The value of magnetic resonance imaging-based tumor shape features for assessing microsatellite instability status in endometrial cancer
Introduction
Endometrial cancer (EC) ranks the sixth most common cancer type in women worldwide (1). Traditionally, the prognosis of EC has depended on the type of pathology that was first proposed by Bokhman in 1983 (2). Type I cancers (65%) are associated with endometrial hyperplasia, obesity, hyperlipidemia, estrogen excess, moderate or high differentiation, high sensitivity to progestogens, and a favorable outcome. In contrast, type II cancers (35%) often lack the above signs and are associated with poor differentiation and less favorable outcomes (2). However, the classification system cannot completely elucidate the gene heterogeneity and molecular diversity, which could assist treatment options and prognosis evaluations in EC patients (3-5). Microsatellite instability (MSI) status, a hypermutable phenotype commonly resulting from defective mismatch repair (MMR) proteins, affects about 30% of patients with EC (6). Recently, MSI status has been considered a promising molecular subtype for risk stratification and therapeutic interventions in patients with EC (3). The US Food and Drug Administration has approved pembrolizumab for the treatment of all advanced MSI-high or deficient MMR solid tumors. Moreover, MSI status testing is also recommended by the National Comprehensive Cancer Network guidelines in detecting Lynch syndrome for EC patients (7). Identifying EC patients with Lynch syndrome can facilitate early detection of other Lynch syndrome-related cancers and find at-risk relatives (8).
MSI status can be tested by immunohistochemistry, polymerase chain reaction, and next-generation sequencing techniques. These methods are based on tumor specimens from invasive biopsy or surgical excision. Additionally, even though MSI screening is recommended for EC patients, it is rarely used in routine clinical practice due to its high cost and dependence on technology, such as sufficient tissue samples and the lengthy process of ordering the test (9,10). Therefore, it is vital to find a noninvasive, interpretable, and easy-to-use method to assess the MSI status in patients with EC.
In recent years, shape and texture features extracted from medical images have been used to assess MSI status in colorectal cancer and EC (11-16). Moreover, quantitative shape features have also been used to describe tumor aggressiveness and predict the gene expression in other tumors (17,18). Compactness can help differentiate benign from malignant pleural lesions (17) and has a specific correlation with human papillomavirus status in head and neck cancers (18). Moreover, subjective shape features, including the shape type and tumor margin, have been used for diagnosing prostate cancer and breast cancer in clinical practice with reference to the Prostate Imaging Reporting and Data System, version 2 (PI-RADS v.2) and the Breast Imaging Reporting and Data System version 5 (BI-RADS v.5), respectively. In EC, large tumor volumes and diameters based on magnetic resonance imaging (MRI) have been associated with poor prognosis (19,20).
This study aimed to explore whether MRI-based tumor shape features could help assess MSI status in patients with EC. Furthermore, most patients with EC are diagnosed at early stages with good prognoses, while those at advanced stages have poor outcomes (21). We intended to conduct a subgroup analysis according to the International Federation of Gynecology and Obstetrics (FIGO) staging system. We present the following article in accordance with the TRIPOD reporting checklist (available at https://qims.amegroups.com/article/view/10.21037/qims-22-77/rc).
Methods
Patients
The study was conducted in accordance with the Declaration of Helsinki (as revised in 2013). The study was approved by the research Ethics Committee of Guangdong Provincial People’s Hospital, and individual consent for this retrospective analysis was waived. From August 2016 to June 2021, 100 patients with histologically confirmed EC and preoperative pelvic 3.0 T MRI examinations were eligible for this study. The inclusion criteria were as follows: (I) those without neoadjuvant therapy for EC before surgery; and (II) those with MSI status evaluation. The exclusion criteria were as follows: (I) those without contrast-enhanced T1-weighted imaging (CE-T1WI; n=3); (II) those with severe MRI artifacts (n=2); and (III) those with a tumor diameter of <1 cm or that was hard to identify (n=7). In all, the remaining 88 patients constituted the study population.
Patient baseline clinicopathologic characteristics, including age, menopausal status, histological type, tumor grade, FIGO stage, and tumor markers, were collected from the electronic medical record system. Tumor markers included carbohydrate antigen 125 (CA125), human epididymis protein 4 (HE4), and carbohydrate antigen 19-9 (CA19-9), with thresholds of ≤35 U/mL, ≤70 pmol/L, and ≤27 U/mL, respectively, based on the normal ranges used at our institution.
MSI status assessment
The MSI status was assessed by immunohistochemical staining using formalin-fixed, paraffin-embedded EC tissue samples. Four primary antibodies (MLH1, MSH2, MSH6, and PMS2) were included in the process. The negative nuclear staining of tumor cells was defined as protein deficient, and any positive nuclear staining was defined as protein proficient. Cases with at least 1 deficient protein were defined as MSI; cases with 4 proficient proteins were classified as microsatellite stability (MSS) (22).
MRI protocols
Preoperative pelvic CE-MRI images were acquired with the GE 3.0 T system (Signa Excite; GE Healthcare, Chicago, IL, USA) for 35 patients and the Philips 3.0 T system (Ingenia; Philips Healthcare, Amsterdam, Netherlands) for the remaining 53 patients. The mean interval between MRI and surgical staging was 7.9 (range, 1–59) days. All patients were required to keep an empty stomach and undergo proper bowel preparation to avoid the artifacts of intestinal gas before MRI examination. The MRI protocols included axial-oblique T2-weighted imaging (T2WI), axial-oblique diffusion-weighted imaging (DWI; including b values of 0/600/800/1,000 s/mm2), and CE-T1WI. CE-T1WI was obtained after administration of 0.1 mmol/kg of the gadolinium-based contrast agent, Magnevist (Schering, Berlin, Germany). Apparent diffusion coefficient (ADC) maps were generated by DWI images. The mean ADC values of tumors were recorded for further analysis in our study. The parameters of all the protocols are provided in Table 1.
Table 1
Scanner | Patients | Sequence | TR/TE (ms) | Matrix | Slice thickness (mm) | Slice gap (mm) | Flip angle | B value (s/mm2) |
---|---|---|---|---|---|---|---|---|
GE Medical Systems | 35 | T2WI | 2,800/119 | 512×512 | 5 | 6 | 90° | N/A |
CE-T1WI | 4/2 | 512×512 | 4 | 2 | 12° | N/A | ||
DWI | 5,200/97 | 256×256 | 5 | 6 | 90° | 0/600/800 | ||
Philips Medical Systems | 53 | T2WI | 1,500/70 | 512×512 | 5 | 6 | 90° | N/A |
CE-T1WI | 3.9/1.37 | 432×432 | 4 | 2 | 10° | N/A | ||
DWI | 6,322/83 | 224×224 | 5 | 7 | 90° | 0/600/800/1,000 |
MRI, magnetic resonance imaging; T2WI, T2-weighted imaging; CE-T1WI, contrast-enhanced T1-weighted imaging; DWI, diffusion-weighted imaging; TR, repetition time; TE, echo time; N/A, not applicable.
Tumor annotation and quantitative shape features extraction
We retrieved the equilibrium phases of axial-oblique CE-T1WI sequences (2–3 min after injection of contrast agents), which showed the maximum contrast between EC tumor and normal myometrium for tumor annotations (23). Axial-oblique T2WI sequences were also retrieved for tumor annotations to check the consistency of quantitative shape features with CE-T1WI. On T2WI, the tumor region was defined as the area of intermediate signal intensity that was different from normal hypointense myometrium and hyperintense endometrium. Tumor annotations were performed with ITK-SNAP software (version 3.8.0; http://www.itksnap.org) (24). Regions of interest (ROIs) of tumors were manually annotated slice by slice by radiologist A (with 4 years of experience in reading pelvic MRI) for all cases on CE-T1WI and T2WI, respectively, to analyze the correlation of quantitative shape features. The ROIs included areas of hemorrhage, necrosis, and cystic tissue. Volumes of interest (VOIs) were then constructed by integrating ROIs of all slices for each tumor. We randomly selected 30 cases for tumor annotation by radiologist B (with 7 years of experience in reading pelvic MRI) on CE-T1WI. The two radiologists were blinded to MSI status during segmentation.
Four quantitative shape features, including the longest diameter, volume, eccentricity, and solidity, were extracted and analyzed with MATLAB R2019a (MathWorks Inc., Natick, MA, USA). The quantitative shape feature definitions and calculation methods are shown in Table 2. Figure 1 displays the 4 quantitative shape features based on CE-T1WI.
Table 2
Shape feature | Definition or calculation |
---|---|
Longest diameter | Longest diameter of the ROI, in cm |
Volume | Number of voxels in the ROI, in mm3 |
Solidity | Ratio of the number of voxels in the ROI to the number of voxels in the 3D convex hull of the ROI (smallest polyhedron containing the ROI) |
Eccentricity | Metric given by where c, a, and b are the longest semi-principal axes, the second and third longest semi-principal axes of the ellipsoid, respectively |
ROI, region of interest; 3D, three-dimensional.
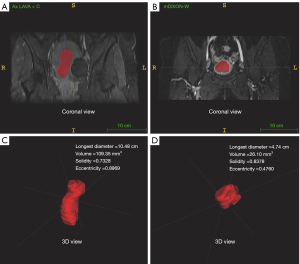
Subjective shape features evaluation
Four subjective shape features, including margin (distinct or indistinct), shape type (regular or irregular), extension beyond corpus (yes or no), and myometrial invasion (≤50% or >50%), were evaluated by radiologist A using the in-house picture archiving and communication system. The margin of the tumor was evaluated on T2WI, which was classified as “distinct” if the tumor margin was clear to identify and “indistinct” if the tumor margin was fuzzy. The shape type of tumor was evaluated on CE-T1WI, which was classified as “regular” if the tumor shape type was oval or round and “irregular” if the tumor shape type was irregular. The extension beyond the corpus was also evaluated on CE-T1WI, which was classified as “yes” if the tumor grew out of the uterine corpus into the cervix or outside the uterus and “no” if the tumor was confined to the uterine corpus. The depth of myometrial invasion was evaluated on CE-T1WI, T2WI, and the DWI, which was classified as “≤50%” if the tumor invaded less than or equal to 50% of the myometrial thickness and “>50%” if the tumor invaded more than 50% of the myometrium thickness. A total of 30 patients were randomly selected by stratified sampling for subjective features assessment in the same way by radiologist B.
Statistical analysis
The differences in clinicopathological characteristics and shape features were assessed by using the Student’s t-test, χ2 test, or Wilcoxon rank-sum test, as appropriate. For measuring interrater reliability, the intraclass correlation coefficient (ICC) was used for continuous quantitative variables, while kappa statistics were used for categorical variables. Pearson correlation coefficient (Pearson r) was used to assess the correlation of continuous quantitative variables. Univariate and multivariate analyses were performed by logistic regression model for MSI status. The feature with a P value <0.05 in the univariate analysis was included in the multivariate analysis. The receiver operating characteristic (ROC) curve was plotted, and the area under the curve (AUC) was calculated to evaluate the predictive performance of shape features for MSI status. A P value <0.05 was considered statistically significant. All statistical analyses were performed with R language (v.4.0.3; The R Project for Statistical Computing, Vienna, Austria).
Results
Patients
A total of 88 patients with EC were finally included in our study. The MSI group included 23 patients (26.1%), and the MSS group included 65 patients (73.9%). The mean age at diagnosis was 54.95 years, with a standard deviation (SD) of 9.08 years. Histologically, 84 were endometrioid, 2 were serous, and 2 were mixed carcinomas (endometrioid mixed clear cell). Among the 84 endometrioid tumors, there were 28, 45, and 11 for grade 1, grade 2, and grade 3 tumors, respectively. For the FIGO stage, 65 of the tumors were stage I, 2 were stage II, 18 were stage III, and 3 were stage IV. The clinicopathological characteristics are summarized in Table 2. No significant differences were found between MSI and MSS in all clinicopathological characteristics (all P values >0.05; Table 3).
Table 3
Characteristics | MSS (n=65) | MSI (n=23) | P value |
---|---|---|---|
Age (years), mean ± SD | 54.88±10.08 | 55.17±5.47 | 0.861 |
Menopausal status, n (%) | 1.00 | ||
Yes | 38 (58.5) | 13 (56.5) | |
No | 27 (41.5) | 10 (43.5) | |
Histology, n (%) | 1.00 | ||
Endometrioid | 62 (95.4) | 22 (95.7) | |
Serous | 2 (3.1) | 0 (0.0) | |
Mixed | 1 (1.5) | 1 (4.3) | |
Tumor grade, n (%) | 0.738 | ||
Grade I | 22 (33.9) | 6 (26.1) | |
Grade II | 31 (47.7) | 14 (60.9) | |
Grade III | 9 (13.8) | 2 (8.7) | |
Not available | 3 (4.6) | 1 (4.3) | |
FIGO stage, n (%) | 0.522 | ||
I | 48 (73.8) | 17 (73.9) | |
II | 2 (3.1) | 0 (0.0) | |
III | 12 (18.5) | 6 (26.1) | |
IV | 3 (4.6) | 0 (0.0) | |
LVSI, n (%) | 0.188 | ||
Absent | 53 (81.5) | 15 (65.2) | |
Present | 12 (18.5) | 8 (34.8) | |
CA125, n (%) | 1.00 | ||
Normal | 35 (53.8) | 13 (56.5) | |
Abnormal | 30 (46.2) | 10 (43.5) | |
HE4, n (%) | 0.835 | ||
Normal | 17 (26.2) | 6 (26.1) | |
Abnormal | 47 (72.3) | 17 (73.9) | |
Unknown | 1 (1.5) | 0 (0.0) | |
CA19-9, n (%) | 0.698 | ||
Normal | 38 (58.5) | 12 (52.2) | |
Abnormal | 26 (40.0) | 11 (47.8) | |
Unknown | 1 (1.5) | 0 (0.0) |
SD, standard deviation; FIGO, International Federation of Gynecology and Obstetrics; LVSI, lymphovascular space invasion; CA125, carbohydrate antigen 125; HE4, human epididymis protein 4; CA19-9, carbohydrate antigen 19-9; MSI, microsatellite instability; MSS, microsatellite stability.
Shape features and MSI status
Quantitative shape features were normalized based on z score normalization, as shown in Figure 2. Among quantitative shape features, no significant difference was found in the longest diameter, volume, or solidity between the two groups (all P values >0.05; Figure 3A-3C). Eccentricity had a statistically significant difference between the MSI and MSS groups (P=0.039; Figure 3D). Tumors with MSI often showed higher eccentricities than those with MSS in EC. Among the 30 randomly selected patients, eccentricity showed a good interrater reliability [ICC =0.981; 95% confidence interval (CI): 0.962–0.991; Figure 3E]. Moreover, a strong correlation was observed between CE-T1WI–derived and T2WI-derived eccentricity (Pearson r=0.68; P<0.001; Figure 3F). Using the median value of 0.74 as the threshold, patients were stratified into two groups: eccentricity low (≤0.74) and eccentricity high (>0.74). The percentages of patients with MSI vs. MSS in the two groups were 13.6% vs. 86.4%, and 38.6% vs. 61.4%, respectively (P=0.032; Figure 3G).
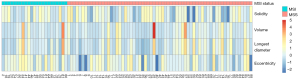
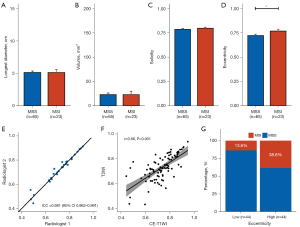
For subjective shape features, shape type (regular vs. irregular) showed a significant difference between the MSI and MSS groups (P=0.033). In the MSI group, 14 (61%) tumors were classified as regular shape, compared with 23 (35%) tumors in the MSS group. The assessment of shape type showed good interrater reliability (kappa value =0.789). In contrast, no significant difference was found in the margin, extension beyond corpus, or myometrial invasion between MSI and MSS (all P values >0.05).
Univariate and multivariate analyses
In the univariate analysis, eccentricity and shape type showed significant differences between MSI and MSS [high vs. low, odds ratio (OR) =3.99, 95% CI: 1.39–11.43, P=0.010; irregular vs. regular, OR =0.35, 95% CI: 0.13–0.94, P=0.037; Table 4]. No significant differences were found in the ADC value, clinicopathological characteristics, and other shape features. Finally, eccentricity and shape type were included in the multivariate analysis, and both remained as independent indicators of MSI status [high vs. low, adjusted OR (AOR) =4.49, 95% CI: 1.50–13.5, P=0.007; irregular vs. regular, AOR =0.30, 95% CI: 0.11–0.86, P=0.026; Table 4].
Table 4
Risk factors | Univariable analysis | Multivariable analysis | |||
---|---|---|---|---|---|
OR (95% CI) | P value | AOR (95% CI) | P value | ||
Age (years) | 1.00 (0.95–1.06) | 0.892 | |||
HE4 | |||||
Normal | Ref | ||||
Abnormal | 1.02 (0.35–3.03) | 0.965 | |||
CA125 | |||||
Normal | Ref | ||||
Abnormal | 0.90 (0.34–2.34) | 0.825 | |||
ADC | 0.65 (0.15–2.83) | 0.552 | |||
Tumor grade | |||||
Grade I | Ref | ||||
Grade II | 1.66 (0.55–4.98) | 0.369 | |||
Grade III | 0.81 (0.14–4.82) | 0.821 | |||
FIGO stage | |||||
I | Ref | ||||
II | 0 (0–Inf) | 0.995 | |||
III | 1.41 (0.46–4.35) | 0.548 | |||
IV | 0 (0–Inf) | 0.994 | |||
Longest diameter | 1.00 (0.79–1.28) | 0.977 | |||
Volume | 1.00 (0.98–1.02) | 0.962 | |||
Solidity | 9.62 (0.01–8389) | 0.512 | |||
Eccentricity | |||||
Low | Ref | Ref | |||
High | 3.99 (1.39–11.43) | 0.010* | 4.49 (1.50–13.5) | 0.007* | |
Margin | |||||
Distinct | Ref | ||||
Indistinct | 0.44 (0.15–1.25) | 0.124 | |||
Shape | |||||
Regular | Ref | Ref | |||
Irregular | 0.35 (0.13–0.94) | 0.037* | 0.30 (0.11–0.86) | 0.026* | |
Extension beyond corpus | |||||
No | Ref | ||||
Yes | 1.00 (0.34–2.94) | 0.995 | |||
Myometrial invasion | |||||
≤50% | Ref | ||||
>50% | 0.95 (0.36–2.45) | 0.908 |
*, P<0.05. HE4, human epididymis protein 4; CA125, carbohydrate antigen 125; ADC, apparent diffusion coefficient; FIGO, International Federation of Gynecology and Obstetrics; OR, odds ratio; AOR, adjusted odds ratio; CI, confidence interval; Ref, reference; Inf, infinity.
Patients with MSI were grouped based on the eccentricity and shape type: eccentricity high and regular shape, eccentricity high and irregular shape, eccentricity low and regular shape, and eccentricity low and irregular shape; the percentages of MSI status patients were 55%, 27%, 21%, and 8%, respectively (Figure 4A). The ROC curves are depicted in Figure 4B. The AUC values of the eccentricity and the shape type to assess MSI were 0.662 (95% CI: 0.554–0.770) and 0.627 (95% CI: 0.512–0.743), respectively. When combining the eccentricity and the shape type to assess MSI, the AUC value increased to 0.727 (95% CI: 0.613–0.842).
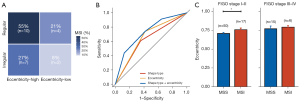
Subgroup analysis
Furthermore, we conducted a subgroup analysis of eccentricity according to the FIGO stage. Eccentricity still showed a significant difference in stages I–II (P=0.039; Figure 4C, left panel). There was no statistical difference in stages III–IV between the MSI and MSS groups (P=0.601; Figure 4C, right panel).
Discussion
Our study indicated that MRI-based tumor quantitative and subjective shape features might help identify MSI status in EC patients. On univariate and multivariate analyses, eccentricity and shape type showed significant differences between MSI and MSS, and those with higher eccentricity and regular shape based on CE-T1WI were more prone to tumors with MSI than those with MSS. Multivariate analysis showed that eccentricity and shape type were independent indicators of MSI status. In the FIGO stage subgroup analysis, the difference of eccentricity remained significant in stages I–II but not significant in stages III–IV.
Quantitative features, including shape features extracted from radiological images, have been used to assess MSI status in colorectal cancer and have achieved good performances (11-13). Moreover, quantitative shape features such as compactness can help differentiate benign from malignant pleural lesions (17) and are related to human papillomavirus status in head and neck cancers (18). In EC, the quantitative features extracted from the CE-T1WI sequence have been used for predicting prognosis and detecting lymphovascular space invasion (LVSI) and deep myometrial infiltration in EC (25-27). Some studies have also confirmed the valuable role of quantitative shape features based on MRI in the assessment of EC (19,20). Tumor volume measured on MRI is highly associated with disease progression in patients with EC (19). The potential value of tumor size based on MRI has also been investigated in assessing the tumor aggressiveness of EC (20). Different from previous assessments of the disease progression and tumor aggressiveness, we attempted to investigate the value of quantitative shape features based on CE-T1WI in assessing MSI status. In this study, we found that the eccentricities of tumors with MSI were higher than those with MSS in EC and showed independent predictive value for MSI status. Our study may add insights into MRI-based shape features for MSI assessment to the literature.
In clinical practice, subjective shape features are vital for diagnosing disease and assessing tumor aggression, such as shape type and tumor margin evaluated by referring to PI-RADS v.2 in prostate cancer and BI-RADS v.5 in breast cancer. Pelvic MRI images are widely performed preoperatively for local staging in EC, and the statuses of extension beyond corpus and myometrial invasion influence the FIGO stage of EC (28,29). In this study, we assessed the shape type, margin, extension beyond corpus, and myometrial invasion based on relative MRI sequences. We found that regular shapes based on CE-T1WI were more typical of tumors with MSI than those with MSS in EC.
Tumors with MSI are characterized by sequence alterations in microsatellites and can accumulate thousands of mutations, which results in tumor heterogeneity (30-32). At the molecular level, MSI tumors show substantive intratumor and intertumor heterogeneity in the genomic influence (30). At the cellular and histological level, 3 histomorphological features (more than 2 tumor-infiltrating lymphocytes per high-power field, differentiation of mucinous, and lack of dirty necrosis) are associated with MSI in colorectal cancer (32), suggesting that the morphology of the tumor with MSI is more complex. Likewise, our study tried to identify the complex morphology of the tumor with MSI at the macro level. We found that the tumor with MSI had significantly high eccentricity, reflecting the macroscopical isotropy. However, the significant difference of eccentricity only remained in stages I–II, presumably because of the small sample size. Moreover, we found that the tumor with MSI often showed a regular shape, which seemed quite the opposite of the tumor heterogeneity. This may be related to the structure of the endometrial cavity limiting the growth of the tumor in some conditions. Nonetheless, shape features based on MRI may offer more clarity in assessing MSI status in EC.
To the best of our knowledge, there have only been several studies assessing MSI status in EC based on radiological images, using traditional methods or radiomics methods (14-16). As for traditional methods, EC tumors with MSI had significantly lower signal intensities than those with MSS on CE images in a study with 71 patients (14). We also chose the CE-T1WI sequences to segment tumors. A similar analysis for assessing MSI status within 6 tumors with MSI and 6 tumors with MSS using reduced field of view diffusion sequences indicated that tumors with MSS had significantly higher ADC values than tumors with MSI (15). In contrast, our data set showed no statistical difference in ADC values between the MSI and MSS groups. This may be because the ADC values in our study are calculated from traditional DWI sequences. As for radiomics methods, a clinical-radiomic machine learning model to predict MSI based on computer tomography (CT) in EC and yielded an AUC value of 0.78 in the training set (n=102) and 0.78 in the test set (n=42) (16). The radiomic method has been widely used in other tumors (33-37), but it faces great challenges in the clinic due to limited interpretability. It is also challenging to delineate the boundaries of EC tumors on CE-CT images. Meanwhile, the simple shape features based on MRI images in our study to assess MSI status achieved an AUC value of 0.727.
Our study had some limitations. First, the sample size of our study was small (n=88) because of the limited EC cases with MSI status results. However, the sample size was good relative to previous similar MRI-based studies in EC (14,15). Second, we only focused on analyzing shape features, which might not have covered some other features strongly associated with MSI status. Although the shape features are interpretable, investigating other features may be our future research direction. Third, as this was a retrospective study at a single center, the results need to be verified by multicenter studies in the future.
Conclusions
In conclusion, quantitative and subjective shape features based on MRI may be potential markers for assessing MSI status in EC. These features may aid in the preliminary screening of EC patients with MSI.
Acknowledgments
Funding: This work was supported by the Key-Area Research and Development Program of Guangdong Province (No. 2021B0101420006), the National Science Fund for Distinguished Young Scholars (No. 81925023), the National Key R&D Program of China (No. 2021YFF1201003), the Guangdong Provincial Key Laboratory of Artificial Intelligence in Medical Image Analysis and Application (No. 2022B1212010011), the National Natural Science Foundation of China (Nos. 82071892, 82072090), and the High-Level Hospital Construction Project (Nos. DFJH201805, DFJHBF202105).
Footnote
Reporting Checklist: The authors have completed the TRIPOD reporting checklist. Available at https://qims.amegroups.com/article/view/10.21037/qims-22-77/rc
Conflicts of Interest: All authors have completed the ICMJE uniform disclosure form (available at https://qims.amegroups.com/article/view/10.21037/qims-22-77/coif). The authors have no conflicts of interest to declare.
Ethical Statement: The authors are accountable for all aspects of the work in ensuring that questions related to the accuracy or integrity of any part of the work are appropriately investigated and resolved. The study was conducted in accordance with the Declaration of Helsinki (as revised in 2013). The study was approved by the research Ethics Committee of Guangdong Provincial People’s Hospital and individual consent for this retrospective analysis was waived.
Open Access Statement: This is an Open Access article distributed in accordance with the Creative Commons Attribution-NonCommercial-NoDerivs 4.0 International License (CC BY-NC-ND 4.0), which permits the non-commercial replication and distribution of the article with the strict proviso that no changes or edits are made and the original work is properly cited (including links to both the formal publication through the relevant DOI and the license). See: https://creativecommons.org/licenses/by-nc-nd/4.0/.
References
- Sung H, Ferlay J, Siegel RL, Laversanne M, Soerjomataram I, Jemal A, Bray F. Global Cancer Statistics 2020: GLOBOCAN Estimates of Incidence and Mortality Worldwide for 36 Cancers in 185 Countries. CA Cancer J Clin 2021;71:209-49. [Crossref] [PubMed]
- Bokhman JV. Two pathogenetic types of endometrial carcinoma. Gynecol Oncol 1983;15:10-7. [Crossref] [PubMed]
- Murali R, Soslow RA, Weigelt B. Classification of endometrial carcinoma: more than two types. Lancet Oncol 2014;15:e268-78. [Crossref] [PubMed]
- Zannoni GF, Vellone VG, Arena V, Prisco MG, Scambia G, Carbone A, Gallo D. Does high-grade endometrioid carcinoma (grade 3 FIGO) belong to type I or type II endometrial cancer? A clinical-pathological and immunohistochemical study. Virchows Arch 2010;457:27-34. [Crossref] [PubMed]
- Bae HS, Kim H, Young Kwon S, Kim KR, Song JY, Kim I. Should endometrial clear cell carcinoma be classified as Type II endometrial carcinoma? Int J Gynecol Pathol 2015;34:74-84. [Crossref] [PubMed]
- Hause RJ, Pritchard CC, Shendure J, Salipante SJ. Classification and characterization of microsatellite instability across 18 cancer types. Nat Med 2016;22:1342-50. [Crossref] [PubMed]
- Abu-Rustum NR, Yashar CM, Bradley K, Campos SM, Chino J, Chon HS, et al. NCCN Guidelines® Insights: Uterine Neoplasms, Version 3.2021. J Natl Compr Canc Netw 2021;19:888-95. [Crossref] [PubMed]
- Palomaki GE, McClain MR, Melillo S, Hampel HL, Thibodeau SN. EGAPP supplementary evidence review: DNA testing strategies aimed at reducing morbidity and mortality from Lynch syndrome. Genet Med 2009;11:42-65. [Crossref] [PubMed]
- Usha L, Dewdney SB, Buckingham LE. Tumor Screening and DNA Testing in the Diagnosis of Lynch Syndrome. JAMA 2016;316:93-4. [Crossref] [PubMed]
- Eriksson J, Amonkar M, Al-Jassar G, Lambert J, Malmenäs M, Chase M, Sun L, Kollmar L, Vichnin M. Mismatch Repair/Microsatellite Instability Testing Practices among US Physicians Treating Patients with Advanced/Metastatic Colorectal Cancer. J Clin Med 2019;8:558. [Crossref] [PubMed]
- Fan S, Li X, Cui X, Zheng L, Ren X, Ma W, Ye Z. Computed Tomography-Based Radiomic Features Could Potentially Predict Microsatellite Instability Status in Stage II Colorectal Cancer: A Preliminary Study. Acad Radiol 2019;26:1633-40. [Crossref] [PubMed]
- Li Z, Dai H, Liu Y, Pan F, Yang Y, Zhang M. Radiomics Analysis of Multi-Sequence MR Images For Predicting Microsatellite Instability Status Preoperatively in Rectal Cancer. Front Oncol 2021;11:697497. [Crossref] [PubMed]
- Zhang W, Huang Z, Zhao J, He D, Li M, Yin H, Tian S, Zhang H, Song B. Development and validation of magnetic resonance imaging-based radiomics models for preoperative prediction of microsatellite instability in rectal cancer. Ann Transl Med 2021;9:134. [Crossref] [PubMed]
- Ahmed M, Al-Khafaji JF, Class CA, Wei W, Ramalingam P, Wakkaa H, Soliman PT, Frumovitz M, Iyer RB, Bhosale PR. Can MRI help assess aggressiveness of endometrial cancer? Clin Radiol 2018;73:833.e11-8. [Crossref] [PubMed]
- Bhosale P, Ramalingam P, Ma J, Iyer R, Soliman P, Frumovitz M, Kundra V. Can reduced field-of-view diffusion sequence help assess microsatellite instability in FIGO stage 1 endometrial cancer? J Magn Reson Imaging 2017;45:1216-24. [Crossref] [PubMed]
- Veeraraghavan H, Friedman CF, DeLair DF, Ninčević J, Himoto Y, Bruni SG, et al. Machine learning-based prediction of microsatellite instability and high tumor mutation burden from contrast-enhanced computed tomography in endometrial cancers. Sci Rep 2020;10:17769. [Crossref] [PubMed]
- Pena E, Ojiaku M, Inacio JR, Gupta A, Macdonald DB, Shabana W, Seely JM, Rybicki FJ, Dennie C, Thornhill RE. Can CT and MR Shape and Textural Features Differentiate Benign Versus Malignant Pleural Lesions? Acad Radiol 2017;24:1277-87. [Crossref] [PubMed]
- Bogowicz M, Riesterer O, Ikenberg K, Stieb S, Moch H, Studer G, Guckenberger M, Tanadini-Lang S. Computed Tomography Radiomics Predicts HPV Status and Local Tumor Control After Definitive Radiochemotherapy in Head and Neck Squamous Cell Carcinoma. Int J Radiat Oncol Biol Phys 2017;99:921-8. [Crossref] [PubMed]
- Todo Y, Watari H, Okamoto K, Hareyama H, Minobe S, Kato H, Sakuragi N. Tumor volume successively reflects the state of disease progression in endometrial cancer. Gynecol Oncol 2013;129:472-7. [Crossref] [PubMed]
- Ytre-Hauge S, Husby JA, Magnussen IJ, Werner HM, Salvesen ØO, Bjørge L, Trovik J, Stefansson IM, Salvesen HB, Haldorsen IS. Preoperative tumor size at MRI predicts deep myometrial invasion, lymph node metastases, and patient outcome in endometrial carcinomas. Int J Gynecol Cancer 2015;25:459-66. [Crossref] [PubMed]
- Siegel RL, Miller KD, Fuchs HE, Jemal A. Cancer statistics, 2022. CA Cancer J Clin 2022;72:7-33. [Crossref] [PubMed]
- McConechy MK, Talhouk A, Li-Chang HH, Leung S, Huntsman DG, Gilks CB, McAlpine JN. Detection of DNA mismatch repair (MMR) deficiencies by immunohistochemistry can effectively diagnose the microsatellite instability (MSI) phenotype in endometrial carcinomas. Gynecol Oncol 2015;137:306-10. [Crossref] [PubMed]
- Kinkel K, Forstner R, Danza FM, Oleaga L, Cunha TM, Bergman A, Barentsz JO, Balleyguier C, Brkljacic B, Spencer JAEuropean Society of Urogenital Imaging. Staging of endometrial cancer with MRI: guidelines of the European Society of Urogenital Imaging. Eur Radiol 2009;19:1565-74. [Crossref] [PubMed]
- Yushkevich PA, Piven J, Hazlett HC, Smith RG, Ho S, Gee JC, Gerig G. User-guided 3D active contour segmentation of anatomical structures: significantly improved efficiency and reliability. Neuroimage 2006;31:1116-28. [Crossref] [PubMed]
- Fasmer KE, Hodneland E, Dybvik JA, Wagner-Larsen K, Trovik J, Salvesen Ø, Krakstad C, Haldorsen IHS. Whole-Volume Tumor MRI Radiomics for Prognostic Modeling in Endometrial Cancer. J Magn Reson Imaging 2021;53:928-37. [Crossref] [PubMed]
- Luo Y, Mei D, Gong J, Zuo M, Guo X. Multiparametric MRI-Based Radiomics Nomogram for Predicting Lymphovascular Space Invasion in Endometrial Carcinoma. J Magn Reson Imaging 2020;52:1257-62. [Crossref] [PubMed]
- Stanzione A, Cuocolo R, Del Grosso R, Nardiello A, Romeo V, Travaglino A, Raffone A, Bifulco G, Zullo F, Insabato L, Maurea S, Mainenti PP. Deep Myometrial Infiltration of Endometrial Cancer on MRI: A Radiomics-Powered Machine Learning Pilot Study. Acad Radiol 2021;28:737-44. [Crossref] [PubMed]
- Haldorsen IS, Salvesen HB. What Is the Best Preoperative Imaging for Endometrial Cancer? Curr Oncol Rep 2016;18:25. [Crossref] [PubMed]
- Bi Q, Chen Y, Wu K, Wang J, Zhao Y, Wang B, Du J. The Diagnostic Value of MRI for Preoperative Staging in Patients with Endometrial Cancer: A Meta-Analysis. Acad Radiol 2020;27:960-8. [Crossref] [PubMed]
- Cortes-Ciriano I, Lee S, Park WY, Kim TM, Park PJ. A molecular portrait of microsatellite instability across multiple cancers. Nat Commun 2017;8:15180. [Crossref] [PubMed]
- Mandal R, Samstein RM, Lee KW, Havel JJ, Wang H, Krishna C, et al. Genetic diversity of tumors with mismatch repair deficiency influences anti-PD-1 immunotherapy response. Science 2019;364:485-91. [Crossref] [PubMed]
- Yamashita R, Long J, Longacre T, Peng L, Berry G, Martin B, Higgins J, Rubin DL, Shen J. Deep learning model for the prediction of microsatellite instability in colorectal cancer: a diagnostic study. Lancet Oncol 2021;22:132-41. [Crossref] [PubMed]
- Li M, Li X, Guo Y, Miao Z, Liu X, Guo S, Zhang H. Development and assessment of an individualized nomogram to predict colorectal cancer liver metastases. Quant Imaging Med Surg 2020;10:397-414. [Crossref] [PubMed]
- Yang X, Wu L, Zhao K, Ye W, Liu W, Wang Y, Li J, Li H, Huang X, Zhang W, Huang Y, Chen X, Yao S, Liu Z, Liang C. Evaluation of human epidermal growth factor receptor 2 status of breast cancer using preoperative multidetector computed tomography with deep learning and handcrafted radiomics features. Chin J Cancer Res 2020;32:175-85. [Crossref] [PubMed]
- Li S, Luo T, Ding C, Huang Q, Guan Z, Zhang H. Detailed identification of epidermal growth factor receptor mutations in lung adenocarcinoma: Combining radiomics with machine learning. Med Phys 2020;47:3458-66. [Crossref] [PubMed]
- Dong D, Fang MJ, Tang L, Shan XH, Gao JB, Giganti F, Wang RP, Chen X, Wang XX, Palumbo D, Fu J, Li WC, Li J, Zhong LZ, De Cobelli F, Ji JF, Liu ZY, Tian J. Deep learning radiomic nomogram can predict the number of lymph node metastasis in locally advanced gastric cancer: an international multicenter study. Ann Oncol 2020;31:912-20. [Crossref] [PubMed]
- Ou J, Wu L, Li R, Wu CQ, Liu J, Chen TW, Zhang XM, Tang S, Wu YP, Yang LQ, Tan BG, Lu FL. CT radiomics features to predict lymph node metastasis in advanced esophageal squamous cell carcinoma and to discriminate between regional and non-regional lymph node metastasis: a case control study. Quant Imaging Med Surg 2021;11:628-40. [Crossref] [PubMed]