Functional magnetic resonance imaging techniques and their development for radiation therapy planning and monitoring in the head and neck cancers
Introduction
Head and neck cancer (HNC) represents a broad spectrum of malignant neoplasms that include those in the sinonasal region, pharynx and larynx, oral cavity, glands and lymphatic system of the neck, and is the sixth most common type of cancer worldwide (1). Over 90% HNCs are squamous cell carcinomas (SCC) and variants. Radiation therapy (RT) remains an important mainstay among treatment strategies in HNC. The progressive introduction of intensity-modulated radiation therapy (IMRT) into the clinics has revolutionized the treatment of HNC over the past 10 years. The ability of IMRT to tightly conform the delivered dose to the irregularly shaped tumors with steep dose gradients enables better treatment efficacy than conventional RT approaches, meanwhile leading to a substantial reduction in normal tissue irradiation and hence the significantly improved normal tissue sparing, e.g., the reduced acute mucositis and late xerostomia (2).
The achieved success and further advancement of highly conformable IMRT rely continuously and heavily on clinical imaging (3). The frequent use of clinical imaging has been propagated into every aspect of HNC radiotherapy treatment, including but not limited to treatment simulation, planning and re-planning, setup verification, dose delivery guidance, motion tracking, and treatment prediction and/or evaluation. Computerized tomography (CT) has long been the workhorse in the arena of radiotherapeutic imaging, in particular for simulation scan and treatment planning by taking the advantages of excellent image resolution, high geometric fidelity, fast scan speed and ease of dose calculation. On the other hand, CT has its own drawbacks and is not able to fully fulfill the requirements imposed by the further advancement of highly conformable IMRT. As such, multi-modality clinical imaging, such as ultrasound, magnetic resonance imaging (MRI), positron emission tomography (PET) and PET/CT, has been proposed and introduced into RT practice to complement CT. MRI, long been a valuable and powerful tool in diagnostic radiology, has increasingly gained more interest from radiation oncology community in recent years. Similar to CT, MRI is also an important and powerful cross-sectional anatomical imaging method that not only reveals the fine structure of tissues, but involves no ionizing radiation, so is attractive for the serial imaging over the entire course of RT without the extra radiation dose being delivered to patients. More importantly, MRI offers superior soft tissue contrast among existing clinical imaging modalities. Furthermore, MRI also provides various functional imaging techniques to facilitate utilization in many important concepts in radiation oncology like biological target volume (BTV), dose painting and adaptive IMRT (4).
The use of anatomical MRI for RT can never be understated, particularly for target and organ-at-risk (OAR) delineation in treatment planning. In the current practice, target and OAR delineation is mostly conducted on axial CT images. Large uncertainties have been found to be associated with target delineation on CT images in head and neck owing to the relatively poor soft tissue contrast of CT images (5,6). As such, it is expected that such delineation uncertainty could be greatly reduced with the aid of anatomical MR images fused to CT, by taking the advantage of superior soft tissue contrast of MRI (7). For anatomical MRI in the head and neck, T2-weighted fast spin echo (FSE) with fat suppression and post-contrast T1-weighted FSE with/without suppression are two most common MRI sequences in diagnostic radiology, and are routinely used for radiotherapy planning. Great efforts have been taken to investigate the merit of anatomical MRI for better target delineation in the head and neck and encouraging results have been reported (8-13).
In addition to anatomical MRI, functional MRI techniques are being proposed and introduced into clinical practice of radiation oncology. The major purpose of this paper is to give a concise introduction and a timely review of functional MRI techniques that are being investigated for head and neck radiotherapy and some new techniques that are not yet explored but may impact head and neck radiotherapy in the future, for multi-disciplinary researchers such as medical physicists, radiotherapist, radiation oncologist, who may not be familiar with functional MRI but have interests in applying these techniques into their clinical RT practice. We will primarily discuss the acquisition and analysis of these functional MRI techniques instead of the post-processing aspects such as segmentation and registration to CT, although they are also indispensable parts in radiotherapy treatment planning and positional verification.
Functional MRI techniques
Diffusion-weighted imaging (DWI)
DWI is an important and valuable non-invasive functional MR technique that can be used to measure diffusivity, i.e., the Brownian motion of water, in the living tissue (14). In DWI acquisition, a strong bi-polar gradient waveform is used to obtain the diffusion-weighted magnetization preparation. The Brownian motion of water in the tissue cannot be completely rephrased by this diffusion-weighted bi-polar gradient and results in the MR signal intensity reduction (15). The diffusion-sensitizing effect by this bi-polar gradient waveform is usually measured by a parameter called b-value that is defined by the shape, strength and duration of the gradient lobe and the time interval between two opposed gradient lobes. The displacement of freely mobile water molecules diffusing from one location to another in a certain time is considered to have a Gaussian distribution for Brownian motion. Based on this Gaussian diffusion behavior, a relationship of mono-exponential decay of DWI signal intensity with regard to the increase of b-value can be established. By fitting the DWI signal decay with regard to b-value to a mono-exponential function, a quantitative index called apparent diffusion coefficient (ADC) can be calculated to indicate the average diffusivity of tissues. In oncological applications, malignant tumor is usually associated with low ADC value due to the increased cellularity and the decreased extra-vascular extra-cellular space (EES) that restrict the Brownian motion of water (14,16,17).
DWI has been proved to be a valuable tool in many aspects of HNC management by a number of studies, including but not limited to tumor detection and characterization, nodal staging, treatment response prediction and post-treatment recurrence evaluation (18-20). For target delineation in head and neck radiotherapy planning, DWI is of value in the identification of metastatic lymph nodes, in particular for small-sized lymph nodes, so as to determine the extent of radiation treatment field (21). Lymph node metastases in the head and neck are mainly based on size criteria of anatomical imaging. A short axis diameter cut-off of 10 mm is commonly used to discriminate metastatic lymph nodes from benign ones. However, according to histopathology, small-sized nodes may also harbor malignancy and excluding these nodes from treatment planning could lead to regional recurrences. Many studies have investigated the performance of DWI on lymph node discrimination in the head and neck. Although various b-values and ADC threshold vary between studies, high sensitivity and high specificity have been reported (21-26). In three studies involving nodal size smaller than 10 mm, the sensitivity and specificity were reported as 84–98% and 88–97%, respectively, much superior to anatomical CT and MRI (7% sensitivity) (21-23). Dirix et al. (27) also compared DWI- and CT-based target volume delineation to pathology. They found that DWI achieved significantly better agreement with pathology than CT (k=0.97 vs. 0.56, P=0.019) (27). As such, DWI-based target delineation is expected to reduce the toxicity induced by the radiotherapy or intensify the treatment on sub-millimeter malignant nodes.
DWI has also been investigated to aid in prediction and evaluation of RT and chemoradiotherapy (CRT) in the head and neck (Figure 1). The ability to predict tumor response before or early during treatment helps to better initiate and adapt RT planning for the purpose of better treatment outcome and reduced toxicity on the individual basis. It is postulated that the reduction of tumor cells and necrosis induced by RT leads to an increase in tumor ADC. Some published data suggest that the pre-treatment baseline ADC of complete responders is significantly lower than the ADC of partial responders (28-30). The ADC increase within/after the treatment of complete responders is also significant when compared to the pre-treatment ADC, while non-responders are more associated with a lower increase or even a decrease of ADC during the treatment (28,31-35). In addition, The ADC change might be a more sensitive and accurate biomarker to predict locoregional control (LRC) than the volume change of tumors (36).
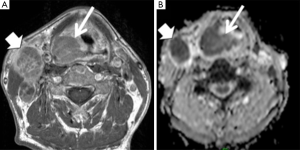
It can be difficult for anatomical imaging to differentiate benign post-treatment tissues alteration like necrosis from residual/recurrent cancer (37). As such, post-treatment DWI can help predict or detect the potential recurrence of HNC. A couple of studies have shown that the ADC of residual or recurrent SCC is significantly lower than that of a benign post-treatment mass (38-40). Sensitivities ranging from 84% to 94% and specificities ranging from 90% to 95% have been reported by using a threshold ADC of 1.3×10−3 mm2/sec (39,40). In particular, as DWI is insensitive to acute inflammation induced by the treatment, DWI might provide advantages over fluorodeoxyglucose (FDG) PET on detection of residual cancer and early reoccurrence in the first few months after treatment (41). Much higher positive predictive values (PPV) of DWI (91–100%) than PPV of PET (64–77%) have been reported in literatures (42,43).
ADC is so far one most frequently used quantitative index for DWI in clinical practice as its calculation has been well integrated with almost all commercial clinical MRI scanners. ADC is calculated based on the assumption of Gaussian diffusion behavior. However, the diffusion behavior in living tissues can be non-Gaussian due to their complex structures that greatly restrict the free diffusion of water. It has been found that the mono-exponential function for ADC calculation does not necessarily best represent the observed DWI signal decay in the living tissues if an extended b-value combination is used. A number of non-Gaussian DWI models have been proposed (44-50). Various quantitative parameters can be derived from these non-Gaussian DWI models other than ADC (Figure 2). These parameters are anticipated to more accurately quantify the diffusivity of the living tissue and provide additional information of tissue. There have been increasing numbers of studies of non-Gaussian DWI for HNC (51-57). Jansen et al. found that diffusion kurtosis imaging (DKI) model significantly better fit the DWI signal decay in HNSCC with an extended b-value up to 1,500 s/cm2 (55). A parameter of apparent kurtosis coefficient Kapp could be calculated from DKI to indicate the deviation of diffusion from Gaussian pattern in living tissues (55). Intravoxel incoherent motion (IVIM) model explores the non-Gaussian DWI at extremely low b-values (<200 s/cm2) to investigate the micro-circulation in tissues as the blood flow within the randomly oriented capillary network can be considered as pseudo-diffusion with a larger “diffusion” coefficient. By using a bi-exponential function for DWI signal fitting, three parameters of D, D* and f could be calculated to indicate the true diffusion coefficient, pseudo-diffusion coefficient due to micro-circulation and the vascularity volume fraction due to micro-circulation (50). IVIM may hold potentials for targeting at cancer angiogenesis. Lai et al. used IVIM to aid the differentiation of NPC and post-chemoradiation fibrosis (53). Lu et al. compared primary tumors and metastatic nodes in HNC patients who received CRT using IVIM parameters and suggested that IVIM might be useful in optimizing treatment planning (51). Besides DKI, stretched exponential model (SEM) can be used to characterize non-Gaussian diffusion using very high b-values, and two parameters of distributed diffusion coefficient DDC and water heterogeneity α can be obtained (49). Lai et al. used SEM for different stages of NPC and found that α was robust and could potentially help in staging and grading prediction in NPC (54).
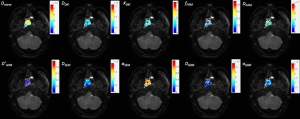
For both Gaussian and non-Gaussian DWI, one major limitation is the relatively low spatial resolution, severe distortion and various artifacts associated with the most widely adopted single-shot echo planar imaging (EPI) pulse sequence for DWI acquisition, in particular for the head and neck due to the complicated tissue structures and various motions within this area. For ADC quantification, the maximum b-value applied is usually below 1,000 sec/mm2 and at least two b-values are needed. In contrast, for non-Gaussian DWI, more b-factors are required in an extended range to capture non-Gaussian behavior. For example, a couple of b-values below 200 sec/mm2 may be used for IVIM and high b-values over 1,000 sec/mm2 are required for DKI. The number and value of b-factors can affect scan time, image quality as well as quantification, so have to be carefully selected and optimized, which need to be further investigated. The development of multi-shot EPI and non-EPI-based DWI is expected to alleviate these issues while at the cost of longer scan time (58,59). Nevertheless, possible deviations in diffusivity measurement by using non-EPI sequences from EPI have yet to been carefully examined.
Compared to Gaussian DWI and ADC, the use of non-Gaussian DWI in RT application in the head and neck is still in its infancy. The published data are still sparse. The prescription of b-factors covering extremely low and high b-values might not be readily allowable on all clinical MRI systems. Meanwhile, fitting and calculation of non-Gaussian parameters can be complicated and have to be done off-line. Furthermore, non-Gaussian DWI quantification might be associated with higher inaccuracy and uncertainty. Therefore, more rigorous studies are anticipated to establish the utilization of on-Gaussian DWI for RT in the head and neck.
Perfusion MRI
Perfusion is physiologically defined as the steady-state delivery of blood to an element of tissue (60). Perfusion emphasizes more on the capillary blood blow and its contact with and irrigation through tissues rather than the luminal blood flow in the large blood vessels. Perfusion can be measured by various clinical imaging methods including MRI. The advantages of MRI perfusion include free-of-radiation, versatility and non-invasiveness possibility compared to other imaging modalities.
DCE-MRI is most frequently used for oncological applications among perfusion MRI methods to investigate various tissue properties such as the microstructure, permeability, tumor angiogenesis and hypoxia (61). Due to the T1-shortening effect after gadolinium (Gd)-based contrast agent injection, the uptake and depletion of Gd by target tissue induces T1-weighted MR signal enhancement and reduction during the perfusion procedure, and these temporal MR signal variation is recorded as voxel-wise time-intensity curves (TIC) by acquiring a series of dynamic MR images using a fast 2D/3D spoiled gradient echo sequence.
DCE-MRI data can be analyzed qualitatively, semi-quantitatively or quantitatively. Qualitative DCE-MRI analysis relies on the observation and categorization of TIC patterns to discriminate malignant and benign lesions. TICs of malignant lesions more frequently show a fast wash-in and fast wash-out TIC pattern which is associated with tumor neoangiogenesis (62). TIC pattern analysis is widely used in breast cancer MRI and has been extended to HNC (Figure 3) (62-66). Semi-quantitative analysis of TIC can be conducted to derive various heuristic parameters to indicate the tissue perfusion property, such as bolus arrival time, time-to-peak, wash-in rate, wash-out rate and maximum enhancement (67). Despite the advantages of straightforward and easy analysis, qualitative and semi-quantitative DCE-MRI suffers from many limitations. For instance, semi-quantitative parameters can be remarkably affected by intrinsic tissue relaxation times, contrast agent registration protocol as well as imaging parameters.
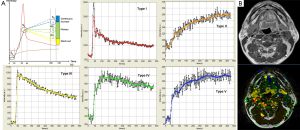
Quantitative DCE-MRI was thus proposed to overcome the above problems based on pharmacokinetic modeling of DCE-MRI signal to set up the quantitative relationship between tissue properties and absolute contrast agent concentration in the tissue (68,69). As such, the conversion of TIC to time-concentration curve (TCC) from DCE-MRI data is essential for quantitative DCE-MRI, which requires a pre-contrast T1 measurement in DCE-MRI acquisition. Among the various pharmacokinetic models proposed for DCE-MRI, the Tofts model is most widely adopted (68,70). For the original Tofts model, three quantitative pharmacokinetic parameters of Ktrans, (the volume transfer constant between the blood plasma and extracellular extravascular space EES, wit unit of 1/min), kep (rate constant between EES and blood plasma, also with unit of 1/min) and ve (volume of EES per unit volume of tissue, ve = Ktrans/kep) could be derived. An additional parameter of vp (volume of blood plasma per unit volume of tissue) can be derived from the extended Tofts model. Despite the theoretical advantage of hardware and imaging protocol independence of quantitative pharmacokinetic parameters, it is still worth noting that the quantification of these parameters can be complicated and the quantified results can be considerably affected by many factors such as arterial input function (AIF) selection and measurement, T1 mapping, hardware imperfections (B0 and B1 inhomogeneity), hematocrit and model fitting algorithms (70). In consequence, reproducibility of quantitative DCE-MRI still needs to be further established through rigorous studies.
The merit and potential of DCE-MRI have been reported by a number of studies for RT and/or CRT applications, mainly focused on prediction and early assessment of RT and CRT treatment response (Figure 4). Hoskin et al. (67) studied the correlation of pre- and post-treatment semi-quantitative DCE-MRI parameters and local tumor control after accelerated RT in HNCs. Cao et al. (71) found that the blood volume increase derived from the Tofts model in available primary tumor during the early course of RT was associated with local control, thus having potentials on intra-treatment modification. Shukla-Dave’s study showed that skewness of Ktrans was the strongest predictor of progression free survival (PFS) and overall survival (OS) in stage IV HNSCC patients with nodal disease, suggesting the importance of pretreatment Ktrans as a predictor of treatment outcome (72). Several other studies also reported that higher overall Ktrans or its increase was predictive of a good outcome following treatment in HNSCC (73-76).
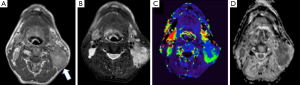
As tumor vascularity could serve as a possible surrogate marker of hypoxia, DCE-MRI also has potential to reflect tumor hypoxia, which is attractive for adaptive and personalized radiotherapy treatment planning because hypoxia is a key factor to determine tumor resistance to treatment (77). In HNC, Newbold et al. (78) reported that Tofts model parameters of Ktrans, kep and ve in a small number of 11 tumor sites had relatively high correlation (r>0.5) with higher pimonidazole scores (>30% of cells), a hypoxia-specific marker used in immunohistochemistry. In another study by Donaldson et al. (79), pharmacokinetic DCE-MRI derived pre-surgical whole-tumor blood flow was found to be negatively correlated with pimonidazole immunostaining and vascular endothelial growth factor (VEGF) messenger RNA (mRNA) expression in the resected HNSCC specimens. It was also reported by Jansen et al. that baseline nodal Ktrans and kep correlated inversely with the proliferation marker Ki67 in the lymph nodes of 12 HNSCC patients. After applying Bonferroni correction, kep was found to be correlated with VEGF expression (80).
DSC-MRI, another type of perfusion MRI, utilizes the T2*-weighted signal loss induced by the susceptibility effect of the injected paramagnetic contrast agent to investigate tissue perfusion (81). Similar to DCE-MRI, qualitative, semi-quantitative and quantitative analysis can be conducted on DSC-MRI data. For quantitative pharmacokinetic analysis, voxel-wise cerebral blood volume (CBV), cerebral blood flow (CBF) and mean transit time (MTT) can be derived. Up to date, DSC-MRI has been focusing on diagnosis rather than therapeutic purpose, sparsely reported for head and neck applications in literatures compared to DCE-MRI (82).
Totally non-invasive perfusion MRI can be conducted by arterial spin labeling (ASL), which explores the use of magnetically labeled arterial blood by labeling RF pulses in feeding vessels to generate endogenous perfusion-weighted image contrast in the target tissue so that exogenous contrast agent does not need to be injected intravenously (83). Through delicate pharmacokinetic modeling, perfusion parameters of CBF, CBV and MTT could theoretically be obtained (84). One major limitation of ASL is its low labeling efficiency and thus low contrast-to-noise ratio (CNR). Until very recently, few studies have reported the use of ASL in the head and neck. Fujima et al. evaluated the feasibility of ASL in 27 patients with HNC by comparing the post-treatment TBF (tumor blood flow) and the reduction rate of TBF between residual and nonresidual tumors after CRT treatment. The result showed that TBF reduction rate was significantly lower in patients with residual tumors, suggesting the potential use of ASL to assess tumor viability in the HNC in the future (85). They further compared the TBF measurement using pseudo-continuous arterial spin labeling (pCASL) to that using DCE-MRI in 18 HNSCC patients. Significant correlation was observed between the absolute TBF values derived from two perfusion MRI methods, although the TBF might be underestimated by ASL in the central tumor ROIs (86).
MR spectroscopy (MRS)
Different from MR imaging in which the spatial distribution of water proton in the living tissue is visualized via spatial encoding in three dimensions, MRS usually aims to non-invasively investigate the concentration of metabolites resonating at different frequencies from water at a specific location within the living tissue in the form of MR spectrum (87). The acquisition and analysis of MRS data are quite different from the standard normal MR imaging methods. Water signal suppression is essential for MRS acquisition because water proton signal is much stronger than the proton signal from metabolites due to the much smaller concentration of these metabolites. Pointed-resolved spectroscopy (PRESS) or stimulated echo acquisition mode (STEAM) sequence is usually applied to acquire the spectrum data within a spatially selected voxel in the target tissue. The acquired MRS data are then Fourier transformed and post processed to obtain the MR spectrum in a plot of proton signal amplitude (indicating the relative concentration) against the resonant frequency in ppm (part per million), or chemical shift. The metabolites that can be investigated via MRS methods include but are not limited to N-acetylaspartate (NAA), choline (Cho), creatine (Cr), lactate, glutamate (Glu), glutamine (Gln) and lipids. Different metabolites are involved in different physiological and pathological procedures, so can be utilized to characterize the metabolism associated with different diseases including cancer (88). MRS has been preliminarily explored for HNC in recent years (Figure 5) (89-93).
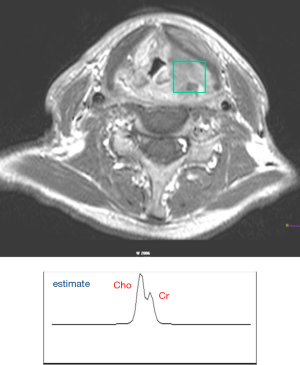
For RT/CRT applications, Bezabeh et al. explored the capability of MRS in providing an indication of the aggressiveness of HNSCC tumors and their response to treatment (94). By comparing the MRS of ex vivo HNSCC tumor specimens from patients with and without treatment failure, they found the Cho-to-Cr (3.2/3.0 ppm) and the 1.3/0.9 ppm spectral intensity ratios (signal due to lipid or lactic acid) were significantly elevated in the patients with poor response. King et al. correlated the pre-treatment and intra-treatment Cho values as well as the Cho change with loco-regional failure, distant metastases, OS, and cancer-related death in 60 HNSCC patients who received RT/CRT and found that MRS did not successfully predict clinical outcome (95). They found in another study that the presence of Cho in a post-treatment mass may serve as a marker of residual cancer but the change in Cho ratios may not be useful for monitoring treatment response (96). Although it is technically possible to obtain spectroscopic imaging in 2D/3D, also called chemical shift imaging (CSI), the scan time can become very long and the data qualify can be deteriorated by many factors, so its use in clinical practice is very limited (97). Besides proton MRS (or 1H MRS), phosphorus MRS (31P MRS) has also been reported for the head and neck applications as phosphorus compounds are involved in energy metabolism of HNC. Maldonado et al. (98) reported that the average pretreatment ratio of phosphomonoesters (PME) to β-nucleotide triphosphates (β-NTP) of 31P MRS was significantly smaller in complete responders to CRT, suggesting the value of 31P MRS for treatment prediction. Chawla et al. (99) studied the correlation of proton MRS and 31P MRS in HNSCC, and suggested that these two MRS techniques may be complementary to each other for the evaluation and prediction of HNSCC treatment response.
It is technically challenging to apply MRS to head and neck applications. The shimming performance could be greatly compromised by the pronounced susceptibility in some head and neck regions. The complex anatomy, air-tissue interface and various motions in the head and neck could also induce many artifacts that reduce MRS data quality thus affect analysis. The analysis and interpretation of MRS data is so different from imaging data that particular technical expertise is needed beyond the normal radiological reading of imaging data. In addition, 31P MRS imposes extra hardware requirements on wide-band excitation and extra receives RF coils equipped with MRI scanner for data acquisition. Meanwhile, the intrinsic low concentration of phosphorus compounds in the tissues leads to the low SNR of 31P MRS data, also hampering its broad use in routine clinical practice.
Blood oxygen level dependent (BOLD) MRI
BOLD-MRI is a popular non-invasive functional imaging method to investigate neural function and connectivity by investigating the hemodynamic response to brain activation. BOLD-MRI detects the MR signal attenuation in tissue adjacent to blood vessels due to the paramagnetic deoxyhemoglobin induced shortening of apparent spin-spin relaxation time T2* and thus the increasing of tissue susceptibility (100). In recent years, BOLD-MRI has been proposed to non-invasively image tumor hypoxia, in particular perfusion related acute hypoxia. In a patient group with heterogeneous cancer types including HNSCC, significant T2*-weighted signal enhancement was seen in 56% patients during carbogen breathing, indicating the improved tissue oxygenation and blood flow. Thus BOLD-MRI was suggested to potentially identify those patients who could benefit from carbogen radiosensitization (101). Rijpkenma et al. (102) assessed the blood oxygenation and vascular effects of breathing a hyperoxic hypercapnic gas mixture using both DCE-MRI and BOLD-MRI. The results showed that BOLD-MRI imaging revealed a significant T2* increase in HNSCC tumors during hypercapnic hyperoxygenation, which correlated to a decrease of the deoxyhemoglobin concentration, thus potentially beneficial for Accelerated Radiotherapy with CarbOgen and Nicotinamide (ACRON). In another study, Kotas et al. (103) successfully measured the heterogeneous oxygenation changes in head-and-neck tumor patients by using BOLD-MRI and concluded that BOLD-MRI was feasible for this purpose in clinical use.
Despite the great advantage of no need of exogenous radioactive tracer administration, BOLD-MRI has some major limitations for hypoxia imaging. First, it does not directly measure the pO2 in tumor and can only serve as a surrogate indicator of tumor hypoxia. Further decoupling of blood flow volume and deoxyhaemoglobin is needed. The sensitivity of BOLD-MRI to diffusion-related chronic hypoxia is still unknown. In practice, intrinsic pronounced susceptibility in the head and neck makes BOLD-MRI more difficult. Meanwhile, BOLD-MRI usually has low signal-to-noise ratio so should be further improved. The possible high failure rate of BOLD-MRI due to respiratory distress may also be concerned (101).
Other functional MRI techniques unexplored for RT
Beyond the functional MRI techniques introduced above, there are still a number of MRI techniques that have been proposed and introduced for diagnostic radiology, while not yet been explored for head and neck RT. Here we just briefly introduce the basic principle of these techniques and their relevance to head and neck RT. Clinical indication and evidence of these techniques for head and neck RT is yet to be established.
MR elastography (MRE)
MRE is a non-invasive quantitative imaging method that allows the measurements of biomechanical properties of living tissues such as elasticity and viscosity by utilizing the phase information of complex MR images under harmonic mechanical excitation of tissue by an external driver (104). MRE could be understood as a “palpation imaging” method extended from the traditional palpation without restrictions by the tumor location that is not accessible to the physician’s hand (105). Encouraging results of MRE have been reported for breast cancer and liver fibrosis (106,107). For HNC, MRE might provide an alternative or complementary option for tumor differentiation and treatment response evaluation. For instance, fibrosis is a common late complication of radiotherapy for HNCs and is difficult to quantify, MRE might be used for this purpose (108). A customized external driver suitable for MRE of the head and neck has been proposed and preliminarily tested on six healthy volunteers and three patients with HNC (109). It is recognized that the propagation of acoustic strain waves in the head and neck can be complicated by the highly heterogeneous and complex anatomies in this region, so MRE phase information might be corrupted. Consequently, the technical challenge is yet to be overcome first before its clinical use in the head and neck.
Spin-lock and chemical exchange saturation transfer (CEST) MRI
Most MRI techniques explore and visualize the abundant free water pool in living tissues to generate MR image contrast. Proton exchange between free water and water bound to macromolecules or labile protons contained in macromolecules represents an important physiological process in living tissues that can also be utilized to generate MRI contrast. Spin-lock imaging (or spin-lattice relaxation in the rotating frame T1ρ imaging) and CEST MRI are two MRI techniques to investigate chemical exchange in tissues.
In spin-lock MRI, an external RF pulse, called spin-lock pulse, is applied on- or off-resonance in the transverse plane to slow down the tissue T2 relaxation procedure in the transverse plane. In theory, Bloch-McConnell equations (110) can be used to quantitatively describe the time evolution of the magnetization of a multi-pool model under proton exchange. In practice, by applying the spin-lock pulse with different pulse durations (called spin-lock time) and measuring the corresponding signal intensities, T1ρ, the spin-lattice relaxation in the rotating frame, can be quantified by fitting the signal decay with regard to spin-lock time to a mono-exponential decay function. Some preliminary results of spin-lock imaging have been reported for diagnosis of HNC (111,112). Spin-lock MRI suffers from banding artifact due to the inhomogeneous B0 and B1 fields (113-115), so can be technically challenging when applied to head and neck due to the pronounced tissue susceptibility. Meanwhile, the relatively strong spin-lock pulses may be concerned in terms of specific absorption rate (SAR) (116). Reports on spin-lock imaging for RT applications for HNCs are still awaited.
CEST has recently been emerging as a promising molecular and cellular MRI method by exploring the in vivo chemical exchange processes between free water and mobile exchangeable exogenous or endogenous agents (117). Although sharing the similar theoretical foundation as spin-lock MRI, CEST MRI has the advantage of higher sensitivity and specificity to particular macromolecules, such as glucose, glutamate, amide, glycogen and glycosaminoglycans (118). In CEST MRI, different irradiation frequencies of a longer but weaker saturation RF pulse than spin-lock pulse for spin-lock imaging are applied to obtain the so-called Z-spectrum, the signal intensity ratios compared to the unsaturated signal intensity as a function of offset frequency from water. Asymmetric magnetization transfer ratio (MTRasym), the subtraction of the MTR at the opposite offset frequency of labile proton pool and that at the offset frequency, and other parameters can be derived from Z-spectrum analysis (119). Some animal studies have suggested the potentials of CEST MRI for RT applications, for example, the differentiation of brain tumor reoccurrence from radiation induced necrosis (120), and in vivo imaging of glucose uptake and metabolism in tumors (121). In head and neck, Yuan et al. (122) preliminarily explored the feasibility and repeatability of amide proton transfer-weighted (APTw) MRI at 3 T. Head and neck tumors showed positive mean APTw ranging from 1.2% to 3.2%, higher than surrounding normal tissues. So far there has been no study that reports the use of CEST MRI for head and neck RT.
Discussion
In this era of personalized and precision medicine, conformal, tailored, and adaptive IMRT is becoming an important trend in the future development of RT for HNC to maximize treatment outcome and minimize toxicity (2). To this end, strong support from clinical imaging is indispensable. Owing to the superior soft tissue contrasts, versatility and non-ionizing radiation nature, MRI is expected to fulfill many underserved needs in radiation oncology and is evolving from a purely diagnostic to a comprehensive diagnostic-therapeutic imaging tool. Dedicated MRI simulators for radiotherapy have been introduced into market and are gaining increasingly use in radiation oncology in recently years. Dedicated MRI simulators considerably overcome some fundamental barriers like patient positioning consistency and reproducibility for the use of MRI in RT, and hopefully largely reduce the uncertainty of MR image co-registration to simulation CT and on-board imaging. More advanced integrated MR-LINAC systems have also been proposed and introduced into clinics to guide the delivery of radiation beams in real time during treatment (123).
In this article, a variety of functional MRI techniques are briefly introduced and reviewed on their basic principle and current status in head and neck radiation oncology. Table 1 briefly summarizes the characteristics of these techniques and possible pathophysiological correlates in head and neck radiotherapy applications. Due to the length limitation, the detail of each technique and in-depth analysis cannot be fully covered in this single paper. The readers still need to refer to references for details.
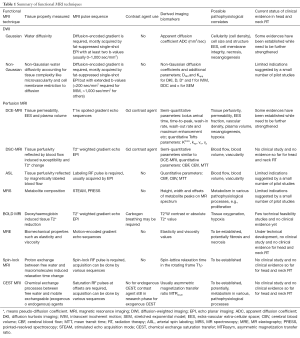
Full table
Compared to anatomical MRI, many functional MRI techniques such as DWI, ASL, and BOLD MRI, are currently heavily reliant on the EPI pulse sequence, which is associated with technical problems of low spatial resolution, severe distortion and various image artifacts. These problems become more severe in the head and neck region due to the complex anatomies, various motions as well as pronounced tissue susceptibility. This may introduce extra uncertainties in simulation, treatment planning and delivery guidance, significantly offsetting the potential advantages of functional MRI over anatomical imaging.
Despite the great advancements of MRI hardware, fast pulse sequences and novel reconstructions to make MRI much faster, scan time remains one major restriction to the wider use of functional MRI in RT. Scan time has to be minimized for patient comfort, while needs to be reasonably long in order to acquire both morphological and functional information from a potentially large volume of the head and neck that covers the primary tumor site and metastatic nodal sites. Since anatomical MRI is currently still indispensable for RT applications, the time slot in the scanning session left for functional MRI has to be well planned and determined depending on specific applications and tasks. Radiobiology and the influence of radiotherapy on tissue functions at different timelines have to be well understood to determine the most appropriate functional MRI techniques. Cost-effectiveness is also an important factor to select the appropriate functional MRI techniques.
Although MRI is versatile in providing a variety of options to investigate tissue function without ionizing radiation, it is worth noting that these functional MRI techniques usually provide surrogate biomarkers of tissue function but lack of sufficient specificity. For example, DCE-MRI can be comprehensively affected by various tissue properties of tissue relaxation time, permeability, vascularity, hematocrit and hypoxia and the individual contribution by these factors can be hard to be further differentiated. BOLD-MRI does not directly measure the pO2 in tissue to reflect hypoxia but rather detects the T2*-weighted MR signal variation due to deoxyhemoglobin, and can also be influenced by blood flow volume. Therefore, functional MRI data should be carefully interpreted. Meanwhile, CNR of some functional MRI techniques such as BOLD-MRI is relatively low, which may also affect their sensitivity to investigate tissue functionality.
To ensure reproducible and reliable performance of functional MRI techniques, it is vital to develop and establish protocols of quality assurance (QA) test for functional MRI techniques. Furthermore, to facilitate cross-center clinical trials in radiation oncology, it is necessary and important to standardize functional MRI protocols like scan parameter prescription and contrast agent administration, as well as post-processing and analysis method. In addition, the time ordering of functional MRI modules should be carefully considered if more than one functional MRI technique is applied. For example, Gd contrast agent administration might affect ADC quantification. Furthermore, cost-effectiveness performance of functional MRI should be carefully evaluated. As such, the timelines of MRI scans needs to be determined and optimized during the whole course of RT treatment for different purposes of pre-treatment prediction, intra- or inter-fraction monitoring, treatment adaptation and post-treatment evaluation.
Although we focus on functional MRI techniques in this article, it should be recognized that functional imaging can be conducted on multiple clinical imaging modalities beyond MRI. PET/CT is also playing an important role in radiation oncology by providing both anatomical and biological imaging information (124,125). Compared to MRI, PET still has the great advantage of higher sensitivity and specificity in spite of ionizing radiation and intrinsic low spatial resolution. Various PET tracers have been developed for functional or biological imaging at the cellular and molecular level to investigate tumor hypoxia, tumor cell proliferation, cell apoptosis, amino-acid transport as well as protein and cell membrane synthesis in the HNC (126,127). The recent introduction of simultaneous PET-MRI scanner in clinics offers an ideal platform to combine the strength and alleviate the weakness of each other, hopefully having greater impact on RT of HNC in the future (128).
Notwithstanding the encouraging preliminary results, functional MRI for RT in the head and neck is still a fertile area of technical and clinical research to be further explored that has yet to see its potential fulfilled. Till now, most of the reported studies are still primarily driven by technical rationale rather than clinical evidence. Greater efforts have to be made to establish credible clinical evidences in future studies to integrate functional MRI in the routine practice of head and neck radiation oncology (129). There is a bright future ahead but still a long way to go.
Acknowledgements
None.
Footnote
Conflicts of Interest: The authors have no conflicts of interest to declare.
References
- Jemal A, Bray F, Center MM, Ferlay J, Ward E, Forman D. Global cancer statistics. CA Cancer J Clin 2011;61:69-90. [Crossref] [PubMed]
- Grégoire V, Jeraj R, Lee JA, O'Sullivan B. Radiotherapy for head and neck tumours in 2012 and beyond: conformal, tailored, and adaptive? Lancet Oncol 2012;13:e292-300. [Crossref] [PubMed]
- Jaffray DA. Image-guided radiotherapy: from current concept to future perspectives. Nat Rev Clin Oncol 2012;9:688-99. [Crossref] [PubMed]
- Ling CC, Humm J, Larson S, Amols H, Fuks Z, Leibel S, Koutcher JA. Towards multidimensional radiotherapy (MD-CRT): biological imaging and biological conformality. Int J Radiat Oncol Biol Phys 2000;47:551-60. [Crossref] [PubMed]
- Rasch C, Steenbakkers R, van Herk M. Target definition in prostate, head, and neck. Semin Radiat Oncol 2005;15:136-45. [Crossref] [PubMed]
- Njeh CF. Tumor delineation: The weakest link in the search for accuracy in radiotherapy. J Med Phys 2008;33:136-40. [Crossref] [PubMed]
- Rasch C, Keus R, Pameijer FA, Koops W, de Ru V, Muller S, Touw A, Bartelink H, van Herk M, Lebesque JV. The potential impact of CT-MRI matching on tumor volume delineation in advanced head and neck cancer. Int J Radiat Oncol Biol Phys 1997;39:841-8. [Crossref] [PubMed]
- Emami B, Sethi A, Petruzzelli GJ. Influence of MRI on target volume delineation and IMRT planning in nasopharyngeal carcinoma. Int J Radiat Oncol Biol Phys 2003;57:481-8. [Crossref] [PubMed]
- Daisne JF, Sibomana M, Bol A, Cosnard G, Lonneux M, Gregoire V. Evaluation of a multimodality image (CT, MRI and PET) coregistration procedure on phantom and head and neck cancer patients: accuracy, reproducibility and consistency. Radiother Oncol 2003;69:237-45. [Crossref] [PubMed]
- Geets X, Daisne JF, Arcangeli S, Coche E, De Poel M, Duprez T, Nardella G, Gregoire V. Inter-observer variability in the delineation of pharyngo-laryngeal tumor, parotid glands and cervical spinal cord: comparison between CT-scan and MRI. Radiother Oncol 2005;77:25-31. [Crossref] [PubMed]
- Chung NN, Ting LL, Hsu WC, Lui LT, Wang PM. Impact of magnetic resonance imaging versus CT on nasopharyngeal carcinoma: primary tumor target delineation for radiotherapy. Head Neck 2004;26:241-6. [Crossref] [PubMed]
- Thiagarajan A, Caria N, Schoder H, Iyer NG, Wolden S, Wong RJ, Sherman E, Fury MG, Lee N. Target volume delineation in oropharyngeal cancer: impact of PET, MRI, and physical examination. Int J Radiat Oncol Biol Phys 2012;83:220-7. [Crossref] [PubMed]
- Webster GJ, Kilgallon JE, Ho KF, Rowbottom CG, Slevin NJ, Mackay RI. A novel imaging technique for fusion of high-quality immobilised MR images of the head and neck with CT scans for radiotherapy target delineation. Br J Radiol 2009;82:497-503. [Crossref] [PubMed]
- Koh DM, Collins DJ. Diffusion-weighted MRI in the body: applications and challenges in oncology. AJR Am J Roentgenol 2007;188:1622-35. [Crossref] [PubMed]
- Stejskal EO, Tanner JE. Spin Diffusion Measurements: Spin Echoes in the Presence of a Time-Dependent Field Gradient. J Chem Phys 1965;42:288-92. [Crossref]
- Padhani AR, Liu G, Koh DM, Chenevert TL, Thoeny HC, Takahara T, Dzik-Jurasz A, Ross BD, Van Cauteren M, Collins D, Hammoud DA, Rustin GJ, Taouli B, Choyke PL. Diffusion-weighted magnetic resonance imaging as a cancer biomarker: consensus and recommendations. Neoplasia 2009;11:102-25. [Crossref] [PubMed]
- Bonekamp S, Corona-Villalobos CP, Kamel IR. Oncologic applications of diffusion-weighted MRI in the body. J Magn Reson Imaging 2012;35:257-79. [Crossref] [PubMed]
- Thoeny HC, De Keyzer F, King AD. Diffusion-weighted MR Imaging in the Head and Neck. Radiology 2012;263:19-32. [Crossref] [PubMed]
- Vandecaveye V, De Keyzer F, Dirix P, Lambrecht M, Nuyts S, Hermans R. Applications of diffusion-weighted magnetic resonance imaging in head and neck squamous cell carcinoma. Neuroradiology 2010;52:773-84. [Crossref] [PubMed]
- Driessen JP, van Kempen PM, van der Heijden GJ, Philippens ME, Pameijer FA, Stegeman I, Terhaard CH, Janssen LM, Grolman W. Diffusion-weighted imaging in head and neck squamous cell carcinomas: a systematic review. Head Neck 2015;37:440-8. [Crossref] [PubMed]
- Vandecaveye V, De Keyzer F, Vander Poorten V, Dirix P, Verbeken E, Nuyts S, Hermans R. Head and neck squamous cell carcinoma: value of diffusion-weighted MR imaging for nodal staging. Radiology 2009;251:134-46. [Crossref] [PubMed]
- Abdel Razek AA, Soliman NY, Elkhamary S, Alsharaway MK, Tawfik A. Role of diffusion-weighted MR imaging in cervical lymphadenopathy. Eur Radiol 2006;16:1468-77. [Crossref] [PubMed]
- de Bondt RB, Hoeberigs MC, Nelemans PJ, Deserno WM, Peutz-Kootstra C, Kremer B, Beets-Tan RG. Diagnostic accuracy and additional value of diffusion-weighted imaging for discrimination of malignant cervical lymph nodes in head and neck squamous cell carcinoma. Neuroradiology 2009;51:183-92. [Crossref] [PubMed]
- Holzapfel K, Duetsch S, Fauser C, Eiber M, Rummeny EJ, Gaa J. Value of diffusion-weighted MR imaging in the differentiation between benign and malignant cervical lymph nodes. Eur J Radiol 2009;72:381-7. [Crossref] [PubMed]
- Perrone A, Guerrisi P, Izzo L, D'Angeli I, Sassi S, Mele LL, Marini M, Mazza D, Marini M. Diffusion-weighted MRI in cervical lymph nodes: differentiation between benign and malignant lesions. Eur J Radiol 2011;77:281-6. [Crossref] [PubMed]
- Sumi M, Sakihama N, Sumi T, Morikawa M, Uetani M, Kabasawa H, Shigeno K, Hayashi K, Takahashi H, Nakamura T. Discrimination of metastatic cervical lymph nodes with diffusion-weighted MR imaging in patients with head and neck cancer. AJNR Am J Neuroradiol 2003;24:1627-34. [PubMed]
- Dirix P, Vandecaveye V, De Keyzer F, Op de Beeck K, Poorten VV, Delaere P, Verbeken E, Hermans R, Nuyts S. Diffusion-weighted MRI for nodal staging of head and neck squamous cell carcinoma: impact on radiotherapy planning. Int J Radiat Oncol Biol Phys 2010;76:761-6. [Crossref] [PubMed]
- Kim S, Loevner L, Quon H, Sherman E, Weinstein G, Kilger A, Poptani H. Diffusion-weighted magnetic resonance imaging for predicting and detecting early response to chemoradiation therapy of squamous cell carcinomas of the head and neck. Clin Cancer Res 2009;15:986-94. [Crossref] [PubMed]
- Hatakenaka M, Shioyama Y, Nakamura K, Yabuuchi H, Matsuo Y, Sunami S, Kamitani T, Yoshiura T, Nakashima T, Nishikawa K, Honda H. Apparent Diffusion Coefficient Calculated with Relatively High b-Values Correlates with Local Failure of Head and Neck Squamous Cell Carcinoma Treated with Radiotherapy. AJNR Am J Neuroradiol 2011;32:1904-10. [Crossref] [PubMed]
- Hatakenaka M, Nakamura K, Yabuuchi H, Shioyama Y, Matsuo Y, Ohnishi K, Sunami S, Kamitani T, Setoguchi T, Yoshiura T, Nakashima T, Nishikawa K, Honda H. Pretreatment apparent diffusion coefficient of the primary lesion correlates with local failure in head-and-neck cancer treated with chemoradiotherapy or radiotherapy. Int J Radiat Oncol Biol Phys 2011;81:339-45. [Crossref] [PubMed]
- Galbán CJ, Mukherji SK, Chenevert TL, Meyer CR, Hamstra DA, Bland PH, Johnson TD, Moffat BA, Rehemtulla A, Eisbruch A, Ross BD. A feasibility study of parametric response map analysis of diffusion-weighted magnetic resonance imaging scans of head and neck cancer patients for providing early detection of therapeutic efficacy. Transl Oncol 2009;2:184-90. [Crossref] [PubMed]
- Vandecaveye V, Dirix P, De Keyzer F, de Beeck KO, Vander Poorten V, Roebben I, Nuyts S, Hermans R. Predictive value of diffusion-weighted magnetic resonance imaging during chemoradiotherapy for head and neck squamous cell carcinoma. Eur Radiol 2010;20:1703-14. [Crossref] [PubMed]
- Berrak S, Chawla S, Kim S, Quon H, Sherman E, Loevner LA, Poptani H. Diffusion weighted imaging in predicting progression free survival in patients with squamous cell carcinomas of the head and neck treated with induction chemotherapy. Acad Radiol 2011;18:1225-32. [Crossref] [PubMed]
- Vandecaveye V, Dirix P, De Keyzer F, Op de Beeck K, Vander Poorten V, Hauben E, Lambrecht M, Nuyts S, Hermans R. Diffusion-weighted magnetic resonance imaging early after chemoradiotherapy to monitor treatment response in head-and-neck squamous cell carcinoma. Int J Radiat Oncol Biol Phys 2012;82:1098-107. [Crossref] [PubMed]
- King AD, Chow KK, Yu KH, Mo FK, Yeung DK, Yuan J, Bhatia KS, Vlantis AC, Ahuja AT. Head and Neck Squamous Cell Carcinoma: Diagnostic Performance of Diffusion-weighted MR Imaging for the Prediction of Treatment Response. Radiology 2013;266:531-8. [Crossref] [PubMed]
- Matoba M, Tuji H, Shimode Y, Toyoda I, Kuginuki Y, Miwa K, Tonami H. Fractional change in apparent diffusion coefficient as an imaging biomarker for predicting treatment response in head and neck cancer treated with chemoradiotherapy. AJNR Am J Neuroradiol 2014;35:379-85. [Crossref] [PubMed]
- Zbären P, Caversaccio M, Thoeny HC, Nuyens M, Curschmann J, Stauffer E. Radionecrosis or tumor recurrence after radiation of laryngeal and hypopharyngeal carcinomas. Otolaryngol Head Neck Surg 2006;135:838-43. [Crossref] [PubMed]
- King AD, Mo FK, Yu KH, Yeung DK, Zhou H, Bhatia KS, Tse GM, Vlantis AC, Wong JK, Ahuja AT. Squamous cell carcinoma of the head and neck: diffusion-weighted MR imaging for prediction and monitoring of treatment response. Eur Radiol 2010;20:2213-20. [Crossref] [PubMed]
- Abdel Razek AA, Kandeel AY, Soliman N, El-shenshawy HM, Kamel Y, Nada N, Denewar A. Role of diffusion-weighted echo-planar MR imaging in differentiation of residual or recurrent head and neck tumors and posttreatment changes. AJNR Am J Neuroradiol 2007;28:1146-52. [Crossref] [PubMed]
- Vandecaveye V, De Keyzer F, Nuyts S, Deraedt K, Dirix P, Hamaekers P, Vander Poorten V, Delaere P, Hermans R. Detection of head and neck squamous cell carcinoma with diffusion weighted MRI after (chemo)radiotherapy: correlation between radiologic and histopathologic findings. Int J Radiat Oncol Biol Phys 2007;67:960-71. [Crossref] [PubMed]
- de Bree R, van der Putten L, Brouwer J, Castelijns JA, Hoekstra OS, Leemans CR. Detection of locoregional recurrent head and neck cancer after (chemo)radiotherapy using modern imaging. Oral Oncol 2009;45:386-93. [Crossref] [PubMed]
- Ryan WR, Fee WE Jr, Le QT, Pinto HA. Positron-emission tomography for surveillance of head and neck cancer. Laryngoscope 2005;115:645-50. [Crossref] [PubMed]
- Abgral R, Querellou S, Potard G, Le Roux PY, Le Duc-Pennec A, Marianovski R, Pradier O, Bizais Y, Kraeber-Bodere F, Salaun PY. Does 18F-FDG PET/CT improve the detection of posttreatment recurrence of head and neck squamous cell carcinoma in patients negative for disease on clinical follow-up? J Nucl Med 2009;50:24-9. [Crossref] [PubMed]
- Yablonskiy DA, Sukstanskii AL. Theoretical models of the diffusion weighted MR signal. NMR Biomed 2010;23:661-81. [Crossref] [PubMed]
- Magin RL, Abdullah O, Baleanu D, Zhou XJ. Anomalous diffusion expressed through fractional order differential operators in the Bloch-Torrey equation. J Magn Reson 2008;190:255-70. [Crossref] [PubMed]
- Hall MG, Barrick TR. From diffusion-weighted MRI to anomalous diffusion imaging. Magn Reson Med 2008;59:447-55. [Crossref] [PubMed]
- Jensen JH, Helpern JA, Ramani A, Lu H, Kaczynski K. Diffusional kurtosis imaging: the quantification of non-gaussian water diffusion by means of magnetic resonance imaging. Magn Reson Med 2005;53:1432-40. [Crossref] [PubMed]
- Yablonskiy DA, Bretthorst GL, Ackerman JJ. Statistical model for diffusion attenuated MR signal. Magn Reson Med 2003;50:664-9. [Crossref] [PubMed]
- Bennett KM, Schmainda KM, Bennett RT, Rowe DB, Lu H, Hyde JS. Characterization of continuously distributed cortical water diffusion rates with a stretched-exponential model. Magn Reson Med 2003;50:727-34. [Crossref] [PubMed]
- Le Bihan D, Breton E, Lallemand D, Aubin ML, Vignaud J, Laval-Jeantet M. Separation of diffusion and perfusion in intravoxel incoherent motion MR imaging. Radiology 1988;168:497-505. [Crossref] [PubMed]
- Lu Y, Jansen JF, Stambuk HE, Gupta G, Lee N, Gonen M, Moreira A, Mazaheri Y, Patel SG, Deasy JO, Shah JP, Shukla-Dave A. Comparing primary tumors and metastatic nodes in head and neck cancer using intravoxel incoherent motion imaging: a preliminary experience. J Comput Assist Tomogr 2013;37:346-52. [Crossref] [PubMed]
- Lu Y, Jansen JF, Mazaheri Y, Stambuk HE, Koutcher JA, Shukla-Dave A. Extension of the intravoxel incoherent motion model to non-gaussian diffusion in head and neck cancer. J Magn Reson Imaging 2012;36:1088-96. [Crossref] [PubMed]
- Lai V, Li X, Lee VH, Lam KO, Chan Q, Khong PL. Intravoxel incoherent motion MR imaging: comparison of diffusion and perfusion characteristics between nasopharyngeal carcinoma and post-chemoradiation fibrosis. Eur Radiol 2013;23:2793-801. [Crossref] [PubMed]
- Lai V, Lee VH, Lam KO, Sze HC, Chan Q, Khong PL. Intravoxel water diffusion heterogeneity MR imaging of nasopharyngeal carcinoma using stretched exponential diffusion model. Eur Radiol 2015;25:1708-13. [Crossref] [PubMed]
- Jansen JF, Stambuk HE, Koutcher JA, Shukla-Dave A. Non-gaussian analysis of diffusion-weighted MR imaging in head and neck squamous cell carcinoma: A feasibility study. AJNR Am J Neuroradiol 2010;31:741-8. [Crossref] [PubMed]
- Yuan J, Yeung DK, Mok GS, Bhatia KS, Wang YX, Ahuja AT, King AD. Non-Gaussian Analysis of Diffusion Weighted Imaging in Head and Neck at 3T: A Pilot Study in Patients with Nasopharyngeal Carcinoma. PLoS One 2014;9:e87024. [Crossref] [PubMed]
- Chen Y, Ren W, Zheng D, Zhong J, Liu X, Yue Q, Liu M, Xiao Y, Chen W, Chan Q, Pan J. Diffusion kurtosis imaging predicts neoadjuvant chemotherapy responses within 4 days in advanced nasopharyngeal carcinoma patients. J Magn Reson Imaging 2015;42:1354-61. [Crossref] [PubMed]
- Porter DA, Heidemann RM. High resolution diffusion-weighted imaging using readout-segmented echo-planar imaging, parallel imaging and a two-dimensional navigator-based reacquisition. Magn Reson Med 2009;62:468-75. [Crossref] [PubMed]
- Pipe JG, Farthing VG, Forbes KP. Multishot diffusion-weighted FSE using PROPELLER MRI. Magn Reson Med 2002;47:42-52. [Crossref] [PubMed]
- Essig M, Shiroishi MS, Nguyen TB, Saake M, Provenzale JM, Enterline D, Anzalone N, Dorfler A, Rovira A, Wintermark M, Law M. Perfusion MRI: the five most frequently asked technical questions. AJR Am J Roentgenol 2013;200:24-34. [Crossref] [PubMed]
- Tofts PS, Kermode AG. Measurement of the blood-brain barrier permeability and leakage space using dynamic MR imaging. 1. Fundamental concepts. Magn Reson Med 1991;17:357-67. [Crossref] [PubMed]
- Kuhl CK, Mielcareck P, Klaschik S, Leutner C, Wardelmann E, Gieseke J, Schild HH. Dynamic breast MR imaging: are signal intensity time course data useful for differential diagnosis of enhancing lesions? Radiology 1999;211:101-10. [Crossref] [PubMed]
- Weinstein D, Strano S, Cohen P, Fields S, Gomori JM, Degani H. Breast fibroadenoma: mapping of pathophysiologic features with three-time-point, contrast-enhanced MR imaging--pilot study. Radiology 1999;210:233-40. [Crossref] [PubMed]
- Asaumi J, Yanagi Y, Hisatomi M, Matsuzaki H, Konouchi H, Kishi K. The value of dynamic contrast-enhanced MRI in diagnosis of malignant lymphoma of the head and neck. Eur J Radiol 2003;48:183-7. [Crossref] [PubMed]
- Sumi M, Nakamura T. Extranodal spread in the neck: MRI detection on the basis of pixel-based time-signal intensity curve analysis. J Magn Reson Imaging 2011;33:830-8. [Crossref] [PubMed]
- Yuan J, Chow SK, Yeung DK, King AD. A five-colour colour-coded mapping method for DCE-MRI analysis of head and neck tumours. Clin Radiol 2012;67:216-23. [Crossref] [PubMed]
- Hoskin PJ, Saunders MI, Goodchild K, Powell ME, Taylor NJ, Baddeley H. Dynamic contrast enhanced magnetic resonance scanning as a predictor of response to accelerated radiotherapy for advanced head and neck cancer. Br J Radiol 1999;72:1093-8. [Crossref] [PubMed]
- Tofts PS, Brix G, Buckley DL, Evelhoch JL, Henderson E, Knopp MV, Larsson HB, Lee TY, Mayr NA, Parker GJ, Port RE, Taylor J, Weisskoff RM. Estimating kinetic parameters from dynamic contrast-enhanced T(1)-weighted MRI of a diffusable tracer: standardized quantities and symbols. J Magn Reson Imaging 1999;10:223-32. [Crossref] [PubMed]
- Tofts PS. Modeling tracer kinetics in dynamic Gd-DTPA MR imaging. J Magn Reson Imaging 1997;7:91-101. [Crossref] [PubMed]
- Sourbron SP, Buckley DL. On the scope and interpretation of the Tofts models for DCE-MRI. Magn Reson Med 2011;66:735-45. [Crossref] [PubMed]
- Cao Y, Popovtzer A, Li D, Chepeha DB, Moyer JS, Prince ME, Worden F, Teknos T, Bradford C, Mukherji SK, Eisbruch A. Early prediction of outcome in advanced head-and-neck cancer based on tumor blood volume alterations during therapy: a prospective study. Int J Radiat Oncol Biol Phys 2008;72:1287-90. [Crossref] [PubMed]
- Shukla-Dave A, Lee NY, Jansen JF, Thaler HT, Stambuk HE, Fury MG, Patel SG, Moreira AL, Sherman E, Karimi S, Wang Y, Kraus D, Shah JP, Pfister DG, Koutcher JA. Dynamic contrast-enhanced magnetic resonance imaging as a predictor of outcome in head-and-neck squamous cell carcinoma patients with nodal metastases. Int J Radiat Oncol Biol Phys 2012;82:1837-44. [Crossref] [PubMed]
- Kim S, Loevner LA, Quon H, Kilger A, Sherman E, Weinstein G, Chalian A, Poptani H. Prediction of response to chemoradiation therapy in squamous cell carcinomas of the head and neck using dynamic contrast-enhanced MR imaging. AJNR Am J Neuroradiol 2010;31:262-8. [Crossref] [PubMed]
- Chawla S, Kim S, Loevner LA, Hwang WT, Weinstein G, Chalian A, Quon H, Poptani H. Prediction of disease-free survival in patients with squamous cell carcinomas of the head and neck using dynamic contrast-enhanced MR imaging. AJNR Am J Neuroradiol 2011;32:778-84. [Crossref] [PubMed]
- Chikui T, Kitamoto E, Kawano S, Sugiura T, Obara M, Simonetti AW, Hatakenaka M, Matsuo Y, Koga S, Ohga M, Nakamura K, Yoshiura K. Pharmacokinetic analysis based on dynamic contrast-enhanced MRI for evaluating tumor response to preoperative therapy for oral cancer. J Magn Reson Imaging 2012;36:589-597. [Crossref] [PubMed]
- Ng SH, Lin CY, Chan SC, Yen TC, Liao CT, Chang JT, Ko SF, Wang HM, Huang SF, Lin YC, Wang JJ. Dynamic contrast-enhanced MR imaging predicts local control in oropharyngeal or hypopharyngeal squamous cell carcinoma treated with chemoradiotherapy. PLoS One 2013;8:e72230. [Crossref] [PubMed]
- Janssen HL, Haustermans KM, Balm AJ, Begg AC. Hypoxia in head and neck cancer: How much, how important? Head Neck 2005;27:622-38. [Crossref] [PubMed]
- Newbold K, Castellano I, Charles-Edwards E, Mears D, Sohaib A, Leach M, Rhys-Evans P, Clarke P, Fisher C, Harrington K, Nutting C. An exploratory study into the role of dynamic contrast-enhanced magnetic resonance imaging or perfusion computed tomography for detection of intratumoral hypoxia in head-and-neck cancer. Int J Radiat Oncol Biol Phys 2009;74:29-37. [Crossref] [PubMed]
- Donaldson SB, Betts G, Bonington SC, Homer JJ, Slevin NJ, Kershaw LE, Valentine H, West CM, Buckley DL. Perfusion estimated with rapid dynamic contrast-enhanced magnetic resonance imaging correlates inversely with vascular endothelial growth factor expression and pimonidazole staining in head-and-neck cancer: a pilot study. Int J Radiat Oncol Biol Phys 2011;81:1176-83. [Crossref] [PubMed]
- Jansen JF, Carlson DL, Lu Y, Stambuk HE, Moreira AL, Singh B, Patel SG, Kraus DH, Wong RJ, Shaha AR, Shah JP, Shukla-Dave A. Correlation of a priori DCE-MRI and (1)H-MRS data with molecular markers in neck nodal metastases: Initial analysis. Oral Oncol 2012;48:717-22. [Crossref] [PubMed]
- Østergaard L. Principles of cerebral perfusion imaging by bolus tracking. J Magn Reson Imaging 2005;22:710-7. [Crossref] [PubMed]
- Razek AA, Elsorogy LG, Soliman NY, Nada N. Dynamic susceptibility contrast perfusion MR imaging in distinguishing malignant from benign head and neck tumors: a pilot study. Eur J Radiol 2011;77:73-9. [Crossref] [PubMed]
- Detre JA, Zhang W, Roberts DA, Silva AC, Williams DS, Grandis DJ, Koretsky AP, Leigh JS. Tissue specific perfusion imaging using arterial spin labeling. NMR Biomed 1994;7:75-82. [Crossref] [PubMed]
- Buxton RB, Frank LR, Wong EC, Siewert B, Warach S, Edelman RR. A general kinetic model for quantitative perfusion imaging with arterial spin labeling. Magn Reson Med 1998;40:383-96. [Crossref] [PubMed]
- Fujima N, Kudo K, Yoshida D, Homma A, Sakashita T, Tsukahara A, Khin Khin T, Yuri Z, Satoshi T, Hiroki S. Arterial spin labeling to determine tumor viability in head and neck cancer before and after treatment. J Magn Reson Imaging 2014;40:920-8. [Crossref] [PubMed]
- Fujima N, Kudo K, Tsukahara A, Yoshida D, Sakashita T, Homma A, Tha KK, Shirato H. Measurement of tumor blood flow in head and neck squamous cell carcinoma by pseudo-continuous arterial spin labeling: comparison with dynamic contrast-enhanced MRI. J Magn Reson Imaging 2015;41:983-91. [Crossref]
- Castillo M, Kwock L, Mukherji SK. Clinical applications of proton MR spectroscopy. AJNR Am J Neuroradiol 1996;17:1-15. [PubMed]
- Kwock L, Smith JK, Castillo M, Ewend MG, Cush S, Hensing T, Varia M, Morris D, Bouldin TW. Clinical applications of proton MR spectroscopy in oncology. Technol Cancer Res Treat 2002;1:17-28. [Crossref] [PubMed]
- Mukherji SK, Schiro S, Castillo M, Kwock L, Muller KE, Blackstock W. Proton MR spectroscopy of squamous cell carcinoma of the extracranial head and neck: in vitro and in vivo studies. AJNR Am J Neuroradiol 1997;18:1057-72. [PubMed]
- Jansen JF, Schoder H, Lee NY, Stambuk HE, Wang Y, Fury MG, Patel SG, Pfister DG, Shah JP, Koutcher JA, Shukla-Dave A. Tumor metabolism and perfusion in head and neck squamous cell carcinoma: pretreatment multimodality imaging with 1H magnetic resonance spectroscopy, dynamic contrast-enhanced MRI, and [18F]FDG-PET. Int J Radiat Oncol Biol Phys 2012;82:299-307. [Crossref] [PubMed]
- Bisdas S, Baghi M, Huebner F, Mueller C, Knecht R, Vorbuchner M, Ruff J, Gstoettner W, Vogl TJ. In vivo proton MR spectroscopy of primary tumours, nodal and recurrent disease of the extracranial head and neck. Eur Radiol 2007;17:251-7. [Crossref] [PubMed]
- Shah GV, Gandhi D, Mukherji SK. Magnetic resonance spectroscopy of head and neck neoplasms. Top Magn Reson Imaging 2004;15:87-94. [Crossref] [PubMed]
- Abdel Razek AA, Poptani H. MR spectrsocopy of head and neck cancer. Eur J Radiol 2013;82:982-9. [Crossref] [PubMed]
- Bezabeh T, Odlum O, Nason R, Kerr P, Sutherland D, Patel R, Smith IC. Prediction of treatment response in head and neck cancer by magnetic resonance spectroscopy. AJNR Am J Neuroradiol 2005;26:2108-13. [PubMed]
- King AD, Yeung DK, Yu KH, Mo FK, Bhatia KS, Tse GM, Vlantis AC, Wong JK, Hu CW, Ahuja AT. Pretreatment and early intratreatment prediction of clinicopathologic response of head and neck cancer to chemoradiotherapy using 1H-MRS. J Magn Reson Imaging 2010;32:199-203. [Crossref] [PubMed]
- King AD, Yeung DK, Yu KH, Mo FK, Hu CW, Bhatia KS, Tse GM, Vlantis AC, Wong JK, Ahuja AT. Monitoring of treatment response after chemoradiotherapy for head and neck cancer using in vivo 1H MR spectroscopy. Eur Radiol 2010;20:165-72. [Crossref] [PubMed]
- Yeung DK, Fong KY, Chan QC, King AD. Chemical shift imaging in the head and neck at 3T: Initial results. J Magn Reson Imaging 2010;32:1248-54. [Crossref] [PubMed]
- Maldonado X, Alonso J, Giralt J, Cucurella MG, del Campo JM, Rovira A, Felip E, Capellades J, Grive E, Rubio D, Gili J. 31Phosphorus magnetic resonance spectroscopy in the assessment of head and neck tumors. Int J Radiat Oncol Biol Phys 1998;40:309-12. [Crossref] [PubMed]
- Chawla S, Kim S, Loevner LA, Quon H, Wang S, Mutale F, Weinstein G, Delikatny EJ, Poptani H. Proton and phosphorous MR spectroscopy in squamous cell carcinomas of the head and neck. Acad Radiol 2009;16:1366-72. [Crossref] [PubMed]
- Ogawa S, Lee TM, Kay AR, Tank DW. Brain magnetic resonance imaging with contrast dependent on blood oxygenation. Proc Natl Acad Sci U S A 1990;87:9868-72. [Crossref] [PubMed]
- Taylor NJ, Baddeley H, Goodchild KA, Powell ME, Thoumine M, Culver LA, Stirling JJ, Saunders MI, Hoskin PJ, Phillips H, Padhani AR, Griffiths JR. BOLD MRI of human tumor oxygenation during carbogen breathing. J Magn Reson Imaging 2001;14:156-63. [Crossref] [PubMed]
- Rijpkema M, Kaanders JH, Joosten FB, van der Kogel AJ, Heerschap A. Effects of breathing a hyperoxic hypercapnic gas mixture on blood oxygenation and vascularity of head-and-neck tumors as measured by magnetic resonance imaging. Int J Radiat Oncol Biol Phys 2002;53:1185-91. [Crossref] [PubMed]
- Kotas M, Schmitt P, Jakob PM, Flentje M. Monitoring of tumor oxygenation changes in head-and-neck carcinoma patients breathing a hyperoxic hypercapnic gas mixture with a noninvasive MRI technique. Strahlenther Onkol 2009;185:19-26. [Crossref] [PubMed]
- Muthupillai R, Lomas DJ, Rossman PJ, Greenleaf JF, Manduca A, Ehman RL. Magnetic resonance elastography by direct visualization of propagating acoustic strain waves. Science 1995;269:1854-7. [Crossref] [PubMed]
- Manduca A, Oliphant TE, Dresner MA, Mahowald JL, Kruse SA, Amromin E, Felmlee JP, Greenleaf JF, Ehman RL. Magnetic resonance elastography: non-invasive mapping of tissue elasticity. Med Image Anal 2001;5:237-54. [Crossref] [PubMed]
- Yin M, Talwalkar JA, Glaser KJ, Manduca A, Grimm RC, Rossman PJ, Fidler JL, Ehman RL. Assessment of hepatic fibrosis with magnetic resonance elastography. Clin Gastroenterol Hepatol 2007;5:1207-1213.e2. [Crossref] [PubMed]
- Plewes DB, Bishop J, Samani A, Sciarretta J. Visualization and quantification of breast cancer biomechanical properties with magnetic resonance elastography. Phys Med Biol 2000;45:1591-610. [Crossref] [PubMed]
- Moloney EC, Brunner M, Alexander AJ, Clark J. Quantifying fibrosis in head and neck cancer treatment: An overview. Head Neck 2015;37:1225-31. [Crossref] [PubMed]
- Yeung DK, Bhatia KS, Lee YY, King AD, Garteiser P, Sinkus R, Ahuja AT. MR elastography of the head and neck: driver design and initial results. Magn Reson Imaging 2013;31:624-9. [Crossref] [PubMed]
- McConnell HM. Reaction rates by nuclear magnetic resonance. J Chem Phys 1958;28:430-1. [Crossref]
- Markkola AT, Aronen HJ, Lukkarinen S, Ramadan UA, Tanttu JI, Sepponen RE. Multiple-slice spin lock imaging of head and neck tumors at 0.1 Tesla: exploring appropriate imaging parameters with reference to T2-weighted spin-echo technique. Invest Radiol 2001;36:531-8. [Crossref] [PubMed]
- Markkola AT, Aronen HJ, Ramadan UA, Halavaara JT, Tanttu JI, Sepponen RE. Determination of T1rho values for head and neck tissues at 0.1 T: a comparison to T1 and T2 relaxation times. Magn Reson Imaging 1998;16:377-83. [Crossref] [PubMed]
- Charagundla SR, Borthakur A, Leigh JS, Reddy R. Artifacts in T(1rho)-weighted imaging: correction with a self-compensating spin-locking pulse. J Magn Reson 2003;162:113-21. [Crossref] [PubMed]
- Chen W, Takahashi A, Han E. Quantitative T(1)(rho) imaging using phase cycling for B(0) and B(1) field inhomogeneity compensation. Magn Reson Imaging 2011;29:608-19. [Crossref] [PubMed]
- Yuan J, Li Y, Zhao F, Chan Q, Ahuja AT, Wang YX. Quantification of T1rho relaxation by using rotary echo spin-lock pulses in the presence of B0 inhomogeneity. Phys Med Biol 2012;57:5003-16. [Crossref] [PubMed]
- Wheaton AJ, Borthakur A, Corbo M, Charagundla SR, Reddy R. Method for reduced SAR T1rho-weighted MRI. Magn Reson Med 2004;51:1096-102. [Crossref] [PubMed]
- Ward KM, Aletras AH, Balaban RS. A new class of contrast agents for MRI based on proton chemical exchange dependent saturation transfer (CEST). J Magn Reson 2000;143:79-87. [Crossref] [PubMed]
- van Zijl PC, Yadav NN. Chemical exchange saturation transfer (CEST): what is in a name and what isn't? Magn Reson Med 2011;65:927-48. [Crossref] [PubMed]
- Liu G, Song X, Chan KW, McMahon MT. Nuts and bolts of chemical exchange saturation transfer MRI. NMR Biomed 2013;26:810-28. [Crossref] [PubMed]
- Zhou J, Tryggestad E, Wen Z, Lal B, Zhou T, Grossman R, Wang S, Yan K, Fu DX, Ford E, Tyler B, Blakeley J, Laterra J, van Zijl PC. Differentiation between glioma and radiation necrosis using molecular magnetic resonance imaging of endogenous proteins and peptides. Nat Med 2011;17:130-4. [Crossref] [PubMed]
- Walker-Samuel S, Ramasawmy R, Torrealdea F, Rega M, Rajkumar V, Johnson SP, Richardson S, Goncalves M, Parkes HG, Arstad E, Thomas DL, Pedley RB, Lythgoe MF, Golay X. In vivo imaging of glucose uptake and metabolism in tumors. Nat Med 2013;19:1067-72. [Crossref] [PubMed]
- Yuan J, Chen S, King AD, Zhou J, Bhatia KS, Zhang Q, Yeung DK, Wei J, Mok GS, Wang YX. Amide proton transfer-weighted imaging of the head and neck at 3 T: a feasibility study on healthy human subjects and patients with head and neck cancer. NMR Biomed 2014;27:1239-47. [Crossref] [PubMed]
- Lagendijk JJ, Raaymakers BW, Raaijmakers AJ, Overweg J, Brown KJ, Kerkhof EM, van der Put RW, Hardemark B, van Vulpen M, van der Heide UA. MRI/linac integration. Radiother Oncol 2008;86:25-9. [Crossref] [PubMed]
- MacManus M, Nestle U, Rosenzweig KE, Carrio I, Messa C, Belohlavek O, Danna M, Inoue T, Deniaud-Alexandre E, Schipani S, Watanabe N, Dondi M, Jeremic B. Use of PET and PET/CT for radiation therapy planning: IAEA expert report 2006-2007. Radiother Oncol 2009;91:85-94. [Crossref] [PubMed]
- Zaidi H, Vees H, Wissmeyer M. Molecular PET/CT imaging-guided radiation therapy treatment planning. Acad Radiol 2009;16:1108-33. [Crossref] [PubMed]
- Bhatnagar P, Subesinghe M, Patel C, Prestwich R, Scarsbrook AF. Functional imaging for radiation treatment planning, response assessment, and adaptive therapy in head and neck cancer. Radiographics 2013;33:1909-29. [Crossref] [PubMed]
- de Bree R. Functional imaging to predict treatment response after (chemo) radiotherapy of head and neck squamous cell carcinoma. Quant Imaging Med Surg 2013;3:231-4. [PubMed]
- Pichler BJ, Kolb A, Nagele T, Schlemmer HP. PET/MRI: paving the way for the next generation of clinical multimodality imaging applications. J Nucl Med 2010;51:333-6. [Crossref] [PubMed]
- Glide-Hurst CK, Low DA, Orton CG. Point/Counterpoint. MRI/CT is the future of radiotherapy treatment planning. Med Phys 2014;41:110601. [Crossref] [PubMed]