshet Terefe M, Yebyo HG, Yimam HH, Yonemoto N, Younis MZ, Yu C, Zaidi Z, Zaki MES, Zenebe ZM, Murray CJL, Naghavi M. Global, Regional, and National Cancer Incidence, Mortality, Years of Life Lost, Years Lived With Disability, and Disability-Adjusted Life-years for 32 Cancer Groups, 1990 to 2015: A Systematic Analysis for the Global Burden of Disease Study. JAMA Oncol 2017;3:524-48.
- Gupta B, Johnson NW, Kumar N. Global Epidemiology of Head and Neck Cancers: A Continuing Challenge. Oncology 2016;91:13-23. [Crossref] [PubMed]
- Noij DP, Martens RM, Marcus JT, de Bree R, Leemans CR, Castelijns JA, de Jong MC, de Graaf P. Intravoxel incoherent motion magnetic resonance imaging in head and neck cancer: A systematic review of the diagnostic and prognostic value. Oral Oncol 2017;68:81-91. [Crossref] [PubMed]
- Jansen JFA, Parra C, Lu Y, Shukla-Dave A. Evaluation of Head and Neck Tumors with Functional MR Imaging. Magn Reson Imaging Clin N Am 2016;24:123-33. [Crossref] [PubMed]
- Thoeny HC, De Keyzer F, King AD. Diffusion-weighted MR imaging in the head and neck. Radiology 2012;263:19-32. [Crossref] [PubMed]
- Wong KH, Panek R, Bhide SA, Nutting CM, Harrington KJ, Newbold KL. The emerging potential of magnetic resonance imaging in personalizing radiotherapy for head and neck cancer: an oncologist's perspective. Br J Radiol 2017;90:20160768. [Crossref] [PubMed]
- Lu Y, Jansen JF, Mazaheri Y, Stambuk HE, Koutcher JA, Shukla-Dave A. Extension of the intravoxel incoherent motion model to non-gaussian diffusion in head and neck cancer. J Magn Reson Imaging 2012;36:1088-96. [Crossref] [PubMed]
- Yuan J, Yeung DK, Mok GS, Bhatia KS, Wang YX, Ahuja AT, King AD. Non-Gaussian analysis of diffusion weighted imaging in head and neck at 3T: a pilot study in patients with nasopharyngeal carcinoma. PLoS One 2014;9:e87024. [Crossref] [PubMed]
- Sasaki M, Sumi M, Eida S, Katayama I, Hotokezaka Y, Nakamura T. Simple and reliable determination of intravoxel incoherent motion parameters for the differential diagnosis of head and neck tumors. PLoS One 2014;9:e112866. [Crossref] [PubMed]
- Widmann G, Henninger B, Kremser C, Jaschke W. MRI Sequences in Head & Neck Radiology - State of the Art. Rofo 2017;189:413-22. [Crossref] [PubMed]
- Fujima N, Yoshida D, Sakashita T, Homma A, Tsukahara A, Tha KK, Kudo K, Shirato H. Intravoxel incoherent motion diffusion-weighted imaging in head and neck squamous cell carcinoma: assessment of perfusion-related parameters compared to dynamic contrast-enhanced MRI. Magn Reson Imaging 2014;32:1206-13. [Crossref] [PubMed]
- Le Bihan D, Breton E, Lallemand D, Aubin ML, Vignaud J, Laval-Jeantet M. Separation of diffusion and perfusion in intravoxel incoherent motion MR imaging. Radiology 1988;168:497-505. [Crossref] [PubMed]
- Jansen JF, Stambuk HE, Koutcher JA, Shukla-Dave A. Non-gaussian analysis of diffusion-weighted MR imaging in head and neck squamous cell carcinoma: A feasibility study. AJNR Am J Neuroradiol 2010;31:741-8. [Crossref] [PubMed]
- Lemke A, Stieltjes B, Schad LR, Laun FB. Toward an optimal distribution of b values for intravoxel incoherent motion imaging. Magn Reson Imaging 2011;29:766-76. [Crossref] [PubMed]
- Gurney-Champion OJ, Froeling M, Klaassen R, Runge JH, Bel A, van Laarhoven HW, Stoker J, Nederveen AJ. Minimizing the Acquisition Time for Intravoxel Incoherent Motion Magnetic Resonance Imaging Acquisitions in the Liver and Pancreas. Invest Radiol 2016;51:211-20. [Crossref] [PubMed]
- Dikaios N, Punwani S, Hamy V, Purpura P, Rice S, Forster M, Mendes R, Taylor S, Atkinson D. Noise estimation from averaged diffusion weighted images: Can unbiased quantitative decay parameters assist cancer evaluation? Magn Reson Med 2014;71:2105-17. [Crossref] [PubMed]
- Ding Y, Hazle JD, Mohamed AS, Frank SJ, Hobbs BP, Colen RR, Gunn GB, Wang J, Kalpathy-Cramer J, Garden AS, Lai SY, Rosenthal DI, Fuller CD. Intravoxel incoherent motion imaging kinetics during chemoradiotherapy for human papillomavirus-associated squamous cell carcinoma of the oropharynx: preliminary results from a prospective pilot study. NMR Biomed 2015;28:1645-54. [Crossref] [PubMed]
- Guo W, Luo D, Lin M, Wu B, Li L, Zhao Y, Yang L, Zhou C. Pretreatment Intra-Voxel Incoherent Motion Diffusion-Weighted Imaging (IVIM-DWI) in Predicting Induction Chemotherapy Response in Locally Advanced Hypopharyngeal Carcinoma. Medicine (Baltimore) 2016;95:e3039. [Crossref] [PubMed]
- Hauser T, Essig M, Jensen A, Laun FB, Munter M, Maier-Hein KH, Stieltjes B. Prediction of treatment response in head and neck carcinomas using IVIM-DWI: Evaluation of lymph node metastasis. Eur J Radiol 2014;83:783-7. [Crossref] [PubMed]
- Lai V, Li X, Lee VH, Lam KO, Fong DY, Huang B, Chan Q, Khong PL. Nasopharyngeal carcinoma: comparison of diffusion and perfusion characteristics between different tumour stages using intravoxel incoherent motion MR imaging. Eur Radiol 2014;24:176-83. [Crossref] [PubMed]
- Lu Y, Jansen JF, Stambuk HE, Gupta G, Lee N, Gonen M, Moreira A, Mazaheri Y, Patel SG, Deasy JO, Shah JP, Shukla-Dave A. Comparing primary tumors and metastatic nodes in head and neck cancer using intravoxel incoherent motion imaging: a preliminary experience. J Comput Assist Tomogr 2013;37:346-52. [Crossref] [PubMed]
- Marzi S, Piludu F, Vidiri A. Assessment of diffusion parameters by intravoxel incoherent motion MRI in head and neck squamous cell carcinoma. NMR Biomed 2013;26:1806-14. [Crossref] [PubMed]
- Sakamoto J, Imaizumi A, Sasaki Y, Kamio T, Wakoh M, Otonari-Yamamoto M, Sano T. Comparison of accuracy of intravoxel incoherent motion and apparent diffusion coefficient techniques for predicting malignancy of head and neck tumors using half-Fourier single-shot turbo spin-echo diffusion-weighted imaging. Magn Reson Imaging 2014;32:860-6. [Crossref] [PubMed]
- Sumi M, Van Cauteren M, Sumi T, Obara M, Ichikawa Y, Nakamura T. Salivary gland tumors: use of intravoxel incoherent motion MR imaging for assessment of diffusion and perfusion for the differentiation of benign from malignant tumors. Radiology 2012;263:770-7. [Crossref] [PubMed]
- Xiao Y, Pan J, Chen Y, Chen Y, He Z, Zheng X. Intravoxel Incoherent Motion-Magnetic Resonance Imaging as an Early Predictor of Treatment Response to Neoadjuvant Chemotherapy in Locoregionally Advanced Nasopharyngeal Carcinoma. Medicine (Baltimore) 2015;94:e973. [Crossref] [PubMed]
- Xiao-ping Y, Jing H, Fei-ping L, Yin H, Qiang L, Lanlan W, Wei W. Intravoxel incoherent motion MRI for predicting early response to induction chemotherapy and chemoradiotherapy in patients with nasopharyngeal carcinoma. J Magn Reson Imaging 2016;43:1179-90. [Crossref] [PubMed]
- Yu XP, Hou J, Li FP, Wang H, Hu PS, Bi F, Wang W. Intravoxel Incoherent Motion Diffusion Weighted Magnetic Resonance Imaging for Differentiation Between Nasopharyngeal Carcinoma and Lymphoma at the Primary Site. J Comput Assist Tomogr 2016;40:413-8. [Crossref] [PubMed]
- Zhang SX, Jia QJ, Zhang ZP, Liang CH, Chen WB, Qiu QH, Li H. Intravoxel incoherent motion MRI: emerging applications for nasopharyngeal carcinoma at the primary site. Eur Radiol 2014;24:1998-2004. [Crossref] [PubMed]
- Bammer R. Basic principles of diffusion-weighted imaging. Eur J Radiol 2003;45:169-84. [Crossref] [PubMed]
- White ML, Zhang Y, Robinson RA. Evaluating tumors and tumorlike lesions of the nasal cavity, the paranasal sinuses, and the adjacent skull base with diffusion-weighted MRI. J Comput Assist Tomogr 2006;30:490-5. [Crossref] [PubMed]
- Dubrulle F, Souillard R, Chechin D, Vaneecloo FM, Desaulty A, Vincent C. Diffusion-weighted MR imaging sequence in the detection of postoperative recurrent cholesteatoma. Radiology 2006;238:604-10. [Crossref] [PubMed]
- Mas-Estelles F, Mateos-Fernandez M, Carrascosa-Bisquert B, Facal de Castro F, Puchades-Roman I, Morera-Perez C. Contemporary non-echo-planar diffusion-weighted imaging of middle ear cholesteatomas. Radiographics 2012;32:1197-213. [Crossref] [PubMed]
- Connolly M, Srinivasan A. Diffusion-Weighted Imaging in Head and Neck Cancer: Technique, Limitations, and Applications. Magn Reson Imaging Clin N Am 2018;26:121-33. [Crossref] [PubMed]
- Sakamoto J, Yoshino N, Okochi K, Imaizumi A, Tetsumura A, Kurohara K, Kurabayashi T. Tissue characterization of head and neck lesions using diffusion-weighted MR imaging with SPLICE. Eur J Radiol 2009;69:260-8. [Crossref] [PubMed]
- Srinivasan A, Dvorak R, Perni K, Rohrer S, Mukherji SK. Differentiation of benign and malignant pathology in the head and neck using 3T apparent diffusion coefficient values: early experience. AJNR Am J Neuroradiol 2008;29:40-4. [Crossref] [PubMed]
- Seethala RR. LiVolsi VA, Baloch ZW. Relative accuracy of fine-needle aspiration and frozen section in the diagnosis of lesions of the parotid gland. Head Neck 2005;27:217-23. [Crossref] [PubMed]
- Yabuuchi H, Matsuo Y, Kamitani T, Setoguchi T, Okafuji T, Soeda H, Sakai S, Hatakenaka M, Nakashima T, Oda Y, Honda H. Parotid gland tumors: can addition of diffusion-weighted MR imaging to dynamic contrast-enhanced MR imaging improve diagnostic accuracy in characterization? Radiology 2008;249:909-16. [Crossref] [PubMed]
- Habermann CR, Gossrau P, Graessner J, Arndt C, Cramer MC, Reitmeier F, Jaehne M, Adam G. Diffusion-weighted echo-planar MRI: a valuable tool for differentiating primary parotid gland tumors? Rofo 2005;177:940-5. [Crossref] [PubMed]
- Ikeda M, Motoori K, Hanazawa T, Nagai Y, Yamamoto S, Ueda T, Funatsu H, Ito H. Warthin tumor of the parotid gland: diagnostic value of MR imaging with histopathologic correlation. AJNR Am J Neuroradiol 2004;25:1256-62. [PubMed]
- Kikuchi M, Haga B, Morihisa K. Gan No Rinsho 1971;17:873-6. [Mixed tumor of the parotid gland with metastasis to the rib]. [PubMed]
- Razek AA, Sadek AG, Kombar OR, Elmahdy TE, Nada N. Role of apparent diffusion coefficient values in differentiation between malignant and benign solitary thyroid nodules. AJNR Am J Neuroradiol 2008;29:563-8. [Crossref] [PubMed]
- Erdem G, Erdem T, Muammer H, Mutlu DY, Firat AK, Sahin I, Alkan A. Diffusion-weighted images differentiate benign from malignant thyroid nodules. J Magn Reson Imaging 2010;31:94-100. [Crossref] [PubMed]
- Bozgeyik Z, Coskun S, Dagli AF, Ozkan Y, Sahpaz F, Ogur E. Diffusion-weighted MR imaging of thyroid nodules. Neuroradiology 2009;51:193-8. [Crossref] [PubMed]
- Payabvash S, Meric K, Cayci Z. Differentiation of benign from malignant cervical lymph nodes in patients with head and neck cancer using PET/CT imaging. Clin Imaging 2016;40:101-5. [Crossref] [PubMed]
- Abdel Razek AA, Soliman NY, Elkhamary S, Alsharaway MK, Tawfik A. Role of diffusion-weighted MR imaging in cervical lymphadenopathy. Eur Radiol 2006;16:1468-77. [Crossref] [PubMed]
- Holzapfel K, Duetsch S, Fauser C, Eiber M, Rummeny EJ, Gaa J. Value of diffusion-weighted MR imaging in the differentiation between benign and malignant cervical lymph nodes. Eur J Radiol 2009;72:381-7. [Crossref] [PubMed]
- Sumi M, Sakihama N, Sumi T, Morikawa M, Uetani M, Kabasawa H, Shigeno K, Hayashi K, Takahashi H, Nakamura T. Discrimination of metastatic cervical lymph nodes with diffusion-weighted MR imaging in patients with head and neck cancer. AJNR Am J Neuroradiol 2003;24:1627-34. [PubMed]
- Zhang Y, Chen J, Shen J, Zhong J, Ye R, Liang B. Apparent diffusion coefficient values of necrotic and solid portion of lymph nodes: differential diagnostic value in cervical lymphadenopathy. Clin Radiol 2013;68:224-31. [Crossref] [PubMed]
- Hejduk B, Bobek-Billewicz B, Rutkowski T, Hebda A, Zawadzka A, Jurkowski MK. Application of Intravoxel Incoherent Motion (IVIM) Model for Differentiation Between Metastatic and Non-Metastatic Head and Neck Lymph Nodes. Pol J Radiol 2017;82:506-10. [Crossref] [PubMed]
- Glastonbury CM, Mukherji SK, O'Sullivan B, Lydiatt WM. Setting the Stage for 2018: How the Changes in the American Joint Committee on Cancer/Union for International Cancer Control Cancer Staging Manual Eighth Edition Impact Radiologists. AJNR Am J Neuroradiol 2017;38:2231-7.
- Cooper T, Biron V, Adam B, Klimowicz AC, Puttagunta L, Seikaly H. Prognostic utility of basaloid differentiation in oropharyngeal cancer. J Otolaryngol Head Neck Surg 2013;42:57. [Crossref] [PubMed]
- Chan MW, Higgins K, Enepekides D, Poon I, Symons SP, Moineddin R, Weinreb I, Shearkhani O, Chen A, Beelen J, Chan A, Maralani PJ. Radiologic Differences between Human Papillomavirus-Related and Human Papillomavirus-Unrelated Oropharyngeal Carcinoma on Diffusion-Weighted Imaging. ORL J Otorhinolaryngol Relat Spec 2016;78:344-52. [Crossref] [PubMed]
- de Perrot T, Lenoir V, Domingo Ayllon M, Dulguerov N, Pusztaszeri M, Becker M. Apparent Diffusion Coefficient Histograms of Human Papillomavirus-Positive and Human Papillomavirus-Negative Head and Neck Squamous Cell Carcinoma: Assessment of Tumor Heterogeneity and Comparison with Histopathology. AJNR Am J Neuroradiol 2017;38:2153-60. [Crossref] [PubMed]
- Driessen JP, van Bemmel AJ, van Kempen PM, Janssen LM, Terhaard CH, Pameijer FA, Willems SM, Stegeman I, Grolman W, Philippens ME. Correlation of human papillomavirus status with apparent diffusion coefficient of diffusion-weighted MRI in head and neck squamous cell carcinomas. Head Neck 2016;38 Suppl 1:E613-8. [Crossref] [PubMed]
- Nakahira M, Saito N, Yamaguchi H, Kuba K, Sugasawa M. Use of quantitative diffusion-weighted magnetic resonance imaging to predict human papilloma virus status in patients with oropharyngeal squamous cell carcinoma. Eur Arch Otorhinolaryngol 2014;271:1219-25. [Crossref] [PubMed]
- Wong KH, Panek R, Welsh L, McQuaid D, Dunlop A, Riddell A, Murray I, Du Y, Chua S, Koh DM, Bhide S, Nutting C, Oyen WJ, Harrington K, Newbold KL. The Predictive Value of Early Assessment After 1 Cycle of Induction Chemotherapy with 18F-FDG PET/CT and Diffusion-Weighted MRI for Response to Radical Chemoradiotherapy in Head and Neck Squamous Cell Carcinoma. J Nucl Med 2016;57:1843-50. [Crossref] [PubMed]
- Surov A, Meyer HJ, Hohn AK, Sabri O, Purz S. Combined Metabolo-Volumetric Parameters of (18)F-FDG-PET and MRI Can Predict Tumor Cellularity, Ki67 Level and Expression of HIF 1alpha in Head and Neck Squamous Cell Carcinoma: A Pilot Study. Transl Oncol 2018;12:8-14. [Crossref] [PubMed]
- Surov A, Meyer HJ, Wienke A. Can Imaging Parameters Provide Information Regarding Histopathology in Head and Neck Squamous Cell Carcinoma? A Meta-Analysis. Transl Oncol 2018;11:498-503. [Crossref] [PubMed]
- Surov A, Meyer HJ, Winter K, Richter C, Hoehn AK. Histogram analysis parameters of apparent diffusion coefficient reflect tumor cellularity and proliferation activity in head and neck squamous cell carcinoma. Oncotarget 2018;9:23599-607. [PubMed]
- Meyer HJ, Leifels L, Hamerla G, Hohn AK, Surov A. ADC-histogram analysis in head and neck squamous cell carcinoma. Associations with different histopathological features including expression of EGFR, VEGF, HIF-1alpha, Her 2 and p53. A preliminary study. Magn Reson Imaging 2018;54:214-7. [Crossref] [PubMed]
- Kim S, Loevner L, Quon H, Sherman E, Weinstein G, Kilger A, Poptani H. Diffusion-weighted magnetic resonance imaging for predicting and detecting early response to chemoradiation therapy of squamous cell carcinomas of the head and neck. Clin Cancer Res 2009;15:986-94. [Crossref] [PubMed]
- Hatakenaka M, Shioyama Y, Nakamura K, Yabuuchi H, Matsuo Y, Sunami S, Kamitani T, Yoshiura T, Nakashima T, Nishikawa K, Honda H. Apparent diffusion coefficient calculated with relatively high b-values correlates with local failure of head and neck squamous cell carcinoma treated with radiotherapy. AJNR Am J Neuroradiol 2011;32:1904-10. [Crossref] [PubMed]
- Ohnishi K, Shioyama Y, Hatakenaka M, Nakamura K, Abe K, Yoshiura T, Ohga S, Nonoshita T, Yoshitake T, Nakashima T, Honda H. Prediction of local failures with a combination of pretreatment tumor volume and apparent diffusion coefficient in patients treated with definitive radiotherapy for hypopharyngeal or oropharyngeal squamous cell carcinoma. J Radiat Res 2011;52:522-30. [Crossref] [PubMed]
- Lambrecht M, Van Calster B, Vandecaveye V, De Keyzer F, Roebben I, Hermans R, Nuyts S. Integrating pretreatment diffusion weighted MRI into a multivariable prognostic model for head and neck squamous cell carcinoma. Radiother Oncol 2014;110:429-34. [Crossref] [PubMed]
- Ng SH, Lin CY, Chan SC, Lin YC, Yen TC, Liao CT, Chang JT, Ko SF, Wang HM, Chang CJ, Wang JJ. Clinical utility of multimodality imaging with dynamic contrast-enhanced MRI, diffusion-weighted MRI, and 18F-FDG PET/CT for the prediction of neck control in oropharyngeal or hypopharyngeal squamous cell carcinoma treated with chemoradiation. PLoS One 2014;9:e115933. [Crossref] [PubMed]
- King AD, Chow KK, Yu KH, Mo FK, Yeung DK, Yuan J, Bhatia KS, Vlantis AC, Ahuja AT. Head and neck squamous cell carcinoma: diagnostic performance of diffusion-weighted MR imaging for the prediction of treatment response. Radiology 2013;266:531-8. [Crossref] [PubMed]
- Chawla S, Kim S, Dougherty L, Wang S, Loevner LA, Quon H, Poptani H. Pretreatment diffusion-weighted and dynamic contrast-enhanced MRI for prediction of local treatment response in squamous cell carcinomas of the head and neck. AJR Am J Roentgenol 2013;200:35-43. [Crossref] [PubMed]
- Nakajo M, Nakajo M, Kajiya Y, Tani A, Kamiyama T, Yonekura R, Fukukura Y, Matsuzaki T, Nishimoto K, Nomoto M, Koriyama C. FDG PET/CT and diffusion-weighted imaging of head and neck squamous cell carcinoma: comparison of prognostic significance between primary tumor standardized uptake value and apparent diffusion coefficient. Clin Nucl Med 2012;37:475-80. [Crossref] [PubMed]
- Srinivasan A, Chenevert TL, Dwamena BA, Eisbruch A, Watcharotone K, Myles JD, Mukherji SK. Utility of pretreatment mean apparent diffusion coefficient and apparent diffusion coefficient histograms in prediction of outcome to chemoradiation in head and neck squamous cell carcinoma. J Comput Assist Tomogr 2012;36:131-7. [Crossref] [PubMed]
- Noij DP, Pouwels PJW, Ljumanovic R, Knol DL, Doornaert P, de Bree R, Castelijns JA, de Graaf P. Predictive value of diffusion-weighted imaging without and with including contrast-enhanced magnetic resonance imaging in image analysis of head and neck squamous cell carcinoma. Eur J Radiol 2015;84:108-16. [Crossref] [PubMed]
- Marzi S, Piludu F, Sanguineti G, Marucci L, Farneti A, Terrenato I, Pellini R, Benevolo M, Covello R, Vidiri A. The prediction of the treatment response of cervical nodes using intravoxel incoherent motion diffusion-weighted imaging. Eur J Radiol 2017;92:93-102. [Crossref] [PubMed]
- King AD, Thoeny HC. Functional MRI for the prediction of treatment response in head and neck squamous cell carcinoma: potential and limitations. Cancer Imaging 2016;16:23. [Crossref] [PubMed]
- Driessen JP, Caldas-Magalhaes J, Janssen LM, Pameijer FA, Kooij N, Terhaard CH, Grolman W, Philippens ME. Diffusion-weighted MR imaging in laryngeal and hypopharyngeal carcinoma: association between apparent diffusion coefficient and histologic findings. Radiology 2014;272:456-63. [Crossref] [PubMed]
- Matoba M, Tuji H, Shimode Y, Toyoda I, Kuginuki Y, Miwa K, Tonami H. Fractional change in apparent diffusion coefficient as an imaging biomarker for predicting treatment response in head and neck cancer treated with chemoradiotherapy. AJNR Am J Neuroradiol 2014;35:379-85. [Crossref] [PubMed]
- Vandecaveye V, Dirix P, De Keyzer F, Op de Beeck K, Vander Poorten V, Hauben E, Lambrecht M, Nuyts S, Hermans R. Diffusion-weighted magnetic resonance imaging early after chemoradiotherapy to monitor treatment response in head-and-neck squamous cell carcinoma. Int J Radiat Oncol Biol Phys 2012;82:1098-107. [Crossref] [PubMed]
- Paudyal R, Oh JH, Riaz N, Venigalla P, Li J, Hatzoglou V, Leeman J, Nunez DA, Lu Y, Deasy JO, Lee N, Shukla-Dave A. Intravoxel incoherent motion diffusion-weighted MRI during chemoradiation therapy to characterize and monitor treatment response in human papillomavirus head and neck squamous cell carcinoma. J Magn Reson Imaging 2017;45:1013-23. [Crossref] [PubMed]
- Padhani AR, Koh DM, Collins DJ. Whole-body diffusion-weighted MR imaging in cancer: current status and research directions. Radiology 2011;261:700-18. [Crossref] [PubMed]
- de Bree R, Castelijns JA, Hoekstra OS, Leemans CR. Advances in imaging in the work-up of head and neck cancer patients. Oral Oncol 2009;45:930-5. [Crossref] [PubMed]
- King AD, Mo FK, Yu KH, Yeung DK, Zhou H, Bhatia KS, Tse GM, Vlantis AC, Wong JK, Ahuja AT. Squamous cell carcinoma of the head and neck: diffusion-weighted MR imaging for prediction and monitoring of treatment response. Eur Radiol 2010;20:2213-20. [Crossref] [PubMed]
- Abdel Razek AA, Kandeel AY, Soliman N, El-shenshawy HM, Kamel Y, Nada N, Denewar A. Role of diffusion-weighted echo-planar MR imaging in differentiation of residual or recurrent head and neck tumors and posttreatment changes. AJNR Am J Neuroradiol 2007;28:1146-52. [Crossref] [PubMed]
- Vandecaveye V, De Keyzer F, Nuyts S, Deraedt K, Dirix P, Hamaekers P, Vander Poorten V, Delaere P, Hermans R. Detection of head and neck squamous cell carcinoma with diffusion weighted MRI after (chemo)radiotherapy: correlation between radiologic and histopathologic findings. Int J Radiat Oncol Biol Phys 2007;67:960-71. [Crossref] [PubMed]
- Lai V, Li X, Lee VH, Lam KO, Chan Q, Khong PL. Intravoxel incoherent motion MR imaging: comparison of diffusion and perfusion characteristics between nasopharyngeal carcinoma and post-chemoradiation fibrosis. Eur Radiol 2013;23:2793-801. [Crossref] [PubMed]
- Schakel T, Hoogduin JM, Terhaard CH, Philippens ME. Diffusion weighted MRI in head-and-neck cancer: geometrical accuracy. Radiother Oncol 2013;109:394-7. [Crossref] [PubMed]
- Vidiri A, Minosse S, Piludu F, Curione D, Pichi B, Spriano G, Marzi S. Feasibility study of reduced field of view diffusion-weighted magnetic resonance imaging in head and neck tumors. Acta Radiol 2017;58:292-300. [Crossref] [PubMed]