Diagnostic performance of artificial intelligence in interpreting thyroid nodules on ultrasound images: a multicenter retrospective study
Introduction
Thyroid nodules, although often benign growths in the thyroid gland, can occasionally suggest underlying malignancies. These nodules are widespread in the general population, found in 19–68% of individuals (1). Alarmingly, about 3–10% result in a thyroid cancer diagnosis (2). Over recent decades, thyroid cancer incidence has significantly risen (3), making it the fifth most common cancer in women worldwide (4). In Thailand, it occupies an even higher rank, being the fourth most diagnosed cancer among women (5). Considering these trends, discussions around the potential benefits and controversies associated with the early and accurate detection of thyroid nodules for cancer prevention and survival rates warrant further exploration (6).
Ultrasonography stands as the primary modality for detecting and characterizing thyroid nodules, informing decisions on fine needle aspiration (FNA). Ultrasonography’s prevalence in clinical practice stems from its non-invasiveness, absence of radiation, cost-effectiveness, and convenience. Additionally, ultrasonography enables concurrent interventions (7). However, the efficacy of ultrasound-based diagnose is hinges not just on image quality or equipment, but significantly on the expertise of the interpreting radiologist. Those less experienced often face higher misdiagnosis rates, unintentionally increasing unnecessary FNA procedures (8).
There has been a consistent push towards refining diagnostic accuracy in this field. Wang et al. utilized ultrasound elastography images to study thyroid microcarcinoma (9). Luo et al. took an innovative approach to classifying thyroid nodules, integrating linear discriminant analysis with elastography images (10). Despite their promise, ultrasound elastography images present their own set of challenges, with various factors possibly affecting their reliability (11). Over time, numerous classification systems for thyroid nodule risk using conventional ultrasound images have emerged. However, concerns remain about their effectiveness due to inherent inconsistencies (12). Pioneers like Liang et al. developed a unique radiomics score aimed at predicting thyroid nodule malignancies, showcasing superior performance over existing methods but requiring manual delineation of regions of interest (13). Concurrently, works by Thomas and Haertling utilized the image similarity model by Google to draw parallels between histological features in thyroid cancer diagnosis based on ultrasound images (14).
In a bid to further enhance diagnosis, Ma et al. introduced a convolutional neural networks (CNN)-based model for thyroid nodule diagnosis, combining two distinct CNN architectures to generate a richer feature map, thus enhancing predictive accuracy (15). Gao et al. adopted a multi-scale CNN approach for thyroid cancer differentiation, harnessing the pre-trained Alexnet’s capabilities (16,17). Additionally, the introduction of the Faster Region-based Convolutional Network for extracting regions of interest (ROIs) from ultrasound images offered a new perspective on malignancy prediction (18,19). Other studies like those by Liu et al. incorporated an Artificial Neural Network, drawing insights from the gray-level co-occurrence matrix and principal component analysis (20). Zhu et al. employed the pretrained VGG-19 model to diagnose thyroid and breast cancers, achieving commendable accuracy rates (21). Cumulatively, these studies signify AI’s transformative potential in enhancing diagnostic precision and mitigating subjective errors, ultimately leading to more accurate patient treatment plans and reduced healthcare costs.
Our study presents a novel “AI” system for thyroid nodule assessment, improving automation and accuracy. Using advanced AI techniques, it predicts malignancy in ultrasound images, mirroring practices from Thai medical centers. Departing from manual ROI segmentation, we employ You Only Look Once (YOLO) deep neural network for more precise and efficient segmentation. For malignancy prediction, we adopted CNNs, specifically using the pre-trained DenseNet121. Furthermore, we integrated the Weakly Supervised Data Augmentation Network (WSDAN) (22), finding it particularly adept at discerning nuanced features on ultrasound images. This model was trained using images from multiple centers in Thailand, including Siriraj Hospital, Vajira Hospital, and the HRH Princess Maha Chakri Sirindhorn Medical Center, resulting in a noticeable boost in our thyroid nodule classification metrics.
Given the outlined advancements and challenges in thyroid nodule diagnosis, the purpose of this study is to evaluate the efficacy of our novel artificial intelligence (AI) system in improving the accuracy of thyroid nodule malignancy detection in ultrasound images, aiming to reduce the reliance on subjective interpretation and decrease the rate of unnecessary FNA procedures. We present this article in accordance with the STARD reporting checklist (available at https://qims.amegroups.com/article/view/10.21037/qims-23-1650/rc).
Methods
In this section, we detail the materials and methods employed during our research, starting with the patient selection criteria, moving on to the methods for ultrasound image acquisition, followed by our data and statistical analysis approach, and concluding with the demographic data and ultrasound characteristics of the examined nodules.
Patients
For this retrospective analysis, we examined 600 nodules, each corresponding to an individual patient who underwent thyroid ultrasonography complemented by ultrasound-guided FNA across three prominent Thai medical institutions: Siriraj Hospital, Vajira Hospital, and HRH Princess Maha Chakri Sirindhorn Medical Center during the period from January 2015 to March 2021. To ensure a comprehensive and unbiased dataset, patients were selected through a consecutive series approach, meaning all eligible patients who presented during the specified timeframe and met the inclusion criteria were considered for the study without omission.
This study was conducted in accordance with the Declaration of Helsinki (as revised in 2013) and approved by the institutional review board of Siriraj Hospital under ID SIRB Protocol No. 851/2563(IRB4). All participating institutions were informed and agreed the study. Given the retrospective nature of this study, the requirement for informed consent was waived.
The dataset adhered to the following inclusion criteria:
- Patients aged 18 years or older.
- The thyroid diagnostic procedure via ultrasound-guided FNA was conducted on the same day.
- The nodule’s diagnosis, whether benign or malignant, was conclusively determined using thyroid FNA cytology.
Given that benign cytological findings usually constitute 80–90% of all thyroid nodule diagnoses, benign cases were chosen proportionally to malignant ones for each year in focus to ensure a balanced dataset.
The dataset’s quality was ensured by applying the following exclusion criteria:
- Nodules yielding inconclusive pathology results.
- Ultrasound images of compromised clarity or quality.
Ultrasound image acquisition
Thyroid ultrasound examinations were carried out by experienced radiologists utilizing high-frequency linear probes across various institutions: Philips iu22 xmatrix at Siriraj Hospital, GE logiq E9 and GE logiq E10 at Vajira Hospital, and GE logiq E9 along with Toshiba Aplio 500 at HRH Princess Maha Chakri Sirindhorn Medical Center. All scans adhered to the American College of Radiology accreditation standards, capturing the thyroid glands in both transverse and longitudinal orientations using grayscale imaging. These images were then archived in a picture archiving and communication system (PACS).
Subsequently, the thyroid nodule ultrasound images from the PACS were retrieved and exported as JPEG files. To maintain patient confidentiality and focus on relevant areas, images displaying personal details or non-ultrasonographic content were trimmed using a software tool developed in-house. Examples of these modifications are illustrated in Figure 1.
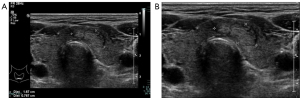
The ultrasound thyroid images were assessed by radiologists with a decade of experience, as well as by third-year diagnostic radiology residents. The evaluation criteria focused on several distinct ultrasound features of each nodule:
- Shape: either taller or wider.
- Margin: delineated as well-defined or ill-defined.
- Echogenicity: characterized as marked hypoechoic, hypoechoic, isoechoic, or hyperechoic.
- Composition: categorized as predominantly cystic, predominantly solid, or entirely solid.
- Calcification: the presence of calcification was identified and further characterized based on size, distinguishing between microcalcification (small-sized calcifications) and macrocalcification (larger-sized calcifications) or determined to be absent.
The American College of Radiology Thyroid Imaging Reporting and Data System (ACR TI-RADS 2017) guidelines (23) were employed to classify each thyroid nodule, based on its ultrasound characteristics, as benign or malignant.
In total, the collection comprised 600 thyroid nodules, with 229 classified as malignant and 371 as benign. These images were then randomly distributed across training, validation, and testing datasets, with allocations of 80%, 10%, and 10% respectively.
Data and statistical analysis
All statistical evaluations were carried out using the SPSS software, version 26. Continuous variables, such as patient age and nodule size, were expressed as mean (with standard deviation) or median (with minimum and maximum values), depending on their distribution characteristics. Categorical variables, including gender, nodule count, and thyroid nodule types (benign or malignant), were detailed using frequencies (and percentages).
The diagnostic performance metrics for assessing thyroid nodules both overall and at individual centers comprised sensitivity, specificity, accuracy, positive predictive value, negative predictive value, and their associated 95% confidence intervals (CIs).
For baseline comparisons across study centers, categorical variables like gender, nodule count, and nodule types were evaluated via the Chi-square test. Continuous variables, namely age and nodule size, were analyzed through one-way analysis of variance (ANOVA) and the Kruskal-Wallis test, respectively. Should significant discrepancies in baseline variables emerge, post hoc comparisons were made between centers.
Associations with malignancy were discerned through univariate analysis. Variables from the univariate analysis with a P value below 0.2 were subsequently entered into a multiple logistic regression model. Adjusted odds ratios (with 95% CIs) were then derived. Any P value below 0.05 was considered indicative of statistical significance. We have specified that all P value tests conducted in our study were two-sided, providing clarity on our statistical approach and analysis framework.
To gauge the level of agreement on final diagnoses, the Cohen’s Kappa statistic was employed, comparing the assessments of the AI, experienced radiologists over 10 years of experience in head and neck imaging, and the resident radiologist.
Thyroid nodule classification system
This study’s thyroid nodule assessment system was developed based on clinical practices across multiple centers in Thailand: Siriraj Hospital, Vajira Hospital, and HRH Princess Maha Chakri Sirindhorn Medical Center. A comprehensive illustration detailing the design and development process is presented in Figure 2. Sequential steps of this system, as outlined in the figure, will be elaborated upon subsequently in this section. The source of our input images stems from the multi-center database discussed in Section Materials. We will delve deeper into the aspects of ROI segmentation, image augmentation, and weakly supervised classification in the following passages.
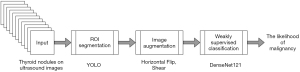
Deep learning techniques: emphasis on WSDAN
Two deep-learning models were employed in this system. The first, YOLO-V5 (24), has seen progressive improvements for object detection and was harnessed for caliper mark detection to segment ROIs on ultrasound images. The latter model, the WSDAN (22), merits a deeper dive given its pivotal role in classifying fine-grained features in our study.
WSDAN, in the realm of fine-grained visual categorization, focuses intently on subtle and localized image differences, which are often discerned from specific image portions. This discernment is crucial for our study as it allows the model to identify intricate patterns and features associated with thyroid nodules. The attention maps, derived from the feature maps of its underlying convolutional neural network (CNN), highlight regions that significantly contribute to the model’s decision-making process. Mathematically, the attention map for an image can be represented as:
the attention map is represented mathematically, where is the feature extraction function, and represents the learnable weights of the model. This discernment enhances the model’s ability to distinguish between benign and malignant nodules, providing a more granular and accurate diagnosis.
The unique advantage of WSDAN lies in its capability to augment these attention maps, thereby spotlighting discriminative regions in the image. This attention-driven focus ensures that the model emphasizes the most informative parts of an image, enhancing its classification accuracy, especially when pitted against conventional CNNs.
In our application, WSDAN was trained for thyroid nodule classification. The model’s outcome furnishes a probability indicative of malignancy. Given the nuances in distinguishing benign from malignant thyroid nodules, the attention-centric architecture of WSDAN ensures robust and precise classifications.
Image transformation for system input
For effective processing and analysis by the thyroid nodule classification system, input images undergo a transformation to ensure optimization for the AI system. The original ultrasound images, as retrieved from the PACS, are depicted in Figure 1, this post-processing involves the removal of any personal information, annotations, and irrelevant regions showing in Figure 1B.
This image refinement ensures that the AI system is not distracted or misled by extraneous data, thereby improving the accuracy and consistency of nodule classification. Moreover, eliminating personal details from the images serves a dual purpose: it not only reduces potential AI confusion but also reinforces patient data privacy.
ROI segmentation
The YOLO-V5 algorithm was specifically designed for target detection (25). Notable advantages of this algorithm include the compactness of the model and its swift computational abilities. Version 5, in particular, stands out for its flexibility and impressively lightweight size compared to its predecessors, making it a popular choice for target detection across various domains.
In the clinical practices across multi-centers in Thailand, caliper marks are typically made to demarcate the boundaries of thyroid nodules. Consequently, most ultrasound im- ages sourced from the PACS exhibit these caliper marks. As illustrated in Figure 3, these caliper marks served as training examples for the YOLO-V5 algorithm to detect caliper mark locations. Once detected, the boundaries of the thyroid were inferred based on these caliper mark positions. However, recognizing that these inferred boundaries might not fully encompass the entire thyroid nodule, we added a margin to each ultrasound image’s ROI. More precisely, a margin equivalent to 40% of the estimated boundary was introduced. This determination was made based on experiments assessing the completeness of the ROI.
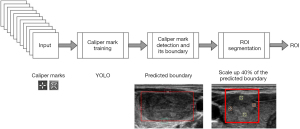
Figure 4 provides visual examples of the ROI segmentation process for both benign and malignant nodules. Figure 4A and Figure 4B present the thyroid nodules as they appear on systematically cropped ultrasound images, representing benign and malignant nodules respectively. Figure 4C and Figure 4D showcase the estimated caliper mark boundaries in red, as predicted by the caliper mark detection model. Finally, Figure 4E and Figure 4F depict the ROIs that have been marginally increased by 40% from the red boundaries illustrated in Figure 4C and Figure 4D.
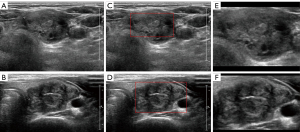
Image inpainting
Referring to the previous study utilizing a subset of this dataset (26), predictions were found to be more accurate when the caliper marks on ultrasound images were inpainted. Consequently, the caliper marks on the segmented ROIs were eliminated using a developed software tool for image inpainting. Algorithm 1 delineates the steps involved in the image inpainting process. Figure 5A presents examples of the inpainted images.
Algorithm 1 Algorithm for Caliper Mark Removal | |
---|---|
Require: ultrasound (U/S) image ROI, threshold value, p(i, j) intensity | |
1: | while each pixel p(i, j) in ROI do |
2: | |
3: | end while |
4: | mask ← dilation(mask) |
5: | |
6: | for each mark pixel (i, j) do |
7: | p(i, j) ← neighbor * tensor7×7 |
8: | end for |
Image augmentation
Our AI system employs two distinct image augmentation methods. The initial approach encompasses basic image transformations: horizontal flipping and shearing, which are visualized in Figure 5, with Figure 5B demonstrating the horizontal flip and Figure 5C highlighting a 0.15% shear effect.
The second technique is embedded within the WSDAN (22). In contrast to conventional random transformations found in earlier deep models (15,16,21,25), WSDAN adopts a strategic direction. It accentuates spatial augmentations, such as image cropping and image dropping, as depicted in. The Augmentation Map, , is derived from
where represents the Attention map. The Attention cropping mechanism is defined as:
Similarly, Attention dropping is expressed as:
The distinguishing feature of WSDAN is its adept use of attention maps. It guides the model to pay attention to multiple regions of the nodule. This ensures optimal attention allocation across pivotal features. Consequently, WSDAN’s spatial augmentation outperforms its random counterparts, culminating in a richer feature extraction process. This fortifies the deep model’s prowess in object localization and classification. The attention-focused augmentation mechanism is pictorially represented in Figure 6.
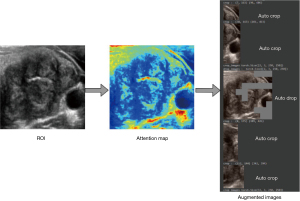
Predicting the likelihood of malignancy
The malignancy prediction is presented as a percentage, as shown in Figure 7. This prediction uses the end-to-end fine-grained classification model, WSDAN. Notably, WSDAN’s capabilities extend beyond image augmentation to encompass fine-grained visual classification, allowing it to differentiate between objects with subtle differences, like thyroid nodules. Thyroid nodule classification is challenging due to its low inter-class variances. Differentiating benign from malignant nodules can be complex, given their significant similarities. Only minor differences, such as calcifications, echotexture, and margins, differentiate them. Being weakly supervised, WSDAN employs not only labels but also automated image cropping and dropping techniques for learning.
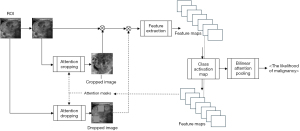
Furthermore, WSDAN’s design is versatile and can be combined with many pre-trained models. In this study, we incorporated the DenseNet121 pre-trained model, based on findings from previous research (26).
Performance evaluation: WSDAN in conjunction with DenseNet121
We assessed the performance of WSDAN when paired with DenseNet121, comparing it to standalone DenseNet121 using the identical dataset referenced in (25). The results are tabulated in Table 1.
Table 1
Classifier | Sensitivity (95% CI) | Specificity (95% CI) | Accuracy (95% CI) | PPV (95% CI) | NPV (95% CI) |
---|---|---|---|---|---|
DenseNet121, % | 79.92 (46.19–94.96) | 78.85 (65.30–88.94) | 78.46 (66.51–87.69) | 47.62 (33.21–62.43) | 93.18 (83.38–97.38) |
WSDAN with DenseNet121, % | 84.62 (54.55–98.08) | 86.54 (74.21–94.41) | 86.15 (75.34–93.47) | 61.11 (43.17–76.48) | 86.15 (75.34–93.47) |
WSDAN, Weakly Supervised Data Augmentation Network; CI, confidence interval; PPV, positive prediction value; NPV, negative prediction value.
The combined approach, integrating WSDAN with DenseNet121, exhibited enhanced effectiveness. Consequently, we utilized this combination to train our model using ultrasound images sourced from Siriraj Hospital, Vajira Hospital, and the HRH Princess Maha Chakri Sirindhorn Medical Center.
Heat map analysis for prediction interpretation
WSDAN employs attention maps during the training process to direct data augmentation. These attention maps highlight the most discriminative regions within nodules. For each case discussed below, the corresponding attention map is presented in Figures 8-10, respectively. Light gray areas on these maps indicate regions of heightened attention. However, due to multiplication with zero values in the original image—a process ensuring normalization—some of these regions become invisible in the attention map.
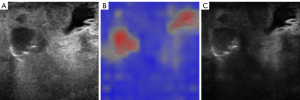
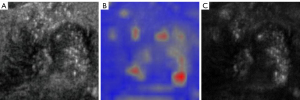
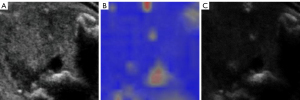
In response to this challenge, we generated heat maps using a methodology adapted from Kunapinun et al. (27), with red zones indicating high-attention regions. This dual analysis—attention maps complemented by heat maps—offers nuanced insights into model interpretation, as discussed in the ensuing prediction results.
- Benign nodule prediction (Figure 8A,8B): the transverse ultrasound depicted a benign nodule, correctly identified by the AI with 99.99% confidence. Figure 8A shows the nodule image, and Figure 8B presents the heat map of nodule type detection. The corresponding attention map merged with the ultrasound image and heat map is shown in Figure 8C. This image showed an isoechoic mixed cystic-solid nodule with lobulated margins. The heat map indicated potential malignancy risks in the fluid and the high reflective tissue of the nodule. Despite these suspicions, the AI correctly classified the nodule as benign.
- Malignant nodule prediction (Figure 9A,9B): the transverse ultrasound showed a malignant nodule, again correctly identified by the AI with 99.99% confidence. Figure 9A displays the nodule image, and Figure 9B shows the heat map of nodule type detection. The attention map’s result, merged with the ultrasound image and heat map, is depicted in Figure 9C. This nodule was hypoechoic, solid, with microcalcifications, and ill-defined margins. The heat map highlighted areas of concern, especially around the microcalcifications. Given these findings, the AI correctly marked it as malignant, suggesting areas to monitor closely.
- False malignant prediction (Figure 10A,10B): the ultrasound depicted a benign nodule, but the AI erroneously classified it as malignant with a confidence of 94.96%. Figure 10A illustrates the nodule image, and Figure 10B depicts the heat map of nodule type detection. The result of the attention map merged with the ultrasound image and heat map is presented in Figure 10C. This nodule exhibited a smooth texture, isoechoic characteristics, and contained a small cyst. Notably, the heat map highlighted suspicious regions, primarily around tissue reflections and macrocalcifications. The misclassification by the AI is likely attributed to the challenge of distinguishing certain macrocalcification points as microcalcifications, possibly influenced by variations in image resolution.
Results
In our exploration, we sought to uncover the efficacy and reliability of the computer-aided diagnosis system in the realm of thyroid ultrasound diagnostics. Central to our analysis was the distribution and utilization of our datasets across various operational groups.
Demographic data
A total of 600 thyroid nodules were examined from the three centers, with participants having an average age of (52.72±15.30) years, spanning an age range from 15 to 90 years. Females constituted a significant portion of the cohort, with 543 participants (90.5%). Out of the 600 nodules, pathology identified 371 (61.8%) as benign and 229 (38.2%) as malignant. The nodules had an average size of 1.9 cm, with sizes ranging from 0.3 to 10.4 cm. Most patients presented with a single nodule, accounting for 30.5% of the cases. The variance in the number of thyroid nodules, their sizes, and their type (benign or malignant) was statistically significant across the three centers (P<0.001 for all three variables). These data points are tabulated in Table 2.
Table 2
Variables | All patients (n=600) | Center A** (n=200) | Center B** (n=200) | Center C** (n=200) | P value |
---|---|---|---|---|---|
Age (years) | 52.72±15.30 | 52.08±14.92 | 53.89±14.55 | 52.19±16.36 | 0.41 |
Sex; female | 543 (90.5) | 180 (90.0) | 178 (89.0) | 185 (92.5) | 0.47 |
No. nodule | <0.001* | ||||
≤5 | 520 (86.7) | 177 (88.5) | 190 (95.0) | 153 (76.5) | |
1 | 183 (30.5) | 64 (32.0) | 76 (38.0) | 43 (21.5) | |
2 | 164 (27.3) | 48 (24.0) | 72 (36.0) | 44 (22.0) | |
3 | 84 (14.0) | 28 (14.0) | 31 (15.5) | 25 (12.5) | |
4 | 47 (7.8) | 20 (10.0) | 9 (4.5) | 18 (9.0) | |
5 | 42 (7.0) | 17 (8.5) | 2 (1.0) | 23 (11.5) | |
>5 | 80 (13.3) | 23 (11.5) | 10 (5.0) | 47 (23.5) | |
Tumor long size (cm)*** | 1.9 (0.3–10.4) | 1.7 (0.3–6.8) | 1.7 (0.4–10.4) | 2.4 (0.4–9.4) | <0.001* |
Benign | 371 (61.8) | 92 (46) | 174 (87) | 105 (52.5) | <0.001* |
Malignant | 229 (38.2) | 108 (54.0) | 26 (13.0) | 95 (47.5) | <0.001* |
Data are represented as mean ± SD or number (%). *, significant P value <0.05; **, Center A refers to Siriraj Hospital, Center B to Vajira Hospital, and Center C to HRH Princess Maha Chakri Sirindhorn Medical Center; ***, tumor long size (cm) represent measurements of the longest dimension of thyroid nodules as observed in the transverse view on U/S images. SD, standard deviation; U/S, ultrasound.
Analysis of a cohort of 600 patients, detailed in Table 2, reveals key demographic and clinical characteristics across three medical centers. The cohort had an average age of 52.72 years [standard deviation (SD) =15.30], with no significant age difference across centers (P=0.41). Females predominated the sample, making up 90.5% of patients, showing consistent gender distribution (P=0.47). Notably, nodule count varied significantly between centers (P<0.001), with most patients (86.7%) having 5 or fewer nodules. Tumor size also differed significantly (P<0.001), averaging 1.9 cm across the sample. Diagnostic outcomes highlighted a significant variance in nodule pathology, with 61.8% benign and 38.2% malignant nodules, showing a distinct difference in the prevalence of benign versus malignant nodules between centers (P<0.001).
Within the test group, 60 nodules were examined, evenly distributed with 20 nodules from each center. The participants in this group had an average age of 54.48±16.62 years and an age range between 36 and 81 years. Females made up 86.7% of this group, represented by 52 patients. From these 60 nodules, 35 (58.3%) were identified as benign, while the remaining 25 (41.7%) were classified as malignant by pathology. The average nodule size in this subgroup was 1.75 cm, ranging from 0.5 to 7.7 cm. Notably, the majority of these patients had two nodules, representing 36.7% of the cases. A comprehensive breakdown of the test group’s demographic data can be found in Table 3, highlighting no significant disparities among the three centers.
Table 3
Variables | All patients (n=60) | Center A** (n=20) | Center B** (n=20) | Center C** (n=20) | P value |
---|---|---|---|---|---|
Age (years) | 54.48±16.62 | 50.55±19.87 | 52.15±12.63 | 60.75±15.50 | 0.11 |
Sex; female | 52 (86.7) | 16 (80.0) | 20 (100.0) | 16 (80.0) | 0.09 |
No. nodule | 0.79 | ||||
≤5 | 50 (83.3) | 16 (80.0) | 17 (85.0) | 17 (85.0) | |
1 | 11 (18.3) | 5 (25.0) | 2 (10.0) | 4 (20.0) | |
2 | 22 (36.7) | 6 (30.0) | 7 (35.0) | 9 (45.0) | |
3 | 8 (13.3) | 2 (10.0) | 5 (25.0) | 1 (5.0) | |
4 | 4 (6.7) | 2 (10.0) | 1 (5.0) | 1 (5.0) | |
5 | 5 (8.3) | 1 (5.0) | 2 (10.0) | 2 (10.0) | |
>5 | 10 (16.7) | 4 (20.0) | 3 (15.0) | 3 (15.0) | |
Tumor long size (cm)*** | 1.75 (0.5–7.7) | 1.55 (0.6–4.6) | 1.85 (0.7–3.6) | 2.3 (0.5–7.7) | 0.34 |
Benign | 35 (58.3) | 10 (50.0) | 15 (75.0) | 10 (50.0) | 0.18 |
Malignant | 25 (41.7) | 10 (50.0) | 5 (25.0) | 10 (50.0) | 0.18 |
Data are represented as mean ± SD or number (%). *, significant P value <0.05; **, Center A refers to Siriraj Hospital, Center B to Vajira Hospital, and Center C to HRH Princess Maha Chakri Sirindhorn Medical Center; ***, tumor long size (cm) represent measurements of the longest dimension of thyroid nodules as observed in the transverse view on U/S images. SD, standard deviation; U/S, ultrasound.
In a focused analysis of a test group comprising 60 patients, summarized in Table 3, we observed the following trends across Centers A, B, and C: The average age was 54.48 years (SD =16.62), without significant differences across centers (P=0.11). The group was predominantly female (86.7%), with consistent gender distribution among the centers (P=0.09). Most patients (83.3%) had five or fewer nodules, showing no significant variance in nodule count (P=0.79). Tumor sizes averaged 1.75 cm, with no significant cross-center differences (P=0.34). Regarding diagnoses, 58.3% of nodules were benign, and 41.7% were malignant, indicating a balanced representation of nodule pathology without specifying the variance across centers.
Ultrasound characteristics of nodules
We have updated the manuscript to include the precision of the 95% CIs for the test results, which pertain to the ultrasound characteristics of nodules in Table 4, specifically for the significant association of micro-calcification with malignant thyroid nodules, which now reads: ‘Micro-calcification emerged as a significant ultrasound characteristic associated with malignant thyroid nodules, with an adjusted odds ratio of 5.21 (95% CI: 1.22–21.19, P=0.02)’. This amendment ensures clarity and thoroughness in the presentation of our findings, as requested.
Table 4
Variables | Number (%) | Risk factor of malignant | ||||
---|---|---|---|---|---|---|
Benign (n=35) | Malignant (n=25) | P value | Adjusted OR (95% CI) | P value | ||
Margin | 0.10 | |||||
Well-defined | 30 (85.7) | 17 (68.0) | 1 | – | ||
Ill-defined | 5 (14.3) | 8 (32.0) | 2.40 (0.54–10.70) | 0.25 | ||
Shape | 0.31 | N/A | N/A | |||
Wider | 29 (82.9) | 18 (72.0) | ||||
Taller | 6 (17.1) | 7 (28.0) | ||||
Echo | 0.41 | N/A | N/A | |||
Hyperechoic | 0 (0.0) | 0 (0.0) | ||||
Isoechoic | 18 (51.4) | 9 (36.0) | ||||
Hypoechoic | 15 (42.9) | 13 (52.0) | ||||
Marked hypoechoic | 2 (5.7) | 3 (12.0) | ||||
Calcification | 0.01* | |||||
None | 27 (77.1) | 10 (40.0) | 1 | – | ||
Macrocalcification | 4 (11.4) | 5 (20.0) | 1.77 (0.35–8.83) | 0.48 | ||
Microcalcification | 4 (11.4) | 10 (40.0) | 5.21 (1.22–21.19) | 0.02* | ||
Composition | 0.06 | |||||
Predominate cyst | 6 (17.1) | 0 (0.0) | ||||
Predominate solid | 9 (25.7) | 5 (20.0) | 1 | – | ||
Solid | 20 (57.1) | 20 (80.0) | 2.83 (0.59–13.58) | 0.19 |
*, significant P value <0.05. OR, odds ratio; CI, confidence interval; N/A, not applicable.
Dataset distribution: train, validation, and test groups
Out of 600 thyroid nodule images, we retrospectively selected a representative image for each nodule. These images were then randomly allocated to the training (80%), validation (10%), and testing (10%) groups. We processed the dataset following the workflow depicted in Figure 11, which outlines the stages from image selection to group allocation, ensuring a systematic approach to data handling and analysis.
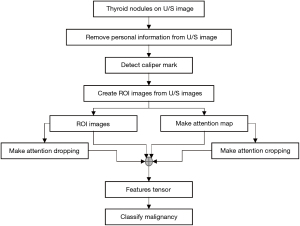
Diagnostic performance: AI, experienced radiologist, and residents
Table 5 details the diagnostic performance in differentiating benign and malignant thyroid nodules across three diagnostic methods: AI, experienced radiologists, and residents.
- AI: AI demonstrated a sensitivity of 80.0% (59.3–93.2%), specificity of 71.4% (53.7–85.4%), accuracy of 75.0% (62.1–85.3%), positive predictive value of 66.7% (53.4–77.8%), and a negative predictive value of 83.3% (68.9–91.8%). Importantly, AI showcased the highest diagnostic performance in center A (P<0.001).
- Experienced radiologists: the metrics for this group were: sensitivity of 40.0% (21.1–61.3%), specificity of 80.0% (63.6–91.6%), accuracy of 63.3% (49.9–75.4%), positive predictive value of 58.8% (38.7–76.4%), and negative predictive value of 65.1% (56.5–72.8%). Like the AI, the experienced radiologists also recorded optimal performance in center A (P=0.011).
- Residents: the residents posted a sensitivity of 64.0% (42.5–82.0%), specificity of 77.1% (59.9–89.6%), accuracy of 71.7% (58.6–82.6%), positive predictive value of 66.7% (50.5–79.8%), and a negative predictive value of 75.0% (63.3–83.9%). Matching the trends seen in AI and experienced radiologists, the residents also had their peak performance in center A (P<0.001).
Table 5
Center | Reader | Sensitivity (95% CI) | Specificity (95% CI) | PPV (95% CI) | NPV (95% CI) | Accuracy (95% CI) | P value |
---|---|---|---|---|---|---|---|
All | AI | 80.0% (59.3–93.2%) | 71.4% (53.7–85.4%) | 66.7% (53.4–77.8%) | 83.3% (68.9–91.8%) | 75.0% (62.1–85.3%) | <0.001* |
Radiologist | 40.0% (21.1–61.3%) | 80% (63.6–91.6%) | 58.8% (38.7–76.4%) | 65.1% (56.5–72.8%) | 63.3% (49.9–75.4%) | 0.09 | |
Resident | 64.0% (42.5–82.0%) | 77.1% (59.9–89.6%) | 66.7% (50.5–79.8%) | 75.0% (63.3–83.9%) | 71.7% (58.6–82.6%) | 0.001* | |
Center A** | AI | 90% (55.5–99.8%) | 100% (69.2–100.0%) | 100.0% | 90.9% (60.9–98.5%) | 95% (75.1–99.9%) | <0.001* |
Radiologist | 60% (26.2–87.8%) | 100% (69.2–100.0%) | 100.0% | 71.4% (53.9–84.2%) | 80% (56.3–94.3%) | 0.01* | |
Resident | 90% (55.5–99.8%) | 90% (55.5–99.8%) | 90% (58.1–98.3%) | 90% (58.1–98.3%) | 90% (68.3–98.8%) | <0.001* | |
Center B** | AI | 80% (28.4– 99.5%) | 66.7% (38.4–88.2%) | 44.4% (25.7–64.9%) | 90.9% (62.6–98.4%) | 70% (45.7–88.1%) | 0.12 |
Radiologist | 40% (5.2–85.3%) | 73.3% (44.9–92.2%) | 33.3% (11.4–66.1%) | 78.6% (62.7–88.9%) | 65% (40.8–84.6%) | 0.61 | |
Resident | 60% (14.7–94.7%) | 80% (51.9–95.7%) | 50% (22.4–77.6%) | 85.7% (66.6–94.8%) | 75% (50.9–91.3%) | 0.13 | |
Center C** | AI | 70% (34.8–93.3%) | 50% (18.7–81.3%) | 58.3% (40.1–74.6%) | 62.5% (34.9–83.8%) | 60% (36.1–80.9%) | >0.99 |
Radiologist | 20% (2.5–55.6%) | 70% (34.8–93.3%) | 40% (12.3–76.0%) | 46.7% (34.4– 59.3%) | 45% (23.1–68.5%) | >0.99 | |
Resident | 40% (12.2–73.8%) | 60% (26.2–87.8%) | 50% (25.5–74.5%) | 50% (32.8– 67.2%) | 50% (27.2–72.8%) | >0.99 |
*, significant P value <0.05; **, Center A refers to Siriraj Hospital, Center B to Vajira Hospital, and Center C to HRH Princess Maha Chakri Sirindhorn Medical Center. AI, artificial intelligence; CI, confidence interval; PPV, positive prediction value; NPV, negative prediction value.
Performance comparison: AI vs. radiologist vs. resident
Table 6 showcases the performance of AI, experienced radiologists, and residents in nodule classification based on diagnostic sensitivity and specificity.
- The AI demonstrated a significantly higher diagnostic sensitivity compared to the experienced radiologist (P=0.04). This trend was particularly evident in centers B and C with P values of 0.02 and 0.02, respectively.
- There was no significant difference in diagnostic sensitivity when comparing AI and residents (P=0.21) or experienced radiologists and residents (P=0.09).
- Across all three methods, there wasn’t a significant difference in diagnostic specificity with P values ranging from 0.40 to 0.76.
Table 6
Performance comparison | All (n=60) | Center A* (n=20) | Center B* (n=20) | Center C* (n=20) | |||||||
---|---|---|---|---|---|---|---|---|---|---|---|
Sensitivity | Specificity | Sensitivity | Specificity | Sensitivity | Specificity | Sensitivity | Specificity | ||||
AI-radiologist | 0.043* | 0.405 | 0.131 | 1.000 | 0.028* | 0.829 | 0.029* | 0.374 | |||
AI-resident | 0.212 | 0.588 | 1.000 | 0.317 | 0.240 | 0.652 | 0.189 | 0.661 | |||
Radiologist-resident | 0.093 | 0.769 | 0.131 | 0.317 | 0.282 | 0.812 | 0.342 | 0.648 |
*, Center A refers to Siriraj Hospital, Center B to Vajira Hospital, and Center C to HRH Princess Maha Chakri Sirindhorn Medical Center. AI, artificial intelligence.
Observer variability analysis
Table 7 provides a detailed breakdown of inter-observer variability among the AI, experienced radiologists, and residents.
- The agreement between AI and the experienced radiologist had a Kappa value of [0.37 (95% CI: 0.16–0.58)], reflecting a fair level of agreement.
- Comparing AI and residents, the Kappa value was [0.33 (95% CI: 0.10–0.57)], also indicating a fair agreement.
- In the comparison between the radiologist and the resident, a Kappa value of [0.53 (95% CI: 0.31–0.74)] was observed, representing a moderate level of agreement.
- Notably, center A consistently exhibited a higher Kappa value, ranging between 0.48 and 0.70, compared to other centers.
Table 7
Readers | Kappa (95% CI) | |||
---|---|---|---|---|
All (n=60) | Center A* (n=20) | Center B* (n=20) | Center C* (n=20) | |
AI-radiologist | 0.37 (0.16–0.58) | 0.48 (0.11–0.85) | 0.69 (0.38–1.00) | 0.000 (N/A) |
AI-resident | 0.33 (0.10–0.57) | 0.70 (0.38–1.00) | 0.27 (0–0.68) | 0.038 (0.0–0.44) |
Radiologist-resident | 0.53 (0.31–0.74) | 0.60 (0.28–0.92) | 0.52 (0.12–0.93) | 0.44 (0.02–0.87) |
*, Center A refers to Siriraj Hospital, Center B to Vajira Hospital, and Center C to HRH Princess Maha Chakri Sirindhorn Medical Center. AI, artificial intelligence; CI, confidence interval; N/A, not applicable.
Discussion
Our study has successfully demonstrated the potential of an AI-driven computer-aided diagnosis system to significantly enhance diagnostic accuracy in thyroid ultrasound imaging. With a sensitivity of 80.0%, specificity of 71.4%, and overall accuracy of 75.0%, our AI system has shown promising results in differentiating between benign and malignant thyroid nodules. These findings are particularly noteworthy given the AI’s superior sensitivity compared to that of experienced radiologists, highlighting its potential to reduce unnecessary FNA procedures. This summary sets the stage for our discussion on the implications of these results, the comparison with existing literature, and the direction for future research in AI applications in thyroid nodule diagnostics.
The advent of computer-aided diagnosis systems utilizing AI has notably enhanced the diagnostic precision of thyroid ultrasound, providing a potential avenue to curtail unwarranted FNA procedures. The comprehensive meta-analysis by Liu et al. (6) substantiates the comparable diagnostic efficacy of these AI systems with veteran radiologists. Yet, earlier research by Gao et al. (16) and Luo et al. (10) spotlighted the system’s inferior specificity relative to its human counterparts.
Our endeavor integrated the DenseNet121 with WSDAN, deploying deep learning to delineate benign from malignant thyroid nodules. Our results—a sensitivity of 80.0%, specificity of 71.4%, and an accuracy of 75.0%—though aligned in sensitivity, registered a marginal decline in specificity and accuracy when juxtaposed with prior findings from Arunrukthavon et al. (26), Ma et al. (15), and Kim et al. (28). A plausible explanation for this discrepancy is the inherent heterogeneity of ultrasound images across distinct centers. An insightful observation from our study revealed the AI’s superior diagnostic proficiency in Center A. This could be attributed to the AI’s familiarity with the substantial and congruent dataset from Center A, as evidenced in Arunrukthavon et al.’s work (26), rendering other centers with varied datasets at a comparative disadvantage.
Our AI system demonstrated an impressive sensitivity, outpacing even experienced radiologists. However, specificity metrics were somewhat parallel between the AI, seasoned radiologists, and residents, with the latter two exhibiting marginally better outcomes. The distinctiveness of our findings when compared to earlier studies might stem from an unbiased, rigorous AI training protocol. Given its elevated sensitivity, AI holds promise in augmenting radiological diagnostics, particularly aiding novices in discerning malignant nodules, thus reducing superfluous FNA interventions.
It is noteworthy that the experienced radiologists’ specificity in our study lagged behind those documented by Choi et al. (10) and Yoo et al. (15), potentially due to a population skew. It is inferred that patients subjected to FNA generally present with ambiguous nodules, posing challenges for ultrasound-based diagnosis.
In cases where the AI misclassified a benign nodule with a 94.96% confidence, potential challenges emerged in distinguishing macrocalcifications from microcalcifications. The visual similarities, compounded by image resolution variations, pose a hurdle for precise characterization. The AI, relying on learned patterns, may misinterpret larger macrocalcifications as smaller ones, leading to misclassifications. Enhancing AI training protocols to encompass nuanced size variations in calcifications is crucial. Future efforts should prioritize diverse datasets and advanced resolution considerations for improved discriminatory capabilities. Collaborative research and ongoing algorithmic refinements are essential for advancing diagnostic precision in varied clinical scenarios.
The interobserver variability findings, particularly the AI trailing slightly behind the consensus between the experienced radiologist and the resident, prompt a closer look at the AI’s learning curve. Further exploration of these nuances will be crucial in optimizing the integration of AI in clinical practice.
In summary, the inter-observer variability analysis not only contributes valuable insights into diagnostic agreement but also underscores the need for careful consideration of dataset characteristics and AI learning curves in the context of thyroid nodule assessment.
Limitation
Our study, however, isn’t without its constraints. The limited dataset spanning a mere three centers might not be sufficiently representative. The employment of JPEG as the chosen format could inadvertently compromise image fidelity, thereby influencing AI diagnostic performance. Envisioned future studies should leverage larger datasets from diverse centers and universal image standards, preferably in Digital Imaging and Communications in Medicine (DICOM) format, to amplify diagnostic precision. Our study’s retrospective nature, coupled with its reliance on static imagery, poses potential biases and could inadvertently hamper radiologist performance. The exclusive focus on nodules with definitive diagnoses inadvertently sidesteps ambiguous or nondiagnostic cytology, circumscribing the broader applicability of our findings.
Conclusions
In this study, the AI demonstrated a heightened sensitivity in diagnosing thyroid nodules when compared to experienced radiologists. However, when it came to specificity, the AI system mirrored the performance of both experienced radiologists and residents. The advantages showcased by the AI in terms of diagnostic sensitivity suggest its potential as a valuable adjunct in the clinical landscape. Deploying this system in con- junction with radiologists may pave the way for enhanced diagnostic accuracy, potentially improving patient care outcomes in the realm of thyroid nodule evaluations. As a step forward, our future research direction involves the incorporation of Doppler imaging into the model, aiming to further enhance its diagnostic capabilities and contribute to the evolving landscape of thyroid nodule assessment.
Acknowledgments
Funding: The work was supported by
Footnote
Reporting Checklist: The authors have completed the STARD reporting checklist. Available at https://qims.amegroups.com/article/view/10.21037/qims-23-1650/rc
Conflicts of Interest: All authors have completed the ICMJE uniform disclosure form (available at https://qims.amegroups.com/article/view/10.21037/qims-23-1650/coif). All authors report that they have funding from the Broadcasting and Telecommunication Research and Development Fund for Public Interest (NBTC), Thailand [grant A63-1(2)-018]. The authors have no other conflicts of interest to declare.
Ethical Statement: The authors are accountable for all aspects of the work in ensuring that questions related to the accuracy or integrity of any part of the work are appropriately investigated and resolved. This study was conducted in accordance with the Declaration of Helsinki (as revised in 2013). Approval for this research was granted by the Institutional Review Board of Siriraj Hospital, under the protocol ID SIRB No. 851/2563(IRB4). All participating institutions were informed and agreed the study. Given the retrospective nature of this study, the requirement for informed consent was waived.
Open Access Statement: This is an Open Access article distributed in accordance with the Creative Commons Attribution-NonCommercial-NoDerivs 4.0 International License (CC BY-NC-ND 4.0), which permits the non-commercial replication and distribution of the article with the strict proviso that no changes or edits are made and the original work is properly cited (including links to both the formal publication through the relevant DOI and the license). See: https://creativecommons.org/licenses/by-nc-nd/4.0/.
References
- Guth S, Theune U, Aberle J, Galach A, Bamberger CM. Very high prevalence of thyroid nodules detected by high frequency (13 MHz) ultrasound examination. Eur J Clin Invest 2009;39:699-706. [Crossref] [PubMed]
- Wolinski K, Stangierski A, Ruchala M. Comparison of diagnostic yield of core-needle and fine-needle aspiration biopsies of thyroid lesions: Systematic review and meta-analysis. Eur Radiol 2017;27:431-6. [Crossref] [PubMed]
- Siegel RL, Miller KD, Jemal A. Cancer statistics, 2019. CA Cancer J Clin 2019;69:7-34. [Crossref] [PubMed]
- Pellegriti G, Frasca F, Regalbuto C, Squatrito S, Vigneri R. Worldwide increasing incidence of thyroid cancer: update on epidemiology and risk factors. J Cancer Epidemiol 2013;2013:965212. [Crossref] [PubMed]
- Tangjaturonrasme N, Vatanasapt P, Bychkov A. Epidemiology of head and neck cancer in Thailand. Asia Pac J Clin Oncol 2018;14:16-22. [Crossref] [PubMed]
- Liu R, Li H, Liang F, Yao L, Liu J, Li M, Cao L, Song B. Diagnostic accuracy of different computer-aided diagnostic systems for malignant and benign thyroid nodules classification in ultrasound images: A systematic review and meta-analysis protocol. Medicine (Baltimore) 2019;98:e16227. [Crossref] [PubMed]
- Haugen BR, Alexander EK, Bible KC, Doherty GM, Mandel SJ, Nikiforov YE, Pacini F, Randolph GW, Sawka AM, Schlumberger M, Schuff KG, Sherman SI, Sosa JA, Steward DL, Tuttle RM, Wartofsky L. 2015 American Thyroid Association Management Guidelines for Adult Patients with Thyroid Nodules and Differentiated Thyroid Cancer: The American Thyroid Association Guidelines Task Force on Thyroid Nodules and Differentiated Thyroid Cancer. Thyroid 2016;26:1-133. [Crossref] [PubMed]
- Choi SH, Kim EK, Kwak JY, Kim MJ, Son EJ. Interobserver and intraobserver variations in ultrasound assessment of thyroid nodules. Thyroid 2010;20:167-72. [Crossref] [PubMed]
- Wang H, Zhao L, Xin X, Wei X, Zhang S, Li Y, Gao M. Diagnostic value of elastosonography for thyroid microcarcinoma. Ultrasonics 2014;54:1945-9. [Crossref] [PubMed]
- Luo S, Kim EH, Dighe M, Kim Y. Thyroid nodule classification using ultrasound elastography via linear discriminant analysis. Ultrasonics 2011;51:425-31. [Crossref] [PubMed]
- Cosgrove D, Barr R, Bojunga J, Cantisani V, Chammas MC, Dighe M, Vinayak S, Xu JM, Dietrich CF. WFUMB Guidelines and Recommendations on the Clinical Use of Ultrasound Elastography: Part 4. Thyroid. Ultrasound Med Biol 2017;43:4-26. [Crossref] [PubMed]
- Yang R, Zou X, Zeng H, Zhao Y, Ma X. Comparison of Diagnostic Performance of Five Different Ultrasound TI-RADS Classification Guidelines for Thyroid Nodules. Front Oncol 2020;10:598225. [Crossref] [PubMed]
- Liang J, Huang X, Hu H, Liu Y, Zhou Q, Cao Q, Wang W, Liu B, Zheng Y, Li X, Xie X, Lu M, Peng S, Liu L, Xiao H. Predicting Malignancy in Thyroid Nodules: Radiomics Score Versus 2017 American College of Radiology Thyroid Imaging, Reporting and Data System. Thyroid 2018;28:1024-33. [Crossref] [PubMed]
- Thomas J, Haertling T. AIBx, Artificial Intelligence Model to Risk Stratify Thyroid Nodules. Thyroid 2020;30:878-84. [Crossref] [PubMed]
- Ma J, Wu F, Zhu J, Xu D, Kong D. A pre-trained convolutional neural network based method for thyroid nodule diagnosis. Ultrasonics 2017;73:221-30. [Crossref] [PubMed]
- Gao L, Liu R, Jiang Y, Song W, Wang Y, Liu J, Wang J, Wu D, Li S, Hao A, Zhang B. Computer-aided system for diagnosing thyroid nodules on ultrasound: A comparison with radiologist-based clinical assessments. Head Neck 2018;40:778-83. [Crossref] [PubMed]
- Krizhevsky A, Sutskever I, Hinton GE. Imagenet classification with deep convolutional neural networks. Commun ACM 2017;60:84-90. [Crossref]
- Buda M, Wildman-Tobriner B, Hoang JK, Thayer D, Tessler FN, Middleton WD, Mazurowski MA. Management of Thyroid Nodules Seen on US Images: Deep Learning May Match Performance of Radiologists. Radiology 2019;292:695-701. [Crossref] [PubMed]
- Ren S, He K, Girshick R, Sun J. Faster R-CNN: Towards Real-Time Object Detection with Region Proposal Networks. IEEE Trans Pattern Anal Mach Intell 2017;39:1137-49. [Crossref] [PubMed]
- Liu C, Xie L, Kong W, Lu X, Zhang D, Wu M, Zhang L, Yang B. Prediction of suspicious thyroid nodule using artificial neural network based on radiofrequency ultrasound and conventional ultrasound: A preliminary study. Ultrasonics 2019;99:105951. [Crossref] [PubMed]
- Zhu YC, AlZoubi A, Jassim S, Jiang Q, Zhang Y, Wang YB, Ye XD. DU H. A generic deep learning framework to classify thyroid and breast lesions in ultrasound images. Ultrasonics 2021;110:106300. [Crossref] [PubMed]
.Hu T Qi H Huang Q Lu Y See better before looking closer: Weakly supervised data augmentation network for fine-grained visual classification. arXiv:1901.09891,2019 .- Tessler FN, Middleton WD, Grant EG, Hoang JK, Berland LL, Teefey SA, Cronan JJ, Beland MD, Desser TS, Frates MC, Hammers LW, Hamper UM, Langer JE, Reading CC, Scoutt LM, Stavros AT. ACR Thyroid Imaging, Reporting and Data System (TI-RADS): White Paper of the ACR TI-RADS Committee. J Am Coll Radiol 2017;14:587-95. [Crossref] [PubMed]
- Jocher G, Stoken A, Borovec J, Jocher G, Stoken A, Borovec J, Kwon Y, Xie T, Fang J, Wong C, Abhiram V, Zhiqiang Wang Z, Fati C, Skalski P, Hogan A, Strobel M, Jain M. ultralytics/yolov5: v7.0 - YOLOv5 SOTA Realtime Instance Segmentation,” Zenodo, Nov 2022. Available online:
10.5281/zenodo.7347926 10.5281/zenodo.7347926 - Jiang P, Ergu D, Liu F, Cai Y. A review of YOLO algorithm developments. Procedia Comput Sci 2022;199:1066-73. [Crossref]
- Arunrukthavon P, Songsaeng D, Keatmanee C, Klabwong S, Ekpanyapong M, Dailey MN. Diagnostic performance of artificial ntelligence for interpreting thyroid cancer in ultrasound images. International Journal of Knowledge and Systems Science 2022;13:1-13. (IJKSS). [Crossref]
- Kunapinun A, Songsaeng D, Buathong S, Dailey MN, Keatmanee C, Ekpanyapong M. Explainable Automated TI-RADS Evaluation of Thyroid Nodules. Sensors (Basel) 2023;23:7289. [Crossref] [PubMed]
- Kim JA, Sung JY, Park SH. Comparison of faster-rcnn, yolo, and ssd for real-time vehicle type recognition. 2020 IEEE International Conference on Consumer Electronics - Asia (ICCE-Asia), Seoul, Korea (South), 2020:1-4.