Identifying 18F-FDG PET-metabolic radiomic signature for lung adenocarcinoma prognosis via the leveraging of prognostic transcriptomic module
Introduction
Lung adenocarcinoma (LUAD) has a high mortality rate and is the most common type of lung cancer (1). Presently, surgical resection is the standard treatment for patients with stage I–IIIA LUAD. However, approximately 35–50% of patients who receive this treatment show recurrence or die within 5 years after surgery (2). This highlights the importance of identifying high-risk patients who require postoperative adjuvant treatment, while sparing low-risk patients its toxicity.
Imaging with 18F-fluorodeoxyglucose positron emission tomography (18F-FDG PET) can reflect metabolic differences between healthy tissue and tumor tissue and plays an important role in high accuracy staging and prognosis of LUAD (3-7). Conventional metabolic features of PET, such as maximum standardized uptake value (SUVmax), have been reported to be valuable prognostic factors for LUAD patients (8,9). However, the prognostic performance of these metabolic features still needs to be improved (10). More sophisticated tools based on existing imaging metrics need to be developed for the purpose of precision medicine.
Radiomics, a high-throughput method that converts medical images into quantitative features, is a newly prominent field that could be the vanguard of precision medicine (11-14). Given that medical images can provide information on the underlying pathophysiology that is associated with a patient’s prognosis (15,16), considerable efforts have been made to identify prognostic signatures based on radiomic features in patients with cancer (17-23). At present, most radiomic signatures are constructed based on the tumor phenotypic radiomic features derived from computed tomography (CT) or PET/CT; however, recent studies have demonstrated that the values of these phenotypic features are sensitive to imaging protocols (24) and the platforms used to calculate them (25), which can result in different values among multicenter datasets. Several studies have adopted data normalization to reduce the impact of multicenter effects (26); however, these signatures tend not to be universal in clinical settings because their application requires precollection of samples for normalization. Moreover, sample risk classification is influenced by the risk composition of other samples adopted for normalization (27). Radiomic signatures based on quantitative phenotypic features are therefore not suitable for clinical translation.
Another approach involves reconstructing radiomic features (e.g., SUVmax) derived from PET/CT using CT for attenuation correction and normalizing based on the patient’s body weight. These kinds of features, reflecting metabolic abnormalities, may be more robust against multicenter effects (28). For example, the recommended cutoff value for SUVmax is typically 2.5 for diagnosis worldwide (29), suggesting the repeatability and reproducibility of metabolic features. By using the metabolic features of PET/CT to develop prognostic radiomic signatures for cancer, it may therefore be possible to circumvent the impact of multicenter effects. However, limited by small-scale samples of public PET image datasets, a single-center retrospective study could introduce selection bias and overfitting risk. Motivated by the phenomenon that metabolic radiomic features of PET can indicate molecular abnormalities (such as messenger RNA [mRNA] or protein dysregulation) within the tumor cells (5), we hypothesized the existence of an association between particular radiomic features and specific clinical outcomes.
In this study, we used the gene expression profile of LUAD patients to identify a robust prognostic coexpression module. We then leveraged this module to extract a radiomic signature from metabolic features of 18F-FDG PET images to predict the death and recurrent risk of patients with LUAD and validated the signature in an independent PET dataset. Finally, we assessed the incremental value of the radiomic signature relative to clinical factors for estimating patient death and recurrent risk.
Methods
Study population
In this study, 4 gene expression datasets and 2 18F-FDG PET image datasets were sourced from public databases. The details of multicenter data as well as the clinical information of the patients are presented in Table 1. The inclusion criteria for the samples were as follows: (I) histologically confirmed LUAD, (II) TNM stage confirmed as stage I–IIIA, (III) survival information and complete clinical information available, (IV) treated with curative resection alone, and (V) each gene expression profile dataset having more than 100 samples. The study was conducted in accordance with the Declaration of Helsinki (as revised in 2013). These details and applications of the analyzed datasets are displayed in Figure S1.
Table 1
Variables | GSE68465 | GSE31210 | GSE50081 | TCGA-LUAD | NR |
---|---|---|---|---|---|
Sample | 334 | 204 | 127 | 15 | 72 |
Age | |||||
≤60 years | 107 | 99 | 19 | 5 | 8 |
>60 years | 227 | 105 | 108 | 10 | 64 |
Sex | |||||
Female | 167 | 109 | 62 | 10 | 19 |
Male | 167 | 95 | 65 | 5 | 53 |
TNM stage | |||||
I | 230 | 162 | 92 | 6 | 57 |
II | 65 | 42 | 35 | 1 | 10 |
III | 39 | 0 | 0 | 6 | 5 |
Grade | |||||
Low | 50 | – | – | – | 24 |
Medium | 157 | – | – | – | 36 |
High | 123 | – | – | – | 12 |
Smoking status | |||||
Non-smoker | 33 | 105 | 23 | – | 14 |
Smoker | 217 | 99 | 92 | – | 58 |
Lymphovascular invasion | |||||
Absent | 249 | – | – | – | 66 |
Present | 83 | – | – | – | 6 |
Pleural invasion | |||||
No | – | – | – | – | 55 |
Yes | – | – | – | – | 17 |
Average OS (months) | 47.54 | 60.47 | 52.44 | 35.57 | 42.79 |
Average RFS (months) | – | 55.85 | 43.30 | 15.90 | 38.20 |
NR, non-small cell lung cancer (NSCLC)–radiogenomics dataset; OS, overall survival; RFS, recurrence-free survival; TCGA-LUAD, The Cancer Genome Atlas–lung adenocarcinoma dataset.
The 4 public gene expression datasets of LUAD tissues used in this study were downloaded from Gene Expression Omnibus (GEO; http://www.ncbi.nlm.nih.gov/geo/) and the Cancer Genome Atlas (TCGA; https://portal.gdc.cancer.gov/). The largest GSE68465 (30) dataset consisting of 334 patients with overall survival (OS) information was used to identify gene coexpression modules. Two independent datasets [GSE31210 (31), GSE50081 (32)] with OS and recurrence-free survival (RFS) information were used to test the robustness and prognostic performance of the coexpression modules. For the microarray datasets generated by Affymetrix platforms, the robust multi-array average algorithm (33) was used for preprocessing of the raw data. For TCGA data derived from Illumina HiSeq 2000 RNA Sequencing Version 2 (Illumina, San Diego, CA, USA), the normalized count values determined by the fragments per kilobase of exon per million fragments mapped (FPKM) method were obtained and log2-transformed to yield the gene expression values. Probe IDs were mapped to gene IDs according to the corresponding platform files. Ensembl (ENSG) gene IDs or gene symbols were mapped to the Entrez gene IDs. The 11,804 genes commonly measured by the 3 platforms, Affymetrix U133 Plus 2.0, Affymetrix U133A and IlluminaHiSeq_RNASeqV2, were used for analysis.
18F-FDG PET digital imaging and communications in medicine (DICOM) image data of TCGA-LUAD (n=15) and non-small cell lung cancer (NSCLC)–radiogenomics (NR; n=72) datasets (34) were downloaded from the Cancer Imaging Archive (TCIA; https://www.cancerimagingarchive.net/, 2020). Both TCGA-LUAD and NR datasets contained OS and RFS information, and the TCGA-LUAD dataset was used as a discovery dataset to develop a prognostic radiomic signature. The NR dataset was then used as an independent validation dataset.
Acquisition, processing, segmentation, and quantitative feature extraction of 18F-FDG PET images
18F-FDG PET image acquisition included routine coverage of base of skull to midthigh with additional spot views where necessary. PET images were reconstructed using CT for attenuation correction with iterative ordered subset expectation maximization reconstruction. The standardized uptake value (SUV) of each patient was normalized based on the patient’s body weight. Table S1 summarizes the PET/CT parameters (dose and uptake time) used to obtain PET images.
PET image processing, segmentation, and quantitative feature extraction were performed within 3D Slicer (Version 4.10.2; https://download.slicer.org/). First, a DICOM image was loaded, and the SUV was normalized with the PET DICOM extension based on the patient’s body weight from the DICOM image header. Next, the interest regions of the image were delineated and modified by 2 experienced radiologists using the semiautomated segmentation approach described by Beichel et al. (35). The final feature set included 5 conventional and 17 new metabolic radiomic features, which were calculated using the PET-IndiC extension (https://www.slicer.org/wiki/Documentation/Nightly/Extensions/PET-IndiC). The 17 new features were designed to characterize the fluorodeoxyglucose uptake pattern within the segmented lesion using descriptive statistics. The detailed descriptions and analytical definitions of these features are represented in Table S2.
Weighted gene coexpression network analysis
Statistical analyses in this study were performed using R software 3.5.3 (http://www.r-project.org/). Weighted gene coexpression network analysis (WGCNA) (36) was performed to identify distinct coexpression modules of prognostic-related genes in the GSE68465 dataset using the “WGCNA” R package (details in Supplementary Methods). The first principal component of each module was picked as the module eigengene, which represents the expression level of the module. For each module, the median expression value of all samples in the GSE68465 dataset was set as the cutoff point to distinguish the high and low expression groups. The homogeneity of each module was calculated by averaging all pairwise Pearson correlation coefficients of genes within the module (37).
Construction of a PET-metabolic radiomic signature
Using the matched radiomic and transcriptomic data of 15 patients in the discovery dataset (TCGA-LUAD), we first calculated the expression level of the prognostic coexpression module. PET-metabolic radiomic features with values that were significantly associated with the expression of the prognostic coexpression module were identified as candidate metabolic features. Based on the candidate metabolic features, we applied multiple linear regression analysis to obtain an optimal set that was most correlated with the expression of the prognostic coexpression module. The set was selected as the PET-metabolic radiomic signature for LUAD prognosis. The median value of regression scores of the signature in the discovery dataset was used as the cutoff value for dividing patients into high-risk (≥ median) and low-risk (< median) groups.
Statistical analyses
OS was defined as the time from the date of initial surgical resection to the date of death or last contact (censored). RFS was defined as the time from the date of initial surgical resection to the first occurrence of disease progression or death from any cause. To avoid deviations in the patient follow-up time among the different datasets, patients’ OS and RFS were truncated at 60 months. Survival curves were estimated using the Kaplan-Meier method and were statistically compared using the log-rank test (38). Multivariate Cox regression analysis was performed to test the independent prognostic value of the signature after the prognosis-associated clinical factors tested were adjusted by univariate Cox regression analysis. Hazard ratio (HR) and 95% CIs were generated using Cox proportional hazards models, and the concordance index (C-index) (39) was also used to estimate the predictive performance of a patient’s survival-influencing factors.
To assess the complementary effect of the PET-metabolic radiomic signature on the clinical model in predicting patient prognosis, a radiomic nomogram was constructed using multivariable linear regression analysis (“rms” R package). Additionally, the performance of the radiomic nomogram was evaluated using the C-index, calibration curve, and decision curve analysis. The net reclassification improvement (NRI) index was then determined to quantify the radiomic signature’s incremental prognosis improvement using the “nricens” R package.
The “clusterProfiler” R package was used to conduct the functional enrichment analysis of genes correlated with the radiomic features based on the current Kyoto Encyclopedia of Genes and Genomes (KEGG) database, in which a hypergeometric test was employed. Spearman’s rank correlation analysis was use to estimate correlations between 2 factors. Wilcoxon rank sum test was used to examine the difference between different groups.
Herein, P values were adjusted using the Benjamini-Hochberg procedure (40) for multiple testing to control the false discovery rate (FDR). A 2-sided P value <0.05 or FDR <0.05 was considered indicative of statistical significance.
Results
Identification of a prognostic coexpression module for LUAD
Figure 1 presents a flowchart of this study. First, we extracted 647 risk-related genes whose overexpression were significantly associated with shorter OS of patients (univariate Cox regression, FDR <0.05 and HR >1; Figure 2A) and then identified 3 coexpression modules of the risk-related genes using WGCNA (blue, turquoise, and gray in Figure 2B,2C). Univariate Cox analysis showed that the expressions of the 3 coexpression modules were significantly associated with a shorter OS in patients (P<0.05 and HR >1; Table 2) in the GSE68465 dataset. Furthermore, for each of the 3 modules, the patients in the high expression group (≥ the median value) had significantly shorter OS than did the patients in the low expression group (< the median value) (Figure 2D-2F).
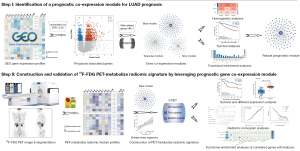
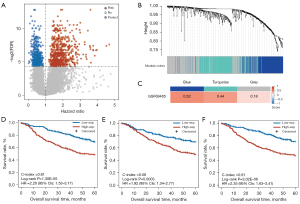
Table 2
Variables | Overall survival | Recurrence-free survival | |||
---|---|---|---|---|---|
HR (95% CI) | P value | HR (95% CI) | P value | ||
GSE68465 | |||||
Blue | 1774.82 (90.00–34,999) | 8.75E-07 | – | – | |
Turquoise | 6,754.30 (200.9–227,041) | 8.79E-07 | – | – | |
Gray | 196,687.70 (6,470–5,979,021) | 2.61E-12 | – | – | |
GSE31210 | |||||
Blue | 1,685 (24.82–114,404) | 0.0006 | 1,758 (93–32,917) | 5.79E-07 | |
Turquoise | 1,721.51 (19.20–154,374) | 0.0012 | 2,304 (99.89–53,133) | 1.33e-06 | |
Gray | 17,142.30 (178.3–1,648,098) | 2.85E-05 | 10,986 (439.9–274,371) | 1.45e-08 | |
GSE50081 | |||||
Blue | 60.96 (3.06–1213) | 0.0071 | 12.76 (0.44–368) | 0.1380 | |
Turquoise | 0.61 (0.02–18.38) | 0.7760 | 0.06 (0.01–2.01) | 0.1150 | |
Gray | 24.51 (0.87–690.9) | 0.0604 | 5.78 (0.14–235.1) | 0.3540 |
HR, hazard ratio.
Next, we tested the homogeneity of the 3 coexpression modules in the 2 validation datasets (GSE31210 and GSE50081). The results (Figure 3A) showed that the blue module, which included 186 genes, had the highest homogeneity scores in the 2 validation sets (GSE31210 score =0.63; GSE50081 score =0.50) when compared with the homogeneity scores in the turquoise module (GSE31210 score =0.21; GSE50081 score =0.40) and the gray module (GSE31210 score =0.24; GSE50081 score =0.18). Furthermore, univariate Cox analysis (Table 2) showed that only the expression of the blue module was significantly associated with OS of patients in the 2 validation datasets (GSE31210: P=0.0006; GSE50081: P=0.0071). Similarly, the expression of the blue module was significantly associated with the RFS of patients in the GSE31210 dataset (P=5.79E-07; HR =1758, 95% CI: 93–32,917) and a similar tendency was observed in the GSE50081 dataset but without statistical significance (P=0.1380; HR =12.76, 95% CI: 0.44–368). Multivariate Cox analysis revealed that the OS was independently predicted by the expression of the blue module after the prognosis-associated clinical factors were adjusted for in the 2 validation cohorts (GSE31210: P=0.0288; GSE50081: P=0.0102; Table S3). The blue module remained significantly associated with the RFS of patients in the multivariate Cox analysis in the GSE31210 dataset (P=3.62E-05; Table S3), and a similar tendency was observed in the GSE50081 dataset but without statistical significance (P=0.2028; Table S3).
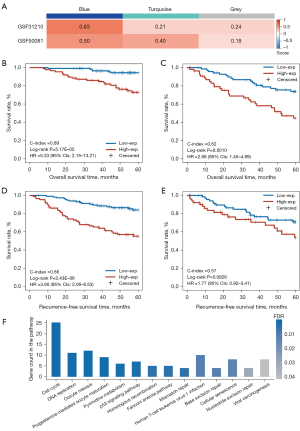
Thereafter, according to the cutoff value (−0.0044) of the blue module defined in the GSE68465 dataset, the patients were divided into high- and low-expression groups with significantly different OS in both the GSE31210 dataset (log-rank P=5.17E-05; HR =5.33, 95% CI: 2.15–13.21; C-index =0.69; Figure 3B) and the GSE50081 dataset (log-rank P=0.0010; HR =2.66, 95% CI: 1.45–4.89; C-index =0.62; Figure 3C). Similar results were observed for the RFS analysis (Table S3); that is, the expression of the blue module was significantly and marginally significantly associated with RFS of patients in the GSE31210 dataset (log-rank P=2.43E-06; HR =3.66, 95% CI: 2.06–6.53; C-index =0.66; Figure 3D) and the GSE50081 dataset (log-rank P=0.0826; HR =1.77, 95% CI: 0.92–3.41; C-index =0.57; Figure 3E), respectively.
These results indicated that the blue module is a robust prognostic factor for LUAD patients. In addition, the functional enrichment analysis showed that the genes of the blue module were significantly enriched in 14 KEGG functional terms (hypergeometric test, FDR <0.05; Figure 3F), including several functions related to LUAD prognosis such as cell cycle, DNA replication, p53 signaling pathway, and “mismatch repair. The results provided biological evidence in support of the ability of the blue module to predict LUAD prognosis.
Construction and validation of 18F-FDG PET-metabolic radiomic signature via the leveraging of the prognostic gene coexpression module
Using the gene expression profile collected in parallel with PET image data in the discovery dataset (TCGA-LUAD) with 15 LUAD patients, we first validated the homogeneity score of the blue module and found that it maintained a high level of homogeneity (score =0.63; Figure 4A) and was significantly higher than the 1000 random scores of the bootstrap approach (P=0.0010). The result indicated the robustness of transcriptomic characteristics within tumor cells in small-scale samples. Additionally, we estimated the homogeneity score of the turquoise (score =0.33; Figure 4A) and gray (score =0.19; Figure 4A) modules, which were not significantly higher than random (P>0.05). We then calculated the expression of the blue module for each sample and extracted 7 candidate metabolic radiomic features whose values were significantly associated with the expression of the blue module (Spearman’s rank correlation, FDR <0.05). Furthermore, we performed multiple linear regression analysis for the candidate features to generate a PET-metabolic radiomic signature (denoted as 3-PET: third quartile, standard deviation, and median; Figure S2) consisting of the 3 features which had the highest correlation with the expression of the blue module (adjusted R2 =0.48; Figure 4B, Supplementary Methods). Using the median (0.0082) as the cutoff value, we divided the patients in the TCGA-LUAD discovery dataset into high- and low-risk patient groups, which yielded significant differences in OS (log-rank P=0.0428; HR =4.64, 95% CI: 0.92–23.51; C-index =0.73; Figure 4C) and RFS (log-rank P=0.0004; HR =18.98, 95% CI: 2.26–159.30; C-index =0.80; Figure 4D).
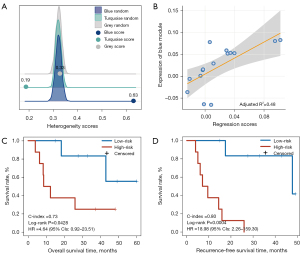
Subsequently, the prognostic performance of 3-PET was validated in an independent PET imaging dataset (the NR dataset) consisting of 72 stage I–IIIA LUAD patients treated with curative resection alone. According to the trained cutoff (0.0082) of 3-PET, 19 patients were classified into the high-risk group and had significantly shorter OS (log-rank P=0.0006; HR =3.64, 95% CI: 1.66–7.98; C-index =0.64; Figure 5A) and RFS (log-rank P=0.0013; HR =4.96, 95% CI: 1.69–14.57; C-index =0.66; Figure 5B) than did the 53 patients classified into the low-risk group. In the multivariate Cox model, 3-PET remained significantly associated with patients’ OS (P=0.0365; HR =2.62, 95% CI: 1.06–6.45; Figure 5C) and RFS (P=0.0175; HR =4.02, 95% CI: 1.28–12.66; Figure 5D) after the prognosis-associated clinical factors (TNM stage or lymphovascular invasion) were adjusted for estimated in the univariate Cox model (Table S4).
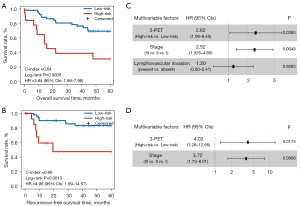
Incremental value and biological interpretation of 3-PET
The multivariate Cox analysis revealed that 3-PET and tumor TNM stage could be used to independently predict OS and RFS in the NR dataset. Consequently, 2 radiomic nomograms for OS and RFS that incorporated a clinical risk factor (TNM stage) and 3-PET were generated using the NR dataset (Figure 6A,6B). The discrimination performance of the radiomic nomograms exhibited significantly higher C-indices relative to those of the clinical nomograms (Figure S3A,S3B) and 3-PET (OS: C-index =0.67; RFS: C-index =0.72; Table 3) based on the NRI indices for OS and RFS (Figure S3C,S3D), respectively. The calibration curves corresponding to the radiomic nomogram at 1-, 3-, and/or 5-year OS and RFS showed good agreement between the estimations and the clinical outcomes in the NR dataset (Figure 6C,6D). Furthermore, the decision-curve analysis showed that the radiomic nomogram exhibited superior performance compared with the clinical nomogram across the majority of the range of reasonable threshold probabilities in the NR dataset (Figure 6E,6F).
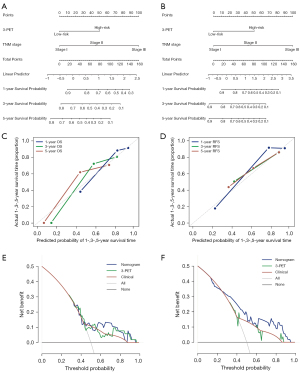
Table 3
Parameters | C-index (95% CI) | |
---|---|---|
OS | RFS | |
Radiomic nomogram | 0.67 (0.57–0.77) | 0.72 (0.58–0.87) |
3-PET | 0.64 (0.55–0.73) | 0.66 (0.54–0.79) |
Clinical nomogram | 0.62 (0.54–0.72) | 0.67 (0.54–0.80) |
OS, overall survival; RFS, recurrence-free survival.
To clarify the underlying biological processes associated with 3-PET, we further analyzed the gene expression profiles collected along with PET image data corresponding to the TCGA-LUAD dataset. First, we identified 1,833, 1,822, and 1,782 genes significantly correlated with the values of the standard deviation, median, and third quartile features (Spearman’s rank correlation, P<0.05), respectively. We found these genes were significantly enriched in several functional pathways related to LUAD progress (hypergeometric test, FDR <0.05; Figure 7A), including cell cycle, p53 signaling pathway, and DNA replication. Then, we analyzed the correlation of 3-PET with the conventional PET prognostic index (SUVmax) and found that the high-risk patients stratified by 3-PET had significantly higher values of SUVmax than did the low-risk patients (Wilcoxon rank sum, P=5.30E-10; Figure 7B), which indicated the good prognostic ability of 3-PET.
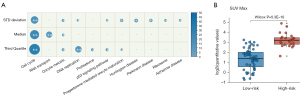
Discussion
LUAD is a clinically heterogeneous disease with large variations in clinical outcomes even among patients with the same TNM stage (41). It is therefore necessary to develop a novel signature to improve the prediction of death or recurrence risk in patients with LUAD. Radiomics is an emerging technique that converts conventional medical images into high dimensional features, providing valuable data to develop noninvasive signatures for cancer prognosis (12,42,43). Compared with tumor phenotypic radiomic features derived from CT or PET/CT, which are sensitive to the multicenter effect (28), metabolic features are more repeatable and reproducible across multicenter cohorts and are more suitable for clinical application. In this study we have therefore developed a noninvasive radiomic signature based on PET-metabolic features with the aid of a transcriptomic module, which could be individually applied in a clinical setting to identify patients with LUAD who have a high risk of death and recurrence.
Thus far, the small-scale of 18F-FDG PET datasets (TCGA, n=15; NR, n=78) has limited the development of a robust PET-metabolic radiomic signature for LUAD prognosis. Additionally, the survival information (OS and RFS) of patients in TCGA dataset cannot not fully reflect the patients’ malignancy degree because they receive different therapeutic strategies after surgery (44,45). Therefore, we hypothesized that using robust molecular information (such as gene expression patterns) related to the clinical outcomes of interest would be an effective method in identifying suitable radiomic features. In this study, we first identified 3 coexpression modules (blue, turquoise, and gray) in a gene expression profile consisting of 334 patients with stage I–IIIA LUAD (GSE68465) and found that the blue module had the highest homogeneity scores in 2 independent expression datasets (GSE31210 and GSE50081). Moreover, the blue module demonstrated better performance in predicting OS and RFS in the 2 independent datasets. The functional enrichment analyses showed that the genes of the blue module were significantly enriched in pathways related to the prognosis of LUAD, such as cell cycle, DNA replication, and p53 signaling pathway. The results showed that the blue module is a robust prognostic factor for LUAD patients and is a worthwhile intermediary in the development of a noninvasive radiomic signature for clinical application.
To confirm our hypothesis that robust molecular information (gene expression patterns) can be used to identify radiomic features related to specific clinical outcomes, we first validated the homogeneity score of the blue module (i.e., the prognostic coexpression module). We found that the homogeneity score was significantly higher than random in the TCGA-LUAD dataset with only 15 samples, supporting the robustness of transcriptomic characteristics within tumor cells in small-scale samples. In the TCGA-LUAD dataset, we further identified 7 PET-metabolic radiomic features whose levels were significantly associated with the expression of the blue module (Spearman’s rank correlation, FDR <0.05). On the other hand, there were no features whose levels were significantly associated with patients’ OS or RFS in the TCGA-LUAD dataset (univariate Cox analysis, P>0.05). Thereafter, by leveraging the expression of the blue module, we developed a PET-metabolic radiomic signature (3-PET) for LUAD prognosis in the TCGA-LUAD discovery dataset. 3-PET could be directly applied to an individual patient to determine the patient’s death or recurrence risk based on the trained cutoff value without any cohort normalization. The prognostic performance of 3-PET was effectively validated in an independent dataset (NR dataset) in which both the 5-year survival and recurrence rate of the high-risk patients predicted by 3-PET was significantly lower than that predicted for the low-risk patients (5-year survival: high-risk vs. low-risk = 0.31 vs. 0.69; 5-year recurrence: high-risk vs. low-risk = 0.47 vs. 0.83). These results indicate good the prognostic performance and robustness of 3-PET in identifying LUAD patients with poor prognosis. Additionally, we compared 3-PET with a conventional metabolic prognostic index (SUVmax) and a published PET phenotypic-based signature (Ahn’s model) (42) in the NR dataset. Although 3-PET showed significant association with SUVmax, the survival analyses in the NR dataset demonstrated the superior prognostic performance of 3-PET (OS: Cox P=0.0012, C-index =0.64; RFS: Cox P=0.0036, C-index =0.66) as compared to SUVmax with grouping by risk cutoff (2.5), with the latter being only potentially associated with OS and RFS of patients (OS: Cox P=0.1500, C-index =0.58; RFS: Cox P=0.0740, C-index =0.63). Similar results were observed for the other risk cutoff value (5.9) for SUVmax (OS: Cox P=0.0023, C-index = 0.63; RFS: Cox P=0.1030, C-index =0.60), which has also been used in several studies (46). Next, for Ahn’s model, the risk scores of LUAD patients were calculated based on a single PET phenotypic-based feature (neighborhood-gray-level-difference-matrix contrast) in the model. The risk scores were not significantly associated with patients’ OS and RFS in the NR dataset (OS: Cox P=0.1036, C-index =0.59; RFS: Cox P=0.3939, C-index =0.58). More importantly, according to the risk cutoff (0.001) all patients were classified as high risk; this suggests that the risk cutoff of a PET/CT phenotypic-based radiomic signature derived from its discovery cohort cannot be directly applied to independent cohorts due to multicenter effects.
Furthermore, combining the clinical TNM stage with 3-PET in a radiomic nomogram significantly improved the prognostic performance of the clinical staging system in the NR dataset. Our results indicated that 3-PET could provide additional prognostic information for patients within the same clinical stage. Therefore, it is worthwhile to develop 3-PET as a noninvasive individual prognostic tool for clinical application. Here, we tentatively performed subgroup analyses and showed that 3-PET also could predict the prognosis of patients with T1 stage LUAD (Figure S4). A similar trend was observed in patients with N0 stage LUAD; that is, the high-risk patients had shorter OS and RFS than did the low-risk patients, but not significantly so. This needs further large-scale validation with multicenter clinical trials.
A radiomic signature that is accurately linked to the underlying biological progression of a disease is favorable for clinical application. Therefore, we also identified the genes corresponding to each feature within 3-PET and found that these correlated genes were also significantly enriched in cell cycle, p53 signaling pathway, and DNA replication functional pathways (Table S5). For example, all 3 features showed strongly positive correlations with genes involved in the cell cycle pathway, including cyclin B1 (CCNB1), cyclin B2 (CCNB2), and cyclin E1 (CCNE1), which have been reported to promote the occurrence and development of LUAD (47,48). These results indicate that 3-PET could reflect the underlying biological characteristics within tumor cells that are strongly associated with clinical outcomes.
Conclusions
In conclusion, the PET-metabolic radiomic signature was able to identify LUAD patients with high risk of death and recurrence who required further adjuvant therapy after surgery, thereby improving prognosis for high risk patients and sparing low risk patients complications associated with adjuvant therapy. This noninvasive approach can provide a more precise diagnosis from imaging studies and may serve as a tool to guide the personalized treatment of patients.
Acknowledgments
The authors thank Professor Zheng Guo (College of Bioinformatics Science and Technology, Harbin Medical University, Harbin, China) for providing significant insights.
Funding: This work was supported by the National Natural Science Foundation of China (61773134, 61803117, and 81872396) and the National Undergraduate Innovation and Entrepreneurship Training Program (202110226018).
Footnote
Conflicts of Interest: All authors have completed the ICMJE uniform disclosure form (available at https://dx.doi.org/10.21037/qims-21-706). The authors have no conflicts of interest to declare.
Ethical Statement: The authors are accountable for all aspects of the work in ensuring that questions related to the accuracy or integrity of any part of the work are appropriately investigated and resolved. The study was conducted in accordance with the Declaration of Helsinki (as revised in 2013). No ethical approval or written informed consent was required for this retrospective study.
Open Access Statement: This is an Open Access article distributed in accordance with the Creative Commons Attribution-NonCommercial-NoDerivs 4.0 International License (CC BY-NC-ND 4.0), which permits the non-commercial replication and distribution of the article with the strict proviso that no changes or edits are made and the original work is properly cited (including links to both the formal publication through the relevant DOI and the license). See: https://creativecommons.org/licenses/by-nc-nd/4.0/.
References
- Siegel RL, Miller KD, Fuchs HE, Jemal A. Cancer Statistics, 2021. CA Cancer J Clin 2021;71:7-33. [Crossref] [PubMed]
- Esposito L, Conti D, Ailavajhala R, Khalil N, Giordano A. Lung Cancer: Are we up to the Challenge? Curr Genomics 2010;11:513-8. [Crossref] [PubMed]
- Chetan MR, Gleeson FV. Radiomics in predicting treatment response in non-small-cell lung cancer: current status, challenges and future perspectives. Eur Radiol 2021;31:1049-58. [Crossref] [PubMed]
- Kim HR, Kim DJ, Lee WW, Jheon S, Sung SW. The significance of maximum standardized uptake values in patients with stage I pulmonary adenocarcinoma. Eur J Cardiothorac Surg 2009;35:712-6; discussion 716-7. [Crossref] [PubMed]
- Na KJ, Choi H. Tumor Metabolic Features Identified by 18F-FDG PET Correlate with Gene Networks of Immune Cell Microenvironment in Head and Neck Cancer. J Nucl Med 2018;59:31-7. [Crossref] [PubMed]
- Dissaux G, Visvikis D, Da-Ano R, Pradier O, Chajon E, Barillot I, Duvergé L, Masson I, Abgral R, Santiago Ribeiro MJ, Devillers A, Pallardy A, Fleury V, Mahé MA, De Crevoisier R, Hatt M, Schick U. Pretreatment 18F-FDG PET/CT Radiomics Predict Local Recurrence in Patients Treated with Stereotactic Body Radiotherapy for Early-Stage Non-Small Cell Lung Cancer: A Multicentric Study. J Nucl Med 2020;61:814-20. [Crossref] [PubMed]
- Silva Y, Riedinger JM, Chrétien ML, Caillot D, Corre J, Guillen K, Cochet A, Tabouret-Viaud C, Loffroy R. Comparison between tumour metabolism derived from 18F-FDG PET/CT and accurate cytogenetic stratification in newly diagnosed multiple myeloma patients. Quant Imaging Med Surg 2021;11:4299-309. [Crossref] [PubMed]
- Inal A, Kucukoner M, Kaplan MA, Urakci Z, Karakus A, Komek H, Dostbil Z, Isikdogan A. Prognostic value of fluorine-18 fluorodeoxyglucose positron emission tomography in patients with advanced non-small cell lung cancer: single center experience. J BUON 2012;17:724-8. [PubMed]
- Mattonen SA, Davidzon GA, Bakr S, Echegaray S, Leung ANC, Vasanawala M, Horng G, Napel S, Nair VS. 18F FDG Positron Emission Tomography (PET) Tumor and Penumbra Imaging Features Predict Recurrence in Non-Small Cell Lung Cancer. Tomography 2019;5:145-53. [Crossref] [PubMed]
- Jiang Y, Yuan Q, Lv W, Xi S, Huang W, Sun Z, Chen H, Zhao L, Liu W, Hu Y, Lu L, Ma J, Li T, Yu J, Wang Q, Li G. Radiomic signature of 18F fluorodeoxyglucose PET/CT for prediction of gastric cancer survival and chemotherapeutic benefits. Theranostics 2018;8:5915-28. [Crossref] [PubMed]
- Lambin P, Rios-Velazquez E, Leijenaar R, Carvalho S, van Stiphout RG, Granton P, Zegers CM, Gillies R, Boellard R, Dekker A, Aerts HJ. Radiomics: extracting more information from medical images using advanced feature analysis. Eur J Cancer 2012;48:441-6. [Crossref] [PubMed]
- Aerts HJ, Velazquez ER, Leijenaar RT, Parmar C, Grossmann P, Carvalho S, Bussink J, Monshouwer R, Haibe-Kains B, Rietveld D, Hoebers F, Rietbergen MM, Leemans CR, Dekker A, Quackenbush J, Gillies RJ, Lambin P. Decoding tumour phenotype by noninvasive imaging using a quantitative radiomics approach. Nat Commun 2014;5:4006. [Crossref] [PubMed]
- Chen B, Yang L, Zhang R, Luo W, Li W. Radiomics: an overview in lung cancer management-a narrative review. Ann Transl Med 2020;8:1191. [Crossref] [PubMed]
- Li J, Zhou Y, Wang P, Zhao H, Wang X, Tang N, Luan K. Deep transfer learning based on magnetic resonance imaging can improve the diagnosis of lymph node metastasis in patients with rectal cancer. Quant Imaging Med Surg 2021;11:2477-85. [Crossref] [PubMed]
- Lambin P, Leijenaar RTH, Deist TM, Peerlings J, de Jong EEC, van Timmeren J, Sanduleanu S, Larue RTHM, Even AJG, Jochems A, van Wijk Y, Woodruff H, van Soest J, Lustberg T, Roelofs E, van Elmpt W, Dekker A, Mottaghy FM, Wildberger JE, Walsh S. Radiomics: the bridge between medical imaging and personalized medicine. Nat Rev Clin Oncol 2017;14:749-62. [Crossref] [PubMed]
- Liu Z, Wang S, Dong D, Wei J, Fang C, Zhou X, Sun K, Li L, Li B, Wang M, Tian J. The Applications of Radiomics in Precision Diagnosis and Treatment of Oncology: Opportunities and Challenges. Theranostics 2019;9:1303-22. [Crossref] [PubMed]
- Liu Z, Meng X, Zhang H, Li Z, Liu J, Sun K, Meng Y, Dai W, Xie P, Ding Y, Wang M, Cai G, Tian J. Predicting distant metastasis and chemotherapy benefit in locally advanced rectal cancer. Nat Commun 2020;11:4308. [Crossref] [PubMed]
- Lu H, Arshad M, Thornton A, Avesani G, Cunnea P, Curry E, Kanavati F, Liang J, Nixon K, Williams ST, Hassan MA, Bowtell DDL, Gabra H, Fotopoulou C, Rockall A, Aboagye EO. A mathematical-descriptor of tumor-mesoscopic-structure from computed-tomography images annotates prognostic- and molecular-phenotypes of epithelial ovarian cancer. Nat Commun 2019;10:764. [Crossref] [PubMed]
- Trebeschi S, Drago SG, Birkbak NJ, Kurilova I, Cǎlin AM, Delli Pizzi A, Lalezari F, Lambregts DMJ, Rohaan MW, Parmar C, Rozeman EA, Hartemink KJ, Swanton C, Haanen JBAG, Blank CU, Smit EF, Beets-Tan RGH, Aerts HJWL. Predicting response to cancer immunotherapy using noninvasive radiomic biomarkers. Ann Oncol 2019;30:998-1004. [Crossref] [PubMed]
- Vaidya P, Bera K, Gupta A, Wang X, Corredor G, Fu P, Beig N, Prasanna P, Patil P, Velu P, Rajiah P, Gilkeson R, Feldman M, Choi H, Velcheti V, Madabhushi A. CT derived radiomic score for predicting the added benefit of adjuvant chemotherapy following surgery in Stage I, II resectable Non-Small Cell Lung Cancer: a retrospective multi-cohort study for outcome prediction. Lancet Digit Health 2020;2:e116-28. [Crossref] [PubMed]
- Xie D, Wang TT, Huang SJ, Deng JJ, Ren YJ, Yang Y, Wu JQ, Zhang L, Fei K, Sun XW, She YL, Chen C. Radiomics nomogram for prediction disease-free survival and adjuvant chemotherapy benefits in patients with resected stage I lung adenocarcinoma. Transl Lung Cancer Res 2020;9:1112-23. [Crossref] [PubMed]
- Yang B, Ji H, Zhong J, Ma L, Zhong J, Dong H, Zhou C, Duan S, Zhu C, Tian J, Zhang L, Wang F, Zhu H, Lu G. Value of 18F-FDG PET/CT-Based Radiomics Nomogram to Predict Survival Outcomes and Guide Personalized Targeted Therapy in Lung Adenocarcinoma With EGFR Mutations. Front Oncol 2020;10:567160. [Crossref] [PubMed]
- Du D, Gu J, Chen X, Lv W, Feng Q, Rahmim A, Wu H, Lu L. Integration of PET/CT Radiomics and Semantic Features for Differentiation between Active Pulmonary Tuberculosis and Lung Cancer. Mol Imaging Biol 2021;23:287-98. [Crossref] [PubMed]
- Midya A, Chakraborty J, Gönen M, Do RKG, Simpson AL. Influence of CT acquisition and reconstruction parameters on radiomic feature reproducibility. J Med Imaging (Bellingham) 2018;5:011020. [Crossref] [PubMed]
- Fornacon-Wood I, Mistry H, Ackermann CJ, Blackhall F, McPartlin A, Faivre-Finn C, Price GJ, O'Connor JPB. Reliability and prognostic value of radiomic features are highly dependent on choice of feature extraction platform. Eur Radiol 2020;30:6241-50. [Crossref] [PubMed]
- Shi Z, Zhovannik I, Traverso A, Dankers FJWM, Deist TM, Kalendralis P, Monshouwer R, Bussink J, Fijten R, Aerts HJWL, Dekker A, Wee L. Distributed radiomics as a signature validation study using the Personal Health Train infrastructure. Sci Data 2019;6:218. [Crossref] [PubMed]
- Qi L, Chen L, Li Y, Qin Y, Pan R, Zhao W, Gu Y, Wang H, Wang R, Chen X, Guo Z. Critical limitations of prognostic signatures based on risk scores summarized from gene expression levels: a case study for resected stage I non-small-cell lung cancer. Brief Bioinform 2016;17:233-42. [Crossref] [PubMed]
- Vargas HA, Kramer GM, Scott AM, Weickhardt A, Meier AA, Parada N, et al. Reproducibility and Repeatability of Semiquantitative 18F-Fluorodihydrotestosterone Uptake Metrics in Castration-Resistant Prostate Cancer Metastases: A Prospective Multicenter Study. J Nucl Med 2018;59:1516-23. [Crossref] [PubMed]
- Wu Y, Li P, Zhang H, Shi Y, Wu H, Zhang J, Qian Y, Li C, Yang J. Diagnostic value of fluorine 18 fluorodeoxyglucose positron emission tomography/computed tomography for the detection of metastases in non-small-cell lung cancer patients. Int J Cancer 2013;132:E37-47. [Crossref] [PubMed]
- Shedden K, Taylor JM, Enkemann SA, Tsao MS, Yeatman TJ, et al. Gene expression-based survival prediction in lung adenocarcinoma: a multi-site, blinded validation study. Nat Med 2008;14:822-7. [Crossref] [PubMed]
- Okayama H, Kohno T, Ishii Y, Shimada Y, Shiraishi K, Iwakawa R, et al. Identification of genes upregulated in ALK-positive and EGFR/KRAS/ALK-negative lung adenocarcinomas. Cancer Res 2012;72:100-11. [Crossref] [PubMed]
- Der SD, Sykes J, Pintilie M, Zhu CQ, Strumpf D, Liu N, Jurisica I, Shepherd FA, Tsao MS. Validation of a histology-independent prognostic gene signature for early-stage, non-small-cell lung cancer including stage IA patients. J Thorac Oncol 2014;9:59-64. [Crossref] [PubMed]
- Irizarry RA, Hobbs B, Collin F, Beazer-Barclay YD, Antonellis KJ, Scherf U, Speed TP. Exploration, normalization, and summaries of high density oligonucleotide array probe level data. Biostatistics 2003;4:249-64. [Crossref] [PubMed]
- Bakr S, Gevaert O, Echegaray S, Ayers K, Zhou M, Shafiq M, Zheng H, Benson JA, Zhang W, Leung ANC, Kadoch M, Hoang CD, Shrager J, Quon A, Rubin DL, Plevritis SK, Napel S. A radiogenomic dataset of non-small cell lung cancer. Sci Data 2018;5:180202. [Crossref] [PubMed]
- Beichel RR, Van Tol M, Ulrich EJ, Bauer C, Chang T, Plichta KA, Smith BJ, Sunderland JJ, Graham MM, Sonka M, Buatti JM. Semiautomated segmentation of head and neck cancers in 18F-FDG PET scans: A just-enough-interaction approach. Med Phys 2016;43:2948-64. [Crossref] [PubMed]
- Langfelder P, Horvath S. WGCNA: an R package for weighted correlation network analysis. BMC Bioinformatics 2008;9:559. [Crossref] [PubMed]
- Zhou M, Leung A, Echegaray S, Gentles A, Shrager JB, Jensen KC, Berry GJ, Plevritis SK, Rubin DL, Napel S, Gevaert O. Non-Small Cell Lung Cancer Radiogenomics Map Identifies Relationships between Molecular and Imaging Phenotypes with Prognostic Implications. Radiology 2018;286:307-15. [Crossref] [PubMed]
- Bland JM, Altman DG. The logrank test. BMJ 2004;328:1073. [Crossref] [PubMed]
- Harrell FE Jr, Lee KL, Mark DB. Multivariable prognostic models: issues in developing models, evaluating assumptions and adequacy, and measuring and reducing errors. Stat Med 1996;15:361-87. [Crossref] [PubMed]
- Dong D, Fang MJ, Tang L, Shan XH, Gao JB, Giganti F, Wang RP, Chen X, Wang XX, Palumbo D, Fu J, Li WC, Li J, Zhong LZ, De Cobelli F, Ji JF, Liu ZY, Tian J. Deep learning radiomic nomogram can predict the number of lymph node metastasis in locally advanced gastric cancer: an international multicenter study. Ann Oncol 2020;31:912-20. [Crossref] [PubMed]
- Devarakonda S, Morgensztern D, Govindan R. Genomic alterations in lung adenocarcinoma. Lancet Oncol 2015;16:e342-51. [Crossref] [PubMed]
- Ahn HK, Lee H, Kim SG, Hyun SH. Pre-treatment 18F-FDG PET-based radiomics predict survival in resected non-small cell lung cancer. Clin Radiol 2019;74:467-73. [Crossref] [PubMed]
- Wang L, Li T, Hong J, Zhang M, Ouyang M, Zheng X, Tang K. 18F-FDG PET-based radiomics model for predicting occult lymph node metastasis in clinical N0 solid lung adenocarcinoma. Quant Imaging Med Surg 2021;11:215-25. [Crossref] [PubMed]
- Uramoto H, Tanaka F. Recurrence after surgery in patients with NSCLC. Transl Lung Cancer Res 2014;3:242-9. [PubMed]
- Zhang Z, Zhang S, Li X, Zhao Z, Chen C, Zhang J, Li M, Wei Z, Jiang W, Pan B, Li Y, Liu Y, Cao Y, Zhao W, Gu Y, Yu Y, Meng Q, Qi L. Reference genome and annotation updates lead to contradictory prognostic predictions in gene expression signatures: a case study of resected stage I lung adenocarcinoma. Brief Bioinform 2021;22:bbaa081.
- Liu J, Dong M, Sun X, Li W, Xing L, Yu J. Prognostic Value of 18F-FDG PET/CT in Surgical Non-Small Cell Lung Cancer: A Meta-Analysis. PLoS One 2016;11:e0146195. [Crossref] [PubMed]
- Wang X, Xiao H, Wu D, Zhang D, Zhang Z. miR-335-5p Regulates Cell Cycle and Metastasis in Lung Adenocarcinoma by Targeting CCNB2. Onco Targets Ther 2020;13:6255-63. [Crossref] [PubMed]
- Wang F, Chen X, Yu X, Lin Q. Degradation of CCNB1 mediated by APC11 through UBA52 ubiquitination promotes cell cycle progression and proliferation of non-small cell lung cancer cells. Am J Transl Res 2019;11:7166-85. [PubMed]