Development and validation of an MRI-based nomogram for the preoperative prediction of tumor mutational burden in lower-grade gliomas
Introduction
Lower-grade gliomas (LGGs) are a heterogeneous group of primary glial brain tumors with highly variable overall survival (OS) (1-3). The management of LGG (WHO grade II and III) is still controversial because LGG is molecularly distinct. Patients with similar clinical features may have diverse outcomes (4,5). However, the clinical significance of some newer biomarkers discovered by genomic studies has not been fully investigated (6,7). Intratumor genetic heterogeneity plays a pivotal role in driving disease progression and therapeutic resistance in LGG (8,9). Intratumor heterogeneity has been linked to metastatic potential and is likely to be an important prognostic feature of human cancer.
While treating cancer with immunotherapy can be highly effective, only some patients respond to these treatments (10,11). Given the promise that these agents have demonstrated in the treatment of refractory disease and the durable responses that occur in some patients, there is great interest in identifying the patients who are most likely to benefit from these therapies. One emerging biomarker for the response to anti-programmed cell death protein 1 (PD-1) therapy is tumor mutational burden (TMB). TMB, the total number of somatic coding mutations in a tumor, is emerging as a promising biomarker for immunotherapy response in cancer patients (12,13). High TMB is an emerging biomarker of sensitivity to immune checkpoint inhibitors and has been shown to be more significantly associated with the response to PD-1 inhibitors and programmed death-ligand 1 (PD-L1) blockade immunotherapy than PD-1 or PD-L1 expression, as measured by immunohistochemistry (IHC) (14,15).
From diagnostics to prognosis to response prediction, new applications for radiogenomics are rapidly being developed (16-18). Radiogenomics aims to correlate radiological features with gene mutations, gene expression patterns, and other genome-related features and is designed to capture the intrinsic tumor heterogeneity and facilitate a deeper understanding of tumor biology (18,19). However, no study has investigated the associations among TMB, magnetic resonance imaging (MRI) features and OS in LGG. In this study, we used TMB to measure heterogeneity and investigated its correlation with MRI features and survival outcomes.
Methods
Patients
The study was conducted in accordance with the Declaration of Helsinki (as revised in 2013). All patient data was acquired from the published The Cancer Genome Atlas LGG (TCGA-LGG) project and within this publication it is stated “Specimens were obtained from patients, with appropriate consent from institutional review boards” (http://cancergenome.nih.gov/). The publicly available clinical data used in this study were released by TCGA. For each patient, the determination of age, laterality, gender, race category, method of sample procurement, first presenting symptom, seizure, neoplasm histologic grade and histological type were based on file ‘‘nationwidechildrens.org_clinical_patient_lgg’’ downloaded from https://tcga-data.nci.nih.gov/tcga/dataAccessMatrix.htm. For the subset of the LGG patients from TCGA, the MR exams were made available by The Cancer Imaging Archive (TCIA) (https://wiki.cancerimagingarchive.net/). All adult patients with LGG were retrospectively reviewed. Patients were only included if they met all of the following inclusion criteria: (I) LGG was confirmed by histopathology; (II) patients underwent MRI (TCIA); and (III) TMB values were available (TCGA). The TMB value of each patient was derived from previously published articles (20). A total of 168 patients (60 with high-TMB gliomas and 108 with low-TMB gliomas) fit the inclusion criteria.
Radiologist review of the MR image features
By referring to previous studies (21-23) the following 20 qualitative imaging descriptors were evaluated: (I) volume (<60 cm3 versus ≥60 cm3); (II) multifocality (no versus yes); (III) intratumoral hemorrhage (no versus yes); (IV) enhancing margin (well defined margin versus poorly-defined margin); (V) necrosis (no versus yes); (VI) proportion contrast-enhanced (CE) tumor (<5% versus ≥5%); (VII) subventricular zone (SVZ) involvement (no versus yes): the tumors located in close contact with the SVZ are classified as SVZ +, while the tumors located distantly from the SVZ are classified as SVZ −; (VIII) multilobar lesions (no versus yes); (IX) extranodular growth (no versus yes); (X) T1 + C/T2 mismatch (no versus yes); (XI) depth (< median or ≥ median); (XII) width (< median or ≥ median); (XIII) length (< median or ≥ median); (XIV) volume (< median or ≥ median); (XV) length (< median or ≥ median); (XVI) the shortest distance between the edge of the lateral and the tumor centroid (CS) (≤30 mm or >30 mm); (XVII) length-width ratio (< median or ≥ median); (XVIII) length-depth ratio (< median or ≥ median); (XIX) width-depth ratio (< median or ≥ median); (XX) location (frontal lobe versus other).
The readers independently made their classifications based on the MR images, and in cases of disagreement, a consensus was reached after discussion. Both evaluators were blinded to patient’s data. The interobserver and intraobserver reproducibility of LGG MR features has been reported in our previous work (24).
Statistical analysis
The patients were divided into low and high TMB groups according to previously published articles. The OS data of each patient were derived from the TCGA. The relationship between intratumor genetic heterogeneity and OS was assessed using Kaplan-Meier analysis and time-dependent receiver operating characteristic (tdROC) analysis (R package). The correlations between TMB and MRI features were explored. A univariate analysis of variables was carried out using the chi-square test (or Fisher’s exact test as appropriate). Binary logistic regression analyses (version 23.0; SPSS Company, Chicago, IL, USA) were performed to identify independent factors of intratumoral genetic heterogeneity (high-TMB group or low-TMB group). The estimated odds ratio (OR) was reported. Two-sided P<0.05 indicated a significant difference. The discrimination of the models was assessed. It was measured using the receiver operating characteristic curve and summarized by the area under the curve (AUC). An AUC of 1.0 indicates perfect concordance, whereas an AUC of 0.5 indicates no relationship. Finally, we performed tenfold cross validation (Figure 1).
Results
Of the 271 patients from the TCGA, 94 had high-TMB gliomas and 177 had low-TMB gliomas. High TBM-subtype was associated with shortest median OS (high vs. low: 50.9 vs. 95.6 months, P<0.05). OS were assessed using Kaplan-Meier analysis and tdROC analysis. Kaplan-Meier analysis (Figure 2A) indicated that there was a significant difference in OS between the high TMB tumors and low TMB tumors. The tdROC (Figure 2B) for the high TMB tumors was 0.74 (95% CI: 0.61–0.86) for survival at 12 months, and 0.71 (95% CI: 0.60–0.82) for survival at 24 months.
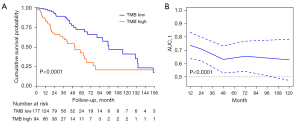
Of the 168 patients from the TCIA, 60 had high-TMB gliomas and 108 had low-TMB gliomas. The relations of selected patient clinical characteristics and MR characteristics to TMB groups are shown in Tables 1,2. Correlation matrix (Figure 3) yielded distinct groups of TMB status and qualitative MRI features of LGG. In univariate analysis, degree of enhancement, proportion CE tumor, extranodular growth, T1 + C/T2 mismatch, location, SVZ and length-width ratio were significant independent predictors of high TMB tumors (P<0.05).
Table 1
Characteristics | Total (n=168) | TMB group | χ2/t | P | |
---|---|---|---|---|---|
TMB low | TMB high | ||||
Histological type, no. (%) | 1.978 | 0.372 | |||
Oligodendroglioma | 69 (41.82) | 46 (42.59) | 23 (40.35) | ||
Astrocytoma | 56 (33.94) | 33 (30.56) | 23 (40.35) | ||
Oligoastrocytoma | 40 (24.24) | 29 (26.85) | 11 (19.3) | ||
Neoplasm histologic grade, no. (%) | 11.435 | 0.001 | |||
GII | 82 (49.7) | 64 (59.26) | 18 (31.58) | ||
GIII | 83 (50.3) | 44 (40.74) | 39 (68.42) | ||
Age at diagnosis, years | −8.258 | <0.001 | |||
Mean | 43.86±13.53 | 38.54±11.65 | 53.95±10.9 | ||
Range | 20–75 | 20–74 | 22–75 | ||
Gender, no. (%) | 3.942 | 0.047 | |||
Female | 78 (47.27) | 45 (41.67) | 33 (57.89) | ||
Male | 87 (52.73) | 63 (58.33) | 24 (42.11) | ||
Race, no. (%) | – | 0.302 | |||
American Indian or Alaska native | 1 (0.61) | 1 (0.93) | 0 (0) | ||
Black or African American | 11 (6.71) | 5 (4.67) | 6 (10.53) | ||
White | 152 (92.68) | 101 (94.39) | 51 (89.47) | ||
Family history of cancer, no. (%) | 0.88 | 0.348 | |||
No | 67 (53.60) | 48 (56.47) | 19 (47.50) | ||
Yes | 58 (46.40) | 37 (43.53) | 21 (52.50) | ||
Method of sample procurement, no. (%) | – | 0.005 | |||
Open biopsy | 6 (3.57) | 4 (3.70) | 2 (3.33) | ||
Subtotal resection | 55 (32.74) | 26 (24.07) | 29 (48.33) | ||
Gross total resection | 107 (63.69) | 78 (72.22) | 29 (48.33) | ||
First presenting symptom, no. (%) | 7.64 | 0.106 | |||
Headaches | 40 (25.00) | 30 (28.57) | 10 (18.18) | ||
Mental status change | 15 (9.38) | 6 (5.71) | 9 (16.36) | ||
Motor or movement change | 11 (6.88) | 9 (8.57) | 2 (3.64) | ||
Seizure | 85 (53.13) | 55 (52.38) | 30 (54.55) | ||
Sensory or visual change | 9 (5.63) | 5 (4.76) | 4 (7.27) | ||
Laterality, no. (%) | – | 0.943 | |||
Left | 79 (48.47) | 52 (49.06) | 27 (47.37) | ||
Midline | 3 (1.84) | 2 (1.89) | 1 (1.75) | ||
Right | 81 (49.69) | 52 (49.06) | 29 (50.88) | ||
IDH/1p19q subtype, no. (%) | 22.295 | <0.001 | |||
IDHmut-non-codel | 85 (51.20) | 63 (59.43) | 22 (36.67) | ||
IDHmut-codel | 43 (25.90) | 31 (29.25) | 12 (20.00) | ||
IDHwt | 38 (22.89) | 12 (11.32) | 26 (43.33) |
LGG, lower-grade glioma; TMB, tumor mutational burden; IDHmut-non-codel, LGG with IDH mutation and no 1p/19q codeletion; IDHmut-codel, LGG with IDH mutation and 1p/19q codeletion; IDHwt, LGG with wild-type IDH.
Table 2
MR features | Total (n=168) | TMB group | χ2 | P | |
---|---|---|---|---|---|
TMB low | TMB high | ||||
Necrosis | 0.149 | 0.700 | |||
Negative | 31 (18.45) | 19 (17.59) | 12 (20.00) | ||
Positive | 137 (81.55) | 89 (82.41) | 48 (80.00) | ||
Multifocality | 10.388 | 0.001 | |||
Negative | 161 (95.83) | 108 (100.00) | 53 (88.33) | ||
Positive | 7 (4.17) | 0 (0) | 7 (11.67) | ||
Hemorrhage | 0.101 | 0.750 | |||
Negative | 142 (84.52) | 92 (85.19) | 50 (83.33) | ||
Positive | 26 (15.48) | 16 (14.81) | 10 (16.67) | ||
Degree of enhancement | 8.925 | 0.003 | |||
Negative | 68 (43.04) | 52 (52.00) | 16 (27.59) | ||
Positive | 90 (56.96) | 48 (48.00) | 42 (72.41) | ||
Proportion CE tumor | 12.984 | <0.001 | |||
Negative | 102 (64.56) | 75 (75.00) | 27 (46.55) | ||
Positive | 56 (35.44) | 25 (25.00) | 31 (53.45) | ||
Enhancing margin | 0.024 | 0.876 | |||
Well-defined | 42 (26.58) | 27 (27.00) | 15 (25.86) | ||
Poorly-defined | 116 (73.42) | 73 (73.00) | 43 (74.14) | ||
Extranodular growth | 19.49 | <0.001 | |||
Negative | 129 (81.65) | 92 (92.00) | 37 (63.79) | ||
Positive | 29 (18.35) | 8 (8.00) | 21 (36.21) | ||
T1 + C/T2 mismatch | 6.763 | 0.009 | |||
Negative | 135 (85.44) | 91 (91.00) | 44 (75.86) | ||
Positive | 23 (14.56) | 9 (9.00) | 14 (24.14) | ||
CS | 1.744 | 0.187 | |||
>30 mm | 86 (51.81) | 59 (55.66) | 27 (45.00) | ||
≤30 mm | 80 (48.19) | 47 (44.34) | 33 (55.00) | ||
Location | 5.169 | 0.023 | |||
Other | 104 (61.90) | 60 (55.56) | 44 (73.33) | ||
Frontal lobe | 64 (38.10) | 48 (44.44) | 16 (26.67) | ||
SVZ | 7.413 | 0.006 | |||
Negative | 59 (35.12) | 46 (42.59) | 13 (21.67) | ||
Positive | 109 (64.88) | 62 (57.41) | 47 (78.33) | ||
Volume | 0.162 | 0.687 | |||
<60 cm3 | 50 (29.76) | 31 (28.70) | 19 (31.67) | ||
≥60 cm3 | 118 (70.24) | 77 (71.30) | 41 (68.33) | ||
Intratumoral vascular | 0.001 | 0.973 | |||
Negative | 4 (2.53) | 2 (2.00) | 2 (3.45) | ||
Positive | 154 (97.47) | 98 (98.00) | 56 (96.55) | ||
Length | 3.303 | 0.069 | |||
< median | 83 (49.4) | 59 (54.63) | 24 (40.00) | ||
≥ median | 85 (50.6) | 49 (45.37) | 36 (60.00) | ||
Width | 0.043 | 0.836 | |||
< median | 83 (49.40) | 54 (50.00) | 29 (48.33) | ||
≥ median | 85 (50.60) | 54 (50.00) | 31 (51.67) | ||
Depth | 0.013 | 0.908 | |||
< median | 83 (49.40) | 53 (49.07) | 30 (50.00) | ||
≥ median | 85 (50.60) | 55 (50.93) | 30 (50.00) | ||
Length-width ratio | 4.192 | 0.041 | |||
< median | 85 (50.60) | 61 (56.48) | 24 (40.00) | ||
≥ median | 83 (49.40) | 47 (43.52) | 36 (60.00) | ||
Length-depth ratio | 0.305 | 0.581 | |||
< median | 86 (51.19) | 57 (52.78) | 29 (48.33) | ||
≥ median | 82 (48.81) | 51 (47.22) | 31 (51.67) | ||
Length-depth ratio | 0.014 | 0.907 | |||
< median | 84 (50.60) | 54 (50.94) | 30 (50.00) | ||
≥ median | 82 (49.40) | 52 (49.06) | 30 (50.00) | ||
Volume | 0.28 | 0.597 | |||
< median | 83 (49.40) | 55 (50.93) | 28 (46.67) | ||
≥ median | 85 (50.60) | 53 (49.07) | 32 (53.33) |
MR, magnetic resonance; TMB, tumor mutational burden; CE, contrast-enhanced; CS, the shortest distance between the edge of the lateral and the tumor centroid; SVZ, subventricular zone.
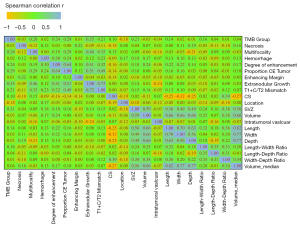
Multivariate logistic regression analysis confirmed that three risk factors (extranodular growth: OR: 8.367, 95% CI: 3.153–22.199, P<0.01; length-width ratio ≥ median: OR: 1.947, 95% CI: 1.025–3.697, P<0.05; frontal lobe: OR: 0.455, 95% CI: 0.229–0.903, P<0.05) were significant independent predictors of high TMB tumors (Table 3). Extranodular growth, nonfrontal lobe tumors and length-width ratio ≥ median were associated with a significantly higher incidence of high TMB tumors (Figure 4). After multivariate analysis, the nomogram was built. The nomogram (Figure 5) showed good calibration and discrimination. The AUC for the ROC curve was 0.736 (95% CI: 0.655–0.817) for high TMB tumors (Figure 6A). Decision curve analysis (DCA) demonstrated that the nomogram was clinically useful (Figure 6B). The average accuracy of the tenfold cross validation was 71.6% for high TMB tumors.
Table 3
MR features | Univariate logistic regression | Multivariate logistic regression |
---|---|---|
Necrosis | ||
Negative | Reference | |
Positive | 0.854 (0.382–1.907) | |
Multifocality | ||
Negative | Reference | |
Positive | – | |
Hemorrhage | ||
Negative | Reference | |
Positive | 1.15 (0.486–2.723) | |
Degree of enhancement | ||
Negative | Reference | |
Positive | 2.844 (1.417–5.708)** | |
Proportion CE tumor | ||
Negative | Reference | |
Positive | 3.444 (1.734–6.842)*** | |
Enhancing margin | ||
Well-defined | Reference | |
Poorly-defined | 1.06 (0.508–2.211) | |
Extranodular growth | ||
Negative | Reference | |
Positive | 6.527 (2.656–16.042)*** | 8.367 (3.153–22.199)** |
T1 + C/T2 mismatch | ||
Negative | Reference | |
Positive | 3.217 (1.293–8.004)* | |
CS | ||
>30 mm | Reference | |
≤30 mm | 1.534 (0.812–2.9) | |
Location | ||
Other | Reference | |
Frontal lobe | 0.455 (0.229–0.903)* | 0.458 (0.212–0.991)* |
SVZ | ||
Negative | Reference | |
Positive | 2.682 (1.302–5.527)** | |
Volume | ||
<60 cm3 | Reference | |
≥60 cm3 | 0.869 (0.438–1.724) | |
Intratumoral vascular | ||
Negative | Reference | |
Positive | 0.571 (0.078–4.169) | |
Length | ||
< median | Reference | |
≥ median | 1.806 (0.952–3.427) | |
Width | ||
< median | Reference | |
≥ median | 1.069 (0.569–2.01) | |
Depth | ||
< median | Reference | |
≥ median | 0.964 (0.513–1.812) | |
Length-width ratio | ||
< median | Reference | |
≥ median | 1.947 (1.025–3.697)* | 2.39 (1.128–5.066)* |
Length-depth ratio | ||
< median | Reference | |
≥ median | 1.195 (0.635–2.247) | |
Length-depth ratio | ||
< median | Reference | |
≥ median | 1.038 (0.551–1.956) | |
Volume | ||
< median | Reference | |
≥ median | 1.186 (0.63–2.232) |
*, P<0.05; **, P<0.01; ***, P<0.001. ORs, odds ratios; MR, magnetic resonance; CE, contrast-enhanced; CS, the shortest distance between the edge of the lateral and the tumor centroid; SVZ, subventricular zone.
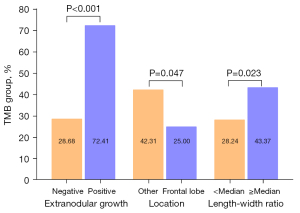
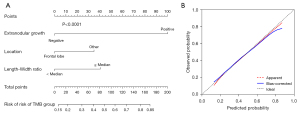
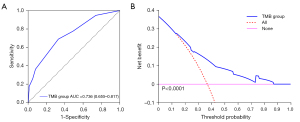
Subgroup analysis (Table 4) of IDHmut-non-codel subtype (n=85) found that SVZ and extranodular growth were significant independent predictors of high TMB tumors (P<0.05). Multivariate logistic regression analysis confirmed that extranodular growth (OR: 4.297, 95% CI: 1.031–17.916, P<0.05) was still the independent predictive factor for TMB tumors.
Table 4
MR features | Univariate logistic regression | Multivariate logistic regression |
---|---|---|
Necrosis | ||
Negative | Reference | |
Positive | 1.059 (0.303–3.706) | |
Hemorrhage | ||
Negative | Reference | |
Positive | 0.702 (0.074–6.645) | |
Degree of enhancement | ||
Negative | Reference | |
Positive | 1.806 (0.638–5.117) | |
Proportion CE tumor | ||
Negative | Reference | |
Positive | 1.402 (0.495–3.968) | |
Enhancing margin | ||
Well-defined | Reference | |
Poorly-defined | 0.941 (0.225–3.939) | |
Extranodular growth | ||
Negative | Reference | |
Positive | 4.297 (1.031–17.916)* | 4.297 (1.031–17.916)* |
T1 + C/T2 mismatch | ||
Negative | Reference | |
Positive | 2.292 (0.468–11.224) | |
CS | ||
>30 mm | Reference | |
≤30 mm | 0.994 (0.362–2.725) | |
Location | ||
Other | Reference | |
Frontal lobe | 0.447 (0.146–1.367) | |
SVZ | ||
Negative | Reference | |
Positive | 4.167 (1.115–15.568)* | |
Volume | ||
<60 cm3 | Reference | |
≥60 cm3 | 3.404 (0.715–16.206) | |
Intratumoral vascular | ||
Negative | Reference | |
Positive | 0.702 (0.060–8.164) | |
Length | ||
< median | Reference | |
≥ median | 2.900 (0.952–8.83) | |
Width | ||
< median | Reference | |
≥ median | 1.641 (0.564–4.771) | |
Depth | ||
< median | Reference | |
≥ median | 2.275 (0.787–6.572) | |
Length-width ratio | ||
< median | Reference | |
≥ median | 2.679 (0.960–7.47) | |
Length-depth ratio | ||
< median | Reference | |
≥ median | 1.313 (0.491–3.510) | |
Width-depth ratio | ||
< median | Reference | |
≥ median | 1.239 (0.468–3.28) | |
Volume | ||
< median | Reference | |
≥ median | 2.55 (0.836–7.776) |
*, P<0.05. ORs, odds ratios; IDHmut-non-codel, lower-grade glioma with IDH mutation and no 1p/19q codeletion; MR, magnetic resonance; CE, contrast-enhanced; CS, the shortest distance between the edge of the lateral and the tumor centroid; SVZ, subventricular zone.
Discussion
LGG form a biologically heterogeneous group of tumors. Intratumoral genetic heterogeneity, variation within a patient’s individual tumor cells, is increasingly seen as a molecular mechanism underlying therapeutic failure and treatment resistance (25). Recently, checkpoint inhibitors (PD-L1 or PD-1 inhibitors) have emerged as potential therapeutic options for adult and pediatric gliomas (26,27). TMB is well known to predict the response to immune checkpoint inhibitors (28,29). Highly mutated tumors are thought to harbor an increased neoantigen burden, making them immunogenic and responsive to immunotherapy (30).
Predicting TMB tumors in low-grade glioma patients is of great clinical importance, as it can potentially help better manage such patients and develop personalized treatment plans. The idea of using MRI is also appealing, as it is already part of the standard clinical diagnostic routine for this population, so the lack of an additional cost/procedure may make potential clinical translation easier. A meta-analysis (n=103,078) showed that for various cancer patients treated with surgery or chemotherapy, the prognostic role of TMB was dependent on cancer type. The meta-analysis (n=103,078) showed that regarding the response to immune checkpoint therapy, the high-TMB group had a significantly higher response rate than the low-TMB group.
There are a number of reports regarding MRI-based prediction models for LGG. Wei and coworkers found that a radiomics signature accurately predicted MGMT promoter methylation in patients with astrocytomas and achieved survival stratification for TMZ chemotherapy (31). Broen and coworkers confirmed that among nonenhancing LGG, the T2-FLAIR mismatch sign represents a highly specific imaging marker for IDH-mutant astrocytoma (32). Wang and coworkers found that a radiomics signature was independent of clinicopathologic data and was a noninvasive pretreatment predictor for LGG patient survival (33). Tay and coworkers confirmed the possibility of a primary cerebral neoplasm representing a protoplasmic astrocytoma in a patient with a large frontal or temporal tumor that has a very high signal on T2 with a large proportion of the tumor showing substantial T2 FLAIR suppression (34).
There are a number of reports regarding the relationship between prognosis and TMB. Alghamri and coworkers (35) found that TMB is negatively associated with clinical outcomes in metastatic patients with EGFR-mutant lung cancer treated with EGFR tyrosine kinase inhibitors. Samstein and coworkers (36) demonstrated that TMB is associated with improved survival in patients receiving immune checkpoint inhibitors across a wide variety of cancer types. Hwang and coworkers (37) found that the mutational burden of primary neuroblastoma may be useful in combination with conventional risk factors to optimize risk stratification and guide treatment decisions, pending prospective validation. Wang and coworkers (20) found that TMB is associated with poor outcomes in diffuse glioma, and the high proliferative activity in the high-TMB group could account for the shorter survival of these patients. However, no study has investigated associations between TMB and MRI features in LGG.
Radiogenomics is a computational discipline that identifies correlations between cross-sectional imaging features and tissue-based molecular data (38,39). Tumor size, tumor location, composition, and MRI features tend to demonstrate significant associations with genomic and molecular factors, likely related to the cell of origin and growth characteristics (39). Tumor heterogeneity (8) has been explored at the histological and genetic levels, and increased levels of intratumor genetic heterogeneity have been reported to be associated with adverse clinical outcomes. In the current study, the correlations between TMB status and MRI features were explored. Multivariate logistic regression analysis confirmed that extranodular growth, nonfrontal lobe tumors and length-width ratio ≥ median can be conveniently used to facilitate the prediction of high-TMB tumors. The nomogram showed good discrimination (AUC: 0.732) for predicting high-TMB gliomas. To our knowledge, this is the first study focusing on the relationships among TMB status, MR features and OS in patients with LGG.
A meta-analysis showed that codeletion of 1p and 19q is associated with better survival rates in glioma (40). Another meta-analysis revealed that patients with glioma harboring IDH mutations have improved OS and progression free survival (PFS), especially patients with WHO grade III and grade II–III (41). TCGA Research Network classified LGG into three categories: LGG with IDH mutation and no 1p/19q codeletion (IDHmut-non-codel), LGG with IDH mutation and 1p/19q codeletion (IDHmut-codel), and LGG with wild-type IDH (IDHwt). The IDHmut-noncodel subtype is linked to the best OS. Subgroup analysis of the IDHmut-noncodel subtype (n=85) confirmed that extranodular growth was still an independent predictive factor of TMB tumors.
This study has several limitations. Tissue samples for the TCGA were collected from many sites and analyzed to reach accrual targets, with usually approximately five hundred specimens per cancer type. For this reason, the MR image data sets are heterogeneous in terms of MR scanner modalities, manufacturers and MR acquisition protocols. However, similar to other articles published using TCGA datasets (42), the lesions were relatively large. In addition, an average accuracy of 71.6% was obtained based on the tenfold cross-validation method for high-TMB tumors. The result of the value was good. Therefore, we believe that our results are not largely affected by this limitation.
In conclusion, our results indicated that distinct OS disadvantages were associated with the high TMB group. Additionally, extranodular growth, nonfrontal lobe tumors and length-width ratio ≥ median can be conveniently used to facilitate the prediction of high-TMB tumors. The calibration curve and DCA demonstrated the usefulness of the proposed nomogram. The average accuracy of the tenfold cross validation was 71.6% for high-TMB tumors.
Acknowledgments
Funding: This study was supported by National Natural Science Foundation of China (grant No. 81801688) and Traditional Chinese Medicine Bureau of Guangdong Province (grant No. 20181116).
Footnote
Conflicts of Interest: All authors have completed the ICMJE uniform disclosure form (available at https://dx.doi.org/10.21037/qims-21-300). The authors have no conflicts of interest to declare.
Ethical Statement: The authors are accountable for all aspects of the work in ensuring that questions related to the accuracy or integrity of any part of the work are appropriately investigated and resolved. The study was conducted in accordance with the Declaration of Helsinki (as revised in 2013). All patient data was acquired from the published TCGA-LGG project and within this publication it is stated “Specimens were obtained from patients, with appropriate consent from institutional review boards” (http://cancergenome.nih.gov/).
Open Access Statement: This is an Open Access article distributed in accordance with the Creative Commons Attribution-NonCommercial-NoDerivs 4.0 International License (CC BY-NC-ND 4.0), which permits the non-commercial replication and distribution of the article with the strict proviso that no changes or edits are made and the original work is properly cited (including links to both the formal publication through the relevant DOI and the license). See: https://creativecommons.org/licenses/by-nc-nd/4.0/.
References
- Darlix A, Gozé C, Rigau V, Bauchet L, Taillandier L, Duffau H. The etiopathogenesis of diffuse low-grade gliomas. Crit Rev Oncol Hematol 2017;109:51-62. [Crossref] [PubMed]
- Ferracci FX, Michaud K, Duffau H. The landscape of postsurgical recurrence patterns in diffuse low-grade gliomas. Crit Rev Oncol Hematol 2019;138:148-55. [Crossref] [PubMed]
- Delgado-López PD, Corrales-García EM, Martino J, Lastra-Aras E, Dueñas-Polo MT. Diffuse low-grade glioma: a review on the new molecular classification, natural history and current management strategies. Clin Transl Oncol 2017;19:931-44. [Crossref] [PubMed]
- Morshed RA, Young JS, Hervey-Jumper SL, Berger MS. The management of low-grade gliomas in adults. J Neurosurg Sci 2019;63:450-7. [Crossref] [PubMed]
- Smits A, Jakola AS. Clinical Presentation, Natural History, and Prognosis of Diffuse Low-Grade Gliomas. Neurosurg Clin N Am 2019;30:35-42. [Crossref] [PubMed]
- Jakola AS, Unsgård G, Kloster R, Solheim O. Diffuse low-grade gliomas. J Neurosurg 2013;119:1354-5. [Crossref] [PubMed]
- Garcia CR, Slone SA, Pittman T, St Clair WH, Lightner DD, Villano JL. Comprehensive evaluation of treatment and outcomes of low-grade diffuse gliomas. PLoS One 2018;13:e0203639. [Crossref] [PubMed]
- McGranahan N, Swanton C. Clonal Heterogeneity and Tumor Evolution: Past, Present, and the Future. Cell 2017;168:613-28. [Crossref] [PubMed]
- Prasetyanti PR, Medema JP. Intra-tumor heterogeneity from a cancer stem cell perspective. Mol Cancer 2017;16:41. [Crossref] [PubMed]
- Prieto PA, Yang JC, Sherry RM, Hughes MS, Kammula US, White DE, Levy CL, Rosenberg SA, Phan GQ. CTLA-4 blockade with ipilimumab: long-term follow-up of 177 patients with metastatic melanoma. Clin Cancer Res 2012;18:2039-47. [Crossref] [PubMed]
- Crow J, Samuel G, Godwin AK. Beyond tumor mutational burden: potential and limitations in using exosomes to predict response to immunotherapy. Expert Rev Mol Diagn 2019;19:1079-88. [Crossref] [PubMed]
- Fancello L, Gandini S, Pelicci PG, Mazzarella L. Tumor mutational burden quantification from targeted gene panels: major advancements and challenges. J Immunother Cancer 2019;7:183. [Crossref] [PubMed]
- Chan TA, Yarchoan M, Jaffee E, Swanton C, Quezada SA, Stenzinger A, Peters S. Development of tumor mutation burden as an immunotherapy biomarker: utility for the oncology clinic. Ann Oncol 2019;30:44-56. [Crossref] [PubMed]
- Chalmers ZR, Connelly CF, Fabrizio D, Gay L, Ali SM, Ennis R, et al. Analysis of 100,000 human cancer genomes reveals the landscape of tumor mutational burden. Genome Med 2017;9:34. [Crossref] [PubMed]
- Hellmann MD, Paz-Ares L. Lung Cancer with a High Tumor Mutational Burden. N Engl J Med 2018;379:1093-4. [Crossref] [PubMed]
- Li M, Li X, Guo Y, Miao Z, Liu X, Guo S, Zhang H. Development and assessment of an individualized nomogram to predict colorectal cancer liver metastases. Quant Imaging Med Surg 2020;10:397-414. [Crossref] [PubMed]
- Attanasio S, Forte SM, Restante G, Gabelloni M, Guglielmi G, Neri E. Artificial intelligence, radiomics and other horizons in body composition assessment. Quant Imaging Med Surg 2020;10:1650-60. [Crossref] [PubMed]
- Bodalal Z, Trebeschi S, Nguyen-Kim TDL, Schats W, Beets-Tan R. Radiogenomics: bridging imaging and genomics. Abdom Radiol (NY) 2019;44:1960-84. [Crossref] [PubMed]
- Pinker K, Chin J, Melsaether AN, Morris EA, Moy L. Precision Medicine and Radiogenomics in Breast Cancer: New Approaches toward Diagnosis and Treatment. Radiology 2018;287:732-47. [Crossref] [PubMed]
- Wang L, Ge J, Lan Y, Shi Y, Luo Y, Tan Y, Liang M, Deng S, Zhang X, Wang W, Tan Y, Xu Y, Luo T. Tumor mutational burden is associated with poor outcomes in diffuse glioma. BMC Cancer 2020;20:213. [Crossref] [PubMed]
- Peng X, Yishuang C, Kaizhou Z, Xiao L, Ma C. Conventional Magnetic Resonance Features for Predicting 1p19q Codeletion Status of World Health Organization Grade II and III Diffuse Gliomas. J Comput Assist Tomogr 2019;43:269-76. [Crossref] [PubMed]
- Liu Z, Liu H, Liu Z, Zhang J. Oligodendroglial tumours: subventricular zone involvement and seizure history are associated with CIC mutation status. BMC Neurol 2019;19:134. [Crossref] [PubMed]
- Park YW, Han K, Ahn SS, Bae S, Choi YS, Chang JH, Kim SH, Kang SG, Lee SK. Prediction of IDH1-Mutation and 1p/19q-Codeletion Status Using Preoperative MR Imaging Phenotypes in Lower Grade Gliomas. AJNR Am J Neuroradiol 2018;39:37-42. [Crossref] [PubMed]
- Zhang S, Wu S, Wan Y, Ye Y, Zhang Y, Ma Z, Guo Q, Zhang H, Xu L. Development of MR-based preoperative nomograms predicting DNA copy number subtype in lower grade gliomas with prognostic implication. Eur Radiol 2021;31:2094-105. [Crossref] [PubMed]
- Bouffet E, Larouche V, Campbell BB, Merico D, de Borja R, Aronson M, et al. Immune Checkpoint Inhibition for Hypermutant Glioblastoma Multiforme Resulting From Germline Biallelic Mismatch Repair Deficiency. J Clin Oncol 2016;34:2206-11. [Crossref] [PubMed]
- Bloch O, Crane CA, Kaur R, Safaee M, Rutkowski MJ, Parsa AT. Gliomas promote immunosuppression through induction of B7-H1 expression in tumor-associated macrophages. Clin Cancer Res 2013;19:3165-75. [Crossref] [PubMed]
- Preusser M, Berghoff AS, Wick W, Weller M. Clinical Neuropathology mini-review 6-2015: PD-L1: emerging biomarker in glioblastoma? Clin Neuropathol 2015;34:313-21. [Crossref] [PubMed]
- Liu L, Bai X, Wang J, Tang XR, Wu DH, Du SS, Du XJ, Zhang YW, Zhu HB, Fang Y, Guo ZQ, Zeng Q, Guo XJ, Liu Z, Dong ZY. Combination of TMB and CNA Stratifies Prognostic and Predictive Responses to Immunotherapy Across Metastatic Cancer. Clin Cancer Res 2019;25:7413-23. [Crossref] [PubMed]
- Schrock AB, Ouyang C, Sandhu J, Sokol E, Jin D, Ross JS, Miller VA, Lim D, Amanam I, Chao J, Catenacci D, Cho M, Braiteh F, Klempner SJ, Ali SM, Fakih M. Tumor mutational burden is predictive of response to immune checkpoint inhibitors in MSI-high metastatic colorectal cancer. Ann Oncol 2019;30:1096-103. [Crossref] [PubMed]
- McGranahan N, Furness AJ, Rosenthal R, Ramskov S, Lyngaa R, Saini SK, et al. Clonal neoantigens elicit T cell immunoreactivity and sensitivity to immune checkpoint blockade. Science 2016;351:1463-9. [Crossref] [PubMed]
- Wei J, Yang G, Hao X, Gu D, Tan Y, Wang X, Dong D, Zhang S, Wang L, Zhang H, Tian J. A multi-sequence and habitat-based MRI radiomics signature for preoperative prediction of MGMT promoter methylation in astrocytomas with prognostic implication. Eur Radiol 2019;29:877-88. [Crossref] [PubMed]
- Broen MPG, Smits M, Wijnenga MMJ, Dubbink HJ, Anten MHME, Schijns OEMG, Beckervordersandforth J, Postma AA, van den Bent MJ. The T2-FLAIR mismatch sign as an imaging marker for non-enhancing IDH-mutant, 1p/19q-intact lower-grade glioma: a validation study. Neuro Oncol 2018;20:1393-9. [Crossref] [PubMed]
- Wang J, Zheng X, Zhang J, Xue H, Wang L, Jing R, Chen S, Che F, Heng X, Li G, Xue F. An MRI-based radiomics signature as a pretreatment noninvasive predictor of overall survival and chemotherapeutic benefits in lower-grade gliomas. Eur Radiol 2021;31:1785-94. [Crossref] [PubMed]
- Tay KL, Tsui A, Phal PM, Drummond KJ, Tress BM. MR imaging characteristics of protoplasmic astrocytomas. Neuroradiology 2011;53:405-11. [Crossref] [PubMed]
- Alghamri MS, Thalla R, Avvari RP, Dabaja A, Taher A, Zhao L, Ulintz PJ, Castro MG, Lowenstein PR. Tumor mutational burden predicts survival in patients with low-grade gliomas expressing mutated IDH1. Neurooncol Adv 2020;2:vdaa042.
- Samstein RM, Lee CH, Shoushtari AN, Hellmann MD, Shen R, Janjigian YY, et al. Tumor mutational load predicts survival after immunotherapy across multiple cancer types. Nat Genet 2019;51:202-6. [Crossref] [PubMed]
- Hwang WL, Wolfson RL, Niemierko A, Marcus KJ, DuBois SG, Haas-Kogan D. Clinical Impact of Tumor Mutational Burden in Neuroblastoma. J Natl Cancer Inst 2019;111:695-9. [Crossref] [PubMed]
- Saini A, Breen I, Pershad Y, Naidu S, Knuttinen MG, Alzubaidi S, Sheth R, Albadawi H, Kuo M, Oklu R. Radiogenomics and Radiomics in Liver Cancers. Diagnostics (Basel) 2018;9:4. [Crossref] [PubMed]
- Story MD, Durante M. Radiogenomics. Med Phys 2018;45:e1111-22. [Crossref] [PubMed]
- Zhao J, Ma W, Zhao H. Loss of heterozygosity 1p/19q and survival in glioma: a meta-analysis. Neuro Oncol 2014;16:103-12. [Crossref] [PubMed]
- Xia L, Wu B, Fu Z, Feng F, Qiao E, Li Q, Sun C, Ge M. Prognostic role of IDH mutations in gliomas: a meta-analysis of 55 observational studies. Oncotarget 2015;6:17354-65. [Crossref] [PubMed]
- Zanfardino M, Pane K, Mirabelli P, Salvatore M, Franzese M. TCGA-TCIA Impact on Radiogenomics Cancer Research: A Systematic Review. Int J Mol Sci 2019;20:6033. [Crossref] [PubMed]