Associations between cerebral blood flow and progression of white matter hyperintensity in community-dwelling adults: a longitudinal cohort study
Introduction
White matter hyperintensity (WMH), as a subtype of cerebral small vessel disease (CSVD), is prevalent in elderly individuals (1). Pathologically, WMHs are mainly characterized by demyelination, pale myelin, oligodendrocyte apoptosis, and vacuolization (2,3). It has been shown that WMHs are related to various geriatric disorders, such as cerebrovascular diseases, dementia, cardiovascular diseases, cognitive impairment, psychiatric disorders, and systemic immunological diseases (4,5). Investigators have found that WMHs in different locations have different functional, histopathological, and etiological features with respect to lateral ventricles (6,7). A better understanding of the pathophysiological mechanism of WMHs is important for the prevention of WMH-related adverse clinical outcomes.
Several studies have demonstrated that ischemia may play a key role in the pathogenesis of WMH (8,9). Cerebral ischemia is characterized by a decline in cerebral blood flow (CBF), and ischemic lesions will occur after the CBF decreases to a threshold for a certain period of time. However, whether CBF reduction precedes or follows WMH progression remains controversial. Previous cross-sectional studies have reported that a higher WMH load is associated with a lower CBF of the gray matter (GM) or cortex (10,11). However, a meta-analysis showed that this association was dampened after removing non-age matched cases and those with dementia (9,12). Increasing evidence has shown that CBF dynamically changes with age, even in normal individuals (13,14), which may confound the relationship between CBF and WMH. Although some studies have evaluated the longitudinal association between CBF and WMH, their findings varied, and baseline global CBF has not been associated with the progression of WMH in older participants (15). However, other studies have reported that after differentiating the locations of WMHs, regional associations of lower CBF with periventricular WMH (PVWMH) progression but not deep WMH (DWMH) progression were found in elderly patients (16,17). Such associations between CBF reduction and WMH progression across the adult lifespan have not been studied. Several studies have found that CBF within WMH is lower than that in normal appearing white matter (NAWM) (18,19), while the differences between CBF changes with age and WMH progression are still unclear.
Traditionally, CBF is usually measured by positron emission tomography (PET), CT perfusion (CTP), and dynamic susceptibility-contrast (DSC) magnetic resonance imaging (MRI). However, these imaging modalities either include exposure to ionizing radiation or require intravenous administration of tracer or contrast agent, which limits their application to individuals with special conditions, such as renal dysfunction (20,21). Pseudo-continuous arterial spin-labeling (pCASL) MRI with optimized parameters is capable of noninvasively measuring CBF using labeled arterial blood as an endogenous tracer (22,23).
In this study, we aimed to investigate the association between CBF and the progression of WMH using pCASL imaging in community-based, asymptomatic adults across the lifespan. We also evaluated the rate of longitudinal change in CBF of GM, NAWM, and WMH as well as the progression of WMH after 2 years of follow-up to determine their causal relationships. We present the following article in accordance with the STROBE reporting checklist (available at https://qims.amegroups.com/article/view/10.21037/qims-22-141/rc).
Methods
Study sample
The participants were recruited from an ongoing, community-based, longitudinal and observational cohort study of Cerebrovascular Accident Monitoring, Epidemiology and caRe quAlity system (CAMERA) (24). The primary objective of the CAMERA study was to determine the risk factors for the progression of subclinical neurovascular diseases and future ischemic cerebrovascular events in asymptomatic adults. The inclusion criteria were as follows: (I) over 30 years old, and (II) no cerebrovascular symptoms within the previous 6 months. Participants who had contraindications to MR examination were excluded. All included participants underwent brain anatomical MRI at baseline. As a substudy, participants who enrolled in the CAMERA study from April 2016 to July 2019 also underwent pCASL perfusion imaging of the brain at baseline. Brain anatomical and perfusion MR scans were also conducted in the 24th month of the follow-up study.
A total of 237 participants completed both brain anatomical and perfusion MR scans at baseline. Of 237 participants, only 88 finished the two-year follow-up MR scan, and nearly two-thirds of participants were lost to follow-up from 2020 due to the coronavirus disease of 2019 (COVID-19) epidemic. Of the 237 included participants, 8 were excluded because of poor image quality at baseline (n=7) and in follow-up (n=1) MR examinations. Finally, a total of 229 participants (mean age 57.32±12.63 years, range 31 to 87 years; 94 males) were included in the final statistical analysis. Of the 229 participants, 84 (mean age 54.06±11.92 years, range 31 to 81 years; 41 males) completed both baseline and follow-up visits with a mean time interval of 2.77±0.44 years (range: 1.75 to 3.33 years). The flowchart of participants is shown in Figure 1.
Baseline demographic and clinical characteristics, including age, gender, systolic blood pressure (SBP), diastolic blood pressure (DBP), high-density lipoprotein cholesterol (HDL-C), low-density lipoprotein cholesterol (LDL-C), total cholesterol (TC), triglycerides (TG), body mass index (BMI), and history of cardiovascular disease (CVD), were collected from clinical records. The vascular risk score (VRS; 0 = none, 5 = worst) of each individual was calculated by summing 5 dichotomous variables reflecting obesity (BMI ≥30), smoking, history of hypertension, hypercholesterolemia, and diabetes (25).
The baseline demographic and clinical characteristics of this study population are summarized in Table 1. Participants who were lost to follow-up were found to have older age (59.21±12.68 vs. 54.06±11.92 years; P=0.003), lower DBP (75.82±9.77 vs. 78.68±9.31 mmHg; P=0.031), and higher LDL-C [3.20, interquartile range (IQR): 2.57–3.78 mmol/L vs. 2.97, IQR: 2.42 to 3.34 mmol/L; P=0.022] than those who completed the follow-up study. No significant differences were found in other clinical characteristics or WMH measurements between participants who completed the follow-up study and those who were lost to follow-up (all P>0.05).
Table 1
Characteristics | All participants (n=229) | Participants with or without follow-up | ||
---|---|---|---|---|
Baseline and follow-up (n=84) | Baseline only (n=145) | P value | ||
Age, years | 57.32±12.63 | 54.06±11.92 | 59.21±12.68 | 0.003 |
Gender, male | 94 (41%) | 41 (48.8%) | 53 (36.6%) | 0.070 |
BMI, kg/m2 | 24.2 (22.2–26.0) | 24.2 (22.1–25.8) | 24.2 (22.2–26.1) | 0.733 |
Hypertension | 71 (31%) | 27 (32.1%) | 44 (30.3%) | 0.769 |
SBP, mmHg | 127.15±17.37 | 127.01±16.49 | 127.24±17.92 | 0.923 |
DBP, mmHg | 76.87±9.68 | 78.68±9.31 | 75.82±9.77 | 0.031 |
Hyperlipidemia | 89 (38.9%) | 27 (32.1%) | 62 (42.8%) | 0.123 |
HDL-C, mmol/L | 1.39 (1.14–1.67) | 1.33 (1.09–1.67) | 1.41 (1.16–1.68) | 0.188 |
LDL-C, mmol/L | 3.13 (2.50–3.62) | 2.97 (2.42–3.34) | 3.20 (2.57–3.78) | 0.022 |
TC, mmol/L | 4.93 (4.30–5.50) | 4.74 (4.28–5.39) | 5.03 (4.37–5.58) | 0.061 |
TG, mmol/L | 1.24 (0.95–1.78) | 1.21 (0.91–1.80) | 1.24 (0.98–1.74) | 0.923 |
Smoking | 25 (10.9%) | 13 (15.5%) | 22 (15.2%) | 0.999 |
Diabetes | 30 (13.1%) | 12 (14.3%) | 18 (12.4%) | 0.689 |
History of CVD | 86 (37.6%) | 4 (4.8%) | 12 (8.3%) | 0.423 |
Vascular risk score | 1.03±0.88 | 0.96±0.86 | 1.06±0.90 | 0.970 |
Fazekas scale | 1.65±1.27 | 1.56±1.24 | 1.70±1.29 | 0.928 |
WMH volume, mL | 0.83 (0.05–2.43) | 0.52 (0.01–2.04) | 1.03 (0.07–2.6) | 0.132 |
PVWMH volume, mL | 0.48 (0–1.36) | 0.38 (0–1.06) | 0.54 (0–1.52) | 0.315 |
DWMH volume, mL | 0.18 (0–1.14) | 0.11 (0–0.74) | 0.21 (0–1.18) | 0.181 |
Values are provided as mean ± standard deviation, median (interquartile range) or numbers (%) for each variable. BMI, body mass index; SBP, systolic blood pressure; DBP, diastolic blood pressure; HDL-C, high-density lipoprotein cholesterol; LDL-C, low-density lipoprotein cholesterol; TC, total cholesterol; TG, triglycerides; CVD, cardiovascular disease; WMH, white matter hyperintensity; PVWMH, periventricular white matter hyperintensity; DWMH, deep white matter hyperintensity.
The study was conducted in accordance with the Declaration of Helsinki (as revised in 2013). The study protocol was approved by the institutional review board of Beijing Tiantan hospital, and written consent form was provided by all participants at each visit.
MRI protocol
Brain MRI was performed on a 3.0 T MR scanner (Ingenia CX; Philips Healthcare, Best, The Netherlands) equipped with a 32-channel head coil. The brain was imaged by T1-weighted (T1W), T2-weighted fluid attenuated inversion recovery (T2-FLAIR), and pCASL sequences. The parameters used for T1W were repeat time (TR)/echo time (TE) 24/5 ms, field of view (FOV) 256×192×128 mm3, spatial resolution 0.6×0.6 mm2, sensitivity encoding acceleration (SENSE) factor 2, and scan time 4 min 8 s. The parameters used for T2-FLAIR were TR/inversion time (TI) 7,000/2,200 ms, TE 140 ms, FOV 230×230×133 mm3, spatial resolution 0.9×0.9 mm2, SENSE factor 2.1, and scan time 2 min 48 s. The parameters used for pCASL were single shot echo planar imaging, TR/TE 4,016/12 ms, FOV 240×240×132 mm3, spatial resolution 3×3 mm2, SENSE factor 3, label duration 1,800 ms, post labeling delay 1,800 ms, number of repetitions 30, background suppression pulse 1,820 and 3,155 ms, and scan time 4 min 31 s. After pCASL imaging, a proton density-weighted reference scan (M0) was performed with identical imaging parameters except for no arterial spin labeling (ASL) and TR 6,000 ms. The slice thicknesses for T1W, T2-FLAIR, and pCASL were 2 mm, 5.5 mm, and 6 mm, respectively. All participants were reminded not to drink coffee/tea on the day before the MR examination. The participants were trained to remain still, were scanned in a calm state, and avoided swallowing during the MR scan to reduce motion artifacts. Quality control was based on visual assessment. Poor image quality included the following conditions: (I) contrast-based: unclear or invisible contrast in GM and WM; and (II) artifact-based: severe head motion, signal drop, geometric distortion, or macrovascular bright spots. A repeat scan was performed when the image quality was poor.
Follow-up brain MR imaging was conducted with the identical imaging protocol on the same MR scanner at the 24th month after the baseline study.
Image analysis
The perfusion data acquired by pCASL were analyzed utilizing established procedures (22) with customized MATrix LABoratory (MATLAB) scripts (Mathworks Inc., Natick, MA, USA). Briefly, the differences between the averaged label and control images were calculated, and the delay times in different imaging slices were corrected. The CBF (unit: mL/100 g/min) was calculated using the above difference maps and proton density-weighted images (M0) after motion correction.
Tissue segmentation was conducted on T1W images to derive binary GM and WM masks using the Oxford Centre for Functional Magnetic Resonance Imaging of the Brain (FMRIB) Software Library v. 6.0 (FSL; Oxford, UK), including a brain extraction tool (BET) for skull strips and FMRIB's Automated Segmentation Tool (FAST) for probabilistic and partial volume tissue segmentation (26,27). GM and WM masks were then generated with a probabilistic threshold of 0.9 (28).
The lesions of WMH on T2-FLAIR images were independently scored by two experienced raters (>3 years of experience in neuroimaging) blinded to the clinical data, and disagreements were resolved through discussion. By judging the different types of WMHs, PVWMH and DWMH were both graded from 0 to 3, based on the Fazekas criteria of “continuity to ventricle” (2). The WMH score was defined as the sum of PVWMH and DWMH scores. Quantitative evaluation of WMHs was conducted by manually segmenting WMH regions using the Computer-Aided System for CArdiovascular Disease Evaluation software v. 1.9 (CASCADE; University of Washington, Seattle, WA, USA). The PVWMH was defined as lesions adjacent to or within 1 cm of the lateral ventricles. The DWMH was defined as lesions located in deep WM tracts that may have adjoined periventricular lesions (29). The volumes of WMH were log transformed, explicitly, log(WMH), to address the nonnormal distribution. Coregistrations of perfusion maps, probabilistic maps, and binary masks of GM and WM to the T2-FLAIR images were performed in Statistical Parametric Software v. 12 (SPM12; Wellcome Trust Centre for Neuroimaging, Institute of Neurology, University College London, London, UK). We then generated NAWM masks by excluding WMH masks from the WM masks. The workflow for image processing is illustrated in Figure 2.
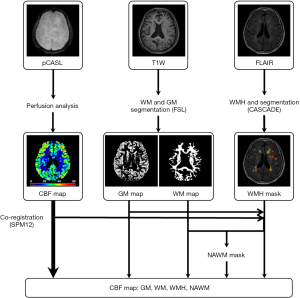
Statistical analysis
Continuous variables with a normal distribution were described as the mean and standard deviation (SD), variables with a nonnormal distribution were described as the median and IQR, and categorical variables were described as counts and percentages. Statistical analysis was performed using the software SPSS26.0 software (IBM Corp., Armonk, NY, USA). Two-tailed P-values less than 0.05 were considered statistically significant.
At baseline, linear regression was used to estimate the associations between CBF and age. Regional dependence of WMH CBF was analyzed using repeated-measure Analysis of Variance (ANOVA) testing with post hoc Bonferroni correction, which included the CBF of NAWM, PVWMH, and DWMH as within-participant variables. In addition, the CBF was also compared among different clinical risk factors for the VRS using one-way ANOVA. An independent t-test was used to compare the age distributions between participants with none-to-mild WMH (Fazekas scale ≤2) and those with moderate-to-severe WMH (Fazekas scale >2). Analyses of linear regression with age and one-way ANOVA on VRS were also conducted on log-transformed volume of WMH, explicitly, log(WMH), because of its non-normal distribution.
To investigate the associations between CBF and WMH at baseline, binary logistic regression was firstly utilized to determine the association of CBF with the presence of WMH as well as the severity of WMH. Age and gender were additionally adjusted in the logistic regression models. Among participants with WMH, linear regression was implemented to investigate the associations between CBF and log(WMH) at baseline (model 1). Analyses were adjusted for age and gender (model 2) and additionally adjusted for VRS and history of CVD (model 3). Analyses were also conducted on PVWMH and DWMH separately.
During follow-up, a linear mixed effect (LME) model was used to assess the longitudinal changes in CBF of GM or NAWM with random intercepts at the subject level. Age at baseline scan, gender, and follow-up interval entered as fixed-effect independent variables. To examine whether the longitudinal change was age-dependent, an age-by-time interaction term was additionally added to the LME models. For participants with WMH at baseline, the same LME models were also conducted on the volume of WMH and CBF within WM lesions to evaluate their age-related changes.
We evaluated the longitudinal associations between annual changes in CBF and WMH. ΔCBF was defined as [(CBF2 − CBF1)/time interval], and Δlog(WMH) was defined as {[log(WMH2) − log(WMH1)]/time interval}. First, whether ΔCBF differed from the progression of WMH was evaluated using LME models where the progress of the Fazekas scale was added as a fixed effect together with age at baseline, gender, and time from the baseline scan. To quantitatively investigate the relationship between the annual changes in CBF and log(WMH), associations between ΔCBF and Δlog(WMH) were assessed using linear regression-adjusted baseline values of both CBF and log(WMH). Baseline values of both CBF and log(WMH) were adjusted on the linear regression analysis. Prospective associations of baseline CBF with the progression of WMH volume during follow-up were estimated with linear regression, and analyses were adjusted for log(WMH) at baseline. Meanwhile, prospective associations of the baseline volume of WMH with changes in CBF during follow-up were also estimated and adjusted for CBF at baseline. To determine whether the above associations were independent of potential confounding factors, the aforementioned linear regression analyses were adjusted for age and gender (model 2) and additionally adjusted for VRS and history of CVD (model 3).
To eliminate any residual partial volume effects, the above models also included GM probability and WM probability as covariates, so that the observed effects on the CBF were not due to the reduced GM or WM fraction.
Results
Age-specific characteristics of CBF and WMH at baseline
At baseline, the mean value of GM CBF was 40.71±7.94 mL/100 g/min. Lower GM CBF was found to be significantly associated with increasing age (coefficient =−0.18, 95% CI: −0.26 to −0.11; P<0.001) (Figure 3A). Of all 229 participants, 174 (76.0%) were found to have PVWMH or DWMH at baseline. The mean values of NAWM CBF and WMH CBF were 26.83±5.29 mL/100 g/min and 17.65±5.37 mL/100 g/min at baseline, respectively. Neither NAWM CBF (P=0.776) nor WMH CBF (P=0.917) was significantly associated with age (Figure 3B,3C). Specifically, repeated ANOVA analysis showed that there was a strong main effect {F[2,238] = 420.82; P<0.001} for the locations of WM on CBF among NAWM, PVWMH (14.24±3.90 mL/100 g/min), and DWMH (20.82±6.03 mL/100 g/min). Multiple comparisons with Bonferroni correction revealed that NAWM CBF was significantly greater than PVWMH CBF (P<0.001) and DWMH CBF (P<0.001), and DWMH CBF was significantly greater than PVWMH CBF (P<0.001). Among the VRS groups, a significant difference was found in GM CBF (P=0.004) but not in NAWM CBF (P=0.233) or WMH CBF (P=0.953).
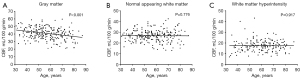
The distribution of the Fazekas scales of this study population is presented in Figure S1. Comparison of none-to-mild WMH (i.e., Fazekas scale ≤2; n=173) and moderate-to-severe WMH (i.e., Fazekas scale >2; n=56) showed a significant difference between the two levels of severity according to age (54.40±11.77 vs. 66.32±10.88 years, respectively; P<0.001). Among participants with WMH, a greater log(WMH) was significantly associated with increasing age (coefficient =0.024, 95% CI: 0.018 to 0.030; Figure 4A). Such associations were also found between PVWMH and age (coefficient =0.026, 95% CI: 0.012 to 0.041; P<0.001; Figure 4B) and between DWMH and age (coefficient =0.043, 95% CI: 0.031 to 0.056; P<0.001; Figure 4C). One-way ANOVA analyses showed no significant difference in total WMH volume among the VRS groups (P=0.134). However, patients with a higher VRS showed significantly greater DWMH volume (P=0.011).
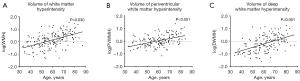
Association between cerebral perfusion and WMH at baseline
Logistic regression analysis showed that GM CBF was negatively associated with the presence of WMH [odds ratio (OR): 0.95; 95% CI: 0.91 to 0.99; P=0.016] at baseline. However, this association was no longer statistically significant after adjusting for age and gender (P=0.284). In the comparison analysis, no significant difference in GM CBF was found between participants with none-to-mild WMH and those with moderate-to-severe WMH (P=0.216). No significant associations were found between NAWM CBF and the presence and severity of WMH (all P>0.05).
In the linear regression for analyzing the associations between CBF and log(WMH) (Table 2), there was a decreasing trend of GM CBF, and the increase in WMH volume did not reach statistical significance (Model 1: B=−0.010, 95% CI: −0.020 to 0.001; P=0.063) after partial volume correction. The associations were not statistically significant after adjusting for age and gender (Model 2: P=0.362) and further adjusting for VRS and history of cardiovascular diseases as covariates (Model 3: P=0.271). There were no significant associations between NAWM CBF and WMH volume (P=0.598).
Table 2
Outcome | Predictor | Model 1† | Model 2‡ | Model 3§ | ||||||||
---|---|---|---|---|---|---|---|---|---|---|---|---|
B | 95% CI | P value | B | 95% CI | P value | B | 95% CI | P value | ||||
log(WMH) | GM CBF | −0.010 | −0.020, 0.001 | 0.063 | −0.005 | −0.015, 0.006 | 0.362 | −0.006 | −0.016, 0.005 | 0.271 | ||
log(WMH) | NAWM CBF | 0.004 | −0.012, 0.021 | 0.598 | 0.004 | −0.012. 0.020 | 0.610 | 0.003 | −0.012, 0.019 | 0.677 | ||
log(WMH) | WMH CBF | −0.016 | −0.031, −0.001 | 0.032 | −0.017 | −0.031, −0.003 | 0.016 | −0.019 | −0.033, −0.005 | 0.009 | ||
log(PVWMH) | PVWMH CBF | 0.023 | 0.004, 0.042 | 0.015 | 0.024 | 0.006, 0.042 | 0.009 | 0.023 | 0.005, 0.041 | 0.012 | ||
log(DWMH) | DWMH CBF | −0.015 | −0.031, 0.001 | 0.059 | −0.009 | −0.024, 0.006 | 0.221 | −0.010 | −0.025, 0.004 | 0.172 |
†, Model 1 only added baseline CBF as independent variables. GM probability and WM probability was additional adjusted for partial volume correction. ‡, Model 2 was additional adjusted for age and gender. §, Model 3 was further additional adjusted for vascular risk factors (i.e., obesity, smoking, history of hypertension, hypercholesterolemia and diabetes) and history of cardiovascular diseases. CI, confidence interval; CBF, cerebral blood flow; GM, gray matter; NAWM, normal appearing white matter; WMH, white matter hyperintensity; PVWMH, periventricular white matter hyperintensity; DWMH, deep white matter hyperintensity.
Within the WMH lesions, there was a significant association between CBF and WMH volume (Model 1: coefficient =−0.016, 95% CI: −0.031 to −0.001; P=0.017), and this association remained statistically significant after adjusting for confounding factors (Model 2: coefficient =−0.017, 95% CI: −0.031 to −0.003; P=0.016; Model 3: coefficient =−0.019, 95% CI: −0.033 to −0.005; P=0.009). Notably, the volumes of PVWMH and DWMH showed opposite effects on the CBF of the corresponding lesions. There was a significantly positive association between the volume of PVWMH and CBF within the lesions (coefficient =0.023, 95% CI: 0.004 to 0.042; P=0.015), and a decreasing trend was observed in CBF within lesions with the increase in the volume of DWMH (coefficient =−0.015, 95% CI: −0.031 to 0.001; P=0.059).
Longitudinal changes of cerebral perfusion and WMH
Of the 84 participants who completed both baseline and follow-up MR examinations, there was a significant decline in GM CBF (3.24 mL/100 g/min per year, 95% CI: −3.88 to −2.61; P<0.001) and NAWM CBF (1.76 mL/100 g/min per year, 95% CI: −2.22 to −1.30; P<0.001) during follow-up (Figure 5). The annual decline rate in CBF did not differ by baseline age (i.e., age-by-time interaction) in either GM (P=0.998) or NAWM (P=0.086). There was no significant difference in the annual change in CBF on the increase of the Fazekas scale (GM: P=0.632; NAWM: P=0.557).
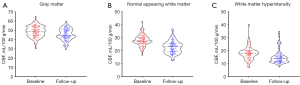
Of 84 participants with both baseline and follow-up MR examinations, 74 (88.1%) had WMH at follow-up and 25 (29.8%) were found to have progression of WMH during follow-up (Figure S1). Of the 25 participants with WMH progression, 10 (40%) had PVWMH progression, 10 (40%) had DWMH progression, and 5 (20%) had progression in both types of WMH. However, there was no significant difference in age (P=0.627) between participants with and without WMH progression. For participants with WMH at baseline, a linear mixed effect model showed that WMH volume, namely, log(WMH), significantly increased with age (coefficient =0.06, 95% CI: 0.04 to 0.07; P<0.001), but no age-by-time interaction effect was found (P=0.108). Similar findings were also found in PVWMH (age effect: coefficient =0.05, 95% CI: 0.03 to 0.07; P<0.001; age-by-time interaction effect: P=0.746) and DWMH (age effect: coefficient =0.06, 95% CI: 0.04 to 0.08; P<0.001; age-by-time interaction effect: P=0.341). Moreover, within the WMH lesions, a significant decline was found in WMH CBF (0.89 mL/100 g/min per year, 95% CI: −1.40 to −0.38; P=0.001) during follow-up. The age-by-time interaction of WMH CBF had a significantly negative effect on age (coefficient =−0.044, 95% CI: −0.084 to −0.003; P=0.034), indicating a greater rate of WMH CBF decline at older baseline ages.
The quantitative analysis results of Δlog(WMH) and ΔCBF with linear regression are shown in Table 3. There were no associations between annual changes in WMH volume and CBF of GM and NAWM (all P>0.05). Within the WMH lesions, significant associations between Δlog(WMH) and ΔWMH CBF were found before (Model 1: coefficient =−0.014, 95% CI: −0.025 to −0.003; P=0.017) and after (Model 2: coefficient =−0.015, 95% CI: −0.026 to −0.004; P=0.009; Model 3: B =−0.019, 95% CI: −0.031 to −0.007; P=0.002) adjusting for clinical characteristics. When separating the total volume of WMH into PVWMH and DWMH, for the annual decrease in CBF, there was an increasing trend in PVWMH volume (Model 1: coefficient =−0.26, 95% CI: −0.049 to −0.003; P=0.029; Model 2: coefficient =−0.24, 95% CI: −0.046 to −0.002; P=0.032; Model 3: coefficient =−0.23, 95% CI: −0.047 to 0.002; P=0.074), whereas this increasing trend was not found in DWMH volume (all P>0.05).
Table 3
Outcome | Predictor | Model 1† | Model 2‡ | Model 3§ | ||||||||
---|---|---|---|---|---|---|---|---|---|---|---|---|
B | 95% CI | P value | B | 95% CI | P value | B | 95% CI | P value | ||||
Δlog(WMH) | ΔGM CBF | −0.005 | −0.012, 0.002 | 0.136 | −0.004 | −0.011, 0.002 | 0.197 | −0.005 | −0.012, 0.002 | 0.139 | ||
Δlog(WMH) | ΔNAWM CBF | −0.007 | −0.017, 0.004 | 0.217 | −0.006 | −0.016, 0.005 | 0.275 | −0.008 | −0.019, 0.003 | 0.164 | ||
Δlog(WMH) | ΔWMH CBF | −0.014 | −0.025, −0.003 | 0.017 | −0.015 | −0.026, −0.004 | 0.009 | −0.019 | −0.031, −0.007 | 0.002 | ||
Δlog(PVWMH) | ΔPVWMH CBF | −0.026 | −0.049, −0.003 | 0.029 | −0.024 | −0.046, −0.002 | 0.032 | −0.023 | −0.047, 0.002 | 0.074 | ||
Δlog(DWMH) | ΔDWMH CBF | −0.004 | −0.020, 0.013 | 0.664 | −0.005 | −0.022, 0.012 | 0.542 | −0.012 | −0.030, 0.006 | 0.190 |
†, Model 1 only added baseline CBF as independent variables and was adjusted for baseline CBF, baseline log(WMH), GM probability and WM probability. ‡, Model 2 was additional adjusted for age and gender. §, Model 3 was further additional adjusted for vascular risk factors (i.e., obesity, smoking, history of hypertension, hypercholesterolemia and diabetes) and history of cardiovascular diseases. CI, confidence interval; CBF, cerebral blood flow; GM, gray matter; NAWM, normal appearing white matter; WMH, white matter hyperintensity; PVWMH, periventricular white matter hyperintensity; DWMH, deep white matter hyperintensity.
Baseline perfusion of GM, NAWM, and WMH was not significantly associated with the progression of WMHs, before and after adjusting for confounding factors (all P>0.05) (Table S1, supplementary appendix online).
Table 4 presents the association between baseline WMH volume and changes in perfusion of brain tissues. The per unit increase in log-WMH volume corresponded to 1.96 mL/100 g/min/year (95% CI: −3.25 to −0.67; P=0.004) of decline in GM CBF and 0.99 mL/100 g/min/year (95% CI: −1.66 to −0.31; P=0.005) of decline in NAWM, respectively. Further adjusting for clinical characteristics did not attenuate the estimates of the above effects. Moreover, baseline WMH volume was also significantly associated with changes in perfusion within WMHs (B=−1.01, 95% CI: −1.81 to −0.20; P=0.015), as shown in Table 4, even though the effects vanished when specifically separated into PVWMH and DWMH.
Table 4
Outcome | Predictor | Model 1† | Model 2‡ | Model 3§ | ||||||||
---|---|---|---|---|---|---|---|---|---|---|---|---|
B | 95% CI | P value | B | 95% CI | P value | B | 95% CI | P value | ||||
ΔGM CBF | log(WMH) | −1.96 | −3.25, −0.67 | 0.004 | −1.90 | −3.32, −0.47 | 0.010 | −2.14 | −3.60, −0.67 | 0.005 | ||
ΔNAWM CBF | log(WMH) | −0.99 | −1.66, −0.31 | 0.005 | −1.20 | −2.08, −0.31 | 0.009 | −1.31 | −2.18, −0.45 | 0.004 | ||
ΔWMH CBF | log(WMH) | −1.01 | −1.81, −0.20 | 0.015 | −1.22 | −2.14, −0.30 | 0.010 | −1.19 | −2.07, −0.32 | 0.008 | ||
ΔPVWMH CBF | log(PVWMH) | −0.48 | −1.13, 0.16 | 0.140 | −0.43 | −1.13, 0.27 | 0.219 | −0.51 | −1.20, 0.18 | 0.142 | ||
ΔDWMH CBF | log(DWMH) | −0.80 | −1.74, 0.14 | 0.093 | −0.40 | −1.49, 0.69 | 0.464 | −0.14 | −1.21, 0.93 | 0.795 |
†, Model 1 added baseline log(WMH) as independent variables and was adjusted for baseline CBF, GM probability and WM probability. ‡, Model 2 was additional adjusted for age and gender. §, Model 3 was further additional adjusted for vascular risk factors (i.e., obesity, smoking, history of hypertension, hypercholesterolemia and diabetes) and history of cardiovascular diseases. CI, confidence interval; CBF, cerebral blood flow; GM, gray matter; NAWM, normal appearing white matter; WMH, white matter hyperintensity; PVWMH, periventricular white matter hyperintensity; DWMH, deep white matter hyperintensity.
Discussion
This study investigated the cross-sectional and longitudinal associations between CBF and WMH in community-based, asymptomatic adults. We found that WMH burden was not directly associated with cortex perfusion at baseline due to the effects of age on both CBF and WMH. In contrast, baseline WMH volume could predict the reduction of perfusion during follow-up. We also found that blood flow changes within WMH were potential indicators for baseline and progression of WMH volume.
Associations between CBF and WMH
In the present study, we found that there was a decreasing trend of GM CBF with the increase of WMH burden. However, this association was attenuated after adjusting for age, gender, and vascular risk factors. It is believed that the pathogenesis or etiology of WMH is multifactorial (1). Previous studies have demonstrated a reduced CBF in patients with moderate-to-severe WMH compared to those with none-to-mild WMH (11,12,30). It has been shown that WMH is commonly seen in cognitively normal, older individuals (31). Consistent with previous studies, in the present study, greater WMH expansion with age was found. Meanwhile, we also found that CBF in GM and NAWM decreased with age at longitudinal rates of 3.24 mL/100 g/min per year and 1.76 mL/100 g/min per year, respectively. Such findings were in accord with previous reports that CBF decreases with aging (13,32,33). A systematic review and meta-analysis of WMH and CBF reported that negative associations between CBF and WMH were weakened after excluding dementia patients or non-age matched participants (12). Our findings of weakened association between GM CBF and WMH further impelled us to consider that the underlying associations might mostly depend on age or age-related risk factors, rather than a direct effect.
Our longitudinal data analyses showed no direct associations between WMH progression and CBF reductions, regardless of GM or WM. However, these longitudinal relationships have brought more controversy. ten Dam et al. found that a decline in CBF measured by phase-contrast imaging during 33 months of follow-up was significantly associated with an increase in the volume of PVWMH in 390 elderly participants with high vascular risk (16). Staffaroni et al. reported that longitudinal reductions in global CBF were associated with increasing WMH burden, adjusting for age, gender, and education in 136 older adults with 2.3-year follow-up (32). In contrast, our findings of the present study were consistent with the report of van der Veen et al., where changes in WMH during follow-up were not associated with parenchymal CBF in 575 patients with manifest arterial disease and a mean age of 57±10 years (34). Nylander et al. also reported that there were no significant associations between supratentorial CBF and changes in WMH volume in 252 participants between the ages of 75 and 80 years. However, their study only provided relative CBF values rather than absolute CBF values (15). Kraut et al. evaluated the regional CBF changes using resting H215O-PET between 38 patients with progressive WM abnormalities and 36 patients with stable WM ratings with an average of 7.7-year follow-up (35). In cases with progressive WMH, the CBF increased in the right inferior temporal gyrus, right anterior cingulate, and left superior temporal gyrus, whereas the CBF reduced in posterior regions, including the right inferior parietal lobule and right occipital pole, but the changes of CBF was not specifically associated with WMH changes. A potential reason for the above inconsistent findings might be due to the differences in study populations, such as age distribution. Apparently, previous studies have mostly focused on older participants, and WMH occurred frequently in such populations, whereas our study enrolled participants across the adult lifespan. Our findings may indicate that the progression of WMH burden and reduction in blood flow over time are not coupled, especially in the earlier pathological stages.
WMH burdens predict changes of CBF
In this study, we found that with more severe WMH at baseline, there was a faster decline in the CBF of GM and NAWM. Our findings are consistent with previous reports (34). In contrast, the values of CBF at baseline were not significantly associated with subsequent changes in WMH volume. There was a debate on the after-effects of hypoperfusion. Two studies reported that baseline global CBF was not associated with worse WMH during follow-up (15,34). In contrast, one study reported that low CBF in NAWM at baseline preceded the development of WMH (36). Another important concern was that none of the above longitudinal studies adjusted for cardiovascular risk factors. Aging and other risk factors for WMH were thought to not only reduce the capillary density in the affected fiber tracts but also subsequently cause hypometabolism and impaired neural transmission (37,38). Disturbed long connection fibers and commissural fibers in WM might reduce the connections between lobes and hemispheres, resulting in a decrease in metabolic requirements. This reduced glucose metabolism and neuronal activity might further lower the supply need of normal WM and cerebral cortex. However, their microstructures remained relatively intact (39). As such, we would assume that the dysfunction of WM may lead to reductions in blood supply to normal brain tissue (i.e., GM and NAWM), but not the reverse.
Differences between the pathophysiology of PVWMH and DWMH
In the present study, periventricular WMH and deep WMH appeared to have different associations with hypoperfusion, in terms of their progressions. Within the PVWMH coverages, a reduction in blood supply was independently associated with the expansion of hyperintensities on FLAIR images over time. The PVWMH was thought to be more hemodynamically determined, and chronic hypoperfusion may contribute to the expansion of lesions in return (6). The periventricular areas are located at the watershed region of cerebral prefusion. These areas are mostly supplied by ventriculofugal vessels originating from the choroidal arteries and striate rami, but these vessels lack anastomose. Thus, periventricular WM was susceptible to decreased CBF (40,41). Unlike PVWMH, no such CBF interactions were found in DWMH. The deep WM areas are mostly supplied by medullary arteries from middle cerebral arteries (6,42). A previous study reported that disrupted microstructure integrity was a sensitive predictor for DWMH growth but not for CBF (17). Demyelinating injury may play a more significant role in DWMH progression rather than hypoperfusion. Increased DWMH was reported to be associated with high plasma homocysteine level, which was a risk factor for small vessel disease (SVD) (43). We also found that participants with higher vascular risk scores corresponded to severe DWMH burden. Thus, DWMH might be more attributed to SVD and its related risk factors. For example, hypertension is one of the common risk factors for SVD and could lead to fibro hyalinosis on small penetrating arteries and arterioles of WM, and further reduction of the blood supply and localized ischemia (6,42,44,45). These findings may further illustrate that these two subtypes of WMH have different pathogeneses.
Associations between CBF of NAWM and WMH
In this study, we distinguished NAWM from WMH. The CBF in NAWM was found to be higher than that within WMH, but it was not significantly associated with the severity of WMH. However, the severity of WMH was reported to reflect the deterioration of NAWM microstructural integrity (46), which might occur prior to the ischemia-related dysfunctions. Compensatory mechanisms may explain the subsequent hypoperfusion of NAWM but still need to be further investigated (47).
CBF measurements by ASL technique
Although measuring WM perfusion was slightly challenging using ASL MRI, our WM CBF results showed reliable estimates and good agreement with that reported in the literature (48,49). Several studies have shown the feasibility of ASL in measuring WM perfusion within a scanning time of <5 min (49-52). In these studies, the WM perfusion signal could be measured using WM masks to eliminate the contamination of GM in elderly patients and provide reliable estimates of WM perfusion (28). In addition, prolonged transit time, especially in older individuals, might result in the underestimation of CBF in dysfunctional WM structures. On the other hand, this underestimation might correspond to a failure of the microstructure so that when the blood was spun, it could not easily penetrate into the capillaries. However, there was a lack of pathological evidence to support this hypothesis.
Limitations
Several limitations of this study should be noted. First, since the participants were recruited from a community-based study, only a few cases had severe WMH. Thus, we had a limited dataset to analyze the perfusion changes in participants with advanced WMH. Second, more flow information, such as arterial transit time, is warranted for a more precise assessment of blood supply in a wider group of people, especially for older populations in future studies. Third, due to the lack of isotropic, high-resolution, anatomical images, we did not evaluate the brain volumetric changes. To eliminate the potential effects of brain atrophy, we finally chose a sufficiently high probabilistic threshold for segmenting the GM and WM masks and adjusted them as covariates in quantitative analyses for partial volume correction. Finally, around two thirds of participants were lost to follow-up visits due to the restrictions of the COVID-19 pandemic. We compared the clinical characteristics between participants who completed the follow-up study and those who were lost to follow-up. We found that most of the baseline clinical characteristics did not differ significantly between the groups, except for age, DBP and LDL-C. Particularly, there were no significant differences in WMH measurements between the two groups.
Conclusions
The WMH burden is not directly associated with cerebral perfusion at baseline due to the effects of age on both WMH and CBF. Baseline WMH burden could predict future reduction of cerebral perfusion. Additionally, blood flow changes within WMH are potential indicators for the occurrence and progression of WMH volume. Periventricular WMH and deep WMH have different associations with hypoperfusion in terms of their progression.
Acknowledgments
Funding: This work was supported by grants from the Beijing Municipal Commission of Health and Family Planning (No. 2016-1-2041), National Natural Science Foundation of China (No. 81771825), Beijing Municipal Science and Technology Commission (Nos. D17110003017002, D17110003017003), and Ministry of Science and Technology of China (Nos. 2017YFC1307904, 2017YFC1307702 and 2016YFC0901001).
Footnote
Reporting Checklist: The authors have completed the STROBE reporting checklist. Available at https://qims.amegroups.com/article/view/10.21037/qims-22-141/rc
Conflicts of Interest: All authors have completed the ICMJE uniform disclosure form (available at https://qims.amegroups.com/article/view/10.21037/qims-22-141/coif). The authors have no conflicts of interest to declare.
Ethical Statement: The authors are accountable for all aspects of the work in ensuring that questions related to the accuracy or integrity of any part of the work are appropriately investigated and resolved. The study was conducted in accordance with the Declaration of Helsinki (as revised in 2013). The study protocol was approved by the institutional review board of Beijing Tiantan Hospital, and written consent form was provided by all participants at each visit.
Open Access Statement: This is an Open Access article distributed in accordance with the Creative Commons Attribution-NonCommercial-NoDerivs 4.0 International License (CC BY-NC-ND 4.0), which permits the non-commercial replication and distribution of the article with the strict proviso that no changes or edits are made and the original work is properly cited (including links to both the formal publication through the relevant DOI and the license). See: https://creativecommons.org/licenses/by-nc-nd/4.0/.
References
- Pantoni L. Cerebral small vessel disease: from pathogenesis and clinical characteristics to therapeutic challenges. Lancet Neurol 2010;9:689-701. [Crossref] [PubMed]
- Fazekas F, Chawluk JB, Alavi A, Hurtig HI, Zimmerman RA. MR signal abnormalities at 1.5 T in Alzheimer's dementia and normal aging. AJR Am J Roentgenol 1987;149:351-6. [Crossref] [PubMed]
- Lin J, Wang D, Lan L, Fan Y. Multiple Factors Involved in the Pathogenesis of White Matter Lesions. Biomed Res Int 2017;2017:9372050. [Crossref] [PubMed]
- Prins ND, van Dijk EJ, den Heijer T, Vermeer SE, Koudstaal PJ, Oudkerk M, Hofman A, Breteler MM. Cerebral white matter lesions and the risk of dementia. Arch Neurol 2004;61:1531-4. [Crossref] [PubMed]
- Hu HY, Ou YN, Shen XN, Qu Y, Ma YH, Wang ZT, Dong Q, Tan L, Yu JT. White matter hyperintensities and risks of cognitive impairment and dementia: A systematic review and meta-analysis of 36 prospective studies. Neurosci Biobehav Rev 2021;120:16-27. [Crossref] [PubMed]
- Kim KW, MacFall JR, Payne ME. Classification of white matter lesions on magnetic resonance imaging in elderly persons. Biol Psychiatry 2008;64:273-80. [Crossref] [PubMed]
- Lampe L, Kharabian-Masouleh S, Kynast J, Arelin K, Steele CJ, Löffler M, Witte AV, Schroeter ML, Villringer A, Bazin PL. Lesion location matters: The relationships between white matter hyperintensities on cognition in the healthy elderly. J Cereb Blood Flow Metab 2019;39:36-43. [Crossref] [PubMed]
- Crane DE, Black SE, Ganda A, Mikulis DJ, Nestor SM, Donahue MJ, MacIntosh BJ. Gray matter blood flow and volume are reduced in association with white matter hyperintensity lesion burden: a cross-sectional MRI study. Front Aging Neurosci 2015;7:131. [Crossref] [PubMed]
- Stewart CR, Stringer MS, Shi Y, Thrippleton MJ, Wardlaw JM. Associations Between White Matter Hyperintensity Burden, Cerebral Blood Flow and Transit Time in Small Vessel Disease: An Updated Meta-Analysis. Front Neurol 2021;12:647848. [Crossref] [PubMed]
- Bastos-Leite AJ, Kuijer JP, Rombouts SA, Sanz-Arigita E, van Straaten EC, Gouw AA, van der Flier WM, Scheltens P, Barkhof F. Cerebral blood flow by using pulsed arterial spin-labeling in elderly subjects with white matter hyperintensities. AJNR Am J Neuroradiol 2008;29:1296-301. [Crossref] [PubMed]
- Kim CM, Alvarado RL, Stephens K, Wey HY, Wang DJJ, Leritz EC, Salat DH. Associations between cerebral blood flow and structural and functional brain imaging measures in individuals with neuropsychologically defined mild cognitive impairment. Neurobiol Aging 2020;86:64-74. [Crossref] [PubMed]
- Shi Y, Thrippleton MJ, Makin SD, Marshall I, Geerlings MI, de Craen AJM, van Buchem MA, Wardlaw JM. Cerebral blood flow in small vessel disease: A systematic review and meta-analysis. J Cereb Blood Flow Metab 2016;36:1653-67. [Crossref] [PubMed]
- Chen JJ, Rosas HD, Salat DH. Age-associated reductions in cerebral blood flow are independent from regional atrophy. Neuroimage 2011;55:468-78. [Crossref] [PubMed]
- Han H, Lin Z, Soldan A, Pettigrew C, Betz JF, Oishi K, Li Y, Liu P, Albert M, Lu H. Longitudinal Changes in Global Cerebral Blood Flow in Cognitively Normal Older Adults: A Phase-Contrast MRI Study. J Magn Reson Imaging 2022; [Epub ahead of print]. [Crossref] [PubMed]
- Nylander R, Fahlström M, Rostrup E, Kullberg J, Damangir S, Ahlström H, Lind L, Larsson EM. Quantitative and qualitative MRI evaluation of cerebral small vessel disease in an elderly population: a longitudinal study. Acta Radiol 2018;59:612-8. [Crossref] [PubMed]
- ten Dam VH, van den Heuvel DM, de Craen AJ, Bollen EL, Murray HM, Westendorp RG, Blauw GJ, van Buchem MA. Decline in total cerebral blood flow is linked with increase in periventricular but not deep white matter hyperintensities. Radiology 2007;243:198-203. [Crossref] [PubMed]
- Promjunyakul NO, Dodge HH, Lahna D, Boespflug EL, Kaye JA, Rooney WD, Silbert LC. Baseline NAWM structural integrity and CBF predict periventricular WMH expansion over time. Neurology 2018;90:e2119-26. [Crossref] [PubMed]
- Bahrani AA, Powell DK, Yu G, Johnson ES, Jicha GA, Smith CD. White Matter Hyperintensity Associations with Cerebral Blood Flow in Elderly Subjects Stratified by Cerebrovascular Risk. J Stroke Cerebrovasc Dis 2017;26:779-86. [Crossref] [PubMed]
- Dolui S, Tisdall D, Vidorreta M, Jacobs DR Jr, Nasrallah IM, Bryan RN, Wolk DA, Detre JA. Characterizing a perfusion-based periventricular small vessel region of interest. Neuroimage Clin 2019;23:101897. [Crossref] [PubMed]
- McDonald RJ, McDonald JS, Kallmes DF, Jentoft ME, Murray DL, Thielen KR, Williamson EE, Eckel LJ. Intracranial Gadolinium Deposition after Contrast-enhanced MR Imaging. Radiology 2015;275:772-82. [Crossref] [PubMed]
- Kuhn T, Becerra S, Duncan J, Spivak N, Dang BH, Habelhah B, Mahdavi KD, Mamoun M, Whitney M, Pereles FS, Bystritsky A, Jordan SE. Translating state-of-the-art brain magnetic resonance imaging (MRI) techniques into clinical practice: multimodal MRI differentiates dementia subtypes in a traditional clinical setting. Quant Imaging Med Surg 2021;11:4056-73. [Crossref] [PubMed]
- Alsop DC, Detre JA, Golay X, Günther M, Hendrikse J, Hernandez-Garcia L, Lu H, MacIntosh BJ, Parkes LM, Smits M, van Osch MJ, Wang DJ, Wong EC, Zaharchuk G. Recommended implementation of arterial spin-labeled perfusion MRI for clinical applications: A consensus of the ISMRM perfusion study group and the European consortium for ASL in dementia. Magn Reson Med 2015;73:102-16. [Crossref] [PubMed]
- Warnert EAH, Steketee RME, Vernooij MW, Ikram MA, Vogel M, Hernandez Tamames JA, Kotek G. Implementation and validation of ASL perfusion measurements for population imaging. Magn Reson Med 2020;84:2048-54. [Crossref] [PubMed]
- Han H, Zhang R, Liu G, Qiao H, Chen Z, Liu Y, Chen X, Li D, Wang Y, Zhao X. Reduction of cerebral blood flow in community-based adults with subclinical cerebrovascular atherosclerosis: A 3.0T magnetic resonance imaging study. Neuroimage 2019;188:302-8. [Crossref] [PubMed]
- Gottesman RF, Schneider AL, Zhou Y, Coresh J, Green E, Gupta N, Knopman DS, Mintz A, Rahmim A, Sharrett AR, Wagenknecht LE, Wong DF, Mosley TH. Association Between Midlife Vascular Risk Factors and Estimated Brain Amyloid Deposition. JAMA 2017;317:1443-50. [Crossref] [PubMed]
- Smith SM. Fast robust automated brain extraction. Hum Brain Mapp 2002;17:143-55. [Crossref] [PubMed]
- Zhang Y, Brady M, Smith S. Segmentation of brain MR images through a hidden Markov random field model and the expectation-maximization algorithm. IEEE Trans Med Imaging 2001;20:45-57. [Crossref] [PubMed]
- Mutsaerts HJ, Richard E, Heijtel DF, van Osch MJ, Majoie CB, Nederveen AJ. Gray matter contamination in arterial spin labeling white matter perfusion measurements in patients with dementia. Neuroimage Clin 2013;4:139-44. [Crossref] [PubMed]
- Geerlings MI, Appelman AP, Vincken KL, Algra A, Witkamp TD, Mali WP, van der Graaf YSMART Study Group. Brain volumes and cerebrovascular lesions on MRI in patients with atherosclerotic disease. The SMART-MR study. Atherosclerosis 2010;210:130-6. [Crossref] [PubMed]
- Bivard A, Cheng X, Lin LT, Levi C, Spratt N, Kleinig T, O'Brien B, Butcher K, Lou M, Zhang JF, Sylaja PN, Cao WJ, Jannes J, Dong Q, Parsons M. Global White Matter Hypoperfusion on CT Predicts Larger Infarcts and Hemorrhagic Transformation after Acute Ischemia. CNS Neurosci Ther 2016;22:238-43. [Crossref] [PubMed]
- Chen J, Mikheev AV, Yu H, Gruen MD, Rusinek H, Ge YAlzheimer's Disease Neuroimaging Initiative. Bilateral Distance Partition of Periventricular and Deep White Matter Hyperintensities: Performance of the Method in the Aging Brain. Acad Radiol 2021;28:1699-708. [Crossref] [PubMed]
- Staffaroni AM, Cobigo Y, Elahi FM, Casaletto KB, Walters SM, Wolf A, Lindbergh CA, Rosen HJ, Kramer JH. A longitudinal characterization of perfusion in the aging brain and associations with cognition and neural structure. Hum Brain Mapp 2019;40:3522-33. [Crossref] [PubMed]
- Lu H, Xu F, Rodrigue KM, Kennedy KM, Cheng Y, Flicker B, Hebrank AC, Uh J, Park DC. Alterations in cerebral metabolic rate and blood supply across the adult lifespan. Cereb Cortex 2011;21:1426-34. [Crossref] [PubMed]
- van der Veen PH, Muller M, Vincken KL, Hendrikse J, Mali WP, van der Graaf Y, Geerlings MISMART Study Group. Longitudinal relationship between cerebral small-vessel disease and cerebral blood flow: the second manifestations of arterial disease-magnetic resonance study. Stroke 2015;46:1233-8. [Crossref] [PubMed]
- Kraut MA, Beason-Held LL, Elkins WD, Resnick SM. The impact of magnetic resonance imaging-detected white matter hyperintensities on longitudinal changes in regional cerebral blood flow. J Cereb Blood Flow Metab 2008;28:190-7. [Crossref] [PubMed]
- Bernbaum M, Menon BK, Fick G, Smith EE, Goyal M, Frayne R, Coutts SB. Reduced blood flow in normal white matter predicts development of leukoaraiosis. J Cereb Blood Flow Metab 2015;35:1610-5. [Crossref] [PubMed]
- Dalby RB, Eskildsen SF, Videbech P, Frandsen J, Mouridsen K, Sørensen L, Jeppesen P, Bek T, Rosenberg R, Østergaard L. Oxygenation differs among white matter hyperintensities, intersected fiber tracts and unaffected white matter. Brain Commun 2019;1:fcz033. [Crossref] [PubMed]
- Zhu S, Qian S, Xu T, Peng H, Dong R, Wang D, Yuan X, Guo L, Zhang Y, Geng D, Zhong C. White Matter Hyperintensity, Immediate Antihypertensive Treatment, and Functional Outcome After Acute Ischemic Stroke. Stroke 2020;51:1608-12. [Crossref] [PubMed]
- Jiaerken Y, Luo X, Yu X, Huang P, Xu X, Zhang MAlzheimer's Disease Neuroimaging Initiative (ADNI). Microstructural and metabolic changes in the longitudinal progression of white matter hyperintensities. J Cereb Blood Flow Metab 2019;39:1613-22. [Crossref] [PubMed]
- ROWBOTHAM GF. LITTLE E. A new concept of the circulation and the circulations of the brain. The discovery of surface arteriovenous shunts. Br J Surg 1965;52:539-42. [PubMed]
- De Reuck J. The human periventricular arterial blood supply and the anatomy of cerebral infarctions. Eur Neurol 1971;5:321-34. [Crossref] [PubMed]
- Read SJ, Pettigrew L, Schimmel L, Levi CR, Bladin CF, Chambers BR, Donnan GA. White matter medullary infarcts: acute subcortical infarction in the centrum ovale. Cerebrovasc Dis 1998;8:289-95. [Crossref] [PubMed]
- Fassbender K, Mielke O, Bertsch T, Nafe B, Fröschen S, Hennerici M. Homocysteine in cerebral macroangiography and microangiopathy. Lancet 1999;353:1586-7. [Crossref] [PubMed]
- Khan U, Porteous L, Hassan A, Markus HS. Risk factor profile of cerebral small vessel disease and its subtypes. J Neurol Neurosurg Psychiatry 2007;78:702-6. [Crossref] [PubMed]
- Scheltens P, Barkhof F, Leys D, Wolters EC, Ravid R, Kamphorst W. Histopathologic correlates of white matter changes on MRI in Alzheimer's disease and normal aging. Neurology 1995;45:883-8. [Crossref] [PubMed]
- Muñoz Maniega S, Chappell FM, Valdés Hernández MC, Armitage PA, Makin SD, Heye AK, Thrippleton MJ, Sakka E, Shuler K, Dennis MS, Wardlaw JM. Integrity of normal-appearing white matter: Influence of age, visible lesion burden and hypertension in patients with small-vessel disease. J Cereb Blood Flow Metab 2017;37:644-56. [Crossref] [PubMed]
- Reuter-Lorenz PA, Cappell KA. Neurocognitive Aging and the Compensation Hypothesis. Current Directions in Psychological Science 2008;17:177-82. [Crossref]
- Giezendanner S, Fisler MS, Soravia LM, Andreotti J, Walther S, Wiest R, Dierks T, Federspiel A. Microstructure and Cerebral Blood Flow within White Matter of the Human Brain: A TBSS Analysis. PLoS One 2016;11:e0150657. [Crossref] [PubMed]
- Wu WC, Lin SC, Wang DJ, Chen KL, Li YD. Measurement of cerebral white matter perfusion using pseudocontinuous arterial spin labeling 3T magnetic resonance imaging--an experimental and theoretical investigation of feasibility. PLoS One 2013;8:e82679. [Crossref] [PubMed]
- Skurdal MJ, Bjørnerud A, van Osch MJ, Nordhøy W, Lagopoulos J, Groote IR. Voxel-Wise Perfusion Assessment in Cerebral White Matter with PCASL at 3T; Is It Possible and How Long Does It Take? PLoS One 2015;10:e0135596. [Crossref] [PubMed]
- Promjunyakul N, Lahna D, Kaye JA, Dodge HH, Erten-Lyons D, Rooney WD, Silbert LC. Characterizing the white matter hyperintensity penumbra with cerebral blood flow measures. Neuroimage Clin 2015;8:224-9. [Crossref] [PubMed]
- Zhuang C, Poublanc J, Mcketton L, Venkatraghavan L, Sobczyk O, Duffin J, Crawley AP, Fisher JA, Wu R, Mikulis DJ. The value of a shorter-delay arterial spin labeling protocol for detecting cerebrovascular impairment. Quant Imaging Med Surg 2021;11:608-19. [Crossref] [PubMed]