Semi-automatic quantitative analysis of the pelvic bony structures on apparent diffusion coefficient maps based on deep learning: establishment of reference ranges
Introduction
Quantification of image features can be used to grade the severity of a disease, to determine appropriate treatment choices, and to monitor the treatment response (1,2). The use of multiparametric magnetic resonance imaging (MRI) coupled with diffusion-weighted imaging (DWI) and apparent diffusion coefficient (ADC) mapping is reported to provide both functional and quantitative information about normal and tumor (primary or metastatic) tissues (3,4). Specifically, in the MRI of the pelvis, ADC maps provide a potential response biomarker that reflects the molecular characteristics of tumors and suggests the best treatment response of bone metastases from prostate cancer (PCa) (5-7).
Pelvic bony structures, including the spine (lumbar vertebra, sacrococcyx), pelvis, and femur, are reportedly the most frequent sites of bone metastases from PCa (8,9). Calculation of the ADC values of the pelvic bones is relevant for the evaluation of PCa metastases (6). Radiologists will usually extract quantitative features by drawing regions of interest (ROIs) on metastatic and normal bony tissues (without metastasis), but this process is both time-consuming and labor intensive (10). Additionally, the measurement accuracy may be hampered by differences in the experience level of clinicians. It is therefore essential to develop an automated and objective ADC analysis method that can reduce the errors of manual analysis.
The automated segmentation of pelvic bony structures is a fundamental step in both automated pelvic image analysis and quantitative information extraction. Deep learning-based convolutional neural networks (CNNs) have been widely used for organ segmentation on magnetic resonance (MR) images (11,12), and they are capable of automatically learning relevant image features to achieve image segmentation (13,14). However, few studies have been conducted on the pelvic bony structure segmentation of MR images.
An abnormality is a significant deviation from the commonly accepted patterns of a normal background tissues (15), and ADC map values are significantly different between metastatic and normal tissues (16). Despite these differences, the differentiation of normal and abnormal tissues can be difficult unless consistent and precise ADC values can be obtained from the normal tissues. In this study, we developed a deep learning method for the segmentation of pelvic bony structures on ADC maps to establish reference ranges for the ADC parameters of normal pelvic bony structures. Our aim for this study is to provide a method for the automatic measurement of the ADC values of pelvic bony structures that could be used for the future detection of abnormalities.
Methods
The study was conducted in accordance with the Declaration of Helsinki (as revised in 2013) and was approved by the institutional review board (No. 20190701). Individual consent for this retrospective analysis was waived.
Data collection
The MR images of 944 consecutive patients who had undergone pelvic imaging for either clinically suspected or confirmed PCa between January 2018 and June 2020 were acquired from the picture archiving and communication system (PACS). The patients were selected according to the following inclusion criteria: (I) patients aged over 50 years, (II) DWI with low (b=0 s/mm2) and high (b=800 or 1,000 s/mm2) b values, and (III) no metastatic radiological characteristics [based on computed tomography (CT), multiparametric MR imaging (MRI) and, if available, bone scintigraphy or positron emission tomography CT (PET-CT)] within the pelvic scanning range. Patients with primary malignant bone tumors (n=24), a history of fractures or surgery (n=25), or benign lesions on pelvic bones (hemangioma: n=10; cyst: n=20; degeneration with an obvious abnormal signal: n=38; and undetermined: n=41) were not included in the analysis. Additionally, 9 patients were considered unevaluable because of incomplete DWI sequences, while 10 patients were excluded due to the suboptimal quality of their images (obvious motion artifacts). Finally, we performed ADC analyses of normal pelvic bony structures by using a data set of 693 patients who ranged from 50 to 95 years of age [including a subset of 288 patients randomly selected for model development (S1) and a subset of 405 patients used for model prediction (S2)]. A data set of 74 patients who received treatment [including 32 PCa patients who received radiotherapy (S3) and 42 PCa patients who received endocrine therapy (S4)] and who were not reported to have had previous pelvic bone metastases was collected for comparison with the ADC measurements. The flowchart of patient enrollment is shown in Figure 1.
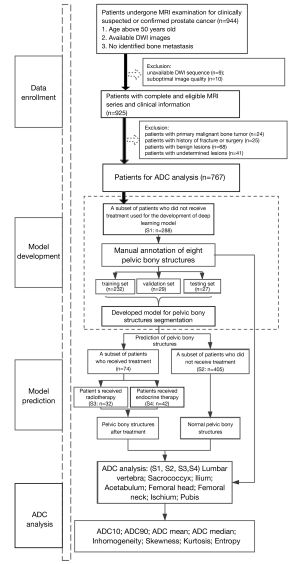
MRI sequences
All data used in our study were anonymized. All images were axial DW images of patients in the supine position acquired from the different b values of MR scanners from 4 different vendors used at our institution with a phased-array coil [3.0 T Achieva (Philips Healthcare, the Netherlands), 3.0 T Discovery (GE Healthcare, Milwaukee, WI, USA), 1.5 T Avanto (Siemens Medical Solutions, Erlangen, Germany), and 3.0 T Interia (Philips Healthcare, the Netherlands)]. Monoexponential ADC maps were created using software from each scanner. Detailed parameters are shown in Table 1.
Table 1
Typical parameters | 3.0 T Achieva (Philips Healthcare, the Netherlands) | 3.0 T Discovery (Ge healthcare, Milwaukee, WI, USA) | 1.5 T Avanto (Siemens Medical Solutions, Erlangen, Germany) | 3.0 T Interia (Philips Healthcare, the Netherlands) |
---|---|---|---|---|
b values (s/mm2) | 0, 800 | 0, 800 | 0, 800 | 0, 1,000 |
Echo time (ms) | 54 | 60 | 54 | 78 |
Repetition time (ms) | 3,400 | 3,000 | 3,300 | 4,959 |
Imaging matrix | 224×224 | 256×256 | 156×180 | 240×240 |
Field of view (mm) | 375 | 360 | 329 | 360 |
Section thickness (mm) | 6 | 8 | 7 | 7 |
Number of slices | 24 | 25 | 24 | 28 |
DWI, diffusion-weighted imaging.
Data annotation for the deep learning model
Digital Imaging and Communications in Medicine (DICOM) images were transformed into Neuroimaging Informatics Technology Initiative (NIfTI) files before analysis, and the images were annotated using ITK-SNAP software (version 3.6.0; http://www.itksnap.org). A subset of 288 patients (no treatment) with DW images (b=800 s/mm2 or b=1,000 s/mm2) and ADC maps were randomly chosen and set aside for algorithmic training purposes (S1). Eight pelvic bony structures (the lumbar vertebra, sacrococcyx, ilium, acetabulum, femoral head, femoral neck, ischium, and pubis) were manually annotated in full and section by section on ADC maps (mask 1 and mask 2) by 2 radiologists (both with more than 3 years of experience). A senior radiologist (with more than 20 years of experience in pelvic imaging) modified the 2 sets of manual annotations (mask 3 and mask 4, respectively). The inter- and intrareader reliability of the manual annotations was assessed by a Dice score, which was defined as the volume of overlap divided by the union volume between the 2 masks.
Specifically, only a portion of the lumbar vertebrae that were within the pelvic scanning range were annotated, usually from the third lumbar vertebra (L3) to the fifth (L5). The number of intervertebral disc slices was relatively small and incomplete due to the large slice thickness of the DW images (6–8 mm); thus, we annotated the lumbar vertebrae continuously, which contained slices of the intervertebral disc. Since DW and ADC images were coregistered by each scanner (the ADC maps were calculated from the DW images), the manually segmented labels on the ADC maps could be matched to the DW images. The senior radiologist reviewed each DW image label that had been copied from the ADC maps and made corrections when necessary. An example of an annotation result is shown in Figure 2.
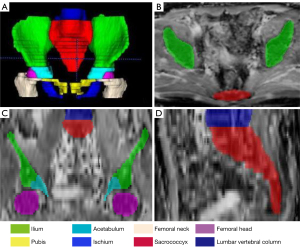
To count the number of pelvic bony structures, we considered the continuous lumbar vertebra to be a single structure, although it consists of 3–5 vertebrae, with the bony structures on the left and right sides considered 2 structures. Thus, there were 14 bony structures at most within scanning range of the pelvis (including 1 lumbar vertebra, 1 sacrococcyx, 2 ilia, 2 acetabula, 2 femoral heads, 2 femoral necks, 2 ischia, and 2 pubes).
Development of the deep learning model
A total of 288 DW images (b=800 or 1,000 s/mm2) and their corresponding ADC maps were randomly selected as input to develop a 3D U-Net CNN algorithm (17) for the automated segmentation of pelvic bony structures, with each sequence considered a separate input channel. The 288 patients were randomly divided into a training set (n=232), a validation set (n=29), and a testing set (n=27) at a ratio of 8:1:1. The classical 3D U-Net architecture is detailed in Appendix 1. All the input images were unified and resized to 64×240×240 (z, y, x) before training. To train the 3D U-Net segmentation models, we exploited an Adam optimizer with an initial learning rate of 10−4. The model was trained for 300 epochs until the validation loss failed to rise, and we used a fixed batch size of 2 to decrease the memory space. The tuning of other hyperparameters (such as weight initialization and dropout for regularization) was randomly searched and automatically executed in the validation set during U-Net development. The CNN was coded by Python3.6, Pytorch 0.4.1, Opencv, Numpy, and SimpleITK.
Quantitative ADC measurements
A subset of 405 patients who did not receive treatment (S2) and a subset of 74 patients who received treatment (S3 and S4) were used for pelvic bony structure segmentation by our proposed model. Before the ADC was calculated, the pelvic bony structures that were automatically segmented by the 3D U-Net CNN algorithm were manually corrected for any mistakes by the senior radiologist, who also verified that the predicted segmentation edges matched the true margins. After being manually corrected, the predicted segmentations were regarded as the reference standard to further assess the segmentation performance of the model as per the scanner and among the different groups of patients in the S2, S3, and S4 data sets.
The segmentations of the 8 pelvic bony structures on the ADC maps were regarded as volumes of interest (VOIs) for the calculation of the ADC value according to the following equation:
where S1 represents the signal intensity at a particular high b value (b1=800 or 1,000 s/mm2 in this study) and S0 represents the baseline signal without diffusion sensitization (b0=0 s/mm2).
An ADC histogram was generated for each VOI, and the following arguments were calculated in this research: 10th percentile (ADC10), 90th percentile (ADC90), ADCmean, ADCmedian, inhomogeneity, skewness, kurtosis, and entropy. The concepts of these parameters are shown in Appendix 2.
To further investigate the potential factors that may influence the ADC measurements of normal pelvic bony structures, we analyzed the effect of image acquisition parameters and age on ADC measurements. To explore whether endocrine therapy or radiotherapy would affect the ADC values, we compared the ADC parameters of patients who did or did not receive treatment (patients who did not receive treatment vs. patients who received endocrine therapy vs. patients who received radiotherapy).
Statistical analysis
Statistical analyses were performed using the SPSS 22.0 software package (IBM Corp., Armonk, NY, USA). Numerical data were averaged across all the patients and are reported as the mean ± standard deviation, while a one-way analysis of variance (ANOVA) was used for both age and Dice comparisons among the different subsets and different pelvic bony structures. For each group of ADC parameters, we used the 95% confidence interval (95% CI) to establish a reference range. The Kruskal-Wallis one-way analysis of variance (ANOVA; k-samples) with a pairwise comparison was used for multiple comparisons of the ADC parameters among the different image acquisition protocols (b value and field strength). Correlations between age, imaging parameters (b-value and field strength), treatment (with/without), and mean ADC values were analyzed by Spearman’s rank-order correlation coefficient. Statistical significance was set at P<0.05.
Results
Patient characteristics
A total of 767 patients were analyzed in this research, with the patient characteristics summarized in Table 2. There was no significant difference in age among the 4 data sets (F=0.431, P=0.786). Table 2 shows the detailed distribution of the bony structures from each data set.
Table 2
Characteristics | Subset of patients who did not receive |
Subset of patients who did not receive treatment (n=405) | Subset of patients who received |
Total (n=693) | |||
---|---|---|---|---|---|---|---|
Training set | Validation set | Testing set | Received |
Received endocrine therapy | |||
Patients | |||||||
No. of patients | 232 | 29 | 27 | 405 | 32 | 42 | 693 |
Age (mean ± SD, years) | 68.33±8.52 | 66.34±9.02 | 67.26±8.97 | 67.67±9.23 | 72.53±8.54 | 71.47±8.64 | 67.82±8.97 |
Pelvic bony structures | |||||||
No. of bony structures | 3,166 | 377 | 341 | 5,569 | 439 | 580 | 10,472 |
Lumbar vertebra | 212 | 24 | 22 | 374 | 23 | 34 | 689 |
Sacrococcyx | 232 | 29 | 27 | 405 | 32 | 42 | 767 |
Ilium | 464 | 58 | 54 | 810 | 64 | 84 | 1,534 |
Acetabulum | 462 | 58 | 52 | 806 | 64 | 84 | 1,526 |
Femoral head | 460 | 56 | 54 | 808 | 64 | 84 | 1,526 |
Femoral neck | 454 | 54 | 48 | 796 | 64 | 84 | 1,500 |
Ischium | 444 | 50 | 44 | 780 | 64 | 84 | 1,466 |
Pubis | 438 | 48 | 40 | 790 | 64 | 84 | 1,464 |
Vendors | |||||||
No. of patients | 232 | 29 | 27 | 405 | 32 | 42 | 693 |
3.0 T Achieva | 12 | 3 | 3 | 111 | 10 | 7 | 146 |
3.0 T Discovery | 155 | 18 | 15 | 100 | 6 | 14 | 308 |
1.5 T Avanto | 43 | 5 | 6 | 90 | 9 | 14 | 167 |
3.0 T Interia | 22 | 3 | 3 | 104 | 7 | 7 | 146 |
SD, standard deviation.
Reliability of the manual annotations
As shown in Table 3, the consistency between mask 1 and mask 2 (average Dice score: 0.87±0.03) was improved after modifications were made by a senior radiologist (average Dice score between mask 3 and mask 4: 0.97±0.03). The high Dice scores of the pelvic bony structures (all above 0.95) between mask 3 and mask 4 confirmed the reliability of the manual annotations.
Table 3
Pelvic bony structures | Mask 1 vs. Mask 2 | Mask 1 vs. Mask 3 | Mask 2 vs. Mask 4 | Mask 3 vs. Mask 4 |
---|---|---|---|---|
Lumbar vertebra | 0.88±0.08 | 0.91±0.05 | 0.92±0.04 | 0.95±0.03 |
Sacrococcyx | 0.88±0.06 | 0.90±0.03 | 0.89±0.05 | 0.98±0.01 |
Ilium | 0.90±0.06 | 0.91±0.04 | 0.90±0.03 | 0.95±0.02 |
Acetabulum | 0.88±0.06 | 0.91±0.03 | 0.91±0.05 | 0.96±0.01 |
Femoral head | 0.88±0.09 | 0.91±0.05 | 0.92±0.05 | 0.98±0.03 |
Femoral neck | 0.89±0.08 | 0.93±0.04 | 0.91±0.03 | 0.97±0.02 |
Ischium | 0.89±0.07 | 0.92±0.04 | 0.90±0.01 | 0.99±0.04 |
Pubis | 0.87±0.07 | 0.90±0.04 | 0.92±0.03 | 0.96±0.03 |
Average | 0.87±0.03 | 0.91±0.01 | 0.91±0.03 | 0.97±0.03 |
Mask 1 and mask 2 were from 1 of the 2 junior radiologists, respectively; mask 3 and mask 4 were the modifications made by a senior radiologist based on mask 1 and mask 2, respectively.
Segmentation accuracy of the deep learning model
Mask 4 was regarded as the reference standard for assessing the segmentation accuracy as indicated by computing the Dice scores between the CNN and manual segmentations. The Dice scores of the deep learning model for segmentation of the pelvic bony structures on the ADC maps ranged from 0.90±0.02 to 0.95±0.03 (Figure 3) in the testing set, with the femoral head and the femoral neck providing the highest Dice scores (0.95±0.03 and 0.94±0.03, respectively). Despite ANOVA testing revealing the scores of the ilium and the pubis to be significantly lower than those of the other regions, all the Dice scores were above 0.90.
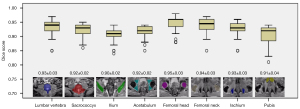
As shown in Table 4, the Dice score of each pelvic bony structure was not significantly different among the different scanners in the S2 data set (P>0.05), and no significant differences in Dice scores were found among the S2, S3, and S4 data sets. The exemplary segmentations are shown in Figure 4. The high Dice scores (all above 0.95) between the automated segmentation and manually corrected segmentation indicate that the manual corrections were not extensive and required only occasional and minor corrections. The main corrections were edits on the iliac region to modify the predicted segmentation edges (Figure 4A).
Table 4
Pelvic bony structures | S2 | S3 | S4 | P value | ||||
---|---|---|---|---|---|---|---|---|
3.0 T Achieva | 3.0 T Discovery | 1.5 T Avanto | 3.0 T Interia | P value | ||||
Lumbar vertebra | 0.92±0.03 | 0.93±0.04 | 0.92±0.04 | 0.92±0.03 | 0.918 | 0.91±0.04 | 0.91±0.03 | 0.349 |
Sacrococcyx | 0.92±0.05 | 0.93±0.03 | 0.94±0.04 | 0.91±0.04 | 0.482 | 0.90±0.06 | 0.91±0.04 | 0.266 |
Ilium | 0.93±0.05 | 0.93±0.04 | 0.90±0.05 | 0.93±0.05 | 0.568 | 0.91±0.05 | 0.90±0.03 | 0.078 |
Acetabulum | 0.92±0.02 | 0.93±0.05 | 0.92±0.04 | 0.92±0.02 | 0.505 | 0.91±0.04 | 0.91±0.04 | 0.629 |
Femoral head | 0.92±0.02 | 0.93±0.04 | 0.93±0.04 | 0.91±0.05 | 0.508 | 0.92±0.06 | 0.92±0.04 | 0.404 |
Femoral neck | 0.92±0.04 | 0.93±0.03 | 0.92±0.04 | 0.90±0.05 | 0.338 | 0.91±0.06 | 0.92±0.04 | 0.714 |
Ischium | 0.91±0.03 | 0.90±0.06 | 0.92±0.04 | 0.92±0.05 | 0.813 | 0.92±0.04 | 0.93±0.04 | 0.358 |
Pubis | 0.90±0.04 | 0.92±0.03 | 0.92±0.04 | 0.90±0.03 | 0.596 | 0.92±0.05 | 0.91±0.05 | 0.770 |
S2: a subset of patients who did not receive treatment used for model prediction. S3: a subset of patients who received radiotherapy. S4: a subset of patients who received endocrine therapy.
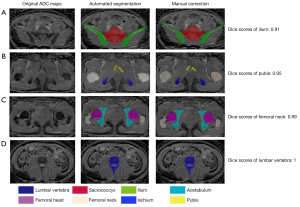
Correlations between various parameters and mean ADC values
As shown in Table 5, the b values (800 and 1,000) and field strength (3.0 and 1.5 T) of the pelvic bony structures were all significantly correlated with the ADC values (all P<0.001), while age and treatment (with or without) were not significant variables (all P>0.05).
Table 5
Pelvic bony structures | Age | b value | Field strength | Treatments |
---|---|---|---|---|
Lumbar vertebra | −0.055 (0.075) | 0.729 (0.001) | 3.323 (0.001) | 0.049 (0.098) |
Sacrococcyx | −0.032 (0.185) | 0.657 (0.001) | 0.401 (0.001) | 0.014 (0.348) |
Ilium | 0.057 (0.057) | 0.122 (0.001) | 0.352 (0.001) | −0.038 (0.148) |
Acetabulum | −0.019 (0.297) | 0.252 (0.001) | 0.550 (0.001) | −0.072 (0.054) |
Femoral head | −0.029 (0.215) | 0.369 (0.001) | 0.635 (0.001) | −0.045 (0.109) |
Femoral neck | −0.017 (0.326) | 0.452 (0.001) | 0.431 (0.001) | −0.052 (0.078) |
Ischium | 0.001 (0.499) | 0.066 (0.038) | 0.670 (0.001) | −0.100 (0.003) |
Pubis | 0.019 (0.302) | 0.190 (0.001) | 0.194 (0.001) | 0.032 (0.191) |
Unless otherwise indicated, data are correlation coefficients (R) and P values. ADC, apparent diffusion coefficient.
The effect of image acquisition parameters on ADC measurements
The ADC histogram analyses of 693 patients with normal pelvic bony structures are presented in Table 6 for each anatomic region, which shows that the image acquisition parameters had a significant impact on the ADC measurements of pelvic bony structures. The scanner with the lowest b value and field strength also yielded the lowest mean ADC measurements on pelvic bony structures except for in the femoral neck and the ischium (lumbar vertebra: 0.93±0.12; sacrococcyx: 0.63±0.09; ilium: 0.47±0.08; acetabulum: 0.41±0.09; femoral head: 0.26±0.05; femoral neck: 0.26±0.06; ischium 0.27±0.07; and pubis: 0.50±0.08). The scanner with the highest b value and field strength (3.0 T Interia: b=1,000 s/mm2) was shown to yield the highest mean ADC measurements on pelvic bony structures except for in the femoral neck and the ischium (lumbar vertebra: 1.52±0.16; sacrococcyx:1.13±0.14; ilium: 0.60±0.12; acetabulum: 0.66±0.12; femoral head: 0.56±0.11; and pubis: 0.63±0.12).
Table 6
Pelvic bony structures | MRI vendors (s/mm2) | No. of patients | ADC10 (95% CI) | ADCmean (95% CI) | ADCmedian (95% CI) | ADC90 (95% CI) |
---|---|---|---|---|---|---|
Lumbar vertebra (n=632) | 3.0 T Achieva (b=800) | 81 | 0.32±0.16 (0.37–0.44) | 1.01±0.33& (0.94–1.09) | 0.86±0.29$ (0.80–0.92) | 3.36±1.13& (3.11–3.61) |
3.0 T Discovery (b=800) | 305 | 0.30±0.03 (0.29–0.30) | 1.06±0.09& (1.05–1.07) | 0.96±0.11& (0.94–0.97) | 2.99±0.47$ (2.94–3.04) | |
1.5 T Avanto (b=800) | 126 | 0.30±0.08 (0.29–0.32) | 0.93±0.12 (0.90–0.95) | 0.81±0.14$ (0.78–0.83) | 2.07±0.39$ (2.00–2.14) | |
3.0 T Intera (b=1,000) | 120 | 0.50±0.18* (0.47–0.53) | 1.52±0.16* (1.49–1.54) | 1.29±0.20* (1.25–1.32) | 4.52±0.75* (4.38–4.65) | |
Reference range | 632 | – | (0.90–1.54) | – | – | |
Sacrococcyx (n=693) | 3.0 T Achieva (b=800) | 111 | 0.38±0.14& (0.35–0.41) | 0.74±0.24& (0.70–0.79) | 0.58±0.19$ (0.54–0.61) | 2.87±0.88& (2.70–3.04) |
3.0 T Discovery (b=800) | 308 | 0.24±0.02$ (0.24–0.25) | 0.80±0.07& (0.79–0.81) | 0.68±0.08& (0.67–0.69) | 2.45±0.31$ (2.42–2.48) | |
1.5 T Avanto (b=800) | 142 | 0.19±0.04 (0.18–0.19) | 0.63±0.09 (0.61–0.64) | 0.51±0.08 (0.50–0.52) | 1.87±0.34 (1,81–1.92) | |
3.0 T Intera (b=1,000) | 132 | 0.49±0.18* (0.46–0.52) | 1.13±0.14* (1.10–1.15) | 0.84±0.13* (0.82–0.86) | 3.83±0.71* (3.72–3.96) | |
Reference range | 693 | – | (0.61–1.15) | – | – | |
Ilium (n=1,386) | 3.0 T Achieva (b=800) | 111 | 0.28±0.10& (0.26–0.29) | 0.49±0.17 (0.45–0.52) | 0.38±0.15 (0.35–0.41) | 2.09±0.65& (1.97–2.21) |
3.0 T Discovery (b=800) | 308 | 0.23±0.02$ (0.22–0.24) | 0.63±0.07* (0.62–0.64) | 0.51±0.07* (0.51–0.52) | 2.22±0.22& (0.20–0.25) | |
1.5 T Avanto (b=800) | 142 | 0.15±0.03 (0.15–0.16) | 0.47±0.08 (0.46–0.48) | 0.40±0.08 (0.39–0.42) | 1.36±0.15$ (1.34–1.39) | |
3.0 T Intera (b=1,000) | 132 | 0.32±0.11* (0.30–0.34) | 0.60±0.12* (0.58–0.62) | 0.47±0.11* (0.45–0.49) | 2.62±0.44* (2.54–2.69) | |
Reference range | 693 | – | (0.45–0.64) | – | – | |
Acetabulum (n=1,378) | 3.0 T Achieva (b=800) | 111 | 0.25±0.08* (0.23–0.26) | 0.51±0.19& (0.47–0.54) | 0.40±0.17$ (0.37–0.43) | 1.94±0.62* (1.82–2.05) |
3.0 T Discovery (b=800) | 304 | 0.19±0.03& (0.18–0.19) | 0.67±0.08* (0.66–0.68) | 0.56±0.08* (0.55–0.57) | 1.78±0.18& (1.76–1.80) | |
1.5 T Avanto (b=800) | 142 | 0.12±0.03$ (0.12–0.13) | 0.41±0.09$ (0.40–0.43) | 0.34±0.09 (0.33–0.36) | 1.20±0.18$ (1.17–1.23) | |
3.0 T Intera (b=1,000) | 132 | 0.25±0.08* (0.23–0.26) | 0.66±0.12* (0.64–0.69) | 0.52±0.11& (0.50–0.54) | 2.31±0.41* (2.24–2.39) | |
Reference range | 689 | – | (0.40–0.69) | – | – | |
Femoral head (n=1,378) | 3.0 T Achieva (b=800) | 111 | 0.23±0.08* (0.21–0.24) | 0.40±0.15$ (0.37–0.43) | 0.25±0.13$ (0.22–0.27) | 1.72±0.54& (1.61–1.82) |
3.0 T Discovery (b=800) | 304 | 0.16±0.03& (0.15–0.16) | 0.53±0.10& (0.51–0.53) | 0.40±0.12& (0.39–0.41) | 1.73±0.21& (1.71–1.76) | |
1.5 T Avanto (b=800) | 142 | 0.08±0.02$ (0.08–0.09) | 0.26±0.05 (0.25–0.27) | 0.15±0.07 (0.14–0.16) | 1.00±0.15$ (0.98–1.03) | |
3.0 T Intera (b=1,000) | 132 | 0.24±0.07* (0.23–0.25) | 0.56±0.11* (0.55–0.58) | 0.45±0.10* (0.43–0.47) | 2.09±0.43* (2.01–2.16) | |
Reference range | 689 | – | (0.25–0.58) | – | – | |
Femoral neck (n=1,352) | 3.0 T Achieva (b=800) | 110 | 0.24±0.08* (0.22–0.25) | 0.44±0.16& (0.41–0.47) | 0.33±0.13& (0.30–0.36) | 1.87±0.64* (1.75–1.99) |
3.0 T Discovery (b=800) | 293 | 0.18±0.02& (0.18–0.19) | 0.50±0.08* (0.49–0.51) | 0.36±0.09* (0.35–0.37) | 1.96±0.24* (1.93–1.99) | |
1.5 T Avanto (b=800) | 141 | 0.11±0.01$ (0.10–0.11) | 0.26±0.06$ (0.25–0.27) | 0.14±0.09$ (0.12–0.16) | 1.13±0.13 (1.11–1.15) | |
3.0 T Intera (b=1,000) | 132 | 0.23±0.08* (0.22–0.24) | 0.47±0.07& (0.45–0.48) | 0.36±0.07* (0.34–0.37) | 2.02±0.39* (1.96–2.09) | |
Reference range | 676 | – | (0.25–0.51) | – | – | |
Ischium (n=1,318) | 3.0 T Achieva (b=800) | 101 | 0.18±0.06* (0.17–0.19) | 0.44±0.16& (0.41–0.47) | 0.35±0.14& (0.32–0.38) | 1.51±0.49* (1.41–1.60) |
3.0 T Discovery (b=800) | 286 | 0.16±0.02* (0.15–0.16) | 0.54±0.08* (0.53–0.55) | 0.44±0.07* (0.43–0.45) | 1.56±0.18* (1.54–1.58) | |
1.5 T Avanto (b=800) | 140 | 0.08±0.02 (0.08–0.09) | 0.27±0.07$ (0.26–0.28) | 0.20±0.10$ (0.19–0.22) | 0.90±0.12 (0.88–0.92) | |
3.0 T Intera (b=1,000) | 132 | 0.18±0.06* (0.17–0.19) | 0.46±0.09& (0.45–0.48) | 0.36±0.09& (0.34–0.37) | 1.64±0.33* (1.59–1.70) | |
Reference range | 659 | – | (0.26–0.55) | – | – | |
Pubis (n=1,316) | 3.0 T Achieva (b=800) | 100 | 0.19±0.07 (0.17–0.20) | 0.49±0.18& (0.45–0.52) | 0.43±0.18& (0.40–0.47) | 1.40±0.48& (1.30–1.49) |
3.0 T Discovery (b=800) | 286 | 0.21±0.07* (0.20–0.22) | 0.61±0.10* (0.60–0.62) | 0.56±0.10* (0.55–0.58) | 1.21±0.14& (1.19–1.22) | |
1.5 T Avanto (b=800) | 140 | 0.24±0.07* (0.23–0.26) | 0.50±0.08& (0.48–0.51) | 0.45±0.08& (0.44–0.47) | 0.88±0.12$ (0.86–0.90) | |
3.0 T Intera (b=1,000) | 132 | 0.23±0.08* (0.21–0.24) | 0.63±0.12* (0.61–0.65) | 0.57±0.12* (0.55–0.59) | 1.56±0.29* (1.51–1.61) | |
Reference range | 658 | – | (0.45–0.65) | – | – |
The symbols “*”, “&”, and “$” represent the values from high to low with significant differences (*, in the significantly highest value among the 4 groups; &, in the second highest value; $, in the third highest value, value without symbol indicates the lowest value). Data with the same symbol indicates that the difference was not significant. ADC, apparent diffusion coefficient.
However, scanners with the same field strength and b value (3.0 T Achieva: b=800 s/mm2; 3.0 T Discovery: b=800 s/mm2) showed significant differences on ADC measurements except for the lumbar vertebra (sacrococcyx: 0.74±0.24 vs. 0.80±0.07; ilium: 0.49±0.17 vs. 0.63±0.07; acetabulum: 0.51±0.19 vs. 0.67±0.08; femoral head: 0.40±0.15 vs. 0.53±0.10; femoral neck: 0.44±0.16 vs. 0.50±0.08; ischium 0.44±0.16 vs. 0.54±0.08; and pubis: 0.49±0.18 vs. 0.61±0.10, all P values <0.001).
In this study, we established the reference ranges for ADC values using a general CI that contained all 4 CIs, with the lower limit of the general CI being the lowest value among the 4 CIs, and vice versa. As shown in Table 6, the reference ranges (95% CI) for normal pelvic bony structures were as follows: 0.90–1.54 for the lumbar vertebra, 0.61–1.15 for the sacrococcyx, 0.45–0.64 for the ilium, 0.40–0.6 for the acetabulum, 0.25-0.58 for the femoral head, 0.25–0.51 for the femoral neck, 0.26–0.55 for the ischium, and 0.45–0.65 for the pubis. Detailed comparisons of the ADC histogram parameters for the different image acquisition parameters are shown in Table S1.
Discussion
This research presents a CNN-based method for the automated segmentation of pelvic bony structures on ADC maps. Focusing on pelvic parts most commonly affected by metastases from PCa, we established a reference range for the ADC values of normal pelvic bony structures using the 95% CI for a group of patients over 50 years of age who had clinically suspected or confirmed PCa. Using the Dice score as a quantitative evaluation criterion, we found the CNN-based method in segmenting 8 pelvic bony structures performed satisfactorily, with Dice scores ranging from 0.90±0.02 to 0.95±0.03 in the testing set. Significant differences in the mean ADC values among different image acquisition parameters were observed in this study. In addition, age and treatment (with or without) were not correlated with the mean ADC values of the pelvic bony structures.
Automated segmentation of pelvic bony structures lays a foundation for subsequent quantitative ADC calculation. The automated segmentation approach for ADC maps presented herein represents a promising step toward an MRI-based quantitative analysis of bone metastases from PCa. Deep learning–based quantitative analyses on medical images reportedly have practical uses in many areas, for example, the fully automatic quantification of left ventricle function from cine MR images (18) and the automated liver biometry on CT and MR images (19). To achieve objectivity and accuracy for a quantitative analysis system, a reliable segmentation algorithm is mandatory, and it usually requires a data set with high variability for model development (18,20). In this study, a total of 767 PCa patients were recruited for ADC analysis. Completing a manual annotation of pelvic bony structure for every patient is laborious and time-consuming. Moreover, there is no clinically validated model that can be used for the automated segmentation of the 8 pelvic bony structures. Therefore, we manually annotated a subset of pelvic data (n=288) for this study and applied them for the segmentation model development, with the developed model then used to predict the rest of the pelvic data (n=479). Our results showed that the model that was trained with 288 patients achieved excellent segmentation performance in the S2, S3, and S4 data sets. The high Dice scores of the automated and manually corrected segmentations indicated that the segmentation performance of the model could achieve the same level of results as those of manual annotation.
To avoid any sampling bias caused by the selection of a localized region in the pelvic bony structures, the VOIs were determined from the ADC maps of each whole pelvic bony structure, as this may be a more reliable approach and could improve the reproducibility of the ADC value and its derivative indicators.
The value of quantitative ADC measurements has been well demonstrated, especially in differentiating the diagnoses and prognoses of different cancers. Assessments with ADC histograms might provide more reliable results that reflect the biological characteristics of heterogeneous lesions (21,22). Despite numerous studies on quantitative ADC analyses, there is a lack of sufficiently robust data on the reference range derived from normal pelvic bony structures. Our study showed that the reference ranges of ADC values for normal pelvic structures could be defined as follows: (0.90–1.54)×10−3 mm2/s for the lumbar vertebra, (0.61–1.15)×10−3 mm2/s for the sacrococcyx, (0.45–0.64)×10−3 mm2/s for the ilium, (0.40–0.69)×10−3 mm2/s for the acetabulum, (0.25–0.58)×10−3 mm2/s for the femoral head, (0.25–0.51)×10−3 mm2/s for the femoral neck, (0.26–0.55)×10−3 mm2/s for the ischium, and (0.45–0.65)×10−3 mm2/s for the pubis.
A previously conducted study reported similar reference ranges to these (16), and defined normal ADC values of (0.43±0.17)×10−3 mm2/s for the iliac crest, 0.33±0.20×10−3 mm2/s for the lumbar vertebrae, and (0.21±0.16)×10−3 mm2/s for the femur in healthy subjects (n=32). These values were outside the lower limit of the reference ranges reported in our study, which might have been due to the lower b value (400 s/mm2) of DW images used to create the ADC maps. A large difference was observed for the lumbar vertebrae [0.33 vs. (0.90–1.54)], as the structure of the lumbar vertebrae in our study contained slices of the vertebral body and disc, resulting in larger ADC values. The upper limit of the reference range is more meaningful than is the lower limit, because metastatic lesions can increase the ADC value of bony structures (23,24). Thus, various research results should be integrated to obtain a universal upper limit of normal bony structures as a threshold for a differential diagnosis across all involved vendors.
We found that different image acquisition parameters including both the diffusion coefficient b value and field strength could have influenced the ADC measurements, as it has been proven that ADC values may differ among the imaging phantoms of different MRI systems (25). Given the statistical differences found among the 4 different scanners, 4 normal distributions of ADC values with 4 CIs were established in this study. However, according to the CIs shown in Table 6, the reference ranges among the different scanners overlap, owing to the interaction of the b value and field strength. Thus, specifying the reference range of each scanner as per their b value and field strength is considered to be overly complicated. Additionally, the statistical differences among the scanners showed no clinical significance and were far fewer in number than the ADC differences between the normal and metastatic pelvic bony structures. Similarly, Messiou et al. (26) established a normal pelvic bone ADC value of (0.47±0.14)×10−3 mm2/s and a metastatic ADC value of (0.98±0.36)×10−3 mm2/s. The difference (approximately 0.51×10−3 mm2/s) between the normal and metastatic bones was larger than the difference observed between the different scanners (as shown in Table 6). Additionally, Nonomura et al. (27) found that the ADC difference in the ilium between the normal and metastatic marrow on a 1.5T MR system was 0.48×10−3 mm2/s (0.8 “versus” 1.3), which was much larger than the ADC difference in the ilium found between the 1.5 and 3.0 T scanners (0.16×10−3 mm2/s). Therefore, this study established general reference ranges for the ADC values that contained all 4 CIs, which is more practicable in clinical settings. While the reference ranges for the ADC values established in this study were applicable for patients in whom the same scanning parameters were used (field strength and b values), the type of MR scanner and imaging protocol should be considered when applying the reference range to clinical practice. Since a specific and widely accepted protocol for quality controls in DWI is still lacking (28), the development of a set of quality control procedures is critical to successful validation if the ADC is to become a useful biomarker in the future (29).
In this study, patient age (>50 years) did not seem to be a statistically significant influence on the ADC measurements, which was inconsistent with the conclusion drawn by Lavdas et al., who noted that the ADCs of bone marrow show significant change with age (10). This discrepancy might be due to the difference in the age range of the patients enrolled in this study. Considering that PCa is more likely to develop in older patients (30,31), we chose to recruit patients for our research that were older than 50 (mean age 67.82±8.97 years, range 50–85 years), while those in the study by Lavdas et al. were younger (mean age 38 years, range 23–68 years). The age-related bone marrow conversion pattern varies by age group and body part; thus, if the reference range can be specified to a specific patient population, it could aid in diagnosis and differential pathology (32).
We recognize several limitations of this study. First, the manual annotations of the 8 pelvic bony structures were based on the anatomical knowledge of the clinicians attached to this study, which introduced a certain degree of subjectivity. Second, for ADC quantification of the lumbar vertebrae and sacrococcyx, we did not exclude the areas of the intervertebral disc and the spinal canal, resulting in higher ADC values of the lumbar vertebra and sacrococcyx. In future studies, spinal canal and intervertebral disc segmentation should be used to further improve the accuracy of ADC analyses. Third, the number of patients who received radiotherapy and endocrine therapy and were also subjected to ADC analysis was small, so a larger sample is needed to establish reference ranges for these patient types. Fourth, selection bias might have been present because all patients in this study were over 50 years of age, and ADC parameters from younger patients were not analyzed.
In conclusion, we established reference ranges of ADC values for normal pelvic bony structures by using a deep learning-based method. The algorithms and measurements presented in this article could provide a basis for developing quantitative radiologic reports in the future.
Acknowledgments
Funding: This work was supported by the Capital’s Fund for Health Improvement and Research (No. 2020-2-40710).
Footnote
Conflicts of Interest: All authors have completed the ICMJE uniform disclosure form (available at https://dx.doi.org/10.21037/qims-21-123). YZ and XW from the commercial company, Beijing Smart Tree Medical Technology Co. Ltd, were collaborating scientists providing technical support under the collaboration regulations and had no financial or other conflicts of interests with respect to this study. The other authors have no conflicts of interest to declare.
Ethical Statement: The authors are accountable for all aspects of the work in ensuring that questions related to the accuracy or integrity of any part of the work are appropriately investigated and resolved. This study was conducted in accordance with the Declaration of Helsinki (as revised in 2013) and was approved by institutional review board (No. 20190701). Individual consent for this retrospective analysis was waived.
Open Access Statement: This is an Open Access article distributed in accordance with the Creative Commons Attribution-NonCommercial-NoDerivs 4.0 International License (CC BY-NC-ND 4.0), which permits the non-commercial replication and distribution of the article with the strict proviso that no changes or edits are made and the original work is properly cited (including links to both the formal publication through the relevant DOI and the license). See: https://creativecommons.org/licenses/by-nc-nd/4.0/.
References
- Lin C, Luciani A, Itti E, El-Gnaoui T, Vignaud A, Beaussart P, Lin SJ, Belhadj K, Brugières P, Evangelista E, Haioun C, Meignan M, Rahmouni A. Whole-body diffusion-weighted magnetic resonance imaging with apparent diffusion coefficient mapping for staging patients with diffuse large B-cell lymphoma. Eur Radiol 2010;20:2027-38. [Crossref] [PubMed]
- Winkel DJ, Breit HC, Shi B, Boll DT, Seifert HH, Wetterauer C. Predicting clinically significant prostate cancer from quantitative image features including compressed sensing radial MRI of prostate perfusion using machine learning: comparison with PI-RADS v2 assessment scores. Quant Imaging Med Surg 2020;10:808-23. [Crossref] [PubMed]
- Padhani AR, Tunariu N. Metastasis Reporting and Data System for Prostate Cancer in Practice. Magn Reson Imaging Clin N Am 2018;26:527-42. [Crossref] [PubMed]
- Ei Khouli RH, Jacobs MA, Mezban SD, Huang P, Kamel IR, Macura KJ, Bluemke DA. Diffusion-weighted imaging improves the diagnostic accuracy of conventional 3.0-T breast MR imaging. Radiology 2010;256:64-73. [Crossref] [PubMed]
- Stecco A, Trisoglio A, Soligo E, Berardo S, Sukhovei L, Carriero A. Whole-Body MRI with Diffusion-Weighted Imaging in Bone Metastases: A Narrative Review. Diagnostics (Basel) 2018;8:45. [Crossref] [PubMed]
- Perez-Lopez R, Mateo J, Mossop H, Blackledge MD, Collins DJ, Rata M, Morgan VA, Macdonald A, Sandhu S, Lorente D, Rescigno P, Zafeiriou Z, Bianchini D, Porta N, Hall E, Leach MO, de Bono JS, Koh DM, Tunariu N. Diffusion-weighted Imaging as a Treatment Response Biomarker for Evaluating Bone Metastases in Prostate Cancer: A Pilot Study. Radiology 2017;283:168-77. [Crossref] [PubMed]
- Reischauer C, Froehlich JM, Koh DM, Graf N, Padevit C, John H, Binkert CA, Boesiger P, Gutzeit A. Bone metastases from prostate cancer: assessing treatment response by using diffusion-weighted imaging and functional diffusion maps--initial observations. Radiology 2010;257:523-31. [Crossref] [PubMed]
- An H, Tao N, Li J, Guan Y, Wang W, Wang Y, Wang F. Detection of Prostate Cancer Metastasis by Whole Body Magnetic Resonance Imaging Combined with Bone Scintigraphy and PSA Levels. Cell Physiol Biochem 2016;40:1052-62. [Crossref] [PubMed]
- Cooperberg MR, Broering JM, Carroll PR. Time trends and local variation in primary treatment of localized prostate cancer. J Clin Oncol 2010;28:1117-23. [Crossref] [PubMed]
- Lavdas I, Rockall AG, Castelli F, Sandhu RS, Papadaki A, Honeyfield L, Waldman AD, Aboagye EO. Apparent Diffusion Coefficient of Normal Abdominal Organs and Bone Marrow From Whole-Body DWI at 1.5 T: The Effect of Sex and Age. AJR Am J Roentgenol 2015;205:242-50. [Crossref] [PubMed]
- Yamashita R, Nishio M, Do RKG, Togashi K. Convolutional neural networks: an overview and application in radiology. Insights Imaging 2018;9:611-29. [Crossref] [PubMed]
- Borra D, Andalò A, Paci M, Fabbri C, Corsi C. A fully automated left atrium segmentation approach from late gadolinium enhanced magnetic resonance imaging based on a convolutional neural network. Quant Imaging Med Surg 2020;10:1894-907. [Crossref] [PubMed]
- LeCun Y, Bengio Y, Hinton G. Deep learning. Nature 2015;521:436-44. [Crossref] [PubMed]
- Park J, Yun J, Kim N, Park B, Cho Y, Park HJ, Song M, Lee M, Seo JB. Fully Automated Lung Lobe Segmentation in Volumetric Chest CT with 3D U-Net: Validation with Intra- and Extra-Datasets. J Digit Imaging 2020;33:221-30. [Crossref] [PubMed]
- Pathan F, D'Elia N, Nolan MT, Marwick TH, Negishi K. Normal Ranges of Left Atrial Strain by Speckle-Tracking Echocardiography: A Systematic Review and Meta-Analysis. J Am Soc Echocardiogr 2017;30:59-70.e8. [Crossref] [PubMed]
- Jacobs MA, Macura KJ, Zaheer A, Antonarakis ES, Stearns V, Wolff AC, Feiweier T, Kamel IR, Wahl RL, Pan L. Multiparametric Whole-body MRI with Diffusion-weighted Imaging and ADC Mapping for the Identification of Visceral and Osseous Metastases From Solid Tumors. Acad Radiol 2018;25:1405-14. [Crossref] [PubMed]
- Cicek O, Abdulkadir A, Lienkamp SS, Brox T, Ronneberger O. 3D U-Net: learning dense volumetric segmentation from sparse annotation. Medical Image Computing and Computer-Assisted Intervention - MICCAI 2016 19th International Conference Proceedings: LNCS 9901 2016:424-32.
- Tao Q, Yan W, Wang Y, Paiman EHM, Shamonin DP, Garg P, Plein S, Huang L, Xia L, Sramko M, Tintera J, de Roos A, Lamb HJ, van der Geest RJ. Deep Learning-based Method for Fully Automatic Quantification of Left Ventricle Function from Cine MR Images: A Multivendor, Multicenter Study. Radiology 2019;290:81-8. [Crossref] [PubMed]
- Wang K, Mamidipalli A, Retson T, Bahrami N, Hasenstab K, Blansit K, Bass E, Delgado T, Cunha G, Middleton MS, Loomba R, Neuschwander-Tetri BA, Sirlin CB, Hsiao A. members of the NASH Clinical Research Network. Automated CT and MRI Liver Segmentation and Biometry Using a Generalized Convolutional Neural Network. Radiol Artif Intell 2019;1:180022 [Crossref] [PubMed]
- Gaonkar B, Beckett J, Villaroman D, Ahn C, Edwards M, Moran S, Attiah M, Babayan D, Ames C, Villablanca JP, Salamon N, Bui A, Macyszyn L. Quantitative Analysis of Neural Foramina in the Lumbar Spine: An Imaging Informatics and Machine Learning Study. Radiol Artif Intell 2019;1:180037 [Crossref] [PubMed]
- Preda L, Casale S, Fanizza M, Fiore MR, Viselner G, Paganelli C, Buizza G, Fontana G, Vitolo V, Barcellini A, Baroni G, Fossati P, Valvo F. Predictive role of Apparent Diffusion Coefficient (ADC) from Diffusion Weighted MRI in patients with sacral chordoma treated with carbon ion radiotherapy (CIRT) alone. Eur J Radiol 2020;126:108933 [Crossref] [PubMed]
- Barrett T, Lawrence EM, Priest AN, Warren AY, Gnanapragasam VJ, Gallagher FA, Sala E. Repeatability of diffusion-weighted MRI of the prostate using whole lesion ADC values, skew and histogram analysis. Eur J Radiol 2019;110:22-9. [Crossref] [PubMed]
- Ward R, Caruthers S, Yablon C, Blake M, DiMasi M, Eustace S. Analysis of diffusion changes in posttraumatic bone marrow using navigator-corrected diffusion gradients. AJR Am J Roentgenol 2000;174:731-4. [Crossref] [PubMed]
- Chan JH, Peh WC, Tsui EY, Chau LF, Cheung KK, Chan KB, Yuen MK, Wong ET, Wong KP. Acute vertebral body compression fractures: discrimination between benign and malignant causes using apparent diffusion coefficients. Br J Radiol 2002;75:207-14. [Crossref] [PubMed]
- Kıvrak AS, Paksoy Y, Erol C, Koplay M, Özbek S, Kara F. Comparison of apparent diffusion coefficient values among different MRI platforms: a multicenter phantom study. Diagn Interv Radiol 2013;19:433-7. [Crossref] [PubMed]
- Messiou C, Collins DJ, Morgan VA, Desouza NM. Optimising diffusion weighted MRI for imaging metastatic and myeloma bone disease and assessing reproducibility. Eur Radiol 2011;21:1713-8. [Crossref] [PubMed]
- Nonomura Y, Yasumoto M, Yoshimura R, Haraguchi K, Ito S, Akashi T, Ohashi I. Relationship between bone marrow cellularity and apparent diffusion coefficient. J Magn Reson Imaging 2001;13:757-60. [Crossref] [PubMed]
- Belli G, Busoni S, Ciccarone A, Coniglio A, Esposito M, Giannelli M, et al. Quality assurance multicenter comparison of different MR scanners for quantitative diffusion-weighted imaging. J Magn Reson Imaging 2016;43:213-9. [Crossref] [PubMed]
- deSouza NM, Winfield JM, Waterton JC, Weller A, Papoutsaki MV, Doran SJ, Collins DJ, Fournier L, Sullivan D, Chenevert T, Jackson A, Boss M, Trattnig S, Liu Y. Implementing diffusion-weighted MRI for body imaging in prospective multicentre trials: current considerations and future perspectives. Eur Radiol 2018;28:1118-31. [Crossref] [PubMed]
- Droz JP, Albrand G, Gillessen S, Hughes S, Mottet N, Oudard S, Payne H, Puts M, Zulian G, Balducci L, Aapro M. Management of Prostate Cancer in Elderly Patients: Recommendations of a Task Force of the International Society of Geriatric Oncology. Eur Urol 2017;72:521-31. [Crossref] [PubMed]
- Boukovala M, Spetsieris N, Efstathiou E. Systemic Treatment of Prostate Cancer in Elderly Patients: Current Role and Safety Considerations of Androgen-Targeting Strategies. Drugs Aging 2019;36:701-17. [Crossref] [PubMed]
- Li Q, Pan SN, Yin YM, Li W, Chen ZA, Liu YH, Wu ZH, Guo QY. Normal cranial bone marrow MR imaging pattern with age-related ADC value distribution. Eur J Radiol 2011;80:471-7. [Crossref] [PubMed]