Nomogram incorporating preoperative MRI-VASARI features for differentiating intracranial extraventricular ependymoma from glioblastoma
Introduction
Ependymoma is a primary tumor of the central nervous system (CNS) tumor that accounts for 2–9% of all intracranial tumors (1,2). Ependymomas have been classified by the World Health Organization based on histological and molecular features into the following categories: supratentorial ependymoma, posterior fossa ependymoma, spinal cord ependymoma, myxopapillary ependymoma, and subependymoma (3,4). Most ependymomas arise from the neoplastic transformation of ventricular ependymal cells, but a small number of ependymomas occurs in the brain parenchyma termed intracranial extraventricular ependymomas (IEEs) (5). IEEs account for 2–3% of intracranial gliomas and 7% of primary CNS tumors (6). On the contrary, glioblastomas (GBM) are much more common, accounting for 51% of all gliomas in the CNS (7). Notably, there are a few similarities between IEE and GBM regarding their overlapping clinical and imaging characteristics. More than 25% of adult ependymomas are misdiagnosed as other brain tumors, particularly as GBM, which affects treatment and prognosis (8-11). The 5- and 10-year overall survival rates for ependymoma are approximately 83% and 79% respectively (11). On the contrary, the median survival of patients with GBM is only 15 months after initial diagnosis and standard treatment. Despite maximum safe surgical resection and multimodal treatment, 70% of patients with GBM have recurrence (12). Recent data suggest the use of a personalized approach for treatment of GBM with targeted drugs, which is promising (13). Therefore, preoperative identification of IEE and GBM can facilitate optimal treatment planning and improve prognosis. Pathological confirmation from brain biopsy remains the only reliable method to diagnose IEE. Brain biopsy is invasive and is associated with a risk of hemorrhage, brain injury, tumor spreading along the needle path or cerebrospinal fluid, and misdiagnosis due to inadequate tissue sampling. New, non-invasive methods are necessary to achieve accurate preoperative differentiation between IEE and GBM.
There have been several studies reporting magnetic resonance imaging (MRI) features of IEE and GBM. IEE often presents relatively well-defined, large, heterogeneous, solid and cystic lesions with mild to moderate edema extending from the cortical surface to the edge of the ventricles, with foci of calcification and heterogeneous enhancement. In contrast, GBM rarely has calcification, is more heterogeneous, and has more extensive peritumoral edema (9,14,15). Clinical MRI reports usually include only a few imaging features such as location, size and enhancing characteristics of the brain tumors, which has limited efficacy in differentiating these two brain tumors. On the other hand, the MRI-Visually AcceSAble Rembrandt Images (VASARI) feature set as defined by The Cancer Imaging Archive (TCIA) utilizes a large number of defined visual features from conventional brain MRI and a standardized scoring system with controlled vocabulary to provide comprehensive standardized reports on brain tumors. MRI-VASARI has been used to diagnose primary brain tumors, predicting survival (16) and tumor progression (17). There are currently 30 morphological features described in this feature set, including the location and characteristics of the tumor components, as well as distinct features such as hemorrhage and pial invasion (18). This feature set has been used to describe tumor morphology and to predict the prognosis of GBM. For instance, Mazurowski et al. found that MRI-VASARI feature set improved the predictive power of survival models for patients with GBM (19). Verduin et al. found an integrated clinical and imaging prognostic model to be robust and of potential clinical relevance (16). In addition, the MRI-VASARI feature set was used to identify GBM and brain metastases (20). The MRI-VASARI feature set has also been used to predict histological grade and tumor progression of lower grade gliomas (21). Hyare et al. used the MRI- VASARI feature set and clinical variables in their model to predict isocitrate dehydrogenase (IDH) mutation status in grade II and grade III astrocytoma (22). However, the value of the imaging feature set for distinguishing IEE from GBM remains largely unknown.
In this study, we conducted a retrospective multicenter case-control study including patients with IEE (n=114) and GBM (n=258). Clinical data and preoperative MRI-VASARI features were assessed, and a multivariate logistic regression method was used to identify the valuable independent predictors that differentiate IEE and GBM. We hypothesized that a predictive model combining clinical and MRI-VASARI features could differentiate between IEE and GBM. The goal of this study was to develop an effective and non-invasive nomogram to diagnose IEE and GBM prior to surgery. We present this article in accordance with the TRIPOD reporting checklist (available at https://qims.amegroups.com/article/view/10.21037/qims-23-1148/rc).
Methods
Patients
This study included consecutive patients with IEE and GBM confirmed via surgical pathological specimen between June 2016 and June 2021 at participating hospitals. Eligibility criteria included the following: patients having pretreatment brain MRI scans, with IEE or GBM confirmed by surgical pathology, and having complete clinicopathological data. The exclusion criteria were as follows: (I) patients who had incomplete clinicopathological data; (II) patients with missing pretreatment MRI; (III) patients with gross motion or artifacts on pretreatment MRI. This cohort was divided into a training set and a validation set at a ratio of 7:3. A flow diagram of the study design is presented in Figure 1. Each patient had a set of clinical and MRI-VASARI imaging features. The clinical information included gender, age, Karnofsky performance status (KPS), and laboratory testing results for lymphocyte, monocyte, eosinophil counts, and serum creatinine. The clinical variables such as serum creatinine and eosinophils were included in the analysis of this cohort because prior studies have indicated the relevance of these variables for survival and prognosis in patients with GBM (23,24). We have previously published a study on the same cohort focusing on MRI imaging findings of IEE and GBM (25). The present study extended our research beyond imaging characteristics and tested a machine learning algorithm for differentiating IEE from GBM.
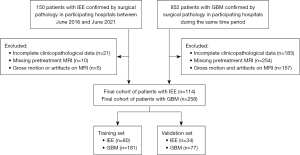
The study was conducted in accordance with the Declaration of Helsinki (as revised in 2013). This study was approved by the Ethics Committees of the First Affiliated Hospital of Guangxi Medical University [IRB No. 2022-KY-E-(236)], Nanning First People’s Hospital (IRB No. 2022-196-01), and Xiangya Hospital of Central South University (IRB No. 202303034). Informed consent was waived due to the retrospective nature of this study.
Preoperative brain MRI acquisition and assessment
Brain MRI scans were obtained using one of the following three scanners: 3 Tesla GE scanner (MR750w), 1.5 Tesla Siemens scanner (MAGNETOM Avanto or Sempra), or 3 Tesla Siemens scanner (MAGNETOM Verio). Routine standard brain MRI was performed, including T1-weighted images (T1WI), T1-weighted images with gadolinium contrast agent (T1WI+C), T2-weighted images (T2WI), and T2-weighted images with fluid-attenuated inversion recovery (T2-FLAIR). A field of view of 220×220 mm, a slice thickness of 5 mm, a matrix of 256×256, and slice spacing of 1 mm were used to acquire all sequences.
A total of 30 imaging features (F1–F30) were evaluated for all brain neoplasms using the MRI-VASARI feature set. Twenty-seven of the 30 features were used, while three (F26, F27, and F28) were excluded due to a lack of post-surgical MR images in some patients. A complete description of the VASARI feature set can be found in the reference (https://wiki.nci.nih.gov/display/CIP/VASARI). Two neuroradiologists (Xiangrong Li and Yuhong Qin, with 15 and 20 years of experience, respectively) independently reviewed the MRI images without knowledge of the clinicopathological findings. Imaging results were recorded by consensus. The final decision was made by a third experienced neuroradiologist (Liling Long, with more than 30 years of experience) in cases where consensus could not be reached. The inter- and intra-class correlation coefficients (ICCs) were used to test the reproducibility of VASARI feature evaluation. Two assessors evaluated the VASARI features from 30 randomly selected patients independently for testing the inter-class correlation coefficient. In addition, assessor 1 evaluated the VASARI features from the same patients again after a 2-week interval. An ICC greater than 0.75 indicated good agreement in feature evaluation.
Predictive modeling and performance evaluation
Significant clinical and MRI-VASARI features in the univariate analysis were included in the multivariate logistic regression analysis. Data from the univariate and multivariate analyses of VASARI features and clinical features are presented in the supplementary file (Table S1 and Table S2). The predictive model was constructed using multivariate logistic regression analysis. Backward stepwise selection was applied to select correlated factors for IEE. For this step, the likelihood ratio test with Akaike’s information criterion (AIC) was used as the stopping rule (26).
The following three models were built: (I) a clinical model using only clinical features as covariates, (II) an MRI-VASARI model using only selected VASARI features as covariates, and (III) a combined model incorporating the clinical features and MRI-VASARI features as covariates. These models were presented in a nomogram.
Receiver operating characteristic curve (ROC) analysis was performed in the training and validation cohorts to assess the performance of the predictive models, and the area under the curve (AUC) values were obtained. After that, the differences in performance among the VASARI model, clinic model and combined model were compared using MedCalc software using the DeLong test. The optimal model was visualized as a nomogram. Calibration curves were plotted on the training and validation sets to assess the nomogram. Finally, decision curve analysis was used to assess the clinical applications of these models and to calculate the anticipated benefit for predictive models across multiple threshold probabilities.
Statistical analysis
All statistical analyses were performed using IBM SPSS Statistics (versions 26.0, IBM), MedCalc Statistics (versions 20.0, IBM) and R statistical software (version 4.1.2; http://www.Rproject.org). The reported statistical significance levels were all two-sided, with statistical significance set at P<0.05. For modeling, all continuous parameters were first tested for normality using the Kolmogorov-Smirnov test. Different variables were compared using the Mann-Whitney test, t-test, and Chi-squared test. Univariate and multivariate binary logistic regressions were used to identify the independent predictors for distinguishing IEE from GBM. Predictive models for distinguishing IEE from GBM were established in the training set based on the selected independent predictors through binary logistic regression.
Results
Clinical data and MRI-VASARI characteristics
The training set had 80 pathologically confirmed IEE and 181 pathologically confirmed GBM cases, while the validation set had 34 pathologically confirmed IEE and 77 pathologically confirmed GBM cases (Table 1). The characteristics of the patients in the training and validation cohorts are presented in Table 1. The ICC of MRI-VASARI features extracted by assessors 1 and 2 in their first extraction ranged from 0.756 to 0.992. The ICC for both extractions performed by doctor 1 ranged from 0.740 to 0.986.
Table 1
Characteristics | Training set | Validation set | |||||
---|---|---|---|---|---|---|---|
IEE (n=80) | GBM (n=181) | P value | IEE (n=34) | GBM (n=77) | P value | ||
Demographics | |||||||
Gender, n (%) | 0.617 | 0.387 | |||||
Male | 56 (70.0) | 121 (66.8) | 22 (64.7) | 43 (55.8) | |||
Female | 24 (30.0) | 60 (33.1) | 12 (35.3) | 34 (44.2) | |||
Age [mean (SD), years] | 25.13 (20.64) | 47.20 (17.13) | <0.001 | 29.21 (18.46) | 46 (15.98) | <0.001 | |
Clinical characteristics [mean (SD)] | |||||||
KPS | 73.88 (7.20) | 85.52 (26.00) | <0.001 | 74.4 (8.24) | 88.0 (23.57) | 0.001 | |
Eosinophil count (μmol/L) | 0.19 (0.27) | 0.09 (0.10) | <0.001 | 0.17 (0.23) | 0.17 (0.49) | 0.973 | |
Serum creatinine (109/L) | 62.29 (30.03) | 84.26 (40.66) | <0.001 | 67.00 (19.69) | 84.44 (20.6) | <0.001 | |
VASARI features [mean (SD)] | |||||||
F3 (eloquent brain) | 1.78 (1.27) | 2.31 (1.17) | 0.001 | 1.32 (0.84) | 1.99 (1.26) | 0.006 | |
F4 (enhancement quality) | 2.83 (0.41) | 2.5 (0.53) | <0.001 | 2.68 (0.47) | 2.44 (0.53) | 0.028 | |
F7 (proportion necrosis) | 3.43 (0.81) | 4.94 (1.13) | <0.001 | 3.41 (0.89) | 4.7 (1.09) | <0.001 | |
F8 (tumor cysts) | 1.79 (0.41) | 1.9 (0.31) | 0.02 | 1.74 (0.45) | 1.9 (0.31) | 0.03 | |
F11 (thickness of margin) | 3.95 (0.27) | 3.34 (0.82) | <0.001 | 3.97 (0.17) | 3.32 (0.91) | <0.001 | |
F29 (tumor size) | 15.13 (4.19) | 10.96 (3.38) | <0.001 | 14.4 (4.69) | 10.6 (3.34) | <0.001 |
IEE, intracranial extraventricular ependymoma; GBM, glioblastoma; SD, standard deviation; KPS, Karnofsky performance status; VASARI, Visually AcceSAble Rembrandt Images.
IEE patients tended to be at a younger age of onset than GBM patients (IEE mean age =25±20.64 years vs. GBM mean age =47±17.13 years, P<0.001), having a relatively lower KPS score (73.88±7.20 vs. 85.52±26.00, P<0.001), a higher eosinophil count (0.19±0.27 vs. 0.09±0.10 µmol/L, P<0.001), and a lower level of serum creatinine [(62.29±30.03) vs. (84.26±40.66) ×109/L, P<0.001].
Compared with GBMs, IEEs were mostly located in non-functional brain areas; they were larger in size but smaller in the proportion of tumor parenchymal necrosis and cystic change, having more prominent tumor enhancement and a thicker enhancing margin. The typical IEE appearance included mixed cystic and solid appearance with the tumor parenchyma being slightly iso-to-low intensity on T1-weighted imaging, slightly moderate-to-high intensity on T2-weighted imaging, and moderate-to-high intensity on T2-FLAIR, as well as cystic degeneration and necrosis. IEE tended to show significant enhancement in the solid component after administration of contrast agent, but there was no enhancement in the cystic, necrotic or calcified regions.
Predictive modeling and model performance
Ten independent predictors were identified, including age (in years), KPS, eosinophil count, serum creatinine, eloquent brain (F3), enhancement quality (F4), proportion necrosis (F7), cysts (F8), thickness of enhancing margin (F11), and tumor size (F29). A nomogram was subsequently generated by incorporating these independent predictors (Figure 2).
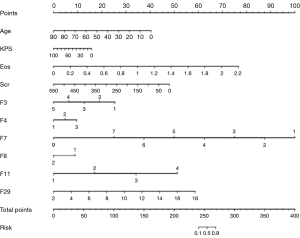
The combined model incorporating clinical features and MRI-VASARI features achieved an AUC of 0.99 [95% confidence interval (95% CI): 0.98–1.00] for the training cohort, and an AUC of 0.97 (95% CI: 0.94–1.00) for the validation cohort, respectively (Figure 3A,3B), as well as a good performance of consistency. A significantly lower AUC was observed for the model after elimination of MRI-VASARI features both in the training cohort (0.87, 95% CI: 0.82–0.91), and in the validation cohort (0.84, 95% CI: 0.77–0.92) (Figure 3A,3B) (P<0.05). Notably, incorporating the MRI-VASARI features into the predictive model showed significantly improved prediction efficiency (P<0.05). The calibration curves for the combined nomogram are presented in Figure 4.
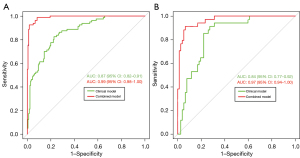
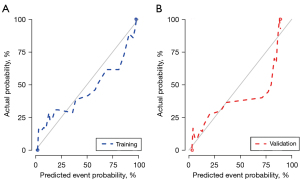
Clinical application
Decision curve analysis (DCA) was used to assess the clinical usefulness of the nomogram (Figure 5). If the threshold probability of a user exceeded 10%, then using the nomogram to predict IEE may be more helpful than using either the diagnose-all or diagnose-none schemes alone. Incorporating both clinical and MRI-VASARI features into a combined model showed additional clinical benefits compared to using the clinical data or the MRI-VASARI features alone.
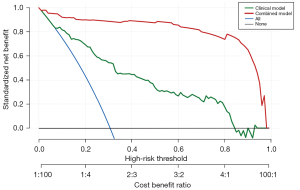
Discussion
We developed a nomogram for differentiating IEE from GBM utilizing the MRI -VASARI features from clinically acquired preoperative brain MRI images in a multi-center cohort. Our study presented the relevance of the MRI-VASARI features in characterizing brain tumors and improving diagnoses prior to surgery. The nomogram model combining VASARI parameters and clinical variables had a robust performance. To the best of our knowledge, our study was the first to build predictive models using MRI-VASARI features to differentiate IEE from GBM.
Our data showed improved performance of the predictive model after incorporating the MRI-VASARI features into the clinical model, with an AUC reaching 0.97. These data implicated the potential usefulness of MRI-VASARI features in differentiating IEE from GBM. Since the performance of a prediction model depended on whether key predictors were incorporated in building the model, our study implied that MRI-VASARI features were the key predictors which improved model performance. This study supported the notion that a nomogram based on tumor imaging and clinical variables could be used for preoperative diagnosis of IEE and GBM, which can assist in clinical decision making for diagnosis and treatment of the brain tumors if validated in a large prospective multicenter study.
This study identified several MRI-VASARI parameters as unique variables for IEE. Generally, IEE was noted to be mostly located in the non-functional brain area, with less necrosis than GBM. IEE originates from the rests of ependymal cells that are retained in the brain parenchyma during embryonic development (5). IEE is mostly located in the white matter area, which is in the non-functional brain area—the cortex rather than white matter is the area for most brain functions (20). In addition, IEE tends to have an expansive growth pattern, but GBM grows much faster. Since necrosis occurs when the nutritional demands of rapid growth exceed the supply of nutrients, IEE may be less susceptible to necrosis than GBM (27-29). It has been reported that the proliferation and apoptosis indices of tumor cells in GBM are higher than in ependymomas regardless of the location of the tumor (30). Therefore, it should not be surprising that the IEE in our cohort was more homogeneous in appearance and with less necrosis than GBM. In addition, our study depicted imaging features of ependymomas that were similar to those reported in the literature, such as heterogeneous solid and/or cystic lesions with mild to moderate edema extending from the cortical surface to the edge of the ventricles and having heterogeneous enhancement (9,15,31).
We found that IEE had thicker tumor enhancing margins and more intense enhancement than GBM. Tumor enhancement is associated with disruption of the blood-brain barrier and increased permeability of the microvasculature, which causes the tumor cells to take up more contrast media (32). Ependymomas have poor development of blood-brain barrier, which results in increased vascular permeability (33,34). Furthermore, ependymomas could overexpress vascular endothelial growth factor in areas where the disruption of blood-brain tumor barrier is evident (32,35-37). These changes to the vasculature may be the source of the increased enhancement observed on brain MRI images of IEEs.
This study also identified several clinical variables as predictors, which were included in the combined nomogram for predicting IEE preoperatively. We found that patients with IEE had a younger age of onset, a lower KPS score, a higher eosinophil count, and a lower serum creatinine level. Our results are consistent with literature regarding ependymoma usually occurring in children and adolescents, while GBM predominantly occurring in adults (1). The lower KPS scores implied relatively poor health in patients with IEE, which may be related to the large IEE tumors causing more severe hydrocephalus-related symptoms such as headache, nausea and vomiting. Eosinophils are thought to be associated with allergies and tumorigenesis. Although the mechanism by which eosinophils associated with tumorigenesis is unclear, another recent study has reported that eosinophil counts are reduced in patients with GBM, which may be associated with prognosis of GBM (38). Since serum creatinine reflects renal function and is closely related to survival (39), the differences in creatinine levels between IEE and GBM identified in our study may potentially affect prognosis and survival of patients with brain tumors. More study is needed to assess the underlying mechanism regarding why and how the serum creatinine level may affect patients with brain tumors.
We also found it intriguing for why only these six VASARI variables, i.e., eloquent brain (F3), enhancement quality (F4), proportion necrosis (F7), tumor cysts (F8), thickness of enhancing margin (F11) and tumor size (F29), were selected as independent predictors after both univariate and multivariate analyses. A recent VASARI study identified four variables being statistically significant for discerning the glioma grades and IDH status, i.e., F4 (enhancing quality), F5 (enhancing portion), F6 (non-enhancing portion), and F7 (necrosis) (40). Since our study was focused on differentiating IEE from GBM rather than glioma tumor grade, it should not be surprising that we found a unique set of six VASARI variables being the independent predictors. Future studies with additional information such as pathological and genetic analyses from surgical specimen may help to advance our knowledge about these brain tumors.
The present study had several limitations. First, this retrospective study spanned a long period of time, which led to unavailability of pathological specimens in some cases. We therefore could not perform further immunohistochemical analysis to identify molecularly heterogeneous ependymomas such as the Non-RELA(ZFTA)-fusion positive ependymomas (41). Second, our sample size was modest, and we could not perform additional analyses such as stratifying the data according to the pathological grade, tumor molecular data, comorbidities, etc. Third, our study had a potential risk for overfitting (42). A total of 10 predictors including six MRI-VASARI features and four clinical features in the nomogram were not optimal, which may decrease the statistical power of the prediction model because of the relatively limited sample size. This was especially critical for the 80 cases in the training set which had only eight cases per feature, below the 10 cases required to test one feature optimally. Nevertheless, the nomogram had a reasonable AUC in the validation set and performed well in the decision curve analysis, implicating our strategy being feasible although more studies should be done to validate our results. Fourth, this study was limited due to lack of comparison analysis between the imaging data and the accuracy of diagnosis by the tumor board, which is the current standard-of-care. We could not include the comparison analysis because of the limitations and challenges for such a retrospective multicenter study. The pre-operative clinical diagnoses from the tumor board for many cases were not documented. In addition, some of the cases were not discussed in tumor board prior to surgery. Therefore, the data for the comparison analysis were insufficient, precluding a meaningful statistical analysis. Fifth, the calibration curve in the validation cohort was less optimal. More research is needed to refine and validate the model performance in a prospective multicenter study with a larger sample size. Lastly, all imaging features identified in our study were derived from visual qualitative evaluation and were not reflective of objective quantitative parameters. Future studies should be performed with computational analysis such as radiomics, machine learning, and artificial intelligence to mine the quantitative imaging features for a more robust predictive modeling.
Conclusions
We have developed a non-invasive imaging approach that uses MRI images obtained during clinical care to differentiate IEE from GBM prior to surgery. This approach could benefit clinical decision-making and enhance the development of individualized treatment plans for brain tumors.
Acknowledgments
We thank staff members in the Departments of Radiology, Neurosurgery, and Pathology at all participating hospitals for their efforts in collecting the information used in this study, and for their helpful discussion and assistance in the data analysis and manuscript preparation. We thank Drs. Guanghui Gong, Qingling Li, and Hongling Yin from Xiangya Hospital, Central South University, Drs. Jingjing Zeng, Juan He, and Yiwu Dang from the Department of Pathology, First Affiliated Hospital of Guangxi Medical University for their assistance in the pathological analysis. We thank Xiangrong Li, Yuhong Qin and Liling Long from First Affiliated Hospital of Guangxi Medical University for their helpful suggestions in collecting the information. We thank our biostatistician Dr. Minxue Shen for the expert statistical assistance and review of our manuscript. Editing assistance was provided by our scientific writer Nancy Linford, PhD (City of Hope National Medical Center, Duarte, CA, USA). Preliminary data with an abstract was presented as an oral report at the European Congress of Radiology conference in Vienna, Austria, March 1-March 5, 2023.
Funding: This research was funded by
Footnote
Reporting Checklist: The authors have completed the TRIPOD reporting checklist. Available at https://qims.amegroups.com/article/view/10.21037/qims-23-1148/rc
Conflicts of Interest: All authors have completed the ICMJE uniform disclosure form (available at https://qims.amegroups.com/article/view/10.21037/qims-23-1148/coif). The authors have no conflicts of interest to declare.
Ethical Statement: The authors are accountable for all aspects of the work in ensuring that questions related to the accuracy or integrity of any part of the work are appropriately investigated and resolved. The study was conducted in accordance with the Declaration of Helsinki (as revised in 2013). This study was approved by the Ethics Committees of the First Affiliated Hospital of Guangxi Medical University [IRB No. 2022-KY-E-(236)], Nanning First People’s Hospital (IRB No. 2022-196-01), and Xiangya Hospital, Central South University (IRB No. 202303034). Informed consent was waived due to the retrospective nature of this study.
Open Access Statement: This is an Open Access article distributed in accordance with the Creative Commons Attribution-NonCommercial-NoDerivs 4.0 International License (CC BY-NC-ND 4.0), which permits the non-commercial replication and distribution of the article with the strict proviso that no changes or edits are made and the original work is properly cited (including links to both the formal publication through the relevant DOI and the license). See: https://creativecommons.org/licenses/by-nc-nd/4.0/.
References
- Jia Z, Yan Y, Wang J, Yang H, Zhan H, Chen Q, He Y, Huang C, Hu Y. Development and validation of prognostic nomogram in ependymoma: A retrospective analysis of the SEER database. Cancer Med 2021;10:6140-8. [Crossref] [PubMed]
- Alshaya W, Mehta V, Wilson BA, Chafe S, Aronyk KE, Lu JQ. Low-grade ependymoma with late metastasis: autopsy case study and literature review. Childs Nerv Syst 2015;31:1565-72. [Crossref] [PubMed]
- Rudà R, Bruno F, Pellerino A, Soffietti R. Ependymoma: Evaluation and Management Updates. Curr Oncol Rep 2022;24:985-93. [Crossref] [PubMed]
- Louis DN, Perry A, Wesseling P, Brat DJ, Cree IA, Figarella-Branger D, Hawkins C, Ng HK, Pfister SM, Reifenberger G, Soffietti R, von Deimling A, Ellison DW. The 2021 WHO Classification of Tumors of the Central Nervous System: a summary. Neuro Oncol 2021;23:1231-51. [Crossref] [PubMed]
- Yuh EL, Barkovich AJ, Gupta N. Imaging of ependymomas: MRI and CT. Childs Nerv Syst 2009;25:1203-13. [Crossref] [PubMed]
- Lester A, McDonald KL. Intracranial ependymomas: molecular insights and translation to treatment. Brain Pathol 2020;30:3-12. [Crossref] [PubMed]
- Louis DN, Perry A, Reifenberger G, von Deimling A, Figarella-Branger D, Cavenee WK, Ohgaki H, Wiestler OD, Kleihues P, Ellison DW. The 2016 World Health Organization Classification of Tumors of the Central Nervous System: a summary. Acta Neuropathol 2016;131:803-20. [Crossref] [PubMed]
- Rudà R, Reifenberger G, Frappaz D, Pfister SM, Laprie A, Santarius T, Roth P, Tonn JC, Soffietti R, Weller M, Moyal EC. EANO guidelines for the diagnosis and treatment of ependymal tumors. Neuro Oncol 2018;20:445-56. [Crossref] [PubMed]
- Leng X, Tan X, Zhang C, Lin H, Qiu S. Magnetic resonance imaging findings of extraventricular anaplastic ependymoma: A report of 11 cases. Oncol Lett 2016;12:2048-54. [Crossref] [PubMed]
- Stupp R, Mason WP, van den Bent MJ, Weller M, Fisher B, Taphoorn MJ, et al. Radiotherapy plus concomitant and adjuvant temozolomide for glioblastoma. N Engl J Med 2005;352:987-96. [Crossref] [PubMed]
- Prados MD, Berger MS. Textbook of Neuro-Oncology, 1st ed. Elsevier Saunders: Philadelphia, PA, USA 2005; ISBN 978-0-7216-8148-1.
- Davis ME. Glioblastoma: Overview of Disease and Treatment. Clin J Oncol Nurs 2016;20:S2-8. [Crossref] [PubMed]
- Khabibov M, Garifullin A, Boumber Y, Khaddour K, Fernandez M, Khamitov F, Khalikova L, Kuznetsova N, Kit O, Kharin L. Signaling pathways and therapeutic approaches in glioblastoma multiforme Int J Oncol 2022;60:69. (Review). [Crossref] [PubMed]
- Shintaku M, Hashimoto K. Anaplastic ependymoma simulating glioblastoma in the cerebrum of an adult. Brain Tumor Pathol 2012;29:31-6. [Crossref] [PubMed]
- Jabeen S, Konar SK, Prasad C, Mahadevan A, Beniwal M, Sadashiva N, Santosh V, Saini J. Conventional and Advanced Magnetic Resonance Imaging Features of Supratentorial Extraventricular Ependymomas. J Comput Assist Tomogr 2020;44:692-8. [Crossref] [PubMed]
- Verduin M, Primakov S, Compter I, Woodruff HC, van Kuijk SMJ, Ramaekers BLT, et al. Prognostic and Predictive Value of Integrated Qualitative and Quantitative Magnetic Resonance Imaging Analysis in Glioblastoma. Cancers (Basel) 2021.
- Peeken JC, Hesse J, Haller B, Kessel KA, Nüsslin F, Combs SE. Semantic imaging features predict disease progression and survival in glioblastoma multiforme patients. Strahlenther Onkol 2018;194:580-90. [Crossref] [PubMed]
- Gutman DA, Cooper LA, Hwang SN, Holder CA, Gao J, Aurora TD, et al. MR imaging predictors of molecular profile and survival: multi-institutional study of the TCGA glioblastoma data set. Radiology 2013;267:560-9. [Crossref] [PubMed]
- Mazurowski MA, Desjardins A, Malof JM. Imaging descriptors improve the predictive power of survival models for glioblastoma patients. Neuro Oncol 2013;15:1389-94. [Crossref] [PubMed]
- Meier R, Pahud de Mortanges A, Wiest R, Knecht U. Exploratory Analysis of Qualitative MR Imaging Features for the Differentiation of Glioblastoma and Brain Metastases. Front Oncol 2020;10:581037. [Crossref] [PubMed]
- Zhou H, Vallières M, Bai HX, Su C, Tang H, Oldridge D, Zhang Z, Xiao B, Liao W, Tao Y, Zhou J, Zhang P, Yang L. MRI features predict survival and molecular markers in diffuse lower-grade gliomas. Neuro Oncol 2017;19:862-70. [Crossref] [PubMed]
- Hyare H, Rice L, Thust S, Nachev P, Jha A, Milic M, Brandner S, Rees J. Modelling MR and clinical features in grade II/III astrocytomas to predict IDH mutation status. Eur J Radiol 2019;114:120-7. [Crossref] [PubMed]
- Madhugiri VS, Venkatesan S, Dutt A, Moiyadi AV, Shetty P, Gupta T, Epari S, Jalali R, Sasidharan GM, Kumar VRR, Ganesh CVS, Ramesh AS, Prabhu AS, Dutt AK. An Analysis of Eosinophil- and Basophil-Based Indices in Patients with Glioblastoma and their Correlation with Survival. World Neurosurg 2023;170:e292-300. [Crossref] [PubMed]
- Chen CY, Lirng JF, Chan WP, Fang CL. Proton magnetic resonance spectroscopy-guided biopsy for cerebral glial tumors. J Formos Med Assoc 2004;103:448-58.
- Li L, Fu Y, Zhang Y, Mao Y, Huang D, Yi X, Wang J, Tan Z, Jiang M, Chen BT. Magnetic resonance imaging findings of intracranial extraventricular ependymoma: A retrospective multi-center cohort study of 114 cases. Cancer Med 2023;12:16195-206. [Crossref] [PubMed]
- Yamashita T, Yamashita K, Kamimura R. A Stepwise AIC Method for Variable Selection in Linear Regression. Commun Stat Theory Methods 2007;36:2395-403.
- Carson KA, Grossman SA, Fisher JD, Shaw EG. Prognostic factors for survival in adult patients with recurrent glioma enrolled onto the new approaches to brain tumor therapy CNS consortium phase I and II clinical trials. J Clin Oncol 2007;25:2601-6. [Crossref] [PubMed]
- Xing Z, Yang X, She D, Lin Y, Zhang Y, Cao D. Noninvasive Assessment of IDH Mutational Status in World Health Organization Grade II and III Astrocytomas Using DWI and DSC-PWI Combined with Conventional MR Imaging. AJNR Am J Neuroradiol 2017;38:1138-44. [Crossref] [PubMed]
- She D, Liu J, Xing Z, Zhang Y, Cao D, Zhang Z. MR Imaging Features of Anaplastic Pleomorphic Xanthoastrocytoma Mimicking High-Grade Astrocytoma. AJNR Am J Neuroradiol 2018;39:1446-52. [Crossref] [PubMed]
- Wagemakers M, Sie M, Hoving EW, Molema G, de Bont ES, den Dunnen WF. Tumor vessel biology in pediatric intracranial ependymoma. J Neurosurg Pediatr 2010;5:335-41. [Crossref] [PubMed]
- Mangalore S, Aryan S, Prasad C, Santosh V. Imaging characteristics of supratentorial ependymomas: Study on a large single institutional cohort with histopathological correlation. Asian J Neurosurg 2015;10:276-81. [Crossref] [PubMed]
- van Tellingen O, Yetkin-Arik B, de Gooijer MC, Wesseling P, Wurdinger T, de Vries HE. Overcoming the blood-brain tumor barrier for effective glioblastoma treatment. Drug Resist Updat 2015;19:1-12. [Crossref] [PubMed]
- Groothuis DR, Vriesendorp FJ, Kupfer B, Warnke PC, Lapin GD, Kuruvilla A, Vick NA, Mikhael MA, Patlak CS. Quantitative measurements of capillary transport in human brain tumors by computed tomography. Ann Neurol 1991;30:581-8. [Crossref] [PubMed]
- Bhowmik A, Khan R, Ghosh MK. Blood brain barrier: a challenge for effectual therapy of brain tumors. Biomed Res Int 2015;2015:320941. [Crossref] [PubMed]
- Korshunov A, Golanov A, Timirgaz V. Immunohistochemical markers for prognosis of ependymal neoplasms. J Neurooncol 2002;58:255-70. [Crossref] [PubMed]
- Fischer I, Gagner JP, Law M, Newcomb EW, Zagzag D. Angiogenesis in gliomas: biology and molecular pathophysiology. Brain Pathol 2005;15:297-310. [Crossref] [PubMed]
- Kurt E, Zheng PP, Hop WC, van der Weiden M, Bol M, van den Bent MJ, Avezaat CJ, Kros JM. Identification of relevant prognostic histopathologic features in 69 intracranial ependymomas, excluding myxopapillary ependymomas and subependymomas. Cancer 2006;106:388-95. [Crossref] [PubMed]
- Huang Z, Wu L, Hou Z, Zhang P, Li G, Xie J. Eosinophils and other peripheral blood biomarkers in glioma grading: a preliminary study. BMC Neurol 2019;19:313. [Crossref] [PubMed]
- Kellum JA, Sileanu FE, Murugan R, Lucko N, Shaw AD, Clermont G. Classifying AKI by Urine Output versus Serum Creatinine Level. J Am Soc Nephrol 2015;26:2231-8. [Crossref] [PubMed]
- Gemini L, Tortora M, Giordano P, Prudente ME, Villa A, Vargas O, Giugliano MF, Somma F, Marchello G, Chiaramonte C, Gaetano M, Frio F, Di Giorgio E, D'Avino A, Tortora F, D'Agostino V, Negro A. Vasari Scoring System in Discerning between Different Degrees of Glioma and IDH Status Prediction: A Possible Machine Learning Application? J Imaging 2023;9:75. [Crossref] [PubMed]
- Fukuoka K, Kanemura Y, Shofuda T, Fukushima S, Yamashita S, Narushima D, et al. Significance of molecular classification of ependymomas: C11orf95-RELA fusion-negative supratentorial ependymomas are a heterogeneous group of tumors. Acta Neuropathol Commun 2018;6:134. [Crossref] [PubMed]
- Moons KGM, Wolff RF, Riley RD, Whiting PF, Westwood M, Collins GS, Reitsma JB, Kleijnen J, Mallett S. PROBAST: A Tool to Assess Risk of Bias and Applicability of Prediction Model Studies: Explanation and Elaboration. Ann Intern Med 2019;170:W1-W33. [Crossref] [PubMed]