Development and validation of qualitative and quantitative models to predict invasiveness of lung adenocarcinomas manifesting as pure ground-glass nodules based on low-dose computed tomography during lung cancer screening
Introduction
With the widespread and routine use of low-dose computed tomography (LDCT) in lung cancer screening (1-5), the detection rates of ground-glass nodules (GGNs) have greatly increased, particularly in Asia (6-8). Most pathologically confirmed GGNs are adenocarcinomas (9,10), including adenocarcinoma in situ (AIS), minimally invasive adenocarcinoma (MIA), and invasive adenocarcinoma (IA) (11,12). Generally, the survival probability of AIS/MIA is nearly 100% after surgical resection, while that of IA ranges from 38% to 95% due to different patterns of growth (13-18).
Although pure GGNs (pGGNs) usually tend to be AIS/MIA, and a solid component tends to be an indicator of invasiveness (12,19), a substantial number of pGGNs are eventually diagnosed as IAs, with an incidence rate of 18.0–40.4% (20-24). As most pGGNs show stable or extremely slow growth on the follow-up screening (25-27), the present guidelines adopt a conservative attitude to pGGNs and recommend annual surveillance with LDCT before surgical intervention (19,28,29). However, previous studies have also observed that more than half of pGGNs progress during follow-up (30,31). The invasiveness may be a marker that indicates transition from the indolent period to the growth stage. Therefore, applying the same management strategy for pGGNs with or without invasiveness may be not appropriate. Recently, studies have demonstrated that IAs with a predominant ground-glass component had a nearly 100% 5-year survival rate after sublobar resection (32,33). Therefore, accurately differentiating AIS/MIA from IA during lung cancer screening will help thoracic surgeons and radiologists to choose whether to implement surgical intervention or conservative surveillance.
Previous studies have found that computed tomography (CT) features of pGGNs, such as morphology, size, attenuation, and vessel changes, could preoperatively and noninvasively predict the invasiveness of lung adenocarcinoma (20-24). However, all of these studies used standard-dose CT data rather than LDCT data from lung cancer screening. Evidence from phantom and in vivo studies suggests that the radiation dose reduction affects the quantification of size and attenuation of lung nodules (34-36). Thus quantitative features of LDCT may be not identical to that of standard-dose CT. Furthermore, previous studies might have been limited by relatively small sample sizes and the absence of an independent dataset to validate the selected qualitative and quantitative features (20-24).
This study aimed to develop and validate models based on qualitative and quantitative features of LDCT to differentiate AIS/MIA from IA that manifested as pGGNs. We also compared the predictive performance of these radiological models with intraoperative frozen section (FS) diagnosis. We present the following article in accordance with the Multivariable Prediction Model for Individual Prognosis or Diagnosis (TRIPOD) reporting checklist (available at https://qims.amegroups.com/article/view/10.21037/qims-21-912/rc).
Methods
Patients
The study was conducted in accordance with the Declaration of Helsinki (as revised in 2013). The study was approved by the Ethics Committees of Sichuan Cancer Hospital and Institute, School of Medicine, University of Electronic Science and Technology of China, and individual consent for this retrospective analysis was waived. A total of 241 pGGNs from 241 patients were consecutively enrolled from the Sichuan Cancer Hospital and Institute who underwent lung cancer screening from March 2018 to December 2020. All the data were analyzed anonymously. The inclusion criteria for this study were as follows: (I) patients with pulmonary pGGNs; (II) patients with an LDCT scan from lung cancer screening; and (III) patients whose pGGNs had been histopathological confirmed by surgical resection. The exclusion criteria were as follows: (I) patients with a history of cancer in the previous 5 years; (II) patients with pathologically confirmed benign pulmonary lesions; and (III) patients whose images were of poor quality due to artifacts.
The primary cohort consisted of 160 patients with 160 pGGNs (96 AIS/MIAs and 64 IAs) who underwent lung cancer screening from March 2018 to December 2020 using a 128-detector CT scanner (Brilliance iCT, Philips Healthcare, Amsterdam, The Netherlands). The validation cohort consisted of 63 patients with 63 pGGNs (39 AIS/MIAs and 24 IAs) who underwent lung cancer screening from February 2019 to December 2020 using a 64-detector dual-source CT scanner (Somatom Definition Flash, Siemens Healthcare, Forchheim, Germany). The age, gender, method of surgical resection, intraoperative FS diagnosis, and paraffin pathological diagnosis were recorded.
Histopathological evaluation
Both intraoperative FS diagnosis and paraffin-embedded tissue pathological diagnosis were performed according to the 2011 International Association for the Study of Lung Cancer, American Thoracic Society, and European Respiratory Society (IASLC/ATS/ERS) classification and the 2015 World Health Organization (WHO) classification for lung adenocarcinoma (11,12). Lung adenocarcinomas were classified as AIS, MIA, and IA. The definition of AIS was an adenocarcinoma lesion less than 3 cm in diameter with a pure lepidic pattern. The MIA classification stipulated a predominant lepidic pattern with an invasive component of less than 5 mm. The IA type was further classified by the predominant pattern using comprehensive histological subtyping of lepidic, acinar, papillary, micropapillary, and solid. The percentage of each histological component was recorded in 5% increments, and the predominant pattern was defined as the pattern with the largest percentage.
Image acquisition
All cases underwent chest LDCT and were instructed to hold their breath for as long as possible at the end of inspiration to ensure the quality of the images. The optimized protocol of LDCT used in this study was derived from our previous phantom study, which had comparable image quality and accurate volumetry compared with standard-dose CT in pGGNs (36). The estimated effective dose of LDCT was 0.69±0.11 mSv in the primary cohort and 0.49±0.11 mSv in the validation cohort. The detailed acquisition and reconstruction parameters are listed in Table 1.
Table 1
Parameters | Primary cohort | Validation cohort |
---|---|---|
Scanner | Brilliance iCT | Somatom Definition Flash |
Tube voltage | 100 kV | 100 kV |
Tube current | 20 or 30 mAs | 10 or 20 or 30 mAs |
Pitch | 0.915 | 1 |
Collimation | 128×0.625 mm | 128×0.6 mm |
Rotation time | 0.4 s | 0.33 s |
Field of view | 350 mm × 350 mm | 350 mm × 350 mm |
Reconstruction | ||
Algorithm | Iterative reconstruction (iDose4, level 6) | Iterative reconstruction (SAFIRE, Strength level 5) |
Slice thickness | 0.625 mm | 0.5 mm |
Slice increment | 0.625 mm | 0.5 mm |
Matrix | 512×512 | 512×512 |
LDCT, low-dose computed tomography.
Qualitative features
The images were evaluated in the lung window setting (level, −600 HU; width, 1,500 HU) by 2 radiologists with 10 years (HQ) and 25 years (PZ) of experience in chest radiology who were blinded to histopathological results. To evaluate the intraobserver reliability, 1 radiologist (HQ) repeated the evaluation at a 1-week interval. The intraclass correlation coefficient (ICC) was then calculated to evaluate the interobserver and intraobserver reliability.
The qualitative features included nodule location, shape (round/oval or irregular), margin (smooth or coarse), nodule-lung interface (clear or blurry), lobulation, spiculation, pleural indentation, air bronchogram, vacuole, and vessel type. The relationships between pGGNs and vessels were categorized into 4 types: type I, vessels passed by pGGNs without detectable supplying branches to lesions; type II, vessels passed through the lesions without obvious morphological changes in traveling path or size; type III, vessels within lesions were tortuous or rigid without an increase in amount; and type IV, more complicated vasculature other than that described in the aforementioned types within pGGNs, for instance, coexistence of irregular vascular dilation and vascular convergence from multiple supplying vessels. The relationship of type III and IV indicated the invasiveness of pGGNs (37,38). Multiple planar reconstruction was used to observe the air bronchogram and vessel type. The representative LDCT images of pGGNs are shown in Figure 1.
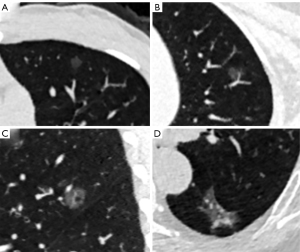
Quantitative features
All pGGNs were automatically detected, segmented, and measured using the uAI platform (United Imaging Healthcare, Shanghai, China), which is an artificial intelligence (AI) software based on deep learning method (39,40). The quantitative features included the diameter, volume, and mean CT attenuation. The diameter was the average of the maximal long-axis diameter and the perpendicular diameter on the maximum transverse plane of the nodule. The volume was calculated by multiplying the number of voxels by the unit volume of a voxel. The mean CT attenuation was the average of attenuation of each voxel.
Statistical analysis and TRIPOD
Statistical analysis was performed with the software SPSS 25.0 (IBM Corp., Chicago, IL, USA; https://www.ibm.com), MedCalc 18.2.1 (MedCalc, Ostend, Belgium; https://www.medcalc.org/), and R 4.0.3 (The R Foundation for Statistical Computing, Vienna, Austria; https://www.r-project.org/). The gender, qualitative features, and intraoperative FS diagnosis were analyzed using the chi-square test or Fisher’s test. Age and quantitative features were analyzed using an independent sample t-test. A P value <0.05 was considered statistically significant.
Independent factors for differentiating AIS/MIA from IA were identified by inputting the significant variables found in the primary cohort using univariate logistic regression analysis. Then, multivariate logistic regression with backward stepwise selection and Akaike’s information criterion was applied to constructed predicting models basing on these significant independent factors. Finally, 3 radiological models to differentiate AIS/MIA from IA, including qualitative, quantitative, and combined qualitative and quantitative models, were trained on the primary cohort and tested on the validation cohort. The clinical variables, including age and gender, were also added into these models.
The area under curve (AUC) of the receiver operating characteristic (ROC) curve was used to evaluate the performance of the radiological models and FS diagnosis in the primary and validation cohorts. The binomial exact method was used to determine the confidence intervals (CI) of AUCs. The optimal cut-off threshold was delimited according to Youden’s index of ROC analysis, and the corresponding sensitivity, specificity, and accuracy were calculated. The Hosmer-Lemeshow test was used to assess the goodness-of-fit of the radiological models. The calibration curve of each radiological model was also plotted, and its corresponding calibration slope and intercept were measured. The DeLong test was used to compare the AUCs among the radiological models and FS diagnosis (41). Multiple comparisons were corrected using the Bonferroni’s method.
As a study to construct predicting models for diagnostic purpose, this article was written following the TRIPOD statement (42). We concluded that the type of this study was type 2b.
Results
Clinical characteristics
The proportion of female patients in the AIS/MIA group was higher than that in the IA group (P=0.012), and cases with AIS/MIA were younger than those with IA (P=0.016) in the primary cohort. No significant difference was found in gender and age in the validation cohort. The AIS/MIA group had the higher rates of sublobar resection than the IA group in both the primary and validation cohorts (P<0.001 and P=0.015) (Table 2).
Table 2
Characteristics | Primary cohort (n=160) | Validation cohort (n=63) | |||||
---|---|---|---|---|---|---|---|
AIS/MIA (n=96) | IA (n=64) | P value | AIS/MIA (n=39) | IA (n=24) | P value | ||
Gender | 0.012 | 0.256 | |||||
Male | 25 | 29 | 8 | 8 | |||
Female | 71 | 35 | 31 | 16 | |||
Age (years) | 50.3±10.8 | 54.3±9.5 | 0.016 | 50.3±13.0 | 53.2±15.8 | 0.423 | |
Lobe | 0.287 | 0.018 | |||||
Left upper lobe | 30 | 14 | 15 | 5 | |||
Left lower lobe | 9 | 6 | 3 | 6 | |||
Right upper lobe | 39 | 33 | 18 | 6 | |||
Right middle lobe | 3 | 5 | 1 | 1 | |||
Right lower lobe | 15 | 6 | 2 | 6 | |||
Shape | 0.044 | 0.185 | |||||
Round/oval | 59 | 29 | 29 | 14 | |||
Irregular | 37 | 35 | 10 | 10 | |||
Margin | 0.024 | 0.999 | |||||
Smooth | 18 | 4 | 1 | 0 | |||
Coarse | 78 | 60 | 38 | 24 | |||
Interface | 0.431 | 0.145 | |||||
Clear | 42 | 24 | 17 | 15 | |||
Blurry | 54 | 40 | 22 | 9 | |||
Lobulation | 0.001 | 0.256 | |||||
No | 91 | 50 | 31 | 16 | |||
Yes | 5 | 14 | 8 | 8 | |||
Spiculation | 0.703 | 0.141 | |||||
No | 91 | 62 | 39 | 22 | |||
Yes | 5 | 2 | 0 | 2 | |||
Pleural indentation | 0.073 | 0.045 | |||||
No | 74 | 41 | 33 | 15 | |||
Yes | 22 | 23 | 6 | 9 | |||
Air bronchogram | <0.001 | 0.721 | |||||
No | 90 | 47 | 34 | 20 | |||
Yes | 6 | 17 | 5 | 4 | |||
Vacuole | 0.007 | 0.049 | |||||
No | 64 | 29 | 29 | 12 | |||
Yes | 32 | 35 | 10 | 12 | |||
Vessel type | <0.001 | <0.001 | |||||
Type I/II | 77 | 10 | 32 | 3 | |||
Type III/IV | 19 | 54 | 7 | 21 | |||
Diameter (mm) | 8.1±2.9 | 12.1±4.4 | <0.001 | 7.1±2.4 | 10.8±4.3 | <0.001 | |
Volume (mm3) | 509.4±399.3 | 1,609.5±1,874.9 | <0.001 | 409.5±418.7 | 1,347.5±1,505.0 | <0.001 | |
Attenuation (HU) | −663.1±63.8 | −639.7±73.1 | 0.033 | −673.8±57.4 | −630.4±65.5 | 0.008 | |
FS diagnosis | <0.001 | <0.001 | |||||
AIS/MIA | 86 | 10 | 38 | 2 | |||
IA | 10 | 54 | 1 | 22 | |||
Surgical method | <0.001 | 0.015 | |||||
Sublobar resection | 80 | 32 | 31 | 12 | |||
Lobectomy | 16 | 32 | 8 | 12 |
Age, diameter, volume, and attenuation are shown in mean ± standard deviation, and the other data are the number of nodules. pGGNs, pure ground-glass nodules; AIS, adenocarcinoma in situ; MIA, minimally invasive adenocarcinoma; IA, invasive adenocarcinoma; FS, frozen section.
Qualitative and quantitative features
All the qualitative features showed good interobserver and intraobserver reliability. The interobserver ICCs ranged from 0.855 to 0.928, and the intraobserver ICCs ranged from 0.893 to 0.955. Therefore, the qualitative features evaluated by the radiologist (PZ) were selected to perform the following analyses.
The qualitative and quantitative features of pGGNs are shown in Table 2. There were significant differences of vacuole (P=0.007 and 0.049) and vessel type (P<0.001 and <0.001) in both the primary and validation cohorts. The shape (P=0.044), margin (P=0.024), lobulation (P=0.001), and air bronchogram (P<0.001) were significantly different in the primary cohort but not in the validation cohort. The nodule location (P=0.018) and pleural indentation (P=0.045) were significantly different in the validation cohort but not in the primary cohort. No significant difference was found in the nodule-lung interface and speculation in the primary or validation cohort. The diameter, volume, and attenuation of AIS/MIA were significantly lower than those of IA in both the primary and validation cohorts (all P<0.001).
Model construction
Univariate logistic regression analysis showed that gender, age, shape, margin, lobulation, air bronchogram, vacuole, vessel type, diameter, volume, and attenuation were independent factors for differentiating AIS/MIA from IA (Table 3). Next, these independent factors were integrated to develop quantitative, qualitative, and combined qualitative and quantitative models using multivariate logistic regression. The calculation formula for the qualitative model was as follows: ln (P/1−P) = −2.41764 + 0.86528 × vacuole + 3.0806 × vessel type, where P is the probability of IA (cutoff >0.41734). The calculation formula for quantitative model was as follows: ln (P/1−P) = 2.83033 + 0.00195 × volume + 0.00737 × attenuation, where P is the probability of IA (cutoff >0.38484). The calculation formula for combined qualitative and quantitative model was as follows: ln (P/1−P) = −2.52384 + 2.46530 × vessel type + 0.00099 × volume, where P is the probability of IA (cutoff >0.54374).
Table 3
Variables | Odds ratio | P value | AUC | Sensitivity | Specificity | Cut-off |
---|---|---|---|---|---|---|
Gender | 2.353 | 0.012 | 0.596 | 0.453 | 0.740 | |
Age | 1.040 | 0.018 | 0.618 | 0.750 | 0.490 | >50.0 years |
Shape | 1.925 | 0.045 | 0.581 | 0.547 | 0.615 | |
Margin | 3.462 | 0.032 | 0.563 | 0.938 | 0.188 | |
Lobulation | 5.096 | 0.003 | 0.583 | 0.219 | 0.948 | |
Air bronchogram | 5.426 | 0.001 | 0.602 | 0.266 | 0.938 | |
Vacuole | 2.414 | 0.008 | 0.607 | 0.547 | 0.667 | |
Vessel type | 21.884 | <0.001 | 0.823 | 0.844 | 0.802 | |
Diameter | 1.383 | <0.001 | 0.773 | 0.703 | 0.729 | >10.0 mm |
Volume | 1.002 | <0.001 | 0.792 | 0.906 | 0.542 | >348.1 mm3 |
Attenuation | 1.005 | 0.036 | 0.599 | 0.469 | 0.729 | >−636.3 HU |
AUC, area under curve.
Model performance
The ROC curves of the radiological models and FS diagnosis are shown in Figure 2. The AUC, sensitivity, specificity, and accuracy in primary and validation cohorts are listed in Table 4. The AUCs of qualitative model, quantitative model, combined qualitative and quantitative model, and FS diagnosis were 0.854 (95% CI: 0.790–0.905), 0.803 (95% CI: 0.733–0.862), 0.873 (95% CI: 0.811–0.920), and 0.870 (95% CI: 0.808–0.918), respectively, in the primary cohort and 0.884 (95% CI: 0.778–0.951), 0.855 (95% CI: 0.744–0.931), 0.875 (95% CI: 0.767–0.945), and 0.946 (95% CI: 0.858–0.987), respectively, in the validation cohort.
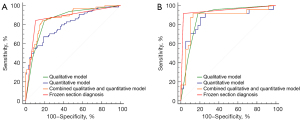
Table 4
Cohorts | AUC (95% CI) | Accuracy | Sensitivity | Specificity |
---|---|---|---|---|
Primary cohort | ||||
Qualitative | 0.854 (0.790–0.905) | 0.819 | 0.844 | 0.802 |
Quantitative | 0.803 (0.733–0.862) | 0.756 | 0.672 | 0.812 |
Combined qualitative and quantitative | 0.873 (0.811–0.920) | 0.831 | 0.844 | 0.823 |
FS diagnosis | 0.870 (0.808–0.918) | 0.875 | 0.844 | 0.896 |
Validation cohort | ||||
Qualitative | 0.884 (0.778–0.951) | 0.857 | 0.917 | 0.821 |
Quantitative | 0.855 (0.744–0.931) | 0.810 | 0.625 | 0.923 |
Combined qualitative and quantitative | 0.875 (0.767–0.945) | 0.889 | 0.917 | 0.872 |
FS diagnosis | 0.946 (0.858–0.987) | 0.952 | 0.917 | 0.974 |
FS, frozen section; AUC, area under curve; CI, confidence interval.
The Hosmer-Lemeshow test yielded non-significant results in both the primary and validation cohorts of the qualitative model (P=0.877 and 0.678), quantitative model (P=0.990 and 0.166), and combined qualitative and quantitative model (P=0.103 and 0.206), which suggested no departure from the perfect fit. The calibration curves of the radiological models for the probability of invasiveness showed good agreement between prediction and observation in both the primary and validation cohorts (Figure 3). According to the DeLong test, no significant difference of the AUCs was found among the radiological models and FS diagnosis in the primary or validation cohort (all corrected P>0.05; Table 5).
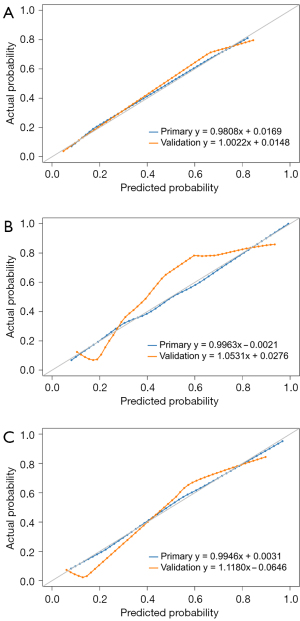
Table 5
Pairwise comparison | Primary cohort | Validation cohort | |||
---|---|---|---|---|---|
Z | P value | Z | P value | ||
Qualitative vs. quantitative | 1.436 | 0.151 | 0.666 | 0.506 | |
Qualitative vs. combined qualitative and quantitative | 1.029 | 0.303 | 0.243 | 0.808 | |
Quantitative vs. combined qualitative and quantitative | 2.482 | 0.013* | 0.594 | 0.552 | |
Qualitative vs. FS diagnosis | 0.377 | 0.706 | 1.083 | 0.279 | |
Quantitative model vs. FS diagnosis | 1.450 | 0.147 | 1.343 | 0.179 | |
Combined qualitative and quantitative vs. FS diagnosis | 0.083 | 0.934 | 1.083 | 0.279 |
*, difference was not significant at P<0.05 corrected with Bonferroni’s method. FS, frozen section.
Discussion
In this study, we developed and validated the qualitative and quantitative models based on LDCT to differentiate AIS/MIA from IA that manifested as pGGNs. The AUCs of these radiological models were equivalent to that of FS diagnosis. The combined qualitative and quantitative model showed the highest accuracy in both the primary and validation cohorts (0.831 and 0.889) among radiological models, suggesting that the vessel type and volume could be preoperative and non-invasive biomarkers to stratify the invasive risk of pGGNs during lung cancer screening.
Although many previous studies have made efforts to identify qualitative and quantitative features of CT to differentiate AIS/MIA from IA that manifested as pGGNs, there were some differences in our study with regard to the research data and methods. First, to our knowledge, this was the first study to use the LDCT data from lung cancer screening. As the number of detected pGGNs continues to increase with the popularization of lung cancer screening and development of AI approaches, the subsequent increase in precision may reduce undue anxiety for patients and avoid unnecessary and excessive examinations, such as standard-dose CT, contrast-enhanced CT, and positron emission tomography (PET). Second, the use of a validation dataset from another CT scanner demonstrated that our models were generalizable to some extent, making our models more reproducible and convincing. Third, the automatic segmentation and measurement methods avoid inter-observer and intra-observer variability of the quantitative features of nodules.
Previous studies have shown that nodule size is a valuable feature for differentiating AIS/MIA from IA in pGGNs (20,21,23,24,43-47). Most of these studies have used the maximum diameter to measure the nodule size; however, the optimal cutoff value has been inconsistent, ranging from 10.0 to 17.2 mm (20,21,23,43,45-47). Chu et al. (24) found that the optimal cutoff value of mean diameter was 10.5 mm, which was close to our result of 10.0 mm in univariate analysis. As another measurement of size, volume has rarely been reported in previous research, which may be due to the workload required to delineate the contour of nodules on each slice of CT images. Fortunately, this issue has been addressed by the development of computer-assisted diagnosis (CAD) systems and advanced AI software based on deep learning algorithms. Relying on volume rather than diameter better reflects the three-dimensional nature of nodules, allows calculation of the volume doubling time (VDT) to more reliably define growth, and reduces subjective inconsistency between and among observers (48). The Lung CT Screening Reporting & Data System (Lung-RADS, version 1.1) was also updated in 2019 to include volume in nodule management (49). Both Han et al. (46) and our study found the volume was of significance to predict the invasiveness of pGGNs, but the optimal cutoff values were divergent (1,349 and 348.1 mm3), yielding similar AUC (0.781 and 0.792) and relatively low specificity (0.637 and 0.542), respectively. It should be noted that the optimal cutoff values of diameter and volume were relatively small and about 56% of pathologically confirmed pGGNs were AIS/MIA in this study, which indicated the positive attitudes towards pGGNs and the great fear of missing IAs for both surgeons and patients, especially in the cancer hospital. The medical environment of China tends to favor cautiousness, but this may also result in overtreatment (50). The attenuation was another significant quantitative feature in predicting invasiveness (51,52). The amount of alveolar airspace and thickness of alveolar walls may cause the difference of attenuation between AIS/MIA and IA (21,53). However, our study and previous studies showed relatively low predicting accuracy of the attenuation in univariate analysis (43,46), which might result from subtle difference of attenuation between pGGNs with and without invasiveness. In the multivariate analysis, the final quantitative model that combined volume and attenuation performed well in the validation cohort, with an accuracy of 0.810, but had unbalanced sensitivity and specificity (0.625 and 0.923, respectively). Furthermore, it was worth noting that the diameter was not included in the final quantitative model. The reason might be the collinearity between diameter and volume.
The vessel type was shown to be a robust qualitative feature that could be used to predict the invasiveness with an AUC of 0.823, a sensitivity of 0.844, and a specificity of 0.802. There are several potential mechanisms for vessel type changes. First, the progress of invasiveness might lead to an increase of oxygen consumption, which could influence the supplying vessel, resulting in an increase of permeability and diameter. Second, as angiogenesis is one of the hallmarks of cancer, the increase in the number of vessels is consistent with the concept of neovascularization (54,55). Third, the fibrosis foci increase when the invasive grade increases, further leading to vascular convergence around the tumor (56). However, the vessel type assessment was subjective and empirical, as it was based on the location and morphological change. To improve the repeatability, Liang et al. (22) transformed this subjective feature into a quantitative measurement of the amount of related vessels through the use of maximum-intensity projection images and volume-rendering technique images. Using the amount of blood vessels “n≥1” as the diagnostic criterion, the AUC for predicting invasiveness was 0.738 with a sensitivity of 1.000 and a specificity of 0.304 (22). The vacuole, which has a bubble-like appearance, has been associated with increasing nodule size (57) and has histological characteristics of collapse and dilated bronchioles (58). Although our univariate analysis showed relatively low predicting performance of the vacuole, the qualitative model combining the vessel type and vacuole showed the highest AUC of 0.884 among the radiological models in the validation dataset.
Intraoperative FS is a common method to guide surgical management for pulmonary nodules. However, the diagnosis of an invasive component cannot be confirmed without entire histologic sampling (11). Our results showed that the intraoperative FS diagnosis was not completely consistent with the final histologic diagnosis, which is similar to the findings of previous studies (17,59,60). A total of 12 AIS/MIAs were overdiagnosed as IAs in FS in our study (10 in the primary cohort and 2 in the validation cohort). This may be due to the subjective overestimation of AIS/MIA by pathologists to avoid IA underestimation. Further efforts should be made to improve the accuracy of FS in distinguishing IA from AIS/MIA to guide intraoperative decisions of pGGNs. The developed radiological models in our study showed equivalent diagnostic performance to FS diagnosis, suggesting that the qualitative and quantitative radiological features of LDCT could be non-invasive biomarkers to identify the invasive component of pGGNs during lung cancer screening.
Our study had several limitations. First, this was a single-center study with a relatively small sample size. However, the standardization of acquisition parameters and the use of the automatic segmentation approach avoided potential confounding biases caused by heterogeneous acquisition parameters and manual contouring. Further external validation datasets are needed to test the reliability of our models. Second, the size of pGGNs included in this study were heterogeneous, with diameters of ranging from 4 to 25 mm. We did not establish the inclusion criteria based on any surgical criteria, such as the Japan Clinical Oncology Group 0201 strategy, which defines a radiologic noninvasive lung cancer as a tumor with a maximum diameter of 20 mm or less and with a consolidation tumor ratio of 0.25 or less (33,61). Further radiological studies should adopt certain surgical criteria to enroll GGNs, and then construct differentiating models between AIS/MIA and IA, which might provide additional help to thoracic surgeons and radiologists in clinical decision-making. Third, the LDCT protocol used in this study may be inapplicable to other scanners and reconstruction algorithms. Previous studies found that the radiation dose reduction increased the CT value and decreased the diameter and volume of pulmonary nodules, but qualitative features, such as the sphericity, were unaffected (34-36). However, these effects were only emerging under specific image acquisition conditions. Therefore, we suggest performing a phantom study to detect the potential effects of radiation dose reduction on quantitative or qualitative features before applying the LDCT protocol in clinical practice.
Conclusions
The diagnostic performances of qualitative and quantitative models based on LDCT to differentiate IA from AIS/MIA in pGGNs were equivalent to intraoperative FS diagnosis. The findings showed that the vessel type and volume can be preoperative and non-invasive biomarkers to assess the invasive risk of pGGNs during lung cancer screening.
Acknowledgments
Funding: This work was supported by the Sichuan Science and Technology Program (Nos. 2021YFS0075, 2021YFS0225, and 2019YJ0585).
Footnote
Reporting Checklist: The authors have completed the Transparent Reporting of a multivariable prediction model for Individual Prognosis or Diagnosis (TRIPOD) reporting checklist. Available at https://qims.amegroups.com/article/view/10.21037/qims-21-912/rc
Conflicts of Interest: All authors have completed the ICMJE uniform disclosure form (available at https://qims.amegroups.com/article/view/10.21037/qims-21-912/coif). The authors have no conflicts of interest to declare.
Ethical Statement: The authors are accountable for all aspects of the work and ensuring that questions related to the accuracy or integrity of any part of the work are appropriately investigated and resolved. The study was conducted in accordance with the Declaration of Helsinki (as revised in 2013). The study was approved by the Ethics Committees of Sichuan Cancer Hospital and Institute, School of Medicine, University of Electronic Science and Technology of China, and individual consent for this retrospective analysis was waived.
Open Access Statement: This is an Open Access article distributed in accordance with the Creative Commons Attribution-NonCommercial-NoDerivs 4.0 International License (CC BY-NC-ND 4.0), which permits the non-commercial replication and distribution of the article with the strict proviso that no changes or edits are made and the original work is properly cited (including links to both the formal publication through the relevant DOI and the license). See: https://creativecommons.org/licenses/by-nc-nd/4.0/.
References
- Aberle DR, Adams AM, Berg CD, Black WC, Clapp JD, Fagerstrom RM, Gareen IF, Gatsonis C, Marcus PM, Sicks JD. Reduced lung-cancer mortality with low-dose computed tomographic screening. N Engl J Med 2011;365:395-409. [Crossref] [PubMed]
- Aberle DR, DeMello S, Berg CD, Black WC, Brewer B, Church TR, Clingan KL, Duan F, Fagerstrom RM, Gareen IF, Gatsonis CA, Gierada DS, Jain A, Jones GC, Mahon I, Marcus PM, Rathmell JM, Sicks JNational Lung Screening Trial Research Team. Results of the two incidence screenings in the National Lung Screening Trial. N Engl J Med 2013;369:920-31. [Crossref] [PubMed]
- Patz EF Jr, Greco E, Gatsonis C, Pinsky P, Kramer BS, Aberle DR. Lung cancer incidence and mortality in National Lung Screening Trial participants who underwent low-dose CT prevalence screening: a retrospective cohort analysis of a randomised, multicentre, diagnostic screening trial. Lancet Oncol 2016;17:590-9. [Crossref] [PubMed]
- Wang Z, Han W, Zhang W, Xue F, Wang Y, Hu Y, Wang L, Zhou C, Huang Y, Zhao S, Song W, Sui X, Shi R, Jiang J. Mortality outcomes of low-dose computed tomography screening for lung cancer in urban China: a decision analysis and implications for practice. Chin J Cancer 2017;36:57. [Crossref] [PubMed]
- de Koning HJ, van der Aalst CM, de Jong PA, Scholten ET, Nackaerts K, Heuvelmans MA, et al. Reduced Lung-Cancer Mortality with Volume CT Screening in a Randomized Trial. N Engl J Med 2020;382:503-13. [Crossref] [PubMed]
- Lee CT. What do we know about ground-glass opacity nodules in the lung? Transl Lung Cancer Res 2015;4:656-9. [PubMed]
- Pedersen JH, Saghir Z, Wille MM, Thomsen LH, Skov BG, Ashraf H. Ground-Glass Opacity Lung Nodules in the Era of Lung Cancer CT Screening: Radiology, Pathology, and Clinical Management. Oncology (Williston Park) 2016;30:266-74. [PubMed]
- Zhang Y, Jheon S, Li H, Zhang H, Xie Y, Qian B, Lin K, Wang S, Fu C, Hu H, Zheng Y, Li Y, Chen H. Results of low-dose computed tomography as a regular health examination among Chinese hospital employees. J Thorac Cardiovasc Surg 2020;160:824-31.e4. [Crossref] [PubMed]
- Kobayashi Y, Mitsudomi T, Sakao Y, Yatabe Y. Genetic features of pulmonary adenocarcinoma presenting with ground-glass nodules: the differences between nodules with and without growth. Ann Oncol 2015;26:156-61. [Crossref] [PubMed]
- Yang W, Sun Y, Fang W, Qian F, Ye J, Chen Q, Jiang Y, Yu K, Han B. High-resolution Computed Tomography Features Distinguishing Benign and Malignant Lesions Manifesting as Persistent Solitary Subsolid Nodules. Clin Lung Cancer 2018;19:e75-83. [Crossref] [PubMed]
- Travis WD, Brambilla E, Noguchi M, Nicholson AG, Geisinger KR, Yatabe Y, et al. International association for the study of lung cancer/american thoracic society/european respiratory society international multidisciplinary classification of lung adenocarcinoma. J Thorac Oncol 2011;6:244-85. [Crossref] [PubMed]
- Travis WD, Brambilla E, Nicholson AG, Yatabe Y, Austin JHM, Beasley MB, Chirieac LR, Dacic S, Duhig E, Flieder DB, Geisinger K, Hirsch FR, Ishikawa Y, Kerr KM, Noguchi M, Pelosi G, Powell CA, Tsao MS, Wistuba I, Panel WHO. The 2015 World Health Organization Classification of Lung Tumors: Impact of Genetic, Clinical and Radiologic Advances Since the 2004 Classification. J Thorac Oncol 2015;10:1243-60. [Crossref] [PubMed]
- Russell PA, Wainer Z, Wright GM, Daniels M, Conron M, Williams RA. Does lung adenocarcinoma subtype predict patient survival?: A clinicopathologic study based on the new International Association for the Study of Lung Cancer/American Thoracic Society/European Respiratory Society international multidisciplinary lung adenocarcinoma classification. J Thorac Oncol 2011;6:1496-504. [Crossref] [PubMed]
- Yoshizawa A, Motoi N, Riely GJ, Sima CS, Gerald WL, Kris MG, Park BJ, Rusch VW, Travis WD. Impact of proposed IASLC/ATS/ERS classification of lung adenocarcinoma: prognostic subgroups and implications for further revision of staging based on analysis of 514 stage I cases. Mod Pathol 2011;24:653-64. [Crossref] [PubMed]
- Yanagawa N, Shiono S, Abiko M, Ogata SY, Sato T, Tamura G. New IASLC/ATS/ERS classification and invasive tumor size are predictive of disease recurrence in stage I lung adenocarcinoma. J Thorac Oncol 2013;8:612-8. [Crossref] [PubMed]
- Zhang J, Wu J, Tan Q, Zhu L, Gao W. Why do pathological stage IA lung adenocarcinomas vary from prognosis?: a clinicopathologic study of 176 patients with pathological stage IA lung adenocarcinoma based on the IASLC/ATS/ERS classification. J Thorac Oncol 2013;8:1196-202. [Crossref] [PubMed]
- Liu S, Wang R, Zhang Y, Li Y, Cheng C, Pan Y, Xiang J, Zhang Y, Chen H, Sun Y. Precise Diagnosis of Intraoperative Frozen Section Is an Effective Method to Guide Resection Strategy for Peripheral Small-Sized Lung Adenocarcinoma. J Clin Oncol 2016;34:307-13. [Crossref] [PubMed]
- Yotsukura M, Asamura H, Motoi N, Kashima J, Yoshida Y, Nakagawa K, Shiraishi K, Kohno T, Yatabe Y, Watanabe SI. Long-Term Prognosis of Patients With Resected Adenocarcinoma In Situ and Minimally Invasive Adenocarcinoma of the Lung. J Thorac Oncol 2021;16:1312-20. [Crossref] [PubMed]
- MacMahon H, Naidich DP, Goo JM, Lee KS, Leung ANC, Mayo JR, Mehta AC, Ohno Y, Powell CA, Prokop M, Rubin GD, Schaefer-Prokop CM, Travis WD, Van Schil PE, Bankier AA. Guidelines for Management of Incidental Pulmonary Nodules Detected on CT Images: From the Fleischner Society 2017. Radiology 2017;284:228-43. [Crossref] [PubMed]
- Lee SM, Park CM, Goo JM, Lee HJ, Wi JY, Kang CH. Invasive pulmonary adenocarcinomas versus preinvasive lesions appearing as ground-glass nodules: differentiation by using CT features. Radiology 2013;268:265-73. [Crossref] [PubMed]
- Lim HJ, Ahn S, Lee KS, Han J, Shim YM, Woo S, Kim JH, Yie M, Lee HY, Yi CA. Persistent pure ground-glass opacity lung nodules ≥ 10 mm in diameter at CT scan: histopathologic comparisons and prognostic implications. Chest 2013;144:1291-9. [Crossref] [PubMed]
- Liang J, Xu XQ, Xu H, Yuan M, Zhang W, Shi ZF, Yu TF. Using the CT features to differentiate invasive pulmonary adenocarcinoma from pre-invasive lesion appearing as pure or mixed ground-glass nodules. Br J Radiol 2015;88:20140811. [Crossref] [PubMed]
- Jin X, Zhao SH, Gao J, Wang DJ, Wu J, Wu CC, Chang RP, Ju HY. CT characteristics and pathological implications of early stage (T1N0M0) lung adenocarcinoma with pure ground-glass opacity. Eur Radiol 2015;25:2532-40. [Crossref] [PubMed]
- Chu ZG, Li WJ, Fu BJ, Lv FJ. CT Characteristics for Predicting Invasiveness in Pulmonary Pure Ground-Glass Nodules. AJR Am J Roentgenol 2020;215:351-8. [Crossref] [PubMed]
- Chang B, Hwang JH, Choi YH, Chung MP, Kim H, Kwon OJ, Lee HY, Lee KS, Shim YM, Han J, Um SW. Natural history of pure ground-glass opacity lung nodules detected by low-dose CT scan. Chest 2013;143:172-8. [Crossref] [PubMed]
- Yankelevitz DF, Yip R, Smith JP, Liang M, Liu Y, Xu DM, Salvatore MM, Wolf AS, Flores RM, Henschke CIInternational Early Lung Cancer Action Program Investigators Group. CT Screening for Lung Cancer: Nonsolid Nodules in Baseline and Annual Repeat Rounds. Radiology 2015;277:555-64. [Crossref] [PubMed]
- Kakinuma R, Muramatsu Y, Kusumoto M, Tsuchida T, Tsuta K, Maeshima AM, Asamura H, Moriyama N. Solitary Pure Ground-Glass Nodules 5 mm or Smaller: Frequency of Growth. Radiology 2015;276:873-82. [Crossref] [PubMed]
- Bai C, Choi CM, Chu CM, Anantham D, Chung-Man Ho J, Khan AZ, Lee JM, Li SY, Saenghirunvattana S, Yim A. Evaluation of Pulmonary Nodules: Clinical Practice Consensus Guidelines for Asia. Chest 2016;150:877-93. [Crossref] [PubMed]
- Mazzone PJ, Silvestri GA, Patel S, Kanne JP, Kinsinger LS, Wiener RS, Soo Hoo G, Detterbeck FC. Screening for Lung Cancer: CHEST Guideline and Expert Panel Report. Chest 2018;153:954-85. [Crossref] [PubMed]
- Kodama K, Higashiyama M, Yokouchi H, Takami K, Kuriyama K, Kusunoki Y, Nakayama T, Imamura F. Natural history of pure ground-glass opacity after long-term follow-up of more than 2 years. Ann Thorac Surg 2002;73:386-92; discussion 392-3. [Crossref] [PubMed]
- Eguchi T, Kondo R, Kawakami S, Matsushita M, Yoshizawa A, Hara D, Matsuoka S, Takeda T, Miura K, Agatsuma H, Sakaizawa T, Tominaga Y, Saito G, Toishi M, Hamanaka K, Hashizume M, Shiina T, Amano J, Koizumi T, Yoshida K. Computed tomography attenuation predicts the growth of pure ground-glass nodules. Lung Cancer 2014;84:242-7. [Crossref] [PubMed]
- Fu F, Zhang Y, Wen Z, Zheng D, Gao Z, Han H, Deng L, Wang S, Liu Q, Li Y, Shen L, Shen X, Zhao Y, Zhao Z, Ye T, Xiang J, Zhang Y, Sun Y, Hu H, Chen H. Distinct Prognostic Factors in Patients with Stage I Non-Small Cell Lung Cancer with Radiologic Part-Solid or Solid Lesions. J Thorac Oncol 2019;14:2133-42. [Crossref] [PubMed]
- Suzuki K, Watanabe SI, Wakabayashi M, Saji H, Aokage K, Moriya Y, Yoshino I, Tsuboi M, Nakamura S, Nakamura K, Mitsudomi T, Asamura HWest Japan Oncology Group and Japan Clinical Oncology Group. A single-arm study of sublobar resection for ground-glass opacity dominant peripheral lung cancer. J Thorac Cardiovasc Surg 2022;163:289-301.e2. [Crossref] [PubMed]
- Solomon J, Mileto A, Nelson RC, Roy Choudhury K, Samei E. Quantitative Features of Liver Lesions, Lung Nodules, and Renal Stones at Multi-Detector Row CT Examinations: Dependency on Radiation Dose and Reconstruction Algorithm. Radiology 2016;279:185-94. [Crossref] [PubMed]
- Jin S, Zhang B, Zhang L, Li S, Li S, Li P. Lung nodules assessment in ultra-low-dose CT with iterative reconstruction compared to conventional dose CT. Quant Imaging Med Surg 2018;8:480-90. [Crossref] [PubMed]
- Liu J, Qing H, Luo H, He C, Wang C, Ren J, Zhou P. Accuracy of Pulmonary Nodule Volumetry at Different Exposure Parameters in Low-Dose Computed Tomography: A Phantom Study. J Comput Assist Tomogr 2019;43:926-30. [Crossref] [PubMed]
- Gao F, Li M, Ge X, Zheng X, Ren Q, Chen Y, Lv F, Hua Y. Multi-detector spiral CT study of the relationships between pulmonary ground-glass nodules and blood vessels. Eur Radiol 2013;23:3271-7. [Crossref] [PubMed]
- Gao F, Sun Y, Zhang G, Zheng X, Li M, Hua Y. CT characterization of different pathological types of subcentimeter pulmonary ground-glass nodular lesions. Br J Radiol 2019;92:20180204. [Crossref] [PubMed]
- Wang Q, Zhou X, Wang C, Liu Z, Huang J, Zhou Y, Li C, Zhuang H, Cheng JZ. WGAN-Based Synthetic Minority Over-Sampling Technique: Improving Semantic Fine-Grained Classification for Lung Nodules in CT Images. IEEE Access 2019;7:18450-63.
- Mu G, Chen Y, Wu D, Zhan Y, Zhou XS, Gao Y. Relu Cascade of Feature Pyramid Networks for CT Pulmonary Nodule Detection. Cham: Springer International Publishing, 2019:444-52.
- DeLong ER, DeLong DM, Clarke-Pearson DL. Comparing the areas under two or more correlated receiver operating characteristic curves: a nonparametric approach. Biometrics 1988;44:837-45. [Crossref] [PubMed]
- Moons KG, Altman DG, Reitsma JB, Ioannidis JP, Macaskill P, Steyerberg EW, Vickers AJ, Ransohoff DF, Collins GS. Transparent Reporting of a multivariable prediction model for Individual Prognosis or Diagnosis (TRIPOD): explanation and elaboration. Ann Intern Med 2015;162:W1-73. [Crossref] [PubMed]
- Eguchi T, Yoshizawa A, Kawakami S, Kumeda H, Umesaki T, Agatsuma H, Sakaizawa T, Tominaga Y, Toishi M, Hashizume M, Shiina T, Yoshida K, Asaka S, Matsushita M, Koizumi T. Tumor size and computed tomography attenuation of pulmonary pure ground-glass nodules are useful for predicting pathological invasiveness. PLoS One 2014;9:e97867. [Crossref] [PubMed]
- Hwang IP, Park CM, Park SJ, Lee SM, McAdams HP, Jeon YK, Goo JM. Persistent Pure Ground-Glass Nodules Larger Than 5 mm: Differentiation of Invasive Pulmonary Adenocarcinomas From Preinvasive Lesions or Minimally Invasive Adenocarcinomas Using Texture Analysis. Invest Radiol 2015;50:798-804. [Crossref] [PubMed]
- Moon Y, Sung SW, Lee KY, Sim SB, Park JK. Pure ground-glass opacity on chest computed tomography: predictive factors for invasive adenocarcinoma. J Thorac Dis 2016;8:1561-70. [Crossref] [PubMed]
- Han L, Zhang P, Wang Y, Gao Z, Wang H, Li X, Ye Z. CT quantitative parameters to predict the invasiveness of lung pure ground-glass nodules (pGGNs). Clin Radiol 2018;73:504.e1-7. [Crossref] [PubMed]
- Qi L, Xue K, Li C, He W, Mao D, Xiao L, Hua Y, Li M. Analysis of CT morphologic features and attenuation for differentiating among transient lesions, atypical adenomatous hyperplasia, adenocarcinoma in situ, minimally invasive and invasive adenocarcinoma presenting as pure ground-glass nodules. Sci Rep 2019;9:14586. [Crossref] [PubMed]
- Devaraj A, van Ginneken B, Nair A, Baldwin D. Use of Volumetry for Lung Nodule Management: Theory and Practice. Radiology 2017;284:630-44. [Crossref] [PubMed]
- American College of Radiology. Lung CT Screening Reporting and Data System (Lung-RADS, Version 1.1) 2019. Available online: https://www.acr.org/Clinical-Resources/Reporting-and-Data-Systems/Lung-Rads
- Oudkerk M, Liu S, Heuvelmans MA, Walter JE, Field JK. Lung cancer LDCT screening and mortality reduction - evidence, pitfalls and future perspectives. Nat Rev Clin Oncol 2021;18:135-51. [Crossref] [PubMed]
- Zhang BW, Zhang Y, Ye JD, Qiang JW. Use of relative CT values to evaluate the invasiveness of pulmonary subsolid nodules in patients with emphysema. Quant Imaging Med Surg 2021;11:204-14. [Crossref] [PubMed]
- Ren H, Liu F, Xu L, Sun F, Cai J, Yu L, Guan W, Xiao H, Li H, Yu H. Predicting the histological invasiveness of pulmonary adenocarcinoma manifesting as persistent pure ground-glass nodules by ultra-high-resolution CT target scanning in the lateral or oblique body position. Quant Imaging Med Surg 2021;11:4042-55. [Crossref] [PubMed]
- Noguchi M. Stepwise progression of pulmonary adenocarcinoma--clinical and molecular implications. Cancer Metastasis Rev 2010;29:15-21. [Crossref] [PubMed]
- Carmeliet P, Jain RK. Molecular mechanisms and clinical applications of angiogenesis. Nature 2011;473:298-307. [Crossref] [PubMed]
- Hanahan D, Weinberg RA. Hallmarks of cancer: the next generation. Cell 2011;144:646-74. [Crossref] [PubMed]
- Noguchi M, Morikawa A, Kawasaki M, Matsuno Y, Yamada T, Hirohashi S, Kondo H, Shimosato Y. Small adenocarcinoma of the lung. Histologic characteristics and prognosis. Cancer 1995;75:2844-52. [Crossref] [PubMed]
- Takahashi S, Tanaka N, Okimoto T, Tanaka T, Ueda K, Matsumoto T, Ashizawa K, Kunihiro Y, Kido S, Matsunaga N. Long term follow-up for small pure ground-glass nodules: implications of determining an optimum follow-up period and high-resolution CT findings to predict the growth of nodules. Jpn J Radiol 2012;30:206-17. [Crossref] [PubMed]
- Saito H, Yamada K, Hamanaka N, Oshita F, Ito H, Nakayama H, Yokose T, Kameda Y, Noda K. Initial findings and progression of lung adenocarcinoma on serial computed tomography scans. J Comput Assist Tomogr 2009;33:42-8. [Crossref] [PubMed]
- Yeh YC, Nitadori J, Kadota K, Yoshizawa A, Rekhtman N, Moreira AL, Sima CS, Rusch VW, Adusumilli PS, Travis WD. Using frozen section to identify histological patterns in stage I lung adenocarcinoma of ≤ 3 cm: accuracy and interobserver agreement. Histopathology 2015;66:922-38. [Crossref] [PubMed]
- Zhu E, Xie H, Dai C, Zhang L, Huang Y, Dong Z, Guo J, Su H, Ren Y, Shi P, Fu R, Qin S, Wu C, Chen C. Intraoperatively measured tumor size and frozen section results should be considered jointly to predict the final pathology for lung adenocarcinoma. Mod Pathol 2018;31:1391-9. [Crossref] [PubMed]
- Suzuki K, Koike T, Asakawa T, Kusumoto M, Asamura H, Nagai K, Tada H, Mitsudomi T, Tsuboi M, Shibata T, Fukuda H, Kato HJapan Lung Cancer Surgical Study Group. (JCOG LCSSG). A prospective radiological study of thin-section computed tomography to predict pathological noninvasiveness in peripheral clinical IA lung cancer (Japan Clinical Oncology Group 0201). J Thorac Oncol 2011;6:751-6. [Crossref] [PubMed]