Structural similarity analysis of midfacial fractures—a feasibility study
Introduction
Computed tomography (CT) is the routine imaging method of choice for the diagnosis of midfacial fractures in emergency department patients (1). Unfortunately, obtaining a good quality image involves a substantial radiation dose (1,2) However, iterative reconstruction (IR) and deep learning algorithms have been proposed to maintain image quality on reducing the radiation dose (1,3).
The IR algorithm was developed to improve the image quality of reconstructed datasets by producing image data that accurately corresponds to the measured projection data (4). The most recent full model-based IR algorithm involves both backward and forward projection. This complex algorithm uses the difference between an estimation of the raw data and the real measured data from the imaging system to reduce the image noise in successive iterations (5).
Recently, deep learning processing was proposed as a completely new strategy to optimize the image quality of reconstructed CT datasets. The proprietary PixelShine™ (PS) algorithm is a software technology based on a deep, artificial neural network (AlgoMedica Inc., Sunnyvale, California, USA). The artificial neural network is trained at pixel level and learns the relationship between baseline and low dose datasets to determine a function that improves the image quality of the dataset.
In a previous study by our research group, we found that both the advanced modeled IR and the PS algorithm substantially improve the noise related image quality of CT protocols for maxillofacial trauma (3). Although outcomes such as the signal-to-noise and contrast-to-noise ratio quantify image noise, they are not directly related to how the fracture visualization is affected. The same is true for other more complex metrics such as noise power spectrum (NPS), noise equivalent quanta (NEQ), the modulation transfer function (MTF) and detective quantum efficiency (DQE) which are related more to the image formation process of the system (6). Thus, human observer studies were designed to perform a specific task or to quantify the image quality using a confidence rating scale. However, human observer studies are prone to the natural limitations of perception and observer predictions bias. Therefore, the Structural Similarity Index Metric (SSIM) was developed for the assessment of image quality (7,8).
SSIM measures the similarity between two images. It is based on luminance differences, contrast differences and structural variations and ranges from minus one (opposite contrast) to zero (completely different) to one (completely identical) (6). Later, a multi-scale structural similarity approach (MS-SSIM) was proposed for the assessment of radiological images. It simulates different spatial resolutions by iterative downsampling and weighting the different values of each component (9). The aim of our study was to assess the feasibility of this metric to measure the structural image quality and fracture characteristics of midfacial fractures. These measures were assessed with reduced scan radiation dose protocols, and IR and deep learning reconstruction algorithms were used for image quality optimization.
We present the following article in accordance with the MDAR checklist (available at https://dx.doi.org/10.21037/qims-21-564).
Methods
Human cadaver specimen
A selection of four fresh frozen human cadaver specimens were obtained from the anatomy section of the Department of Neurosciences of the University Medical Center Groningen (University of Groningen, Groningen, the Netherlands), in accordance with our institute’s regulations. The study was conducted in accordance with the Declaration of Helsinki (as revised in 2013).
Artificial infliction of fractures
Unilateral zygomaticomaxillary fractures were inflicted on each specimen using an experimental design whereby a free-falling mass was used to simulate a blunt facial trauma. In this experiment, the biomechanical tolerance of the zygomaticomaxillary complex to small and non-dislocated fractures was studied. More details regarding this experimental approach were described previously by our research group (10).
Computer tomography imaging
The specimens were scanned using a third generation Siemens SOMATOM Force Computed Tomography (CT) scanner (Siemens Healthcare AG, Erlangen, Germany). Each specimen was scanned using both a standard maxillofacial trauma protocol (ref mAs 50) and a reduced radiation dose reduced protocol where the reference mAs was reduced to the lowest limit (ref mAs 20). The raw data of both protocols were reconstructed using a model-based IR algorithm with strengths of one, three and five, the latter being the highest strength possible (ADMIRE, Siemens Healthcare AG, Erlangen, Germany) and the Hr59d bone kernel. Subsequently, all the datasets were subjected to the deep learning PS algorithm (v.1.2.57) to improve the image quality further. The datasets were all exported according to the Digital Imaging and Communications in Medicine (DICOM) standard. The acquisition and reconstruction parameters are summarized in Table 1.
Table 1
Parameter | Value |
---|---|
Tube voltage | 80 kV |
Tube current modulation | CARE Dose4D |
Quality reference mAs | 50 & 20 |
ADMIRE strength | 1, 3 & 5 |
Field of view | 220.0 mm |
Collimation | 192×0.6 mm |
Average scan length | 118 mm |
Slice thickness | 0.6 mm |
Position increment | 0.4 mm |
Grayscale depth | 12 bit |
Pitch | 0.6 |
Rotation time | 0.5 s |
Exposure time | 0.5 s |
Scan time | 3.4 s |
Matrix | 512×512 |
Reconstruction kernels | Bone Hr59d |
Post-processing | PixelShineTM deep learning processing (v.1.2.57) |
CT, computed tomography; ADMIRE, Advanced Modelled Iterative Reconstruction.
Nondisplaced fracture selection
The datasets were assessed by an experienced independent European certified head and neck radiologist (BD) to identify fractures suitable for analysis, with particular attention being paid to minimal and non-dislocated fractures. The standard maxillofacial trauma datasets (ref. mAs 50) were assessed on a medical diagnostic display.
Osseous anatomy attenuation
All the datasets were imported into a Python software application for data analysis (Python Software Foundation. Python Language Reference, version 3.6.1. Available on http://www.python.org). A Hounsfield unit (HU) based sigmoidal soft thresholding function was applied so that only the osseous anatomy of all the datasets was the point of focus, using the following equation:
After attenuation, the effects of radiation dose reduction, IR strength and the use of the PS algorithms on fracture visibility were assessed in two experiments (Figure 1).
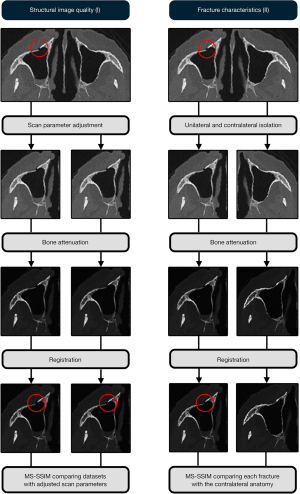
Structural image quality measurements (I)
In the first experiment, the MS-SSIM was used to assess the effects of radiation dose reduction, IR strength and the use of the PS algorithm on structural image quality. An area of interest was isolated, consisting of a 1,283-pixel cube, using the fracture as the centre. The MS-SSIM was calculated to compare the corresponding areas of interest of the varying radiation dose protocols (ref. mAs 50 & 20), AMDIRE strengths (1,3 & 5) and after applying the PS algorithm (no & yes).
Assessment of fracture characteristics (II)
In the second experiment, the MS-SSIM was used to measure the differences in fracture characteristics by comparing each fracture location with its contralateral anatomy. Using the fracture locations as the centre, a spherical area of interest was isolated using an 8-pixel radius. Accordingly, the uninjured side of the midface was computationally mirrored and superimposed on the side where a fracture had been inflicted. As we only wanted to measure the differences imposed by the inflicted fracture, registration was used to undo misalignment due to anatomical asymmetry and imperfect positioning of the specimens within the gantry during data acquisition. First, solid registration aligned the fracture and uninjured side orthogonally, initialized with manual estimations of the registration angles and offsets. Secondly, elastic registration corrected for misalignments due to global mismatching, solid registration and anatomical asymmetry between the fractured and uninjured sides (11). The MS-SSIM was calculated and compared for each combination of datasets where the radiation doses, IR strength and PS algorithm had been adjusted. The experiments are summarized in Figure 1.
Statistical analysis
The data were analysed with the Statistical Package for the Social Sciences (IBM Corp. Released 2015. IBM SPSS Statistics for Windows, Version 23.0. Armonk, NY, USA: IBM Corp.). The MS-SSIM outcomes were presented as means and standard deviations. The normality was tested with Q-Q plots and the Kolmogorov-Smirnov test.
Results
Fractures
Zygomaticomaxillary fractures were successfully inflicted on all four cadavers from which a total of twelve individual fracture locations were selected as eligible for data analysis. The fracture locations included the anterior maxillary sinus (n=6), posterior maxillary sinus (n=3), lateral orbital wall (n=4), and zygomatic arch (n=1).
Structural image quality measurements
The MS-SSIM outcomes are presented in Table 2 and Figure 2. The greatest decrease in structural image quality was found for radiation dose reduction (0.980036±0.011904). Regarding the IR algorithm, a decrease in structural image quality occurred with an increase in strength, whereas the highest effect was found for the 1 to 5 ADMIRE strengths (0.995399±0.001059). The effects of the PS algorithm were minimal because the MS-SSIM outcomes were almost identical to one (0.999996±0.000002).
Table 2
Parameter | Reference | Comparison | MS SSIM (mean ± SD) |
---|---|---|---|
Radiation dose protocol1 | Ref. mAs 50 | Ref. mAs 20 | 0.980036±0.011904 |
ADMIRE strength2 | 1 | 3 | 0.999249±0.000170 |
1 | 5 | 0.995399±0.001059 | |
3 | 5 | 0.998303±0.000401 | |
PS use3 | No | Yes | 0.999996±0.000002 |
1, Only the ref. mAs 50 radiation dose was adjusted to ref. mAs 20 and the ADMIRE was kept at strength 1 and PixelShine was not used. 2, Only the ADMIRE strength was adjusted from 1 to 3 and 5 and the radiation dose was kept at ref. mAs 50 and PixelShine was not used. 3, Only the PixelShine was used and the radiation dose was kept at ref. mAs 50 and the ADMIRE strength was kept at 1. MS-SSIM, multi-scale structural similarity index metric; SD, standard deviation; Ref. mAs, milliampere-seconds reference; ADMIRE, advanced modeled iterative reconstruction; PS, PixelShine.
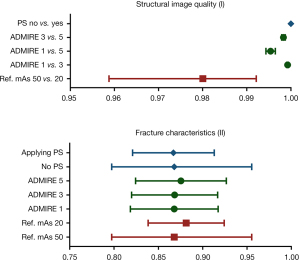
Fracture characteristics analysis
The MS-SSIM outcomes are presented in Table 3 and Figure 2. The MS-SSIM outcomes of all the datasets were below 0.90. Regarding radiation dose reduction and the IR algorithm, the MS-SSIM tended to increase, indicating higher structural similarity when comparing the fractured side to the contralateral non-fractured side. No clear trend was observed after applying the PS algorithm.
Table 3
Radiation dose | ADMIRE strength | PS used | MS SSIM (mean ± SD)1 |
---|---|---|---|
Ref. mAs 50 | 1 | No | 0.878369±0.043958 |
Ref. mAs 20 | 1 | No | 0.881375±0.042911 |
Ref. mAs 50 | 3 | No | 0.874766±0.047197 |
Ref. mAs 20 | 3 | No | 0.884703±0.046482 |
Ref. mAs 50 | 5 | No | 0.879656±0.037421 |
Ref. mAs 20 | 5 | No | 0.880935±0.037421 |
Ref. mAs 50 | 1 | Yes | 0.866955±0.046103 |
Ref. mAs 20 | 1 | Yes | 0.867904±0.049528 |
Ref. mAs 50 | 3 | Yes | 0.876707±0.046604 |
Ref. mAs 20 | 3 | Yes | 0.868314±0.048533 |
Ref. mAs 50 | 5 | Yes | 0.874656±0.048757 |
Ref. mAs 20 | 5 | Yes | 0.875457±0.051219 |
1, the reference category involved the ref. mAs 50 protocol, IR strength 1 and not using the PS algorithm. MS-SSIM, Multi Scale Structural Similarity Index Metric; ADMIRE, Advanced Modeled Iterative Reconstruction; PS, PixelShine; SD, standard deviation; Ref. mAs, Milliampere-seconds Reference.
Discussion
The concept of structural similarity measurements is based on the assumption that the human visual system is highly adapted to extracting structural information from the scene whereas a measure of structural similarity can provide a good approximation of the perceived image quality (9). In this study the MS-SSIM was used to study structural image quality and changes to midfacial fracture characteristics. Scan protocols with reduced radiation doses were assessed and the IR and PS algorithms were used to improve the image quality of the outcome measures related to structural similarity. We found that the structural image quality worsened with radiation dose reduction, while the effects of IR and PS were small. Especially the MS-SSIM of the PS algorithm was observed to be exceptionally close to one, indicating a nearly identical structural image compared to datasets where the PS had not been applied.
This result is in line with the fracture characteristics analysis where no clear trend was observed on applying the PS algorithm (Figure 2). This indicates that the PS algorithm denoises the dataset without affecting the fracture characteristics. Radiation dose reduction and IR strength tend to increase the MS-SSIM, indicating a greater similarity between the fracture and the non-fracture side. Although the effects were small, an MS-SSIM tendency towards ‘one’ indicates a potential smoothing or erasing of the individual fractures. Despite the above results, we found that the MS-SSIM for fracture characteristics’ analysis was lower than 0.90 for all the datasets, which might have been caused by the anatomical variance of the contralateral non-fractured side. Even though the variance was reduced to a minimum with solid and elastic registration, the differences in internal bony architecture could have also led to structural dissimilarities. One could consider unilateral analysis of the fracture side before and after inflicting the fracture. However, repositioning the specimen in the gantry can also lead to dissimilarities due to a variance in orthogonal resolution. The results of this feasibility study can be used for the conceptualization of future research to explore the effects of these scan parameters further on structural image quality and changes to fracture characteristics.
In conclusion, this feasibility human cadaver study of a structural similarity analysis of midfacial fractures provides an assessment method for structural image quality and fracture characteristics using the multi-scale structural similarity index metric. Structural image quality tends to be affected the most by radiation dose reduction, whereas the effects of IR and PS tend to be small. Fracture characteristic analyses was confined due to anatomical variances, but the data indicate that reducing the radiation dose and increasing the IR strength negatively affects the MS-SSIM, while the PS algorithm does not tend to affect this measure.
Acknowledgments
Funding: None.
Footnote
Reporting Checklist: The authors have completed the MDAR reporting checklist. Available at https://dx.doi.org/10.21037/qims-21-564
Conflicts of Interest: All authors have completed the ICMJE uniform disclosure form (available at https://dx.doi.org/10.21037/qims-21-564). The authors have no conflicts of interest to declare.
Ethical Statement: The authors are accountable for all aspects of the work in ensuring that questions related to the accuracy or integrity of any part of the work are appropriately investigated and resolved. The study was conducted in accordance with the Declaration of Helsinki (as revised in 2013). In this study, informed consent was not required because the study was conducted using human cadaver specimen. The specimens were obtained and approved according the regulations of the section anatomy of the Department of Neurosciences of the University Medical Center Groningen (University of Groningen, Groningen, the Netherlands).
Open Access Statement: This is an Open Access article distributed in accordance with the Creative Commons Attribution-NonCommercial-NoDerivs 4.0 International License (CC BY-NC-ND 4.0), which permits the non-commercial replication and distribution of the article with the strict proviso that no changes or edits are made and the original work is properly cited (including links to both the formal publication through the relevant DOI and the license). See: https://creativecommons.org/licenses/by-nc-nd/4.0/.
References
- Hooper T, Eccles G, Milliken T, Mathieu-Burry JR, Reed W. Dose reduction in CT imaging for facial bone trauma in adults: A narrative literature review. J Med Radiat Sci 2019;66:122-32. [Crossref] [PubMed]
- Brenner DJ, Hall EJ. Computed tomography--an increasing source of radiation exposure. N Engl J Med 2007;357:2277-84. [Crossref] [PubMed]
- Rozema R, Kruitbosch HT, van Minnen B, Dorgelo B, Kraeima J, van Ooijen PMA. Iterative reconstruction and deep learning algorithms for enabling low-dose computed tomography in midfacial trauma. Oral Surg Oral Med Oral Pathol Oral Radiol 2021;132:247-54. [Crossref] [PubMed]
- Willemink MJ, de Jong PA, Leiner T, de Heer LM, Nievelstein RA, Budde RP, Schilham AM. Iterative reconstruction techniques for computed tomography Part 1: technical principles. Eur Radiol 2013;23:1623-31. [Crossref] [PubMed]
- Liu L. Model-based Iterative Reconstruction: A Promising Algorithm for Today's Computed Tomography Imaging. J Med Imaging Radiat Sci 2014;45:131-6. [Crossref] [PubMed]
- Renieblas GP, Nogués AT, González AM, Gómez-Leon N, Del Castillo EG. Structural similarity index family for image quality assessment in radiological images. J Med Imaging (Bellingham) 2017;4:035501. [Crossref] [PubMed]
- Prieto G, Guibelalde E, Chevalier M, Turrero A. Use of the cross-correlation component of the multiscale structural similarity metric (R* metric) for the evaluation of medical images. Med Phys 2011;38:4512-7. [Crossref] [PubMed]
- Wang Z, Bovik AC, Sheikh HR, Simoncelli EP. Image quality assessment: from error visibility to structural similarity. IEEE Trans Image Process 2004;13:600-12. [Crossref] [PubMed]
- Wang Z, Simoncelli EP, Bovik AC. Multiscale structural similarity for image quality assessment. Available online: https://ieeexplore.ieee.org/document/1292216
- Rozema R, Doff MH, van Ooijen PM, Postmus D, Westerlaan HE, Boomsma MF, van Minnen B. Diagnostic reliability of low dose multidetector CT and cone beam CT in maxillofacial trauma-an experimental blinded and randomized study. Dentomaxillofac Radiol 2018;47:20170423. [Crossref] [PubMed]
- Marstal K, Berendsen F, Staring M, Klein S. SimpleElastix: A User-Friendly, Multi-lingual Library for Medical Image Registration. Available online: https://ieeexplore.ieee.org/document/7789568