Dynamic contrast-enhanced magnetic resonance imaging for risk stratification in patients with prostate cancer
Introduction
Prostate cancer (PCa) is one of the most well recognized cancers for individualized treatment based on tumor aggressiveness, staging and recurrence risk (1,2). Gleason Score (GS), derived from the Gleason grading system, is the classification system based on the architectural pattern of the PCa tissue, which is related to cancer aggressiveness. GS ≥7 is grouped and classified as clinically significant cancer (CSC), while GS ≤6 is classified as non-CSC, aiding in individualized treatment plans for PCa patients (3). When assessing tumor aggressiveness by the invasive approach with classic systemic trans-rectal ultrasound guided biopsy (TRUS-Bx), it is widely accepted that sampling error is inevitable and that a likelihood of complications from invasive procedures exists (4-6). Therefore, the need for a functional imaging modality such as dynamic contrast-enhanced magnetic resonance imaging (DCE-MRI) has emerged in non-invasive evaluation of tumor aggressiveness.
When a malignant tumor develops in the base of the normal prostate gland, the cells go through a process called “angiogenesis.” This, the development of a new network of weak blood vessels, is a complex process resulting from the diverse contributions of endothelial cells, the extracellular matrix, as well as soluble factors such as vascular endothelial growth factor and fibroblast growth factor (7). Typical features of the newly developed blood vessels are weakness in terms of high permeability to macromolecules, arteriovenous shunt formation and high vascular tortuosity, heterogeneity of the tumor and intermittent or unstable blood flow due to the gradient from increased interstitial pressure. DCE-MRI aids in visualizing all of these characteristics of angiogenesis (8).
There have been attempts to assess PCa aggressiveness with DCE-MRI using various pharmacokinetic models and diverse post-processing software programs (9-13). However, the results are inconsistent and controversial, and in any case require further verification. Moreover, most of those studies reported differences of perfusion parameters between non-cancerous and cancerous lesions in the prostate gland, or perfusion parameters derived from a single slice of the tumor, or TRUS-Bx results that can serve as a reference standard (10,12). Thus, there remains a research gap in terms of tumor heterogeneity in the whole tumor volume and sampling error associated with TRUS-Bx.
Therefore, in the present study, we aimed to verify the usefulness of DCE-perfusion parameters for the whole-tumor volume in evaluation of PCa aggressiveness based on a pathological topographic map as a reference standard.
We present the following article in accordance with the STARD reporting checklist (available at https://dx.doi.org/10.21037/qims-21-455).
Methods
The study was conducted in accordance with the Declaration of Helsinki (as revised in 2013). This retrospective study was approved by the institutional review board of the Inje University Haeundae Paik Hospital and individual consent for this retrospective analysis was waived.
Patient-selection criteria
A total of 175 patients diagnosed with biopsy-confirmed PCa between August 2018 and September 2020 were recruited for the study. Among them, 98 patients meeting the following inclusion criteria were selected: (I) radical prostatectomy; (II) 3T prostate MRI scan including DCE-sequence, and (III) pathologic report of GS. Among these patients, 2 who had undergone prostate MRI on a different scanner were excluded, as were another 2 due to failure of image transmission to the dedicated perfusion software. Finally, then, 94 patients (mean age: 68.7 years, range, 47–83 years) were included in the analysis (Figure 1).
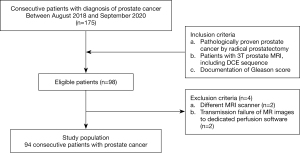
MRI acquisition
All MRI examinations had been performed with a single 3.0-T MRI machine (Achieva TX; Philips Medical imaging, Best, Netherlands) with a body-parallel array coil (SENSE Torso/cardiac coil; USA Instruments, Gainesville, FL, USA). MRI sequences consisted of essential T2-weighted images (T2WI) in three planes and diffusion weighted images (b values of 0, 100, 1,000, 2,000 s/mm2). For DCE sequences, axial pre- and post-contrast T1-spoilled gradient echo [i.e., fast-field echo (FFE)] sequences were performed. For T1 mapping, dual flip angles (5, 15 degrees) were used on pre-contrast T1 sequence. For contrast enhancement, 0.1 mmol/kg or 0.2 mL/kg of contrast agent (meglumine gadoterate, Dotarem™, Guerbet, France) was injected through the antecubital vein at the rate of 3.0 mL/s followed by 30 mL normal saline flushing at the same injection rate. The temporal resolution of the T1-FFE sequence was 3.6 s, and 70 repetitive dynamic images affording full coverage of the prostate gland were acquired. The details of the MRI protocols are provided in Table 1.
Table 1
Parameter | T2-weighted axial/coronal/sagittal | DWI axial | Pre-contrast T1 FFE axial | Dynamic contrast enhanced T1 FFE axial | Post-contrast T1 SPIR axial |
---|---|---|---|---|---|
TR (msec) | 3,370.7/3,600.7/2,908.5 | 5,725 | 10.0 | 3.5 | 667.3 |
TE (msec) | 100/80/90 | 73 | 1.6 | 1.2 | 10.0 |
Slice thickness (mm) | 3 | 3 | 4 | 4 | 3 |
Interslice gap (mm) | 0.3 | 0.3 | 0 | 0 | 0.3 |
Matrix size | 316×272/308×272/316×255 (respectively) | 120×118 | 216×166 | 216×166 | 308×266 |
Flip angle (degree) | – | – | 5/15 | 8 | – |
FOV (mm × mm) | 220×220 | 240×240 | 300×300 | 300×300 | 220×220 |
b-values (s/mm2) | – | 0, 100, 1,000, 2,000 | – | – | – |
Number of slices | 30/30/26 | 30 | 18 | 18 | 30 |
Acquisition time (minute/second) | 2 min 15 sec/2 min 24 sec/2 min 25 sec | 7 min 9 sec | 10 sec/11 sec | 4 min 13 sec | 2 min 26 sec |
DWI, diffusion-weighted images; SPIR, spectral presaturation with inversion recovery; FFE, fast-field echo; TR, repetition time; TE, echo time; FOV; field of view.
Measurements of perfusion parameters
The acquired MR images from the DCE sequences were initially arranged in a picture archiving and communication system (PACS) (m-view; INFINITT healthcare, Seoul, Korea). Then, the arranged DICOM images were transported to a workstation (IntelliSpace Portal, version 10.0, Philips, Best, Netherlands) equipped with dedicated perfusion software (MR permeability) that performs automated quantitation of the perfusion parameters of measured regions of interests (ROIs) under an extended Tofts model (14). The volume transfer constant, Ktrans, was defined as influx from blood plasma to the extracellular extravascular space (EES). The rate constant kep was defined as efflux from the EES to the blood plasma. The volume fractions of EES and blood plasma were represented as ve and vp, respectively (14). In addition, area of ROI was automatically calculated. The area of ROI was defined as an average of calculated areas of tumor on each tumor bearing slice. The MR permeability software used a population-based model arterial input function (AIF) which used a medium bi-exponential model that was pre-selected according to the user selective injection duration as follows; short (less than 5 s), medium (between 5 and 10 s) and long (longer than 10 s).
On the PACS workstation, two radiologists (with 17 and 4 years’ experience, respectively) blinded to the GS of each PCa reviewed T2WI, diffusion-weighted images (DWI) and corresponding apparent diffusion coefficient (ADC) maps, and recorded the Prostate Imaging-Reporting and Data system (PI-RADS) score by consensus based on PI-RADS v.2.0 (15). After referencing the topographic map, the zonal location and margin of tumor also were determined by consensus for each slice-bearing tumor. Two radiologists with 17 and 4 years’ experience independently drew free-hand ROIs along the tumor border on the DCE-images. In cases of multiple lesions in one patient, an index tumor which was defined as the largest tumor on the topographic map was selected. After tumor segmentation, the perfusion parameters on each slice were averaged to obtain a representative value for the whole-tumor volume.
Histology
Experienced urologists had conducted all of the radical prostatectomies. After the surgery, the excised prostate gland including the PCa was fixed in formalin and embedded in paraffin. Then, a serial section was acquired from the base to the apex of the prostate gland, followed by preparation of slides with hematoxylin and eosin staining. A dedicated pathologist interpreted each slide and determined the GS of the PCa (16). The reference standard for tumor segmentation on MR images was assessed according to the topographic maps of the prepared slides.
Statistical analysis
T-testing was performed to analyze the differences of the perfusion parameters between the CSC and non-CSC groups. To evaluate the diagnostic performance of the perfusion parameters for discriminating CSC from non-CSC, a receiver operating characteristic (ROC) curve analysis by pair-wise comparison was performed, and the areas under the curves (AUCs) were calculated. Diagnostic predictive values (i.e., accuracy, sensitivity, and specificity) were evaluated following determination of the most appropriate cutoff value. Inter-rater variability for measurements of perfusion parameters was assessed by the intra-class correlation coefficients (ICCs, poor; <0.2, fair; 0.21–0.60, good; 0.61–0.80, excellent; >0.81). P values <0.05 were considered to indicate significant differences. All of the statistical analyses were performed using MedCalc 19.6.1 (MedCalc, Mariakerke, Belgium).
Results
Patient demographics
The study population was comprised of CSC (GS 7, n=57; GS 8, n=9; GS 9, n=11) and non-CSC (GS 6, n=17) groups. The average time interval between MRI and surgery was 31.4 days (range, 5–83 days). Their respective demographics are provided in Table 2.
Table 2
Parameter | Total | CSC (n=77) | Non-CSC (n=17) | P value |
---|---|---|---|---|
Mean PSA (range), ng/mL | 21.51 (0.02–149) | 24.51 (0.85–149) | 7.95 (0.02–20.4) | 0.0001 |
Tumor location, n [%] | ||||
Peripheral zone | 48 [51] | 37 [48] | 11 [65] | |
Transitional zone | 30 [32] | 24 [31] | 6 [35] | |
Diffuse | 16 [17] | 16 [21] | 0 | |
PI-RADS v2, n [%] | ||||
3 | 9 [10] | 3 [4] | 6 [35] | |
4 | 32 [34] | 26 [34] | 6 [35] | |
5 | 53 [56] | 48 [62] | 5 [29] |
CSC, clinically significant cancer; PI-RADS v2, Prostate Imaging Reporting and Data System version 2; PSA, prostate-specific antigen.
Comparison of perfusion parameters between CSC and non-CSC groups
Among the retrieved parameters, ve showed a statistically significant difference between the CSC (0.238±0.095) and non-CSC groups (0.300±0.126) (P=0.0308) (Figures 2,3). On subgroup analysis, ve showed a difference between GS 6 (0.300±0.126) and GS 7 (0.240±0.097) (P=0.0489). Area of ROI was also different between CSC and non-CSC (201.89±163.87 and 84.99±85.82 mm2, respectively) (P=0.0054). Further analysis according to PIRADS score revealed that the areas of ROI were significantly different from one another (for PIRADS 3, 45.92±31.94; for PIRADS 4, 97.21±50.35; for PIRADS 5, 254.08±174.87, all P<0.0001). The detailed results for all of the perfusion parameters are summarized in Table 3.
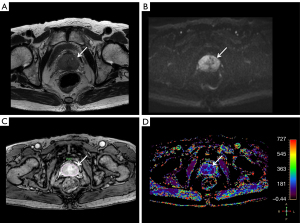
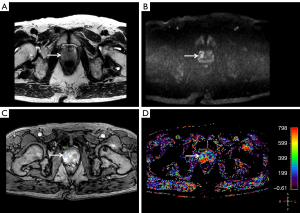
Table 3
Parameter | CSC (n=77) | Non-CSC (n=17) | P value |
---|---|---|---|
Ktrans (min−1) | 0.074±0.030 | 0.076±0.025 | 0.7682 |
kep (min−1) | 0.345±0.140 | 0.297±0.127 | 0.2003 |
ve | 0.238±0.095 | 0.300±0.126 | 0.0308 |
vp | 0.071±0.048 | 0.059±0.046 | 0.3234 |
Area of ROI (mm2) | 201.89±163.87 | 84.99±85.82 | 0.0054 |
Data are mean ± standard deviation. CSC, clinically significant cancer; Ktrans, volume transfer constant; kep, rate constant; ve, extracellular extravascular space volume fraction; vp, fractional volume of plasma; ROI, region of interest.
Diagnostic Performance of perfusion parameters for differentiating CSC from non-CSC
The AUC of area of ROI was 0.807 (95% CI, 0.713–0.881), with a maximum accuracy of 81%, a sensitivity of 82%, and a specificity of 76% under a cutoff value of >90.09 mm2.
The AUC of ve was 0.643 (95% CI, 0.538–0.739), with a maximum accuracy of 64%, a sensitivity of 66%, and a specificity of 53% under a cutoff value of ≤0.262.
None of the other perfusion parameters showed significant differences. Their AUC values were as follows: Ktrans, 0.539 (95% CI, 0.433–0.643); kep, 0.597 (95% CI, 0.491–0.697); vp, 0.594 (95% CI, 0.487–0.694) (Table 4, Figure 4).
Table 4
Parameter | AUC (95% CI) | P value |
---|---|---|
Ktrans | 0.539 (0.433–0.643) | 0.5853 |
kep | 0.597 (0.491–0.697) | 0.2275 |
ve | 0.643 (0.538–0.739) | 0.0458 |
vp | 0.594 (0.487–0.694) | 0.2325 |
Area of ROI | 0.807 (0.713–0.881) | <0.0001 |
CSC, clinically significant cancer; AUC, area under curve; CI, confidence interval; Ktrans, volume transfer constant; kep, rate constant; ve, extracellular extravascular space volume fraction; vp, fractional volume of plasma; ROI, region of interest.
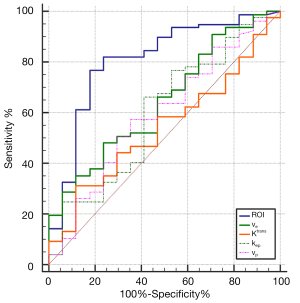
Inter-rater variability
Regarding inter-rater variability, ICC of each parameter was 0.90 (95% CI, 0.857–0.933) for area of ROI, 0.834 (95% CI, 0.760–0.888) for ve, 0.939 (95% CI, 0.913–0.959) for Ktrans, 0.920 (95% CI, 0.885–0.946) for kep, and 0.886 (95% CI, 0.823–0.926) for vp, which indicated excellent inter-rater agreements.
Discussion
Our observations revealed that area of ROI and ve were significant parameters in discriminating CSC from non-CSC. Regarding area of ROI, our results correspond with previous studies (17-19). Among many prognostic factors, it is well known that as tumor volume increases, so too does the pathologic GS, and that the disease tends to progress even after radical prostatectomy (20). Friedersdorff et al. reported that tumor volume showed a significant difference between CSC (n=131) and non-CSC (n=65), with a P value <0.0001, and also that a positive correlation existed between GS and tumor volume (Pearson correlation coefficient R; 0.385, P<0.0001) (17). Donati et al. observed that derived tumor volume on ADC maps was an independent predictor of GS of PCa. They also observed a positive correlation between GS and tumor volume, with correlation coefficients of 0.453 (GS 6), 0.643 (GS 7), and 0.980 (GS ≥8), respectively (18). Verma et al. found a positive correlation between Gleason grade and tumor volume as measured on ADC maps (R; 0.39, P<0.05) or T2WI images (R; 0.40, P<0.05) (19).
In terms of ve, it is generally accepted that as the tumor goes through angiogenesis with the help of soluble factors such as vascular endothelial growth factor, vascular permeability significantly increases, and thus the tumor has a larger interstitial space than does a normal gland. Beyond the scope of differentiating PCa from normal glands, we postulate that as GS increases, the effective interstitial space for perfusion decreases. From the radiological perspective, there is supporting evidence for decreased interstitial space in cases of higher GS in the literature. Previous studies by Verma et al. observed that a negative correlation existed between ADC values and post-surgical Gleason grade (R; −0.26, P<0.05) (19). In addition, Park et al. reported that ADC values were negatively correlated with GS (R; −0.3240, P=0.0016) (21). It is thought that Brownian movement of water molecules in tumor tissue is influenced by tissue architecture such as cell density, and that decreases in ADC values of tumor tissue represents increased cell density (22). Consequently, ve as a perfusion parameter, specifically the EES volume fraction, might play a role in discriminating GS ≥7 from GS 6. To our knowledge, previous observations that ve could help in differentiating CSC from non-CSC are scarce in the literature (23). From the viewpoint of pathology, previous observations are that whereas Gleason grade 5 showed a disorganized gland structure, Gleason grade 4 showed a higher cellular density and less stromal space than Gleason grade 3 (16,24,25). Therefore, GS 7 (3+4 or 4+3) could show less interstitial space compared with GS 6 (3+3), which in fact was observed in the present study as a decrease in the fractional volume of interstitial space.
Another important observation of the current study was that the parameter Ktrans did not show any difference in discrimination of CSC from non-CSC. By contrast, Ktrans demonstrated promising results in previous studies. Wei et al. observed that diagnostic performance of Ktrans (AUC, 0.736; P<0.001) showed a better outcome for PCa than did ve or vp under the extended Tofts model (10). Afshari Mirak et al. also found that Ktrans was higher in CSC than in non-CSC (0.43, 0.32, respectively, P=0.005) (9). Meanwhile, Vos et al. reported that 75th percentile Ktrans could discriminate CSC from non-CSC, especially in the peripheral zone, but that mean Ktrans was not statistically significant (AUC, 0.68; P=0.06) (11). In our opinion, this difference could be attributable to the discrepancy between the pertinent study populations; in any case, the efficacy of Ktrans for stratification of PCa aggressiveness remains controversial. Further verification in a much larger study population is needed.
This study has several limitations. First, instead of individual-based AIF, the population-based model AIF was adopted for the perfusion software, which could not reflect individual physiologic variations such as cardiac output and significant vascular stenosis. However, at present, the population-based model AIF is a generally accepted tool for assessing permeability in major MR vendors. It uses a bi-exponential model which makes the model suitable for any temporal resolution and does not incorporate recirculation effects after the first bolus passage. Second, there is the inherent limitation of the wide inter-observer variability of perfusion parameters from different perfusion analysis solutions. It has been reported that the range of variability could be as wide as 20% (26). Therefore, we made every effort to perform DCE-MRI scans in the same MRI scanner and with uniform acquisition parameters as well as the same perfusion analysis software. Our results revealed that ICCs of all perfusion parameters were within a range of 0.83–0.94, which indicated excellent inter-rater agreements. This observation corresponds well with a previous study (27). The measurement of the whole tumor volume is known to be more reproducible than those on single representative slice or small solid portion of the tumor (27).
In conclusion, size of the tumor and interstitial space volume fraction are significant parameters for discrimination of CSC from non-CSC.
Acknowledgments
We appreciate John Hare for his English editing.
Funding: This work was supported by the GUERBET research grant (GK-DTR-2021-48).
Footnote
Reporting Checklist: The authors have completed the STARD reporting checklist. Available at https://dx.doi.org/10.21037/qims-21-455
Conflicts of Interest: All authors have completed the ICMJE uniform disclosure form (available at https://dx.doi.org/10.21037/qims-21-455). SHK serves as an unpaid editorial board member of Quantitative Imaging in Medicine and Surgery, and reports that this work was supported by the GUERBET research grant (GK-DTR-2021-48). The other authors have no conflicts of interest to declare.
Ethical Statement: The authors are accountable for all aspects of the work in ensuring that questions related to the accuracy or integrity of any part of the work are appropriately investigated and resolved. The study was conducted in accordance with the Declaration of Helsinki (as revised in 2013). This retrospective study was approved by the institutional review board of the Inje University Haeundae Paik Hospital and individual consent for this retrospective analysis was waived.
Open Access Statement: This is an Open Access article distributed in accordance with the Creative Commons Attribution-NonCommercial-NoDerivs 4.0 International License (CC BY-NC-ND 4.0), which permits the non-commercial replication and distribution of the article with the strict proviso that no changes or edits are made and the original work is properly cited (including links to both the formal publication through the relevant DOI and the license). See: https://creativecommons.org/licenses/by-nc-nd/4.0/.
References
- Heidenreich A, Bastian PJ, Bellmunt J, Bolla M, Joniau S, van der Kwast T, Mason M, Matveev V, Wiegel T, Zattoni F, Mottet NEuropean Association of Urology. EAU guidelines on prostate cancer. part 1: screening, diagnosis, and local treatment with curative intent-update 2013. Eur Urol 2014;65:124-37. [Crossref] [PubMed]
- Heidenreich A, Bastian PJ, Bellmunt J, Bolla M, Joniau S, van der Kwast T, Mason M, Matveev V, Wiegel T, Zattoni F, Mottet NEuropean Association of Urology. EAU guidelines on prostate cancer. Part II: Treatment of advanced, relapsing, and castration-resistant prostate cancer. Eur Urol 2014;65:467-79. [Crossref] [PubMed]
- Epstein JI. An update of the Gleason grading system. J Urol 2010;183:433-40. [Crossref] [PubMed]
- Corcoran NM, Hong MK, Casey RG, Hurtado-Coll A, Peters J, Harewood L, Goldenberg SL, Hovens CM, Costello AJ, Gleave ME. Upgrade in Gleason score between prostate biopsies and pathology following radical prostatectomy significantly impacts upon the risk of biochemical recurrence. BJU Int 2011;108:E202-10. [Crossref] [PubMed]
- Cohen MS, Hanley RS, Kurteva T, Ruthazer R, Silverman ML, Sorcini A, Hamawy K, Roth RA, Tuerk I, Libertino JA. Comparing the Gleason prostate biopsy and Gleason prostatectomy grading system: the Lahey Clinic Medical Center experience and an international meta-analysis. Eur Urol 2008;54:371-81. [Crossref] [PubMed]
- Borghesi M, Ahmed H, Nam R, Schaeffer E, Schiavina R, Taneja S, Weidner W, Loeb S. Complications After Systematic, Random, and Image-guided Prostate Biopsy. Eur Urol 2017;71:353-65. [Crossref] [PubMed]
- van Moorselaar RJ, Voest EE. Angiogenesis in prostate cancer: its role in disease progression and possible therapeutic approaches. Mol Cell Endocrinol 2002;197:239-50. [Crossref] [PubMed]
- Alonzi R, Padhani AR, Allen C. Dynamic contrast enhanced MRI in prostate cancer. Eur J Radiol 2007;63:335-50. [Crossref] [PubMed]
- Afshari Mirak S, Mohammadian Bajgiran A, Sung K, Asvadi NH, Markovic D, Felker ER, Lu D, Sisk A, Reiter RE, Raman SS. Dynamic contrast-enhanced (DCE) MR imaging: the role of qualitative and quantitative parameters for evaluating prostate tumors stratified by Gleason score and PI-RADS v2. Abdom Radiol (NY) 2020;45:2225-34. [Crossref] [PubMed]
- Wei C, Jin B, Szewczyk-Bieda M, Gandy S, Lang S, Zhang Y, Huang Z, Nabi G. Quantitative parameters in dynamic contrast-enhanced magnetic resonance imaging for the detection and characterization of prostate cancer. Oncotarget 2018;9:15997-6007. [Crossref] [PubMed]
- Vos EK, Litjens GJ, Kobus T, Hambrock T, Hulsbergen-van de Kaa CA, Barentsz JO, Huisman HJ, Scheenen TW. Assessment of prostate cancer aggressiveness using dynamic contrast-enhanced magnetic resonance imaging at 3 T. Eur Urol 2013;64:448-55. [Crossref] [PubMed]
- Chen YJ, Chu WC, Pu YS, Chueh SC, Shun CT, Tseng WY. Washout gradient in dynamic contrast-enhanced MRI is associated with tumor aggressiveness of prostate cancer. J Magn Reson Imaging 2012;36:912-9. [Crossref] [PubMed]
- Sureka B, Elhence P, Khera PS, Choudhary GR, Pandey H, Garg PK, Yadav K, Goel A. Quantitative contrast-enhanced perfusion kinetics in multiparametric MRI in differentiating prostate cancer from chronic prostatitis: results from a pilot study. Br J Radiol 2019;92:20190181 [Crossref] [PubMed]
- Tofts PS. Modeling tracer kinetics in dynamic Gd-DTPA MR imaging. J Magn Reson Imaging 1997;7:91-101. [Crossref] [PubMed]
- Park SY, Jung DC, Oh YT, Cho NH, Choi YD, Rha KH, Hong SJ, Han K. Prostate Cancer: PI-RADS Version 2 Helps Preoperatively Predict Clinically Significant Cancers. Radiology 2016;280:108-16. [Crossref] [PubMed]
- Epstein JI, Allsbrook WC Jr, Amin MB, Egevad LLISUP Grading Committee. The 2005 International Society of Urological Pathology (ISUP) Consensus Conference on Gleason Grading of Prostatic Carcinoma. Am J Surg Pathol 2005;29:1228-42. [Crossref] [PubMed]
- Friedersdorff F, Groß B, Maxeiner A, Jung K, Miller K, Stephan C, Busch J, Kilic E. Does the Prostate Health Index Depend on Tumor Volume?-A Study on 196 Patients after Radical Prostatectomy. Int J Mol Sci 2017;18:488. [Crossref] [PubMed]
- Donati OF, Afaq A, Vargas HA, Mazaheri Y, Zheng J, Moskowitz CS, Hricak H, Akin O. Prostate MRI: evaluating tumor volume and apparent diffusion coefficient as surrogate biomarkers for predicting tumor Gleason score. Clin Cancer Res 2014;20:3705-11. [Crossref] [PubMed]
- Verma S, Rajesh A, Morales H, Lemen L, Bills G, Delworth M, Gaitonde K, Ying J, Samartunga R, Lamba M. Assessment of aggressiveness of prostate cancer: correlation of apparent diffusion coefficient with histologic grade after radical prostatectomy. AJR Am J Roentgenol 2011;196:374-81. [Crossref] [PubMed]
- Eichelberger LE, Koch MO, Daggy JK, Ulbright TM, Eble JN, Cheng L. Predicting tumor volume in radical prostatectomy specimens from patients with prostate cancer. Am J Clin Pathol 2003;120:386-91. [Crossref] [PubMed]
- Park H, Kim SH, Lee Y, Son JH. Comparison of diagnostic performance between diffusion kurtosis imaging parameters and mono-exponential ADC for determination of clinically significant cancer in patients with prostate cancer. Abdom Radiol (NY) 2020;45:4235-43. [Crossref] [PubMed]
- Tamada T, Sone T, Jo Y, Toshimitsu S, Yamashita T, Yamamoto A, Tanimoto D, Ito K. Apparent diffusion coefficient values in peripheral and transition zones of the prostate: comparison between normal and malignant prostatic tissues and correlation with histologic grade. J Magn Reson Imaging 2008;28:720-6. [Crossref] [PubMed]
- Winkel DJ, Breit HC, Shi B, Boll DT, Seifert HH, Wetterauer C. Predicting clinically significant prostate cancer from quantitative image features including compressed sensing radial MRI of prostate perfusion using machine learning: comparison with PI-RADS v2 assessment scores. Quant Imaging Med Surg 2020;10:808-23. [Crossref] [PubMed]
- Epstein JI, Egevad L, Amin MB, Delahunt B, Srigley JR, Humphrey PA. Grading Committee. The 2014 International Society of Urological Pathology (ISUP) Consensus Conference on Gleason Grading of Prostatic Carcinoma: Definition of Grading Patterns and Proposal for a New Grading System. Am J Surg Pathol 2016;40:244-52. [Crossref] [PubMed]
- Pierorazio PM, Walsh PC, Partin AW, Epstein JI. Prognostic Gleason grade grouping: data based on the modified Gleason scoring system. BJU Int 2013;111:753-60. [Crossref] [PubMed]
- Heye T, Davenport MS, Horvath JJ, Feuerlein S, Breault SR, Bashir MR, Merkle EM, Boll DT. Reproducibility of dynamic contrast-enhanced MR imaging. Part I. Perfusion characteristics in the female pelvis by using multiple computer-aided diagnosis perfusion analysis solutions. Radiology 2013;266:801-11. [Crossref] [PubMed]
- Lambregts DM, Beets GL, Maas M, Curvo-Semedo L, Kessels AG, Thywissen T, Beets-Tan RG. Tumour ADC measurements in rectal cancer: effect of ROI methods on ADC values and interobserver variability. Eur Radiol 2011;21:2567-74. [Crossref] [PubMed]