The influence of a deep learning image reconstruction algorithm on the image quality and auto-analysis of pulmonary nodules at ultra-low dose chest CT: a phantom study
Introduction
Lung cancer is one of the major threats to human health, and its morbidity and mortality rank first among cancers (1,2). Early diagnosis of lung cancer is the key to the successful treatment and prolonged survival of patients (3). In 2011, the National Lung Screening Test (NLST) reported that low-dose computed tomography (LDCT) is more sensitive to the early detection of lung cancer than chest X-rays, and that LDCT lung cancer screening reduces deaths by 15 to 20% (4,5). LDCT has become an effective method for early lung cancer screening, but it is critical to maintain image quality and diagnostic confidence while reducing radiation dose (4,6).
A number of strategies, such as tube current and tube voltage modulation, and multiple reconstruction techniques, such as iterative reconstruction (IR) algorithms, have been developed and used for the purpose of dose reduction and image quality improvement (7,8). The Filtered Back Projection (FBP) reconstruction algorithm has been the historical standard for CT image reconstruction. However, the use of FBP, particularly when lower radiation doses are used, results in higher image noise, causing fringe artifacts and poor low contrast-to-noise ratio, affecting image evaluation (9-11). Compared with FBP, IR techniques can reduce image noise and radiation dose while ensuring image quality (12,13). The adaptive statistical iterative reconstruction algorithm-V (ASIR-V) is one of the IR algorithms developed to further reduce image noise (9,14,15). However, the nonlinear and non-stationary characteristics of IR algorithms make the spatial resolution dependent on contrast and dose, and change the noise intensity and texture of the image (16). In addition, high level IR algorithms may produce wax-like or plastic-like artifacts, which may affect image quality and evaluation of low contrast lesions.
With the increased use of machine learning as a subset of artificial intelligence, a deep learning image reconstruction (DLIR) algorithm (TrueFidelity, GE Healthcare) has been introduced and showed great potential in medical imaging (17-19). Deep learning-based image reconstruction technology in general can suppress image noise while minimizing the change in noise texture or anatomical and pathological structures (20,21). Specifically, DLIR is based on Deep Convolutional Neural Networks (DCNN), and uses normal-dose, high-quality FBP images to train the algorithm to distinguish noise and artifacts from signals, which reduces image noise, suppress spectral artifacts, and improve the detectability and resolution of low-contrast objects (22). To the best of our knowledge, the effect of DLIR algorithm on the detection, characterization, and image quality of pulmonary nodules (PNs) in chest CT at different dose levels has yet to be evaluated. This is important because this knowledge may help to optimize radiation dose and improve the detection and characterization of lesions in chest CT examinations.
Computer-aided diagnostic systems have been used for automatic detection and analysis of pulmonary nodules for many years (23). With the development of computer technology and the improvement of hardware, Artificial Intelligence (AI) is now widely used in imaging. With the emergence of deep learning, the application of artificial intelligence technology to the intelligent detection of pulmonary nodules based on chest CT images has become a hot topic in this field (24).
Therefore, the purpose of this study was to compare image quality, detection rate, and characterization of pulmonary nodules in chest CT, using a phantom scanned with different tube currents and reconstructed with FBP, ASIR-V and DLIR algorithms.
We present the following article in accordance with the STARD reporting checklist (available at https://qims.amegroups.com/article/view/10.21037/qims-21-815/rc).
Methods
Phantom
In the present study, a Lungman chest phantom (Lungman ph-1, Kyoto Kagaku Inc, Japan) was used (Figure 1). Trachea and pulmonary vessels were simulated by a mesh structure connected to the mediastinum. The lungs were simulated by air naturally filled in the phantom. A total of 9 isolated spherical nodules included ground glass nodules (GGNs) and solid nodules (SNs) with different diameters (8, 10, 12 mm) and different attenuations (−800, −630, +100 HU for each diameter) were randomly placed in a thorax anthropomorphic phantom.

Scanning techniques
A 256-row CT scanner (Revolution CT, GE Healthcare, Milwaukee, WI, USA) was used to scan the phantom with nine simulated artificial pulmonary nodules (PNs), and eight scans were performed at 70 kVp with different tube currents (20, 30, 50, 70, 90, 100, 120 and 150 mA), 0.5s rotation speed, helical scan mode with 0.992:1 pitch, 40 mm detector width, 360 mm display field of view, and 512×512 matrix. Apart from the tube current, the other parameters did not change for the repeated scans, including the position of phantom and nodules.
Image reconstruction and processing
The raw data of the above eight scans were reconstructed using FBP, ASIR-V and DLIR algorithms at different strengths. All reconstructed images were axial, with a slice thickness and interval of 0.625 mm. Thus, a total of 7 subsets of images at each dose level were obtained: FBP, 30%ASIR-V, 50%ASIR-V, 80%ASIR-V, DLIR-Low, DLIR-Medium, DLIR-High. All reconstructed images were then transferred to an AW4.7 workstation (GE Healthcare, Milwaukee, WI, USA) and an image processing machine with an artificial intelligence (AI) software (Intelligent 4D Imaging System for Chest CT 5.5, YITU Healthcare) for image analysis and processing.
Image quality assessment
Two radiologists independently reviewed all images in random order, blinded to the scanning parameters and reconstruction algorithms, and graded the images on a scale from 1 (worst) to 4 (best) for each of the following imaging features: image contrast, image noise and nodule edge. The mean score of image contrast, image noise and nodule edge were evaluated as overall image quality. A score greater than or equal to 3 is considered to meet clinical requirements (as shown in Table 1).
Table 1
Score | Image contrast | Image noise | Nodule edge |
---|---|---|---|
4 points | Excellent image contrast | Minimal image noise | Completely clear edge |
3 points | Acceptable contrast | Less than average image noise | Clear edge |
2 points | Suboptimal contrast | Average image noise | Less clear edge |
1 point | Poor contrast | Unacceptable image noise | Beyond recognition edge |
The long/short diameters and average CT attenuation value (HU), standard deviation (SD) of PNs were measured automatically by an AI software (Intelligent 4D Imaging System for Chest CT 5.5, YITU Healthcare). The numbers of recognized nodules were counted manually. The mean CT and standard deviation of each nodule was recorded as CT1 and SD1, respectively. We selected the anterior sternum at the same level as the nodule for the air measurement, which recorded CT2 for the mean CT value and SD2 for the standard deviation. The contrast-to-noise [CNR, CNR=(CT1−CT2)/SD2], signal-to-noise (SNR, SNR=CT1/SD1), detection rate of PNs and the deformation degree (DD, DD=long diameter/short diameter) of pulmonary nodules were calculated by a technician for each reconstruction algorithm and at each dose level. The same window width and window level were used for image display.
Radiation dose
To assess radiation exposure, the system automatically records volume CT dose index (CTDIvol, in mGy) and dose length product (DLP, in mGy*cm). The effective radiation dose (ED) is the product of radiation weight factor K and DLP: ED=DLP × K, and K is 0.014 mSv/(mGy·cm) for the chest (European Standard Guide).
Statistical analysis
The CT value, SD, SNR and CNR values were expressed as mean ± standard deviation, and the values with FBP, ASIR-V algorithm and DLIR algorithm at different strengths were analyzed by using the Analysis of Variance (ANOVA test). A P value of less than 0.05 was considered statistically significant. The Kappa test was used to evaluate the interobserver consistency of image quality score: A Kappa value of 0.75 indicated good consistency, 0.4< Kappa value <0.75 indicated medium consistency, and a Kappa value <0.4 indicated poor consistency. Also, the subjective image quality scores were analyzed by using the non-parametric analysis of variance (Kruskal-Wallis test). All statistical analyses were performed with SPSS statistical software (version 22.0, IBM SPSS Statistics).
Results
Radiation dose
Radiation dose increased as the tube current increased, which is shown in Table 2.
Table 2
mA | 20 | 30 | 50 | 70 | 90 | 100 | 120 | 150 |
---|---|---|---|---|---|---|---|---|
CTDIvol (mGy) | 0.13 | 0.2 | 0.34 | 0.47 | 0.61 | 0.67 | 0.81 | 1.01 |
DLP (mGy*cm) | 4.88 | 7.32 | 12.19 | 17.07 | 21.95 | 24.38 | 29.25 | 36.57 |
ED (mSV) | 0.06 | 0.10 | 0.17 | 0.23 | 0.30 | 0.34 | 0.41 | 0.51 |
CTDIvol, volume CT dose index; DLP, dose-length product; ED, effective dose.
Subjective analysis
The two physicians had good consistency in the evaluation of image contrast, noise of the background and nodules (κ=0.78, 0.87 and 0.82). Under different mA conditions, there were significant differences in the image quality scores of PNs among different reconstruction groups (P<0.05, P=0.001). Under different reconstruction conditions, there were significant differences in image noise of PNs (P<0.05, P=0.004), but no significant difference for the other measurements (P>0.05). As for the subjective image quality score (image contrast, noise and nodule edge), the FBP algorithm resulted in the lowest mean scores, with the mean scores of DLIR being slightly higher than those of ASIR-V. The 80% ASIR-V, DLIR-H, and DLIR-M were better than other reconstruction algorithms in image contrast, noise, and nodule edge. DLIR algorithm with different strengths led to better overall image quality compared with the ASIR-V or FBP algorithms under 20 and 30 mA; and in other mA conditions, DLIR-M, DLIR-H and ASIR-V had similar and good image quality (Table 3). Among DLIR with different strengths, medium strength level resulted in the best image contrast and nodule edge, followed by the high strength level and then the low strength level, while DLIR-H performed the best in reducing image noise (as shown in Table 3).
Table 3
mA | IR | Image contrast | Image noise | Nodule edge | Overall |
---|---|---|---|---|---|
20 | FBP | 2.33 | 2.11 | 2.00 | 2.15 |
30%ASIR-V | 2.56 | 2.22 | 2.22 | 2.33 | |
50%ASIR-V | 2.56 | 2.44 | 2.33 | 2.44 | |
80%ASIR-V | 2.56 | 2.56 | 2.33 | 2.48 | |
DLIR-L | 2.33 | 2.78 | 2.33 | 2.48 | |
DLIR-M | 2.44 | 2.89 | 2.56 | 2.63 | |
DLIR-H | 2.44 | 2.89 | 2.22 | 2.52 | |
30 | FBP | 2.56 | 2.33 | 2.44 | 2.44 |
30%ASIR-V | 2.56 | 2.56 | 2.44 | 2.52 | |
50%ASIR-V | 2.89 | 2.67 | 2.44 | 2.67 | |
80%ASIR-V | 3.11 | 3.11 | 2.67 | 2.96 | |
DLIR-L | 2.44 | 2.67 | 2.44 | 2.52 | |
DLIR-M | 3.00 | 3.00 | 2.56 | 2.85 | |
DLIR-H | 2.78 | 3.33 | 2.89 | 3 | |
50 | FBP | 2.78 | 2.67 | 2.67 | 2.7 |
30%ASIR-V | 3.22 | 3.00 | 3.22 | 3.15 | |
50%ASIR-V | 3.33 | 3.56 | 3.33 | 3.41 | |
80%ASIR-V | 3.33 | 3.56 | 3.33 | 3.41 | |
DLIR-L | 2.89 | 3.11 | 3.00 | 3.00 | |
DLIR-M | 3.22 | 3.44 | 3.44 | 3.37 | |
DLIR-H | 3.33 | 3.56 | 3.33 | 3.41 | |
70 | FBP | 2.78 | 2.67 | 2.67 | 2.7 |
30%ASIR-V | 3.00 | 2.89 | 3.00 | 2.96 | |
50%ASIR-V | 3.22 | 3.33 | 3.22 | 3.26 | |
80%ASIR-V | 3.22 | 3.33 | 3.22 | 3.26 | |
DLIR-L | 3.00 | 3.00 | 3.00 | 3.00 | |
DLIR-M | 3.44 | 3.44 | 3.44 | 3.44 | |
DLIR-H | 3.33 | 3.67 | 3.44 | 3.48 | |
90 | FBP | 3.22 | 2.89 | 3.00 | 3.04 |
30%ASIR-V | 3.22 | 3.11 | 3.11 | 3.15 | |
50%ASIR-V | 3.44 | 3.56 | 3.56 | 3.52 | |
80%ASIR-V | 3.67 | 3.67 | 3.67 | 3.67 | |
DLIR-L | 3.33 | 3.22 | 3.33 | 3.30 | |
DLIR-M | 3.67 | 3.67 | 3.67 | 3.67 | |
DLIR-H | 3.56 | 3.67 | 3.56 | 3.59 | |
100 | FBP | 3.11 | 3.11 | 3.11 | 3.11 |
30%ASIR-V | 3.22 | 3.22 | 3.11 | 3.19 | |
50%ASIR-V | 3.33 | 3.33 | 3.33 | 3.33 | |
80%ASIR-V | 3.56 | 3.67 | 3.67 | 3.63 | |
DLIR-L | 3.22 | 3.22 | 3.11 | 3.19 | |
DLIR-M | 3.44 | 3.56 | 3.54 | 3.48 | |
DLIR-H | 3.56 | 3.89 | 3.56 | 3.67 | |
120 | FBP | 3.33 | 3.11 | 3.11 | 3.19 |
30%ASIR-V | 3.33 | 3.11 | 3.22 | 3.48 | |
50%ASIR-V | 3.44 | 3.44 | 3.44 | 3.67 | |
80%ASIR-V | 3.67 | 3.67 | 3.44 | 3.19 | |
DLIR-L | 3.33 | 3.11 | 3.22 | 3.22 | |
DLIR-M | 3.67 | 3.44 | 3.56 | 3.56 | |
DLIR-H | 3.78 | 3.89 | 3.44 | 3.70 | |
150 | FBP | 3.89 | 3.56 | 3.56 | 3.67 |
30%ASIR-V | 3.89 | 3.56 | 3.56 | 3.67 | |
50%ASIR-V | 4.00 | 4.00 | 4.00 | 4.00 | |
80%ASIR-V | 4.00 | 4.00 | 4.00 | 4.00 | |
DLIR-L | 3.89 | 3.56 | 3.56 | 3.67 | |
DLIR-M | 4.00 | 4.00 | 4.00 | 4.00 | |
DLIR-H | 4.00 | 4.00 | 4.00 | 4.00 |
FBP, filtered back projection; ASIR-V, adaptive statistical iterative reconstruction; DLIR-L/M/H, deep learning image reconstruction-low/medium/high.
Quantitative analysis
The detection of PNs
There were differences in the detection rate of PNs among different tube currents and different reconstruction algorithms. The detection rates at 20 mA were the lowest, caused by the excessive image noise due to lower dose, and the detection rate was similar for all reconstruction algorithms (6/9, 66.67%). At the next dose level (tube current of 30 mA), the detection rate of DLIR-H was significantly higher than the others (9/9, 100%). When the tube current was equal to or greater than 50 mA, there was no difference among different reconstruction algorithms (as shown in Table 4).
Table 4
mA | Reconstruction algorithm | Detection rate | DD |
---|---|---|---|
20 | FBP | 6/9 (66.67%) | 1.09 |
30%ASIR-V | 6/9 (66.67%) | 1.17 | |
50%ASIR-V | 6/9 (66.67%) | 1.09 | |
80%ASIR-V | 6/9 (66.67%) | 1.12 | |
DLIR-L | 6/9 (66.67%) | 1.11 | |
DLIR-M | 6/9 (66.67%) | 1.08 | |
DLIR-H | 6/9 (66.67%) | 1.11 | |
Mean | 6/9 (66.67%) | 1.11 | |
30 | FBP | 6/9 (66.67%) | 1.08 |
30%ASIR-V | 6/9 (66.67%) | 1.11 | |
50%ASIR-V | 8/9 (88.89%) | 1.08 | |
80%ASIR-V | 8/9 (88.89%) | 1.12 | |
DLIR-L | 7/9 (77.78%) | 1.07 | |
DLIR-M | 7/9 (77.78%) | 1.06 | |
DLIR-H | 9/9 (100.00%)‡ | 1.07 | |
Mean | 7.28/9 (80.95%) | 1.08 | |
50 | FBP | 8/9 (88.89%) | 1.10 |
30%ASIR-V | 8/9 (88.89%) | 1.17 | |
50%ASIR-V | 8/9 (88.89%) | 1.14 | |
80%ASIR-V | 9/9 (100.00%) | 1.1 | |
DLIR-L | 8/9 (88.89%) | 1.1 | |
DLIR-M | 8/9 (88.89%) | 1.12 | |
DLIR-H | 8/9 (88.89%) | 1.08 | |
Mean | 8.14/9 (90.47%) | 1.12 | |
70 | FBP | 8/9 (88.89%) | 1.17 |
30%ASIR-V | 8/9 (88.89%) | 1.14 | |
50%ASIR-V | 9/9 (100.00%) | 1.13 | |
80%ASIR-V | 9/9 (100.00%) | 1.08 | |
DLIR-L | 8/9 (88.89%) | 1.09 | |
DLIR-M | 8/9 (88.89%) | 1.07 | |
DLIR-H | 8/9 (88.89%) | 1.08 | |
Mean | 8.28/9 (92.06%) | 1.11 | |
90 | FBP | 8/9 (88.89%) | 1.06 |
30%ASIR-V | 9/9 (100.00%) | 1.1 | |
50%ASIR-V | 9/9 (100.00%) | 1.08 | |
80%ASIR-V | 9/9 (100.00%) | 1.08 | |
DLIR-L | 8/9 (88.89%) | 1.07 | |
DLIR-M | 9/9 (100.00%) | 1.07 | |
DLIR-H | 9/9 (100.00%) | 1.11 | |
Mean | 8.71/9 (96.83%) | 1.08 | |
100 | FBP | 9/9 (100.00%) | 1.12 |
30%ASIR-V | 9/9 (100.00%) | 1.1 | |
50%ASIR-V | 9/9 (100.00%) | 1.12 | |
80%ASIR-V | 9/9 (100.00%) | 1.08 | |
DLIR-L | 9/9 (100.00%) | 1.07 | |
DLIR-M | 9/9 (100.00%) | 1.07 | |
DLIR-H | 9/9 (100.00%) | 1.08 | |
Mean | 9/9 (100.00%) | 1.09 | |
120 | FBP | 9/9 (100.00%) | 1.10 |
30%ASIR-V | 9/9 (100.00%) | 1.1 | |
50%ASIR-V | 9/9 (100.00%) | 1.07 | |
80%ASIR-V | 9/9 (100.00%) | 1.08 | |
DLIR-L | 9/9 (100.00%) | 1.06 | |
DLIR-M | 9/9 (100.00%) | 1.07 | |
DLIR-H | 9/9 (100.00%) | 1.04 | |
Mean | 9/9 (100.00%) | 1.07 | |
150 | FBP | 9/9 (100.00%) | 1.11 |
30%ASIR-V | 9/9 (100.00%) | 1.09 | |
50%ASIR-V | 9/9 (100.00%) | 1.08 | |
80%ASIR-V | 9/9 (100.00%) | 1.07 | |
DLIR-L | 9/9 (100.00%) | 1.08 | |
DLIR-M | 9/9 (100.00%) | 1.07 | |
DLIR-H | 9/9 (100.00%) | 1.08 | |
Mean | 9/9 (100.00%) | 1.08 |
‡, at this condition, the detection rate of DLIR-H was significantly higher than the others. DD, the deformation degree; FBP, filtered back projection; ASIR-V, adaptive statistical iterative reconstruction; DLIR-L/M/H, deep learning image reconstruction-low/medium/high.
The characterization of PNs
Regardless of the reconstruction algorithm, there were significant differences in the characterization of PNs among different tube currents. The DDmean at 50 mA dose level was higher than 20 and 30 mA, but in general, the results showed the trend of a decreased degree of nodule deformation with the increase of tube current (P=0.005, Table 4).
Regardless of tube current and reconstruction strength, the DDmean of DLIR, ASIR-V and FBP were 1.08, 1.10 and 1.11, respectively. There was significant difference between DLIR and ASIR-V (P=0.002) and between DLIR and FBP (P=0.013). The DDmean were 1.08, 1.07, 1.08, 1.12, 1.09, 1.08 and 1.11 for DLIR-L, DLIR-M, DLIR-H, 30%, 50%, 80% ASIR-V and FBP, respectively. DLIR-M performed best among all reconstruction algorithm groups, with the smallest DDmean of 1.07 (P=0.001) (Figure 2).
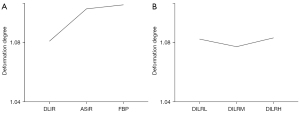
CT attenuation value, Image Noise and CNR
Under different mA conditions, there was no statistically significant difference in CT value of the GGNs or SNs among different reconstruction groups (P>0.05). However, there were significant differences in SDnodule, SDair, SNR and CNR among different groups (all P<0.05; Tables 5,6).
Table 5
mA | Reconstruction algorithm | CTnodule | SDnodule | CTair | SDair | SNR | CNR |
---|---|---|---|---|---|---|---|
20 | DLIR-L | −724.79±105.42 | 56.88±7.45 | −1,002.45±5.41 | 32.95±1.99 | −12.75±0.99 | 8.44±3.21 |
DLIR-M | −721.96±105.58 | 49.89±12.47 | −999.58±5.16 | 23.84±1.12 | −15.80±7.36 | 11.59±4.34 | |
DLIR-H | −728.61±106.23 | 44.76±11.76 | −1,001.53±4.46 | 13.13±1.00 | −17.37±5.80 | 20.88±8.30 | |
30%ASIR-V | −737.62±133.42 | 116.17±34.54 | −96.72±5.73 | 77.27±3.52 | −7.36±4.63 | 2.91±1.77 | |
50%ASIR-V | −732.43±115.35 | 113.53±20.00 | −972.85±2.13 | 66.53±3.82 | −6.68±2.03 | 3.64±1.81 | |
80%ASIR-V | −723.63±116.92 | 78.17±24.10 | −982.56±4.61 | 51.19±5.35 | −9.92±2.75 | 5.14±2.49 | |
FBP | −719.50±108.49 | 145.64±22.03 | −956.46±7.01 | 88.42±7.56 | −5.02±0.98 | 2.70±1.40 | |
30 | DLIR-L | −718.89±93.32 | 53.39±8.55 | −1,005.10±2.23 | 30.39±1.51 | −13.82±3.45 | 9.46±3.23 |
DLIR-M | −714.88±97.62 | 48.24±11.09 | −104.46±2.35 | 21.24±0.81 | −15.75±5.20 | 13.61±4.46 | |
DLIR-H | −724.79±100.79 | 44.52±14.00 | −1,003.82±2.87 | 12.14±0.13 | −18.37±8.80 | 23.01±8.28 | |
30%ASIR-V | −724.80±91.41 | 129.72±21.27 | −970.69±4.33 | 70.83±4.79 | −5.82±1.78 | 3.51±1.37 | |
50%ASIR-V | −722.09±82.07 | 114.13±12.37 | −976.07±3.44 | 61.70±4.44 | −6.24±1.26 | 4.12±1.46 | |
80%ASIR-V | −719.91±91.39 | 86.92±11.47 | −987.32±2.26 | 45.96±2.81 | −8.39±1.50 | 5.85±2.14 | |
FBP | −723.72±99.66 | 168.11±32.85 | −961.45±3.02 | 82.92±7.05 | −4.54±1.65 | 2.85±1.12 | |
50 | DLIR-L | −724.02±101.23 | 40.57±7.36 | −1,005.46±1.66 | 25.56±1.61 | 18.44±4.71 | 11.16±4.39 |
DLIR-M | −721.09±108.25 | 40.64±4.93 | −1,005.29±0.83 | 18.49±2.14 | 17.95±3.51 | 15.89±7.08 | |
DLIR-H | −726±112.23 | 33.69±10.06 | −1,003±1.71 | 10.10±0.81 | 23.87±10.09 | 27.78±12.16 | |
30%ASIR-V | −728.68±103.02 | 121.63±25.73 | −973.42±3.71 | 68.33±4.36 | 6.32±1.99 | 3.62±1.61 | |
50%ASIR-V | −722.76±109.39 | 104.61±18.75 | −979.17±2.03 | 55.77±3.61 | 7.05±1.29 | 4.91±1.94 | |
80%ASIR-V | −721.60±103.41 | 71.56±24.17 | −989.08±2.64 | 41.79±5.38 | 10.78±2.98 | 6.45±2.56 | |
FBP | −726.57±99.77 | 144.57±25.19 | −963.36±4.18 | 77.31±4.83 | 5.21±1.41 | 3.07±1.32 | |
70 | DLIR-L | −730.11±100.98 | 38.17±8.77 | −1,005.85±3.74 | 21.87±3.82 | 20.67±8.78 | 13.38±6.46 |
DLIR-M | −731.87±109.73 | 33.34±8.23 | −1,004.62±2.17 | 15.63±1.85 | 24.07±11.06 | 17.92±8.24 | |
DLIR-H | −730.68±107.73 | 32.17±9.51 | −1,004.79±1.53 | 8.79±0.86 | 25.66±12.94 | 31.94±14.91 | |
30%ASIR-V | −731.70±108.04 | 104.08±41.96 | −974.34±1.83 | 62.56±2.36 | 8.60±4.74 | 3.91±1.82 | |
50%ASIR-V | −733.39±112.72 | 95.79±18.29 | −981.79±0.93 | 52.69±3.07 | 8.10±2.84 | 4.76±2.27 | |
80%ASIR-V | −735.19±104.07 | 65.66±18.23 | −990.18±1.98 | 41.37±2.08 | 12.80±7.14 | 6.08±2.27 | |
FBP | −730.26±107.73 | 140.83±23.96 | −966.67±3.24 | 75.27±5.65 | 5.42±1.78 | 3.19±1.59 | |
90 | DLIR-L | −738.99±107.52 | 34.85±7.64 | −1,005.48±2.16 | 20.39±1.51 | 22.36±7.75 | 13.14±5.45 |
DLIR-M | −735.89±104.99 | 33.89±5.07 | −1,005.76±2.83 | 14.27±1.04 | 21.95±3.67 | 19.13±7.91 | |
DLIR-H | −735.05±104.50 | 30.72±5.93 | −1,005.40±2.88 | 8.21±0.67 | 24.61±5.55 | 33.17±13.12 | |
30%ASIR-V | −741.62±105.48 | 103.52±12.21 | −976.09±3.31 | 60.45±8.07 | 7.18±0.77 | 3.89±1.67 | |
50%ASIR-V | −738.21±107.83 | 89.77±9.96 | −983.28±1.76 | 49.42±4.34 | 8.30±1.57 | 5.00±2.25 | |
80%ASIR-V | −766.27±146.75 | 56.96±13.50 | −952.80±65.95 | 39.40±4.28 | 14.77±7.52 | 5.07±4.94 | |
FBP | −743.69±98.97 | 131.93±13.24 | −969.41±2.10 | 70.03±4.87 | 5.73±1.26 | 3.26±1.53 | |
100 | DLIR-L | −727.83±101.18 | 32.17±4.16 | −1,004.21±3.05 | 19.18±3.17 | 22.97±4.71 | 15.24±7.24 |
DLIR-M | −730.04±108.11 | 31.36±2.98 | −1,004.68±2.59 | 14.37±1.44 | 23.52±4.52 | 19.63±8.83 | |
DLIR-H | −724.19±100.42 | 30.50±3.14 | −1,003.78±2.56 | 8.02±1.04 | 24.11±5.28 | 35.98±15.34 | |
30%ASIR-V | −741.23±95.67 | 106.67±18.76 | −978.77±1.44 | 56.72±2.72 | 7.03±0.93 | 4.19±1.71 | |
50%ASIR-V | −732.90±97.58 | 85.84±9.49 | −983.88±2.24 | 50.58±3.54 | 8.58±1.18 | 5.01±2.06 | |
80%ASIR-V | −735.57±99.09 | 58.48±12.42 | −990.27±4.07 | 39.10±4.15 | 12.76±1.46 | 6.71±3.06 | |
FBP | −736.57±111.72 | 121.52±19.44 | −971.49±2.58 | 69.21±5.35 | 6.23±1.68 | 3.40±1.63 | |
120 | DLIR-L | −737.77±105.54 | 28.34±4.64 | −1,006.37±3.23 | 17.54±1.42 | 26.41±4.69 | 15.68±6.94 |
DLIR-M | −734.61±106.68 | 30.05±4.36 | −1,005.01±2.53 | 12.31±1.48 | 24.63±3.39 | 22.69±10.41 | |
DLIR-H | −729.54±103.97 | 25.20±4.38 | −1,005.34±2.69 | 7.24±0.97 | 29.81±7.04 | 39.18±16.81 | |
30%ASIR-V | −734.40±99.50 | 91.12±15.56 | −981.59±2.49 | 55.41±4.92 | 8.34±2.35 | 4.56±2.04 | |
50%ASIR-V | −744.11±103.41 | 75.32±9.34 | −987.19±3.52 | 47.31±5.27 | 9.90±0.88 | 5.25±1.02 | |
80%ASIR-V | −741.07±109.36 | 51.68±12.47 | −998.79±1.95 | 36.84±3.06 | 14.69±2.29 | 7.01±3.33 | |
FBP | −740.89±103.93 | 139.62±10.98 | −968.30±3.05 | 73.43±4.27 | 5.35±1.01 | 3.17±1.62 | |
150 | DLIR-L | −735.81±94.41 | 23.51±4.05 | −1,005.21±3.40 | 15.92±1.75 | 32.04±6.81 | 17.45±7.32 |
DLIR-M | −734.44±93.17 | 24.63±4.24 | −1,004.50±3.51 | 11.10±1.12 | 30.41±5.96 | 24.91±9.91 | |
DLIR-H | −732.24±103.23 | 23.32±7.47 | −1,004.07±2.64 | 6.65±0.96 | 34.72±13.66 | 42.79±20.26 | |
30%ASIR-V | −755.51±142.11 | 80.31±18.95 | −955.96±66.96 | 55.66±10.79 | 10.27±4.81 | 4.12±3.56 | |
50%ASIR-V | −738.56±103.15 | 76.32±16.44 | −986.44±3.65 | 46.86±4.96 | 10.10±2.98 | 5.51±2.81 | |
80%ASIR-V | −735.13±103.16 | 52.51±11.51 | −994.17±1.96 | 35.52±2.91 | 14.40±3.09 | 7.46±3.37 | |
FBP | −745.57±91.79 | 102.95±17.85 | −975.67±2.21 | 62.48±4.22 | 7.41±1.49 | 3.75±1.67 |
GGNs, ground glass nodules; SD, standard deviation; SNR, signal-to-noise ratio; CNR, contrast-to-noise ratio; FBP, filtered back projection; ASIR-V, adaptive statistical iterative reconstruction; DLIR-L/M/H, deep learning image reconstruction-low/medium/high.
Table 6
mA | Reconstruction algorithm | CTnodule | SDnodule | CTair | SDair | SNR | CNR |
---|---|---|---|---|---|---|---|
20 | DLIR-L | 132.47±24.61 | 37.62±5.61 | −1,000.85±2.87 | 34.68±3.91 | 3.57±0.80 | 33.01±4.48 |
DLIR-M | 123.30±1.45 | 30.37±5.75 | −1,001.70±5.08 | 23.50±4.49 | 4.16±0.81 | 48.12±4.19 | |
DLIR-H | 119.82±13.01 | 19.10±2.23 | −1,000.42±4.01 | 13.76±1.37 | 6.28±0.21 | 82.01±8.66 | |
30%ASIR-V | 145.01±51.71 | 101.05±27.33 | −964.33±6.02 | 76.91±8.35 | 1.64±1.11 | 14.58±2.31 | |
50%ASIR-V | 148.60±40.99 | 84.53±28.43 | −974.73±0.49 | 62.30±4.28 | 2.07±1.41 | 18.09±1.55 | |
80%ASIR-V | 142.39±36.89 | 64.65±5.78 | −982.78±4.14 | 50.08±7.39 | 2.23±0.69 | 22.91±4.53 | |
FBP | 153.82±19.94 | 123.09±41.4 | −957.41±2.45 | 86.56±4.77 | 1.43±0.78 | 12.87±0.90 | |
30 | DLIR-L | 136.05±15.51 | 33.83±11.34 | −1,000.69±6.06 | 28.89±3.99 | 4.49±2.13 | 39.85±5.50 |
DLIR-M | 133.47±17.93 | 25.67±4.20 | −1,002.88±4.74 | 20.05±1.45 | 5.38±1.67 | 56.89±4.62 | |
DLIR-H | 125.32±4.87 | 18.76±7.1 | −1,001.66±3.49 | 11.53±1.47 | 7.42±2.71 | 98.76±12.28 | |
30%ASIR-V | 144.64±17.08 | 108.56±25.09 | −972.80±4.54 | 66.56±3.77 | 1.39±0.37 | 16.84±1.25 | |
50%ASIR-V | 153.80±20.49 | 95.07±477.73 | −910.55±4.62 | 58.91±4.88 | 1.92±0.90 | 15.13±8.80 | |
80%ASIR-V | 158.00±11.77 | 64.20±5.90 | −987.23±1.61 | 44.58±4.34 | 2.46±0.04 | 25.87±2.80 | |
FBP | 158.72±33.67 | 136.47±14.86 | −958.44±5.87 | 82.93±4.39 | 1.19±0.36 | 13.51±1.14 | |
50 | DLIR-L | 131.74±15.93 | 29.32±2.39 | −1,005.60±1.03 | 25.70±1.84 | 4.53±0.82 | 44.37±2.46 |
DLIR-M | 133.83±17.33 | 20.07±1.31 | −1,005.77±1.05 | 18.15±1.44 | 6.73±1.35 | 63.01±4.21 | |
DLIR-H | 133.20±18.21 | 13.14±1.21 | −1,005.36±1.04 | 10.57±3.78 | 10.20±1.76 | 108.24±8.59 | |
30%ASIR-V | 128.45±27.67 | 99.82±2.88 | −975.60±0.47 | 62.88±4.39 | 1.28±0.24 | 17.60±1.22 | |
50%ASIR-V | 150.80±16.33 | 78.29±12.66 | −928.88±0.38 | 54.61±3.69 | 1.94±0.09 | 19.244.36 | |
80%ASIR-V | 148.07±16.06 | 55.56±14.14 | −989.44±1.68 | 43.93±3.89 | 2.80±0.89 | 26.02±2.14 | |
FBP | 140.15±8.38 | 127.27±14.80 | −962.77±4.15 | 79.62±3.77 | 1.11±0.17 | 13.88±0.76 | |
70 | DLIR-L | 134.19±5.38 | 26.66±0.43 | −1,005.07±2.78 | 21.91±1.95 | 5.04±0.28 | 52.26±4.64 |
DLIR-M | 130.75±7.75 | 21.62±2.72 | −1,004.95±1.89 | 15.08±1.39 | 6.12±0.93 | 75.75±6.82 | |
DLIR-H | 131.96±6.97 | 11.57±1.58 | −1,004.71±2.23 | 8.98±0.52 | 11.58±2.06 | 126.84±7.31 | |
30%ASIR-V | 136.75±16.69 | 100.77±5.39 | −974.18±6.81 | 66.08±11.35 | 1.35±0.09 | 17.11±2.59 | |
50%ASIR-V | 143.89±3.42 | 96.44±6.86 | −982.99±3.47 | 51.68±6.57 | 1.50±0.07 | 22.03±2.65 | |
80%ASIR-V | 144.64±20.62 | 54.30±7.09 | −992.57±2.55 | 37.76±6.85 | 2.67±0.27 | 30.73±5.15 | |
FBP | 130.86±27.04 | 142.51±12.10 | −970.38±2.21 | 70.65±3.82 | 0.91±0.13 | 15.61±0.60 | |
90 | DLIR-L | 127.10±8.85 | 20.00±4.38 | −1,005.48±3.08 | 19.03±1.99 | 6.55±1.36 | 60.01±6.91 |
DLIR-M | 129.49±10.05 | 17.47±0.23 | −1,004.78±2.90 | 14.01±2.13 | 7.42±0.65 | 82.32±13.43 | |
DLIR-H | 128.14±9.59 | 10.89±3.69 | −1,004.33±2.78 | 7.99±0.50 | 12.73±4.27 | 142.18±9.96 | |
30%ASIR-V | 140.05±5.83 | 98.06±17.89 | −977.86±4.46 | 58.36±6.77 | 1.45±0.20 | 19.34±2.31 | |
50%ASIR-V | 139.94±18.58 | 75.11±17.85 | −981.30±5.79 | 53.50±6.57 | 1.90±0.28 | 21.18±2.79 | |
80%ASIR-V | 140.33±20.82 | 48.32±4.45 | −993.23±0.29 | 36.89±3.60 | 2.91±0.37 | 30.93±3.15 | |
FBP | 148.58±15.07 | 128.82±16.39 | −973.46±3.32 | 65.49±3.69 | 1.16±0.05 | 17.16±0.79 | |
100 | DLIR-L | 130.30±10.41 | 31.39±7.70 | −1,003.58±1.73 | 20.10±1.86 | 4.39±1.52 | 56.77±5.72 |
DLIR-M | 130.29±10.56 | 22.84±4.97 | −936.01±8.93 | 13.83±1.12 | 6.00±2.00 | 76.29±11.36 | |
DLIR-H | 129.35±11.64 | 11.22±2.04 | −1,004.52±1.63 | 8.25±0.83 | 11.95±3.54 | 138.48±14.36 | |
30%ASIR-V | 131.14±10.05 | 110.53±33.36 | −980.39±2.91 | 56.74±2.65 | 1.27±0.42 | 19.62±1.01 | |
50%ASIR-V | 139.49±10.66 | 106.23±24.79 | −985.36±0.81 | 49.65±0.74 | 1.39±0.47 | 22.66±0.55 | |
80%ASIR-V | 149.41±12.31 | 69.22±21.32 | −991.70±3.29 | 36.84±2.52 | 2.29±0.68 | 31.06±1.99 | |
FBP | 147.03±5.95 | 160.67±47.45 | −972.42±3.41 | 67.85±4.38 | 0.96±0.25 | 16.55±1.21 | |
120 | DLIR-L | 133.49±5.21 | 22.91±0.88 | −1,004.85±4.49 | 16.23±1.81 | 5.84±0.41 | 70.76±8.14 |
DLIR-M | 132.21±6.44 | 16.87±2.19 | −1,002.74±4.76 | 12.48±0.89 | 7.91±0.96 | 91.26±6.75 | |
DLIR-H | 133.11±5.52 | 11.57±2.85 | −1,003.72±4.49 | 6.54±0.40 | 12.15±3.92 | 174.28±10.47 | |
30%ASIR-V | 135.42±11.56 | 77.03±13.92 | −981.61±0.96 | 51.03±1.63 | 1.78±0.17 | 21.90±0.71 | |
50%ASIR-V | 133.35±9.09 | 60.87±20.50 | −986.43±1.64 | 44.55±2.51 | 2.36±0.77 | 25.20±1.61 | |
80%ASIR-V | 139.51±10.50 | 35.79±13.23 | −994.70±3.39 | 33.43±2.64 | 4.25±1.46 | 34.08±2.93 | |
FBP | 148.56±22.65 | 130.11±22.93 | −966.88±2.71 | 72.17±1.84 | 1.15±0.18 | 15.46±0.50 | |
150 | DLIR-L | 131.75±3.45 | 18.11±1.65 | −1,004.91±1.60 | 15.37±1.36 | 7.32±0.71 | 74.35±6.52 |
DLIR-M | 131.34±3.61 | 13.54±1.24 | −1,004.90±1.72 | 11.48±2.15 | 9.70±0.74 | 101.25±18.17 | |
DLIR-H | 130.73±4.88 | 9.02±2.11 | −1,004.90±1.03 | 6.87±0.46 | 15.00±0.22 | 165.72±11.06 | |
30%ASIR-V | 135.30±2.15 | 77.84±9.19 | −984.25±3.60 | 51.09±5.16 | 1.76±0.22 | 22.06±2.21 | |
50%ASIR-V | 135.73±11.27 | 77.65±25.70 | −988.34±1.22 | 45.21±3.97 | 1.88±0.61 | 24.99±2.27 | |
80%ASIR-V | 137.77±5.41 | 49.04±29.64 | −994.82±3.60 | 35.75±4.30 | 3.46±1.62 | 32.01±4.09 | |
FBP | 146.16±12.18 | 108.91±18.26 | −976.30±5.48 | 62.70±6.38 | 1.36±0.19 | 18.02±1.74 |
SNs, solid nodules; SD, standard deviation; SNR, signal-to-noise ratio; CNR, contrast-to-noise ratio; FBP, filtered back projection; ASIR-V, adaptive statistical iterative reconstruction; DLIR-L/M/H, deep learning image reconstruction-low/medium/high.
Compared with ASIR-V and FBP, DLIR significantly reduced the SD value (in HU) of GGNs [DLIR (low, medium and high): 38.49±12.66, 36.51±10.88, 33.11±11.17; ASIR-V (30%, 50% and 80%): 106.65±27.92, 94.41±20.00, 65.24±19.62; FBP: 136.90±26.93]. Meanwhile, SNR and CNR were increased (for SNR, DLIR (low, medium and high): 21.18±8.00, 21.76±7.37, 24.81±10.01; ASIR-V (30%, 50% and 80%): 7.62±3.23, 8.14±2.19, 12.31±4.51; FBP: 5.61±1.56; for CNR, DLIR (low, medium and high): 12.99±6.08, 18.17±8.44, 31.84±14.86; ASIR-V (30%, 50% and 80%): 3.84±1.93, 4.74±2.08, 6.22±3.00; FBP: 3.18±1.41). Different degrees of DLIR, ASIR-V and FBP had different effects on the SD value (in HU) of SNs [DLIR (low, medium and high): 27.48±8.01, 21.06±5.82, 13.15±4.65; ASIR-V (30%, 50% and 80%): 96.70±20.44, 84.27±25.53, 55.13±16.39; FBP: 132.23±26.51]. The overall SNR and CNR of GGNs and SNs were significantly higher and SD value significantly lower with DLIR reconstruction algorithm than with ASIR-V at all dose levels (Figure 3).
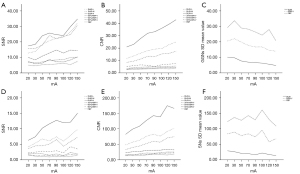
Discussion
In this study, we evaluated the influence of three types of reconstruction algorithms (FBP, ASIR-V, DLIR) on the image quality, detection rate and characterization of pulmonary nodules using AI software analysis in ultra-low dose chest CT using a lung nodule phantom. The results showed that under a wide range of dose levels, DLIR significantly reduced image noise and provided better overall image quality score compared with FBP and ASIR-V algorithms. At ultra-low dose conditions (30 mA), DLIR-H had the highest PNs detection rate. In addition, DLIR-M had a minimal negative effect on PNs characterization. Therefore, our results suggest that DLIR can provide the best noise reduction and the highest SNR, while ensuring the image quality and satisfying the diagnostic requirements, and better make up for the deficiencies caused by low-dose conditions.
ASIR-V is a new generation iterative reconstruction algorithm that contains more advanced noise modelling and object modelling than the previous version ASIR. ASIR-V has also added some physics modelling (9) to maintain image quality in low dose scans with low tube voltage and tube current. However, with the increase of ASIR-V level, there is a potential risk of creating ‘wax-like’ artifacts while providing deep image noise reduction (9,14). DLIR builds a new image chain through years of clinical application experience of FBP and model-based iterative reconstruction (MBIR), reducing noise without changing the image texture, and improving the spatial resolution and the detection of lesions (25-27). Therefore, DLIR is expected to outperform ASIR-V with better dose performance and image quality. As expected, our study results showed that the images reconstructed with DLIR exhibited the best detection rate and minimal negative effect on the characterization of PNs.
Our study showed that DLIR algorithm was used to achieve image noise reduction under the ultra-low dose, and we found that the difference of image reconstruction effects between DLIR-L and 30% ASIR-V was higher than that between DLIR-H and 80% ASIR-V, and the degree of lesion edge texture change of DLIR was also lower than that of ASIR-V. In the subjective rating of image quality, the image reconstructed by DLIR-H achieved more satisfactory results, and we found that the greatest image noise reduction with DLIR-H did not affect physicians’ judgment and assessment of nodules, especially the nodules with diameter of >8 mm.
As for the detection of nodules, we found that when mA was greater than 70mA, there was no difference in the detection results of nodules between DLIR and ASIR-V (9/9, 100%), probably due to the sufficient X-ray signals and the high contrast between the nodules and the background. At 50 and 70 mA, DLIR could achieve 88.89% nodule detection (8/9). A surprising finding was that at 30 mA, DLIR-H achieved a 100% detection rate. When the radiation dose was too low (20 mA), no reconstruction method could improve the detection of nodules. Therefore, we should pay attention to the dose reduction and maintain sufficient dosage in clinical application.
Regarding of image quality, we found that under the condition of ultra-low dose, such as 20mA, the clarity of displaying GGNs under the DLIR reconstruction was higher than FBP and ASIR-V algorithms, which could be attributed to the better ability of DLIR in separating signal and noise under ultra-low dose conditions. This study also showed that the deformation degree (long diameter/short diameter) of nodules in DLIR reconstructed images was the smallest with the nodule shape closer to the standard spherical nodule than that of the ASIR-V and FBP, and we believe it might be an indicator that DLIR has less impact on image texture while reducing image noise under low dose conditions (DD with DLIR-L, M, H: 1.08, 1.07, 1.08; ASIR-V-30%, 50%, 80%: 1.12, 1.09, 1.08; FBP: 1.10, respectively).
This study has the following limitations: first, it is believed that pulmonary nodules less than 5mm have a poor correlation with lung cancer (28,29), our study only analyzed nodules with a diameter greater than or equal to 8 mm, and did not discuss nodules with a smaller diameter, which may lead to positive bias in the results; second, the study used only phantom images which have higher contrast between the nodules and lung parenchyma than in patient images. The performance in simple phantoms may not fully represent how it performs in complex patient imaging. We will perform an image quality study on clinical patients by DLIR in the following work; third, at 30 mA, DD of PNs was lower than 50 mA, which was considered to be caused by the limited spatial resolution of the edge of nodules due to low dose and the small sample size. However, with the increase of mA, DD generally showed a downward trend; fourth, only one scan was performed in our study without repeated acquisition which could be another limitation of our study. In order to make up for this limitation to the greatest extent, this study had taken each single nodule as the study object to increase sample size. Of course, this did not change the fact that the sample size was small.
In conclusion, DLIR algorithm further optimizes image quality and improves the detection of PNs with minimum negative effect on the characterization of lesions in ultra-low dose conditions, in comparison with ASIR-V and FBP algorithms. DLIR algorithm can be a potential reconstruction technique in ultra-low dose lung PNs screening and DLIR-M is recommended for screening pulmonary nodules.
Acknowledgments
We would like to thank Youren Wang for taking part in this experiment. We also would like to thank Dr. Xuan Zhang for her help in generating DLIR images. At the same time, we also thank Mr. Michael Li (a native English speaker) for his help in the proofreading of this paper.
Funding: None.
Footnote
Reporting Checklist: The authors have completed the STARD reporting checklist. Available at https://qims.amegroups.com/article/view/10.21037/qims-21-815/rc
Conflicts of Interest: All authors have completed the ICMJE uniform disclosure form (available at https://qims.amegroups.com/article/view/10.21037/qims-21-815/coif). JL is an employee of GE Healthcare, the manufacturer of the CT system used in this study. The other authors have no conflicts of interest to declare.
Ethical Statement: The authors are accountable for all aspects of the work in ensuring that questions related to the accuracy or integrity of any part of the work are appropriately investigated and resolved. The research object of this study is a phantom. Ethics committee approval and informed consent are not required.
Open Access Statement: This is an Open Access article distributed in accordance with the Creative Commons Attribution-NonCommercial-NoDerivs 4.0 International License (CC BY-NC-ND 4.0), which permits the non-commercial replication and distribution of the article with the strict proviso that no changes or edits are made and the original work is properly cited (including links to both the formal publication through the relevant DOI and the license). See: https://creativecommons.org/licenses/by-nc-nd/4.0/.
References
- Bray F, Ferlay J, Soerjomataram I, Siegel RL, Torre LA, Jemal A. Global cancer statistics 2018: GLOBOCAN estimates of incidence and mortality worldwide for 36 cancers in 185 countries. CA Cancer J Clin 2018;68:394-424. [Crossref] [PubMed]
- Siegel RL, Miller KD, Jemal A. Cancer statistics, 2019. CA Cancer J Clin 2019;69:7-34. [Crossref] [PubMed]
- Naruke T, Goya T, Tsuchiya R, Suemasu K. Prognosis and survival in resected lung carcinoma based on the new international staging system. J Thorac Cardiovasc Surg 1988;96:440-7. [Crossref] [PubMed]
- Aberle DR, Adams AM, Berg CD, Black WC, Clapp JD, Fagerstrom RM, Gareen IF, Gatsonis C, Marcus PM, Sicks JD. Reduced lung-cancer mortality with low-dose computed tomographic screening. N Engl J Med 2011;365:395-409. [Crossref] [PubMed]
- Aberle DR, DeMello S, Berg CD, Black WC, Brewer B, Church TR, Clingan KL, Duan F, Fagerstrom RM, Gareen IF, Gatsonis CA, Gierada DS, Jain A, Jones GC, Mahon I, Marcus PM, Rathmell JM, Sicks JNational Lung Screening Trial Research Team. Results of the two incidence screenings in the National Lung Screening Trial. N Engl J Med 2013;369:920-31. [Crossref] [PubMed]
- de Koning HJ, Meza R, Plevritis SK, ten Haaf K, Munshi VN, Jeon J, Erdogan SA, Kong CY, Han SS, van Rosmalen J, Choi SE, Pinsky PF, Berrington de Gonzalez A, Berg CD, Black WC, Tammemägi MC, Hazelton WD, Feuer EJ, McMahon PM. Benefits and harms of computed tomography lung cancer screening strategies: a comparative modeling study for the U.S. Preventive Services Task Force. Ann Intern Med 2014;160:311-20. [Crossref] [PubMed]
- McCollough CH, Primak AN, Braun N, Kofler J, Yu L, Christner J. Strategies for reducing radiation dose in CT. Radiol Clin North Am 2009;47:27-40. [Crossref] [PubMed]
- Geyer LL, Schoepf UJ, Meinel FG, Nance JW Jr, Bastarrika G, Leipsic JA, Paul NS, Rengo M, Laghi A, De Cecco CN. State of the Art: Iterative CT Reconstruction Techniques. Radiology 2015;276:339-57. [Crossref] [PubMed]
- De Marco P, Origgi D. New adaptive statistical iterative reconstruction ASiR-V: Assessment of noise performance in comparison to ASiR. J Appl Clin Med Phys 2018;19:275-86. [Crossref] [PubMed]
- Fleischmann D, Boas FE. Computed tomography--old ideas and new technology. Eur Radiol 2011;21:510-7. [Crossref] [PubMed]
- Fält T, Söderberg M, Hörberg L, Christoffersen C, Lång K, Abul-Kasim K, Leander P. Simulated Dose Reduction for Abdominal CT With Filtered Back Projection Technique: Effect on Liver Lesion Detection and Characterization. AJR Am J Roentgenol 2019;212:84-93. [Crossref] [PubMed]
- Singh S, Kalra MK, Hsieh J, Licato PE, Do S, Pien HH, Blake MA. Abdominal CT: comparison of adaptive statistical iterative and filtered back projection reconstruction techniques. Radiology 2010;257:373-83. [Crossref] [PubMed]
- Padole A, Ali Khawaja RD, Kalra MK, Singh S. CT radiation dose and iterative reconstruction techniques. AJR Am J Roentgenol 2015;204:W384-92. [Crossref] [PubMed]
- Tang H, Yu N, Jia Y, Yu Y, Duan H, Han D, Ma G, Ren C, He T. Assessment of noise reduction potential and image quality improvement of a new generation adaptive statistical iterative reconstruction (ASIR-V) in chest CT. Br J Radiol 2018;91:20170521. [Crossref] [PubMed]
- Benz DC, Gräni C, Mikulicic F, Vontobel J, Fuchs TA, Possner M, Clerc OF, Stehli J, Gaemperli O, Pazhenkottil AP, Buechel RR, Kaufmann PA. Adaptive Statistical Iterative Reconstruction-V: Impact on Image Quality in Ultralow-Dose Coronary Computed Tomography Angiography. J Comput Assist Tomogr 2016;40:958-63. [Crossref] [PubMed]
- Verdun FR, Racine D, Ott JG, Tapiovaara MJ, Toroi P, Bochud FO, Veldkamp WJH, Schegerer A, Bouwman RW, Giron IH, Marshall NW, Edyvean S. Image quality in CT: From physical measurements to model observers. Phys Med 2015;31:823-43. [Crossref] [PubMed]
- Willemink MJ, Noël PB. The evolution of image reconstruction for CT-from filtered back projection to artificial intelligence. Eur Radiol 2019;29:2185-95. [Crossref] [PubMed]
- Sun J, Li H, Li J, Yu T, Li M, Zhou Z, Peng Y. Improving the image quality of pediatric chest CT angiography with low radiation dose and contrast volume using deep learning image reconstruction. Quant Imaging Med Surg 2021;11:3051-8. [Crossref] [PubMed]
- Sun J, Li H, Li J, Cao Y, Zhou Z, Li M, Peng Y. Performance evaluation of using shorter contrast injection and 70 kVp with deep learning image reconstruction for reduced contrast medium dose and radiation dose in coronary CT angiography for children: a pilot study. Quant Imaging Med Surg 2021;11:4162-71. [Crossref] [PubMed]
- Wu D, Kim K, Li Q. Computationally efficient deep neural network for computed tomography image reconstruction. Med Phys 2019;46:4763-76. [Crossref] [PubMed]
- Li Y, Jiang Y, Liu H, Yu X, Chen S, Ma D, Gao J, Wu Y. A phantom study comparing low-dose CT physical image quality from five different CT scanners. Quant Imaging Med Surg 2022;12:766-80. [Crossref] [PubMed]
- Hsieh J, Liu E, Nett B, Tang J, Thibault JB, Sahney S. A new era of image reconstruction: TrueFidelity™. Technical white paper on deep learning image reconstruction. 2019 GE Healthcare.
- Fu B, Wang G, Wu M, Li W, Zheng Y, Chu Z, Lv F. Influence of CT effective dose and convolution kernel on the detection of pulmonary nodules in different artificial intelligence software systems: A phantom study. Eur J Radiol 2020;126:108928. [Crossref] [PubMed]
- Setio AA, Jacobs C, Gelderblom J, van Ginneken B. Automatic detection of large pulmonary solid nodules in thoracic CT images. Med Phys 2015;42:5642-53. [Crossref] [PubMed]
- Jensen CT, Liu X, Tamm EP, Chandler AG, Sun J, Morani AC, Javadi S, Wagner-Bartak NA. Image Quality Assessment of Abdominal CT by Use of New Deep Learning Image Reconstruction: Initial Experience. AJR Am J Roentgenol 2020;215:50-7. [Crossref] [PubMed]
- Greffier J, Hamard A, Pereira F, Barrau C, Pasquier H, Beregi JP, Frandon J. Image quality and dose reduction opportunity of deep learning image reconstruction algorithm for CT: a phantom study. Eur Radiol 2020;30:3951-9. [Crossref] [PubMed]
- Akagi M, Nakamura Y, Higaki T, Narita K, Honda Y, Zhou J, Yu Z, Akino N, Awai K. Deep learning reconstruction improves image quality of abdominal ultra-high-resolution CT. Eur Radiol 2019;29:6163-71. [Crossref] [PubMed]
- Wood DE, Kazerooni EA, Baum SL, Eapen GA, Ettinger DS, Hou L, et al. Lung Cancer Screening, Version 3.2018, NCCN Clinical Practice Guidelines in Oncology. J Natl Compr Canc Netw 2018;16:412-41. [Crossref] [PubMed]
- Gierada DS, Pinsky P, Nath H, Chiles C, Duan F, Aberle DR. Projected outcomes using different nodule sizes to define a positive CT lung cancer screening examination. J Natl Cancer Inst 2014;106:dju284. [Crossref] [PubMed]