Dual-layer spectral detector computed tomography parameters can improve diagnostic efficiency of lung adenocarcinoma grading
Introduction
An estimated 1.8 million people die of lung cancer every year, and nearly 85% of cases are non-small cell lung cancer (NSCLC), of which, lung adenocarcinoma (LUAD) is a common subtype (1,2). The degree of lung cancer differentiation is an important factor in the treatment and prognosis of patients (3,4). Computed tomography (CT) has become an important tool to diagnose NSCLC, but it is difficult to differentiate lung cancer through morphology and enhancement pattern using conventional CT. In dual-layer spectral detector CT (DLCT), which is a recent, novel energy-spectrum imaging method, the mutual interference between different energy rays is avoided and the accuracy of data is guaranteed (5). It also has the characteristics of synchronization, homology, and simultaneity, which can produce better data registration and image consistency at different stages, thus reducing measurement error (6).
Several studies have suggested that using the extra information acquired with DLCT can be used to better differentiate LUAD; however, the results have been inconsistent (7-10). Lin et al. found that the normalized iodine density (NID) and a slope of the spectral curve (λ) between 40 and 100 keV in the both phases were both valuable for grading adenocarcinoma, and λ had the best diagnostic efficiency in the venous phase (7). Fehrenbach et al. found that the iodine density (ID), NID, and λ between 40 and 120 keV of the poorly-differentiated group was higher than those of the well–moderately-differentiated group in the arterial phase, but there was no significant difference between them (8). Iwano et al. showed that the ID of poorly-differentiated lung cancer was lower than that of well–moderately-differentiated cancer (9). Li et al. showed that ID could reflect the degree of differentiation of tumors in the venous phase, and that the higher the differentiation, the higher the values of the corresponding spectral parameters (10). These inconsistent, contrasting results may have been due to several reasons such as single-phase scanning, investigating only one spectral parameter at a time, and the absence of morphological parameters (7,10).
Therefore, we conducted a prospective study using a double-phase enhanced scanning method, more spectral CT parameters, and combining morphological parameters with spectral CT parameters to improve the diagnostic efficiency of LUAD differentiation. We present the following study in accordance with the STARD reporting checklist (available at https://qims.amegroups.com/article/view/10.21037/qims-22-2/rc).
Methods
Patients
This was a prospective study of consecutive patients pathologically diagnosed with lung cancer who underwent enhanced lung DLCT scanning in the Radiology Department of Nanxishan Hospital of Guangxi Zhuang Autonomous Region between 1st August 2020 and 31st March 2021. The inclusion criteria were as follows: (I) DLCT scan within 7 days before surgery; (II) short diameter of tumor focus >2 cm, and solid type lung cancer; (III) no clinical antineoplastic treatment received before enrollment; (IV) complete clinical and DLCT data; (V) CT scan performed with breath holding; (VI) satisfactory image quality; and (VII) no other cancer history. The exclusion criteria were as follows: (I) untreated hyperthyroidism; (II) history of allergy to iodine contrast medium; and (III) complication of damage to the heart, liver, kidney, or other important organs. A flow chart of the inclusion/exclusion process is displayed in Figure 1.
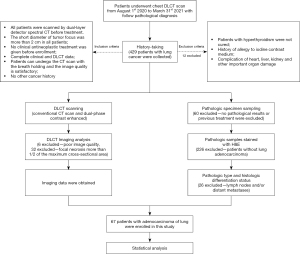
The study was conducted in accordance with the Declaration of Helsinki (as revised in 2013). The study was approved by the Ethics Committee of the Nanxishan Hospital of Guangxi Zhuang Autonomous Region (No. 2020KY-E-30) and informed consent was provided by all participants.
CT examination
All DLCT scans were performed with a Philips IQon Spectral CT scanner (Philips Healthcare, Amsterdam, The Netherlands). The scans included pulmonary CT conventional non-enhanced, and contrast-enhanced scans (both arterial and venous phase). The non-ionic contrast agent (70 mL iopromide; Beijing Beilu Pharmaceutical Co., Ltd., Beijing, China) was administered via an antecubital vein at an intended flow rate of 3.0 mL/s with a high-pressure syringe, followed by 30 mL of saline, injected at the same flow rate. Scans were performed using the bolus chase method. The region of interest (ROI) was located in the descending aorta with a trigger threshold CT number of 150 Hounsfield units (HU). The arterial and venous phases were started 6 and 36 seconds, respectively, after contrast agent injection. The spectral parameters included tube voltage of 120 kVp, spectral CT adaptive current, collimator width of 64 mm × 0.625 mm, pitch of 1.234, 0.27-second rotation time, and a matrix of 512×512. After the scans were completed, the data obtained in the enhanced double-phase were reconstructed by projected spatial spectral reconstruction (Spectral, level 4). The image reconstruction thickness was 1 mm and the image spacing was 1 mm.
Imaging analysis
The data were sent to a Philips Spectral Diagnostic Suite 9.0 workstation (Philips Healthcare), and were processed and analyzed by 2 radiologists with more than 5 years’ experience of chest CT diagnosis. To mitigate potential cognitive biases, the investigators were blinded to any patients’ clinical data. Then, 3 consecutive image slices containing the maximum cross-section of the tumor and the upper and lower layers were chosen for measurement, and round or oval ROIs were drawn as large as possible (covering between half and two-thirds of the lesion area) to minimize the influences of noise and the partial volume effect. First, ROIs were sketched on the contrast-enhanced and the unenhanced conventional CT images and the morphological parameters of the tumor were evaluated (short and long diameters, lobulation sign, spicule sign, pleural indentation sign, vessel convergence sign, bronchus encapsulated air sign, calcification, and liquefactive necrosis). The ROI was placed on solid regions of the tumor, avoiding areas with vessels, calcification, and cystic or necrotic change. Next, data were obtained for other image parameters [virtual monoenergetic CT images (40 and 70 keV), the effective atomic number (Zeff) images, the ID images, etc.]. The NID of lung lesions, normalized to the ID values of the aorta in the aortopulmonary window level, was used to minimize the influence of the individual circulation status and scanning times: NID = ID/IDaorta. The slope of the spectral curve (λ) was defined as the difference in CT number at 40 and 70 keV divided by the energy difference; λ = [CT number (40 keV) – CT number (70 keV)]/(70–40) keV.
Histochemical examination
Immunohistochemical (IHC) staining results were divided into poorly, moderately, and well-differentiated adenocarcinoma. Without knowing the clinical information and spectral CT results, the sampling of histochemical samples was carried out jointly by a pathologist and a radiologist to ensure that the samples covered, to the greatest possible extent, the same volumes as the ROIs in the scan data. The tumor specimens were analyzed by a pathologist with 14 years of IHC staining experience. All sections were numbered and evaluated, and their pathological types and histological differentiation were analyzed and recorded. The histologic criteria used for diagnosis were in accordance with the World Health Organization Classification of Lung Tumors (11,12).
Statistical analysis
Kappa and intraclass correlation coefficient (ICC) tests were used to test the consistency of the ROI measurement results of the 2 radiologists, with a kappa score >0.8 or ICC score >0.7 indicating satisfactory agreement between them. All parameters were then averaged over the measurements made by the 2 physicians. The Shapiro-Wilk test and Bartlett’s test were used on all measurements to test for normality and homogeneity of variance. The measurement data subject to normal distribution were expressed as mean ± standard deviation, and the counting data were expressed as n (%). One-way analysis of variance (ANOVA) was adopted for all measurement parameters, and chi-square test was adopted for counting data. For statistically significant parameters between the subgroups, a receiver operating characteristic (ROC) curve was drawn with the pathological examination results as the gold standard, and the area under the curve (AUC) was calculated. The AUC, the best diagnostic threshold, sensitivity, and specificity of the spectral parameters for differential diagnosis were analyzed. Finally, the prediction probability of the parameters with statistical difference between the 2 groups, including morphological parameters and morphological parameters combined with the most effective spectral parameters for diagnosis, was calculated by binary logistic analysis. The ROC curves were plotted by predicting probability to analyze their joint diagnostic efficiency. A two-tailed P<0.05 indicated that the difference was statistically significant.
All data were analyzed using SPSS 21.0 (IBM Corp., Armonk, NY, USA).
Results
Patients and conventional CT parameters
A total of 67 patients met the inclusion criteria, and because of the small number of highly-differentiated samples, patients were divided into 3 groups: the well-, well–moderately-differentiated group, and poorly-differentiated adenocarcinoma. As shown in Table 1, the CT number and CT increment number in the arterial phase of the well-, and well–moderately-differentiated group were higher than in the poorly-differentiated group (P<0.05). The incidence of the vascular cluster sign, air bronchial sign, and liquefaction necrosis in the well-, and well–moderately-differentiated group was lower than that in the poorly-differentiated group (P<0.05). There was no significant difference in the other parameters among the 3 groups.
Table 1
Parameter | Poorly-differentiated group (n=28) | Moderately-differentiated group (n=25) | Well–moderately-differentiated group (n=39) | χ2/F value | P value |
---|---|---|---|---|---|
General information | |||||
Gender (male)a | 16 (57.1) | 10 (40.0) | 17 (43.6) | 1.557 | 0.459 |
Age (years)b | 62.1±8.7 | 63.1±9.9 | 64.3±10.1 | 1.427 | 0.245 |
Morphological parameter | |||||
Short diameter (mm)b | 30.39±15.39 | 27.34±15.18 | 24.83±12.97 | 0.162 | 0.851 |
Long diameter (mm)b | 42.25±19.14 | 45.96±20.28 | 44.78±18.33 | 0.267 | 0.767 |
Lobulation sign (Y)a | 10 (35.7) | 15 (60.0) | 23 (59.0) | 3.557 | 0.169 |
Spicule sign (Y)a | 11 (39.3) | 9 (36.0) | 16 (41.0) | 0.752 | 0.687 |
Pleural indentation sign (Y)a | 11 (39.3) | 15 (60.0) | 23 (59.0) | 2.557 | 0.278 |
Vessel convergence sign (Y)a | 16 (57.1) | 10 (40.0) | 12 (30.8) | 7.100 | 0.029†‡ |
Bronchus encapsulated air sign (Y)a | 17 (60.7) | 8 (32.0) | 11 (28.2) | 7.493 | 0.024†‡ |
Calcification (Y)a | 1 (3.6) | 2 (8.0) | 3 (7.7) | 0.505 | 0.777 |
Liquefactive necrosis (Y)a | 18 (64.3) | 6 (24.0) | 11 (28.2) | 9.145 | 0.010†‡ |
Conventional CT | |||||
Unenhanced CT number (HU)b | 32.49±7.79 | 34.11±15.52 | 33.93±14.95 | 0.132 | 0.877 |
AP-CT number (HU)b | 72.12±16.45 | 84.10±15.46 | 85.03±15.02 | 9.381 | <0.001†‡ |
VP-CT number (HU)b | 87.64±16.91 | 95.29±19.01 | 98.85±20.97 | 1.468 | 0.236 |
AP-CT number# (HU)b | 39.65±13.69 | 47.83±22.07 | 51.07±20.34 | 6.340 | 0.003†‡ |
VP-CT number# (HU)b | 55.18±15.76 | 59.49±22.16 | 64.89±25.97 | 0.792 | 0.456 |
a, data are number of patients, with percentages in parentheses; b, data are mean ± standard deviation; †, difference between poorly-differentiated group and well–moderately-differentiated group; ‡, difference between poorly-differentiated group and moderately-differentiated group. AP, arterial phase; CT number#, CT increment number; CT, computed tomography; HU, Hounsfield unit; VP, venous phase; Y, yes.
CT spectral quantitative parameters
As shown in Table 2, except for NID in the venous phase, all CT spectral parameters in the well- and well–moderately-differentiated group in both the arterial and venous phases were higher than in the poorly-differentiated group (P<0.05).
Table 2
Parameter | Poorly-differentiated group (n=28) | Moderately-differentiated group (n=25) | Well–moderately-differentiated group (n=39) | F value | P value |
---|---|---|---|---|---|
AP | |||||
Zeff | 7.85±0.21 | 8.07±0.27 | 8.10±0.24 | 10.260 | <0.001†‡ |
ID | 0.99±0.34 | 1.38±0.53 | 1.43±0.48 | 8.258 | 0.001†‡ |
NID | 0.10±0.04 | 0.12±0.05 | 0.13±0.04 | 5.669 | 0.005†‡ |
λ | 1.99±0.68 | 2.76±1.06 | 2.87±0.96 | 8.340 | <0.001†‡ |
VP | |||||
Zeff | 8.08±0.15 | 8.28±0.32 | 8.29±0.28 | 6.184 | 0.003†‡ |
ID | 1.41±0.39 | 1.82±0.70 | 1.82±0.63 | 5.728 | 0.005†‡ |
NID | 0.31±0.07 | 0.39±0.17 | 0.40±0.17 | 3.852 | 0.025† |
λ | 2.76±0.63 | 3.64±1.40 | 3.68±1.23 | 6.153 | 0.003†‡ |
All data are mean ± standard deviation. †, difference between poorly-differentiated group and well–moderately-differentiated group; ‡, difference between poorly-differentiated group and moderately-differentiated group. DLCT, dual-layer spectral detector computed tomography; λ, slope of the spectral curve; AP, arterial phase; ID, iodine density; NID, normalized iodine density; VP, venous phase; Zeff, effective atomic number.
As shown in Table 3 and Figure 2, all CT spectral parameters had good diagnostic efficiency in differentiating the well–moderately- and poorly-differentiated groups, and the diagnostic efficiency of arterial phase parameters was higher than that of venous phase parameters (P<0.05). Among them, NID had the best diagnostic efficiency in the arterial phase.
Table 3
Parameter | AUC | Thresholds | Sensitivity (%) | Specificity (%) | 95% CI | P value |
---|---|---|---|---|---|---|
AP | ||||||
Zeff | 0.804 | 7.92 | 84.6 | 71.4 | 0.694–0.915 | <0.001 |
ID | 0.809 | 1.04 | 84.6 | 75.0 | 0.698–0.920 | <0.001 |
NID | 0.817 | 0.11 | 92.9 | 82.1 | 0.713–0.922 | <0.001 |
λ | 0.802 | 2.08 | 84.6 | 71.4 | 0.690–0.913 | <0.001 |
VP | ||||||
Zeff | 0.731 | 8.13 | 71.8 | 67.9 | 0.612–0.850 | 0.001 |
ID | 0.715 | 1.75 | 46.2 | 92.9 | 0.594–0.837 | 0.003 |
NID | 0.789 | 0.34 | 64.1 | 92.9 | 0.680–0.899 | <0.001 |
λ | 0.734 | 3.49 | 48.7 | 92.9 | 0.615–0.852 | 0.001 |
λ, slope of the spectral curve; AP, arterial phase; AUC, area under the curve; CI, confidence interval; ID, iodine density; NID, normalized iodine density; VP, venous phase; Zeff, effective atomic number.
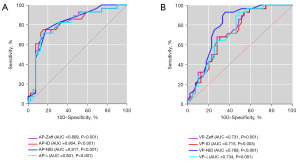
Diagnostic efficiency
As shown in Table 4 and Figure 3, ROC curve analysis of diagnosing the degree of differentiation of LUAD using morphological parameters alone, and combining morphological parameters with spectral parameters in arterial phase. When morphological parameters combined with NID were considered in the arterial phase, AUC =0.916, 95% confidence interval (CI): 0.908–0.999, and sensitivity and specificity of 96.4% and 82.1%, respectively (Figure 3).
Table 4
Parameter | AUC | Sensitivity (%) | Specificity (%) | 95% CI | P value |
---|---|---|---|---|---|
MP | 0.774 | 60.7 | 84.6 | 0.657–0.892 | <0.001 |
MP + AP-CT | 0.858 | 89.3 | 74.4 | 0.770–0.946 | <0.001 |
MP + AP-NID | 0.916 | 96.4 | 82.1 | 0.908–0.999 | <0.001 |
ROC, receiver operating characteristic; AUC, area under the curve; CI, confidence interval; CT, computed tomography; MP, morphological parameters; AP, arterial phase; MP + AP-CT, combination of morphology and CT enhancement value in the arterial phase; MP + AP-NID, combination of morphology and NID in the arterial phase; NID, normalized iodine density.
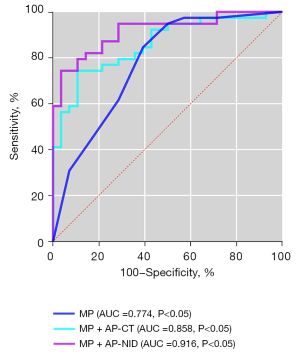
Reliability of measurements
For all the given parameters, the kappa and ICC tests found that the measurement results were consistent between the 2 radiologists (P>0.05).
Discussion
Compared with the venous phase, arterial phase spectral parameters had higher diagnostic efficiency for the pathological grade of LUAD, and NID had the best diagnostic efficiency in the arterial phase (Table 3, Figure 2). We also found that combining the spectral and morphological parameters significantly improved the diagnostic efficiency (Table 4, Figure 3).
Our results contradict those of previous studies that concluded that spectral parameters in the arterial phase had higher diagnostic efficiency (7,10), which may be due to the aggregation of contrast media in tumors and the time to peak (13,14). Our study showed that the diagnostic efficiency of the arterial phase was better than that of the venous phase. Based on the scan time of the conventional chest CT, the arterial phase was scanned at 6 seconds after contrast injection and the venous phase after 36 seconds. For the enhanced CT, the ID level of tumors is mainly affected by the degree of neovascularity (15). Abnormalities of angiogenesis and the vascular network in lung cancer tissues do not support rapid tumor growth (14). Generally, the higher the tumor malignancy, the faster the tumor vessels grow, the higher the microvessel density, and the more sufficient the blood supply (16). Arterial ID mainly reflects tumor blood supply and capillary density, whereas venous ID is considered an indicator of blood supply balance and may reflect late retention of contrast agent in the stroma (17). Moreover, in the arterial stage, due to the disorderly and tortuous microvessel network, the flow rate of contrast medium is often slow, leading to poor microvessel filling in well–moderately-differentiated tumor cells. Over time, the contrast retention within a poorly-differentiated tumor gradually increased by the time of scanning at the venous stage, resulting in the difference between the differently differentiated tumors, so we believe that the spectral parameters can better reflect the tumor microcirculation at the arterial stage. Our statistical analysis and ROC results indicated that the optimal quantitative parameters that maximize sensitivity and specificity can be generated from the arterial period alone. This finding suggests that reductions in scanning time and radiation dose are possible, and can be applied in clinical practice. Li et al. showed in a study of differentiating gastric adenocarcinoma that NID had higher diagnostic efficiency in the arterial phase (18), thus lending further support to the hypothesis that the arterial phase more effectively reflects differences in iodine uptake between tumors with different levels of differentiation.
Previous studies have shown that spectral CT has great value in tumor differentiation. Chuang-Bo et al. found that the spectral CT parameters in the arterial phase showed improved accuracy for evaluating the degree of differentiation in colon cancer (19). Liang et al. showed that NID is a useful predictor of angiogenesis and degree of differentiation of moderately and poorly-differentiated gastric adenocarcinomas (20). Liu et al. reported that spectral quantitative parameters can be used to evaluate the pathological grading of esophageal squamous cell carcinoma (13). Yingying et al. showed that the DLCT technology can be used as a supplementary method to provide more information for preoperative grading of gliomas and the prognosis of patients (21). As shown in Table 3 and Figure 2A, we found that among all the spectral parameters, NID had the highest diagnostic efficiency in the arterial phase, and the NID of poorly-differentiated adenocarcinoma was lower than that of well–moderately-differentiated adenocarcinoma; that is, the iodine uptake rate of well–moderately-differentiated adenocarcinoma is higher than that of poorly-differentiated adenocarcinoma. In this regard, our results are consistent with previous studies (13,19,20,21). However, some research results are inconsistent with our conclusions (7,9,10). Lin et al. found that λ in the venous phase provided the best diagnostic efficiency in distinguishing poorly-differentiated cancers from well–moderately-differentiated cancers (7). Iwano et al. found that poorly-differentiated tumors tended to have lower ID than well–moderately-differentiated tumors (9). Li et al. believe that ID in the venous phase is a useful indicator for evaluating tumor angiogenesis and prognosis (10). However, their samples were NSCLC rather than LUAD and NID is mainly affected by the iodine uptake of the lesion itself. For example, the NID of lesions with severe necrosis may be affected by severe ID deviation (10). Although it is difficult to completely avoid micro-necrotic areas in tumors when measuring parameters, we avoided obvious necrotic areas when sketching the ROIs, which should reduce the result bias. Therefore, we believe that NID has the highest diagnostic efficiency in the arterial phase.
As shown in Table 4 and Figure 3, although the enhancement degree of traditional CT can also reflect the blood supply of tumors, and all spectral parameters significantly positively correlate with the enhancement degree of CT, spectral parameters are more effective in predicting the differentiation degree of LUAD. Furthermore, it has been shown that DLCT may reduce the influence of beam hardening and improve measurement accuracy (21), which further confirms that spectral parameters have better diagnostic efficiency than CT increment number and can better reflect the pathological characteristics of LUAD.
In the present study, there were significant differences of the spectral parameters between poorly-differentiated and moderately-differentiated adenocarcinoma; except for NID in the venous phase, the same results appeared for the poorly-differentiated and well–moderately-differentiated groups. Liu et al. (13) found that spectral quantitative parameters were different between poorly-, moderately-, and well-differentiated esophageal squamous cell carcinoma, and that NID in the arterial phase had the highest differential diagnostic efficiency, which may indicate that capillary density and tumor neovascularization increase with decreasing tumor differentiation. However, there were no differences in the spectral parameters between the moderately-differentiated and well–moderately-differentiated groups in our study, which may be due to sample overlap between the 2 groups. In addition, the small sample size for the well-differentiated group may be another reason.
To the best of our knowledge, our study is the first to combine morphological and spectral parameters to assess the degree of differentiation of LUAD. As shown in Table 1, many morphological signs of LUAD (i.e., the vessel convergence sign, bronchus encapsulated air sign, and liquefactive necrosis) were higher in poorly-differentiated tumors than in well–moderately-differentiated tumors. In previous studies, the morphological characteristics of lung cancer were not combined with spectral parameters to assess the degree of differentiated of LUAD (7-10) and conventional CT has some limitations in identifying the degree of differentiation by morphological signs (22). Combining the morphological parameters with spectral parameters can better estimate the degree of differentiation.
There were still several potential limitations in our study. First, the results were limited by the relatively small sample. A larger sample is required to further confirm the reliability of the results. Second, because of calcification, enhanced vascular shadow, and liquefaction necrosis, it was difficult to be completely consistent in delineating the ROI on the images with the pathological section. Third, the spectral parameters of unenhanced CT were not included in this study, though they may be of certain significance for the identification of material components. Combining spectral parameters with morphology has better diagnostic efficacy than CT enhancement values, and thus can better reflect the pathological features of LUAD. In future research, longitudinal studies will need to be conducted, and more attention should be paid to the combination of spectral CT and morphological parameters to predict tumor invasion, lymph node metastasis, tumor recurrence, and prognosis.
In conclusion, DLCT quantitative parameters, especially NID in the arterial phase, may provide valuable information for the differentiation of well–moderately- and poorly-differentiated LUAD. In addition, the diagnostic efficiency can be further improved by combining the spectral and morphological parameters.
Acknowledgments
The authors thank the devoted staff who contributed to the recruitment, screening, and enrollment of the patients in this study.
Funding: None.
Footnote
Reporting Checklist: The authors have completed the STARD reporting checklist. Available at https://qims.amegroups.com/article/view/10.21037/qims-22-2/rc
Conflicts of Interest: All authors have completed the ICMJE uniform disclosure form (available at https://qims.amegroups.com/article/view/10.21037/qims-22-2/coif). XZ is employed by Philips (China) Investment Co., Ltd., Chengdu Branch. The other authors have no conflicts of interest to declare.
Ethical Statement: The authors are accountable for all aspects of the work in ensuring that questions related to the accuracy or integrity of any part of the work are appropriately investigated and resolved. The study was conducted in accordance with the Declaration of Helsinki (as revised in 2013). The study was approved by the Ethics Committee of the Nanxishan Hospital of Guangxi Zhuang Autonomous Region (No. 2020KY-E-30) and informed consent was provided by all participants.
Open Access Statement: This is an Open Access article distributed in accordance with the Creative Commons Attribution-NonCommercial-NoDerivs 4.0 International License (CC BY-NC-ND 4.0), which permits the non-commercial replication and distribution of the article with the strict proviso that no changes or edits are made and the original work is properly cited (including links to both the formal publication through the relevant DOI and the license). See: https://creativecommons.org/licenses/by-nc-nd/4.0/.
References
- Sher T, Dy GK, Adjei AA. Small cell lung cancer. Mayo Clin Proc 2008;83:355-67. [Crossref] [PubMed]
- Sung H, Ferlay J, Siegel RL, Laversanne M, Soerjomataram I, Jemal A, Bray F. Global Cancer Statistics 2020: GLOBOCAN Estimates of Incidence and Mortality Worldwide for 36 Cancers in 185 Countries. CA Cancer J Clin 2021;71:209-49. [Crossref] [PubMed]
- Zheng XQ, Huang JF, Lin JL, Chen L, Zhou TT, Chen D, Lin DD, Shen JF, Wu AM. Incidence, prognostic factors, and a nomogram of lung cancer with bone metastasis at initial diagnosis: a population-based study. Transl Lung Cancer Res 2019;8:367-79. [Crossref] [PubMed]
- Saijo N, Niitani H, Tominaga K, Eguchi K, Koketsu H, Fujino T, Ishikawa S. Comparison of survival in nonresected well differentiated and poorly differentiated adenocarcinoma of the lung. J Cancer Res Clin Oncol 1980;97:71-9. [Crossref] [PubMed]
- Hickethier T, Wenning J, Bratke G, Maintz D, Michels G, Bunck AC. Evaluation of soft-plaque stenoses in coronary artery stents using conventional and monoenergetic images: first in-vitro experience and comparison of two different dual-energy techniques. Quant Imaging Med Surg 2020;10:612-23. [Crossref] [PubMed]
- Gao L, Lu X, Wen Q, Hou Y. Added value of spectral parameters for the assessment of lymph node metastasis of lung cancer with dual-layer spectral detector computed tomography. Quant Imaging Med Surg 2021;11:2622-33. [Crossref] [PubMed]
- Lin LY, Zhang Y, Suo ST, Zhang F, Cheng JJ, Wu HW. Correlation between dual-energy spectral CT imaging parameters and pathological grades of non-small cell lung cancer. Clin Radiol 2018;73:412.e1-7. [Crossref] [PubMed]
- Fehrenbach U, Kahn J, Böning G, Feldhaus F, Merz K, Frost N, Maurer MH, Renz D, Hamm B, Streitparth F. Spectral CT and its specific values in the staging of patients with non-small cell lung cancer: technical possibilities and clinical impact. Clin Radiol 2019;74:456-66. [Crossref] [PubMed]
- Iwano S, Ito R, Umakoshi H, Ito S, Naganawa S. Evaluation of lung cancer by enhanced dual-energy CT: association between three-dimensional iodine concentration and tumour differentiation. Br J Radiol 2015;88:20150224. [Crossref] [PubMed]
- Li Q, Li X, Li XY, Huo JW, Lv FJ, Luo TY. Spectral CT in Lung Cancer: Usefulness of Iodine Concentration for Evaluation of Tumor Angiogenesis and Prognosis. AJR Am J Roentgenol 2020;215:595-602. [Crossref] [PubMed]
- Travis WD, Brambilla E, Nicholson AG, Yatabe Y, Austin JHM, Beasley MB, Chirieac LR, Dacic S, Duhig E, Flieder DB, Geisinger K, Hirsch FR, Ishikawa Y, Kerr KM, Noguchi M, Pelosi G, Powell CA, Tsao MS, Wistuba I, Panel WHO. The 2015 World Health Organization Classification of Lung Tumors: Impact of Genetic, Clinical and Radiologic Advances Since the 2004 Classification. J Thorac Oncol 2015;10:1243-60. [Crossref] [PubMed]
- Travis WD, Brambilla E, Noguchi M, Nicholson AG, Geisinger KR, Yatabe Y, et al. International association for the study of lung cancer/american thoracic society/european respiratory society international multidisciplinary classification of lung adenocarcinoma. J Thorac Oncol 2011;6:244-85. [Crossref] [PubMed]
- Liu YH, Zhu SC, Shi DP, Wei Y, Sun MH, Wu S, Li LL. Clinical value of spectral CT imaging in preoperative evaluation of pathological grading of esophageal squamous cell carcinoma. Zhonghua Yi Xue Za Zhi 2017;97:3406-11. [PubMed]
- Yu Y, Wang X, Shi C, Hu S, Zhu H, Hu C. Spectral Computed Tomography Imaging in the Differential Diagnosis of Lung Cancer and Inflammatory Myofibroblastic Tumor. J Comput Assist Tomogr 2019;43:338-44. [Crossref] [PubMed]
- Satoh A, Shuto K, Okazumi S, Ohira G, Natsume T, Hayano K, Narushima K, Saito H, Ohta T, Nabeya Y, Yanagawa N, Matsubara H. Role of perfusion CT in assessing tumor blood flow and malignancy level of gastric cancer. Dig Surg 2010;27:253-60. [Crossref] [PubMed]
- Du JR, Jiang Y, Zhang YM, Fu H. Vascular endothelial growth factor and microvascular density in esophageal and gastric carcinomas. World J Gastroenterol 2003;9:1604-6. [Crossref] [PubMed]
- Pan Z, Pang L, Ding B, Yan C, Zhang H, Du L, Wang B, Song Q, Chen K, Yan F. Gastric cancer staging with dual energy spectral CT imaging. PLoS One 2013;8:e53651. [Crossref] [PubMed]
- Li R, Li J, Wang X, Liang P, Gao J. Detection of gastric cancer and its histological type based on iodine concentration in spectral CT. Cancer Imaging 2018;18:42. [Crossref] [PubMed]
- Chuang-Bo Y, Tai-Ping H, Hai-Feng D, Yong-Jun J, Xi-Rong Z, Guang-Ming M, Chenglong R, Jun W, Yong Y. Quantitative assessment of the degree of differentiation in colon cancer with dual-energy spectral CT. Abdom Radiol (NY) 2017;42:2591-6. [Crossref] [PubMed]
- Liang P, Ren XC, Gao JB, Chen KS, Xu X. Iodine Concentration in Spectral CT: Assessment of Prognostic Determinants in Patients With Gastric Adenocarcinoma. AJR Am J Roentgenol 2017;209:1033-8. [Crossref] [PubMed]
- Yingying L, Zhe Z, Xiaochen W, Xiaomei L, Nan J, Shengjun S. Dual-layer detector spectral CT-a new supplementary method for preoperative evaluation of glioma. Eur J Radiol 2021;138:109649. [Crossref] [PubMed]
- Li Q, Li X, Li XY, He XQ, Chu ZG, Luo TY. Histological subtypes of solid-dominant invasive lung adenocarcinoma: differentiation using dual-energy spectral CT. Clin Radiol 2021;76:77.e1-7. [Crossref] [PubMed]