Deep learning reconstruction allows low-dose imaging while maintaining image quality: comparison of deep learning reconstruction and hybrid iterative reconstruction in contrast-enhanced abdominal CT
Introduction
Reconstruction algorithms based on machine deep learning are increasingly being implemented in routine clinical practice (1-8). Deep learning reconstruction (DLR) has been shown to reduce image noise and improve the contrast-to-noise ratio (CNR) with shorter reconstruction times than model-based iterative reconstruction (MBIR) (1-4). A deep convolutional neural network is used by the first commercially available DLR tool for computed tomography (CT) [advanced intelligent clear-IQ engine (AiCE)] to recognize true signals amongst the noise in an image. This deep learning algorithm is trained to provide a high-dose MBIR image from the corresponding data at 12.5–75% of the dose of the target image, and during the training process, the discriminating statistical features that identify signals separately from the noise and artifacts are learned (9-11). Previous studies have shown that DLR has better image quality characteristics than filtered back projection (FBP) or MBIR under the same conditions, when performing low-dose abdominal CT imaging (3,12). However, in actual daily clinical practice, hybrid iterative reconstruction (hybrid-IR) is often used, and the clinical usefulness of DLR should be compared with hybrid-IR. In the future, DLR may replace hybrid-IR as the mainstay; however, DLR must be able to reduce radiation exposure and while guaranteeing lesion detectability. Evaluation of diagnostic accuracy in low-contrast tasks in hepatic enlargement differs from evaluation in high-contrast tasks (e.g., detection of calcifications and pulmonary nodules), as a small change in radiation dose has a large impact on the detectability of low-contrast objects (13-18).
The aim of this study was to compare the radiation dose and corresponding image quality of abdominal-CT images reconstructed with DLR and hybrid-IR in order to determine the usefulness of DLR in routine clinical practice. We present the following article in accordance with the GRRAS reporting checklist (available at https://qims.amegroups.com/article/view/10.21037/qims-21-1216/rc).
Methods
Patients and study design
The study was conducted in accordance with the Declaration of Helsinki (as revised in 2013). The study design was approved by the institutional review board of Iwate Medical University (No. MH2019-093), and informed consent was waived due to the retrospective nature of the study. Between May 2020 and April 2021, 287 dynamic abdominal low-dose CT scans with AiCE were performed using a 320-row multidetector CT scanner (Aquilion ONE PRISM; Canon Medical Systems, Otawara, Japan) with suspected liver tumors. Of these, 75 patients underwent repeated dynamic abdominal routine-dose CT with adaptive iterative dose reduction 3D (AIDR 3D) on a 320-row multidetector CT scanner (Aquilion ONE GENESIS; Canon Medical Systems) within 3 months for close examination or follow-up. Patients under 20 years of age and patients for whom the body mass index (BMI) category (<20, 20–24.9, and ≥25) changed between examinations were excluded from the study. Patients who underwent surgery, transcatheter arterial chemoembolization, or drug treatment and those who had a liver injury during the study were also excluded.
CT technique
All examinations were performed with similar imaging parameters, except for noise level (Table 1). A standardized injection procedure was used across all patients. The antecubital vein was injected with contrast enhancement materials (Iohexol, Omnipaque, Daiichi-Sankyo, Tokyo, Japan; iomeprol, Iomeron, Eisai, Tokyo, Japan; or iopamidol, Iopamiron, Bayer Yakuhin, Osaka, Japan) using a 20-gauge catheter at a dose of 600 mgI/kg. The scan delays were determined using an automatic bolus-tracking program (Canon Medical Systems) for arterial and portal venous phase imaging. The region of interest (ROI) cursor was placed at the level of the diaphragmatic dome in the aorta. Once the trigger threshold of 100 HU was achieved, scanning for the arterial and portal venous phases began automatically at 20 and 60 s, respectively. However, the arterial phase images were not evaluated in this study.
Table 1
Parameter | AiCE | AIDR 3D |
---|---|---|
Tube voltage (kVp) | 120 | |
Tube current (mA) | Automatic exposure control | |
Noise level | 16 | 12 |
Beam collimation (mm) | 80×0.5 | |
Pitch | 0.813 | |
Rotation time (s) | 0.6 | |
Slice thickness and interval (mm) | 3 |
CT, computed tomography; AiCE, advanced intelligent clear-IQ engine; AIDR 3D, adaptive iterative dose reduction 3D.
Estimation of patient radiation dose
For each examination, the volume CT dose index (CTDIvol) and dose-length product (DLP) were recorded from the dose reports generated by the scanner. The radiation effective dose (ED) was calculated as the DLP multiplied by an abdominal-specific correction coefficient (0.015 mSv/mGy × cm) (19). The method described in the American Association of Physicists in Medicine Task Group Report 204 was used to calculate the size-specific dose estimate (SSDE) for all examinations, and the scout CT images was used to measure the transverse and anteroposterior diameters of each patient (19).
Objective assessment of image quality
For each patient, the mean attenuation of the hepatic parenchyma and bilateral erector spinae muscles within circular ROIs were measured by a medical student (IN, 3rd year student) under the supervision of a CT technologist (YO, with 18 years of experience in abdominal CT). Each ROI area was set to approximately 150 mm2. Hepatic attenuation was measured in the portal venous phase in three separate areas (the left lobe and the anterior and posterior segments of the right lobe) at the level of the main portal vein. Areas with large vessels, focal changes in parenchymal density, and prominent artifacts were avoided. Attenuation of the two erector spinae muscles was measured in the portal venous phases avoiding the areas of macroscopic fat infiltration. The standard deviation (SD) of the attenuation value of the hepatic parenchyma and the bilateral erector spinae muscles was defined as the image noise. The CNR of the abdominal aorta was calculated by subtracting the mean attenuation of the ROI of the erector spinae muscle from that of the ROI of the hepatic parenchyma and dividing this difference by the image noise of the erector spinae muscle.
Subjective assessment of image quality
Two blinded radiologists (EM and AT with 3 and 13 years of experience in abdominal CT, respectively) were randomly presented the two image sets. Standard abdominal window settings (width, 300 HU; level, 50 HU) were used to view the images. The radiologists independently graded the quality of portal phase axial CT images using a five-point Likert scale for image noise, artifacts, sharpness, and diagnostic acceptability as follows: 1, unacceptable; 2, suboptimal; 3, average; 4, above average; and 5, excellent. Patients who were included in the study, with or without hepatocellular carcinoma (HCC) nodules, were evaluated for image noise, artifacts, and sharpness. Patients with HCC >1 cm were evaluated for diagnostic acceptability. One attending radiologist with 15 years of clinical experience in abdominal CT interpretation reviewed the study patients’ medical records and radiology information for confirmation of the lesion. All HCC nodules >1 cm in diameter were diagnosed through careful image review according to the Liver Imaging Reporting and Data System (LI-RADS; version 2018), which includes a combination of arterial enhancement and washout on portal and delayed phase dynamic CT images (20). The observers assessed the diagnostic acceptability of the HCC lesions after the patients were informed of the number and location of the lesions. Prior to image quality assessment, the radiologists underwent a training session including abdominal CT images from 10 patients to get familiar with the scoring system.
Statistical analysis
Patients were divided into three BMI categories (<20, 20–24.9, and ≥25) to compare the radiation dose and image quality. All numerical values are reported as mean ± SD. We used a paired t-test to compare the quantitative image analysis data. Differences in the qualitative analysis were compared using the Wilcoxon signed-rank test. Kappa statistics for the two readers’ assessments of image quality were calculated. Statistical analysis was performed using the SPSS software (version 24; IBM Corp., Armonk, NY, USA). Statistical significance was set at P<0.05.
Results
Patients
Overall, 71 patients [61 men and 10 women; average age, 71.9 years (range, 44–87 years); mean body weight, 65.2 kg (range, 41–100 kg); mean height, 163.4 cm (range, 146–181 cm); mean BMI, 24.3 kg/m2 (range, 17.8–32.2 kg/m2)] who underwent both low-dose CT with AiCE and routine-dose CT with AIDR 3D served as the study group. Of the 71 patients, 34 had a total of 45 HCCs >1 cm.
Radiation dose
Overall, the AiCE protocol allowed reduction of CTDIvol by 44.3%, ED by 43.7%, and SSDE by 44.9% compared to AIDR 3D (Table 2). All radiation dose parameters were significantly lower for the AiCE protocol compared with those for the AIDR 3D scans for the entire population as well as when the patients were divided according to their BMI category (P<0.001).
Table 2
Parameter (mean ± SD) | All patients | BMI <20 (n=7) | BMI 20–24.9 (n=37) | BMI ≥25 (n=27) | |||||||||||
---|---|---|---|---|---|---|---|---|---|---|---|---|---|---|---|
AiCE | AIDR 3D | Dose reduction (%) | AiCE | AIDR 3D | Dose reduction (%) | AiCE | AIDR 3D | Dose reduction (%) | AiCE | AIDR 3D | Dose reduction (%) | ||||
CTDIvol (mGy) | 10.8±3.9 | 19.4±5.6 | 44.3 | 6.9±1.1 | 11.8±2.3 | 41.5 | 8.7±2.1 | 16.8±3.1 | 48.2 | 14.7±2.9 | 25.0±3.5 | 41.2 | |||
ED (mSv) | 4.9±2.0 | 8.7±3.0 | 43.7 | 3.1±0.7 | 5.1±1.4 | 39.2 | 3.8±1.0 | 7.3±1.6 | 47.9 | 6.9±2.4 | 11.6±2.1 | 40.5 | |||
SSDE (mGy) | 14.6±4.1 | 26.5±5.7 | 44.9 | 10.5±1.2 | 18.4±2.7 | 42.9 | 12.5±2.4 | 24.0±3.4 | 47.9 | 18.7±2.9 | 32.0±3.3 | 41.6 |
AiCE, advanced intelligent clear-IQ engine; AIDR 3D, adaptive iterative dose reduction 3D; SD, standard deviation; BMI, body mass index; CTDIvol, volume CT dose index; ED, effective dose; SSDE, size-specific dose estimate.
Objective assessment of image quality
The mean image noise and CNR for the low-dose AiCE and standard-dose AIDR 3D protocols are presented in Table 3. In all patients, AiCE had significantly lower noise in the erector spinae muscles and liver parenchyma, and higher CNR in the liver parenchyma than AIDR 3D (P<0.001). Results for image noise varied when analyzed per BMI category. For all BMI categories, the CNR of the liver parenchyma was significantly higher in AiCE than in AIDR 3D (P≤0.001).
Table 3
Parameter | All patients | BMI <20 (n=7) | BMI 20–24.9 (n=37) | BMI ≥25 (n=27) | |||||||||||
---|---|---|---|---|---|---|---|---|---|---|---|---|---|---|---|
AiCE | AIDR 3D | P* | AiCE | AIDR 3D | P* | AiCE | AIDR 3D | P* | AiCE | AIDR 3D | P* | ||||
Image noise (mean ± SD) | |||||||||||||||
Erector spinae muscles | 11.7±6.3 | 12.9±6.6 | <0.001 | 9.5±1.7 | 11.6±3.4 | 0.028 | 10.8±6.2 | 11.7±6.5 | 0.002 | 13.6±6.8 | 14.8±7.0 | 0.054 | |||
Hepatic parenchyma | 9.2±0.9 | 9.8±0.9 | <0.001 | 8.9±0.8 | 9.2±0.7 | 0.391 | 8.9±0.8 | 9.5±0.7 | <0.001 | 9.6±1.0 | 10.4±0.7 | <0.001 | |||
CNR (mean ± SD) | |||||||||||||||
Hepatic parenchyma | 5.0±1.5 | 4.3±1.3 | <0.001 | 5.3±1.5 | 4.3±0.9 | 0.033 | 5.3±1.3 | 4.7±1.3 | 0.001 | 4.4±1.5 | 3.7±1.2 | <0.001 |
*, statistical comparison using t-test. CNR, contrast-to-noise ratio; AiCE, advanced intelligent clear-IQ engine; AIDR 3D, adaptive iterative dose reduction 3D; BMI, body mass index; SD, standard deviation.
Subjective assessment of image quality
Table 4 shows the results of the subjective image quality assessments. On a five-point Likert scale, there was no significant difference in image noise between AiCE and AIDR 3D overall, however, AiCE was significantly superior in the subgroup with BMI ≥25 (P=0.034). The assessment of artifacts was not significantly different for the whole group or for each subgroup. For sharpness, AiCE was significantly better than AIDR 3D both in the whole group and in each subgroup (P<0.001–0.025). Overall, AiCE was superior to AIDR 3D in terms of diagnostic acceptability (P=0.001). Figures 1,2 show an example of noise reduction, sharpness, and diagnostic acceptability with AiCE and AIDR 3D. Between the two readers, there was substantial to almost perfect inter-observer agreement for all subjective image quality metrics (κ =0.66, 0.43, 0.71, and 0.82, for noise, artifacts, sharpness, and diagnostic acceptability, respectively).
Table 4
Parameter (mean ± SD) | All patients | BMI <20 (n=7) | BMI 20–24.9 (n=37) | BMI ≥25 (n=27) | |||||||||||
---|---|---|---|---|---|---|---|---|---|---|---|---|---|---|---|
AiCE | AIDR 3D | P* | AiCE | AIDR 3D | P* | AiCE | AIDR 3D | P* | AiCE | AIDR 3D | P* | ||||
Noise | 4.0±0.5 | 3.9±0.4 | 0.239 | 4.1±0.3 | 3.8±0.7 | 0.157 | 4.0±0.3 | 3.9±0.4 | 0.206 | 4.0±0.5 | 3.9±0.5 | 0.034 | |||
Artifacts | 4.0±0.4 | 4.0±0.3 | 0.467 | 4.1±0.5 | 4.1±0.4 | 0.317 | 4.0±0.3 | 4.0±0.3 | 0.257 | 4.1±0.5 | 4.0±0.4 | 0.739 | |||
Sharpness | 4.1±0.5 | 3.9±0.5 | <0.001 | 3.9±0.7 | 3.5±0.5 | 0.025 | 4.2±0.5 | 4.0±0.4 | <0.001 | 4.1±0.4 | 3.9±0.5 | 0.008 | |||
Diagnostic acceptability | 3.9±0.7 | 3.7±0.6 | 0.001 | 4.3±0.4 | 3.9±0.3 | 0.104 | 4.1±0.5 | 3.9±0.5 | 0.003 | 3.4±0.7 | 3.3±0.6 | 0.375 |
*, statistical comparison using Wilcoxon signed-rank test. SD, standard deviation; BMI, body mass index; AiCE, advanced intelligent clear-IQ engine; AIDR 3D, adaptive iterative dose reduction 3D.
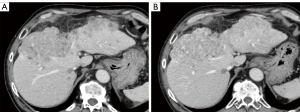
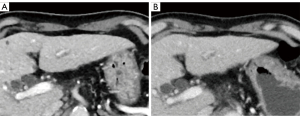
Discussion
In our retrospective study, we showed that the use of a low-dose protocol with AiCE can improve CNR compared to the standard AIDR 3D protocol, while improving image noise and significantly reducing radiation dose by more than 40%. In fact, all CNRs according to BMI category were higher with AiCE. Image noise was also well reduced using this AiCE protocol. Even though images were acquired with higher target noise levels, final image noise of the images reconstructed with AiCE was lower than for the routine clinical protocol acquired with lower target noise levels and were reconstructed with AIDR3D. Importantly, no subjective reduction in image quality was identified in the AiCE cohort. According to literature, when patients are grouped according to BMI, AIDR 3D-reconstructed images show large variability in terms of radiation dose reduction and quantitative image quality assessment (15); however, our study results do not support this. This may have been due to technical improvements in the AIDR 3D by the vendor. Previous reports have shown that when comparing AiCE and MBIR, the noise reduction effect of vendor-specific MBIR is reduced as the body size increases, whereas AiCE has a consistent noise reduction effect, regardless of body size (3). Similarly, for the images reconstructed with AiCE in the current study, no significant variation was observed in terms of radiation dose reduction and quantitative image quality assessment in the groups classified by BMI. In abdominal CT images, subtle low-contrast lesions in the parenchymal organs may get obscured due to high image noise, increasing the risk of missing liver tumors (13,17,21-23). CNR is necessary to delineate small tumors with low contrast. An important finding of this study is that, despite the inevitable decrease in CNR due to photon starvation in larger patients, AiCE decreased more slowly than AIDR 3D.
In many medical institutions, dynamic CT plays an important role in the imaging diagnosis of HCC. In LI-RADS, in addition to arterial phase hyperenhancement, washout appearance (a visual assessment in which liver enhancement decreases over time from early to late phases, resulting in portal hypo-enhancement or delayed phase hypo-enhancement) is also evaluated in tumor diagnosis (20). The washout appearance of HCC is related to the degree of tumor differentiation. In this study, not only were subjective measures such as image noise, artifacts, and sharpness equal to or partially superior to AIDR 3D, but there was also a significant difference in the delineation of HCC in the portal phase despite significant light reduction under AiCE use. This information is important in the routine clinical use of DLR. In addition, a substantial noise reduction might be associated with an over smoothing of the image leading to a blotchy appearance with IR algorithms in particular (14,24), but such an event has not been reported in DLR (3-9,25).
This study has several limitations. First, the sample size was relatively small, and the retrospective study was conducted using a single cohort during various temporal phases at one institution. Second, because of the low prevalence of HCC in both groups, the assessment of the potential diagnostic superiority of DLR over hybrid-IR was not considered to be appropriate. Third, in our study, the mean BMI was lower than the global average. Finally, the present comparative study was conducted between two reconstruction algorithms (AIDR 3D and AiCE) from the same manufacturer; hence, to generalize the findings to other vendors may not be possible. Recently, GE Healthcare has developed the DRL algorithm “TrueFidelity” and has reported on their image evaluation (5,8). Noda et al. (8) reported that TrueFidelity improves the image quality through noise reduction and improved CNR on pancreatic low-dose CT compared with hybrid-IR. Although DLR appears to be a promising technology, the methods still constitute an upcoming field of technology; hence, this study could be considered preliminary. Therefore, further investigation is required.
In conclusion, our study shows that abdominal CT examinations with AiCE can reduce the overall radiation dose by more than 40% without loss of image quality compared to routine-dose CT examinations with AIDR 3D.
Acknowledgments
Funding: This work was supported by JSPS KAKENHI (19K08160) and Regional Medical Research of Iwate.
Footnote
Reporting Checklist: The authors have completed the GRRAS reporting checklist. Available at https://qims.amegroups.com/article/view/10.21037/qims-21-1216/rc
Conflicts of Interest: All authors have completed the ICMJE uniform disclosure form (available at https://qims.amegroups.com/article/view/10.21037/qims-21-1216/coif). KA is an employee of Canon Medical Systems Corporation. The other authors have no conflicts of interest to declare.
Ethical Statement: The authors are accountable for all aspects of the work and assure that questions related to the accuracy or integrity of any part of the work will be appropriately investigated and resolved. The study was conducted in accordance with the Declaration of Helsinki (as revised in 2013). This retrospective study was approved by the institutional review board of Iwate Medical University (No. MH2019-093), and the requirement for informed consent was waived.
Open Access Statement: This is an Open Access article distributed in accordance with the Creative Commons Attribution-NonCommercial-NoDerivs 4.0 International License (CC BY-NC-ND 4.0), which permits the non-commercial replication and distribution of the article with the strict proviso that no changes or edits are made and the original work is properly cited (including links to both the formal publication through the relevant DOI and the license). See: https://creativecommons.org/licenses/by-nc-nd/4.0/.
References
- Nam JG, Hong JH, Kim DS, Oh J, Goo JM. Deep learning reconstruction for contrast-enhanced CT of the upper abdomen: similar image quality with lower radiation dose in direct comparison with iterative reconstruction. Eur Radiol 2021;31:5533-43. [Crossref] [PubMed]
- Park C, Choo KS, Jung Y, Jeong HS, Hwang JY, Yun MS. CT iterative vs deep learning reconstruction: comparison of noise and sharpness. Eur Radiol 2021;31:3156-64. [Crossref] [PubMed]
- Tamura A, Mukaida E, Ota Y, Kamata M, Abe S, Yoshioka K. Superior objective and subjective image quality of deep learning reconstruction for low-dose abdominal CT imaging in comparison with model-based iterative reconstruction and filtered back projection. Br J Radiol 2021;94:20201357. [Crossref] [PubMed]
- Brady SL, Trout AT, Somasundaram E, Anton CG, Li Y, Dillman JR. Improving Image Quality and Reducing Radiation Dose for Pediatric CT by Using Deep Learning Reconstruction. Radiology 2021;298:180-8. [Crossref] [PubMed]
- Jensen CT, Liu X, Tamm EP, Chandler AG, Sun J, Morani AC, Javadi S, Wagner-Bartak NA. Image Quality Assessment of Abdominal CT by Use of New Deep Learning Image Reconstruction: Initial Experience. AJR Am J Roentgenol 2020;215:50-7. [Crossref] [PubMed]
- Narita K, Nakamura Y, Higaki T, Akagi M, Honda Y, Awai K. Deep learning reconstruction of drip-infusion cholangiography acquired with ultra-high-resolution computed tomography. Abdom Radiol (NY) 2020;45:2698-704. [Crossref] [PubMed]
- Akagi M, Nakamura Y, Higaki T, Narita K, Honda Y, Zhou J, Yu Z, Akino N, Awai K. Deep learning reconstruction improves image quality of abdominal ultra-high-resolution CT. Eur Radiol 2019;29:6163-71. [Crossref] [PubMed]
- Noda Y, Iritani Y, Kawai N, Miyoshi T, Ishihara T, Hyodo F, Matsuo M. Deep learning image reconstruction for pancreatic low-dose computed tomography: comparison with hybrid iterative reconstruction. Abdom Radiol (NY) 2021;46:4238-44. [Crossref] [PubMed]
- Nakamura Y, Higaki T, Tatsugami F, Honda Y, Narita K, Akagi M, Awai K. Possibility of Deep Learning in Medical Imaging Focusing Improvement of Computed Tomography Image Quality. J Comput Assist Tomogr 2020;44:161-7. [Crossref] [PubMed]
- Higaki T, Nakamura Y, Zhou J, Yu Z, Nemoto T, Tatsugami F, Awai K. Deep Learning Reconstruction at CT: Phantom Study of the Image Characteristics. Acad Radiol 2020;27:82-7. [Crossref] [PubMed]
- Lenfant M, Chevallier O, Comby PO, Secco G, Haioun K, Ricolfi F, Lemogne B, Loffroy R. Deep Learning Versus Iterative Reconstruction for CT Pulmonary Angiography in the Emergency Setting: Improved Image Quality and Reduced Radiation Dose. Diagnostics (Basel) 2020;10:558. [Crossref] [PubMed]
- Singh R, Digumarthy SR, Muse VV, Kambadakone AR, Blake MA, Tabari A, Hoi Y, Akino N, Angel E, Madan R, Kalra MK. Image Quality and Lesion Detection on Deep Learning Reconstruction and Iterative Reconstruction of Submillisievert Chest and Abdominal CT. AJR Am J Roentgenol 2020;214:566-73. [Crossref] [PubMed]
- Schindera ST, Odedra D, Mercer D, Thipphavong S, Chou P, Szucs-Farkas Z, Rogalla P. Hybrid iterative reconstruction technique for abdominal CT protocols in obese patients: assessment of image quality, radiation dose, and low-contrast detectability in a phantom. AJR Am J Roentgenol 2014;202:W146-52. [Crossref] [PubMed]
- Geyer LL, Schoepf UJ, Meinel FG, Nance JW Jr, Bastarrika G, Leipsic JA, Paul NS, Rengo M, Laghi A, De Cecco CN. State of the Art: Iterative CT Reconstruction Techniques. Radiology 2015;276:339-57. [Crossref] [PubMed]
- Mello-Amoedo CD, Martins AN, Tachibana A, Pinho DF, Baroni RH. Comparison of Radiation Dose and Image Quality of Abdominopelvic CT Using Iterative (AIDR 3D) and Conventional Reconstructions. AJR Am J Roentgenol 2018;210:127-33. [Crossref] [PubMed]
- Yoshioka K, Tanaka R, Takagi H, Ueyama Y, Kikuchi K, Chiba T, Arakita K, Schuijf JD, Saito Y. Ultra-high-resolution CT angiography of the artery of Adamkiewicz: a feasibility study. Neuroradiology 2018;60:109-15. [Crossref] [PubMed]
- Schindera ST, Nelson RC, Toth TL, Nguyen GT, Toncheva GI, DeLong DM, Yoshizumi TT. Effect of patient size on radiation dose for abdominal MDCT with automatic tube current modulation: phantom study. AJR Am J Roentgenol 2008;190:W100-5. [Crossref] [PubMed]
- Schindera ST, Torrente JC, Ruder TD, Hoppe H, Marin D, Nelson RC, Szucs-Farkas Z. Decreased detection of hypovascular liver tumors with MDCT in obese patients: a phantom study. AJR Am J Roentgenol 2011;196:W772-6. [Crossref] [PubMed]
- American Association of Physicists in Medicine. Size-specific dose estimates (SSDE) in pediatric and adult body CT examinations. College Park, MD, USA: American Association of Physicists in Medicine, 2011.
- American College of Radiology. Liver Imaging Reporting and Data System version 2018. Available online: https://www.acr.org/Clinical-Resources/Reporting-and-Data-Systems/LI-RADS/CT-MRI-LI-RADS-v2018
- Funama Y, Awai K, Miyazaki O, Nakayama Y, Goto T, Omi Y, Shimonobo T, Liu D, Yamashita Y, Hori S. Improvement of low-contrast detectability in low-dose hepatic multidetector computed tomography using a novel adaptive filter: evaluation with a computer-simulated liver including tumors. Invest Radiol 2006;41:1-7. [Crossref] [PubMed]
- Christianson O, Winslow J, Frush DP, Samei E. Automated Technique to Measure Noise in Clinical CT Examinations. AJR Am J Roentgenol 2015;205:W93-9. [Crossref] [PubMed]
- Schindera ST, Diedrichsen L, Müller HC, Rusch O, Marin D, Schmidt B, Raupach R, Vock P, Szucs-Farkas Z. Iterative reconstruction algorithm for abdominal multidetector CT at different tube voltages: assessment of diagnostic accuracy, image quality, and radiation dose in a phantom study. Radiology 2011;260:454-62. [Crossref] [PubMed]
- Park SB. Advances in deep learning for computed tomography denoising. World J Clin Cases 2021;9:7614-9. [Crossref] [PubMed]
- Bernard A, Comby PO, Lemogne B, Haioun K, Ricolfi F, Chevallier O, Loffroy R. Deep learning reconstruction versus iterative reconstruction for cardiac CT angiography in a stroke imaging protocol: reduced radiation dose and improved image quality. Quant Imaging Med Surg 2021;11:392-401. [Crossref] [PubMed]