Incorporating the clinical and radiomics features of contrast-enhanced mammography to classify breast lesions: a retrospective study
Introduction
Contrast-enhanced mammography (CEM) (1) is a breast imaging technique that can facilitate the visualization of breast lesions by depicting tumor neo-angiogenesis through intravenous administration of iodinated contrast material (2-5). In 2011, the United States Food and Drug Administration approved CEM for clinical use as an adjunct to mammography or ultrasound in the diagnostic setting (6,7). For this technique, a set of low-energy (LE), high-energy (HE) and dual-energy subtraction (DES) images are produced for each craniocaudal (CC) or mediolateral oblique (MLO) view (6).
In the diagnostic setting, the pooled sensitivity of CEM is reported to be as high as 89–97%, whereas its pooled specificity is not that satisfactory, approximately 66–84% (8,9). Several studies aimed to seek new ways to improve the diagnostic efficacy of CEM, including establishing computer-aided diagnostic systems (10-12), combining the radiomics features of CEM and digital breast tomosynthesis (13), or incorporating the Breast Imaging Reporting and Data System (BI-RADS) descriptors of CEM images into neural networks (14). These studies have shown promising prospects for improving the classification of breast lesions.
Some studies (15-17) have indicated that assessing the clinical image features of CEM by using the BI-RADS lexicons can help differentiate breast lesions. Meanwhile, radiomics is progressing rapidly (18) and radiomics analysis allows for digital decoding of medical images into quantitative features and mining of the underlying information contained therein (19,20). However, no study so far has focused on combining the clinical image features and radiomics features of CEM for diagnosing breast lesions. Only one study (21) integrated the radiomics signature and CEM-reported lymph node status to predict axillary lymph node metastasis in breast cancer.
In our study, we aimed to explore whether better classification results for breast lesions could be achieved by combining the clinical image features interpreted by the radiologists and the radiomics features of CEM images than by using either set of the features alone.
Methods
Study population
We retrospectively collected data from consecutive female patients who underwent CEM at two research centers between August 2017 and February 2020. The Institutional Review Board of each center approved this study. The patient written informed consent was waived for this retrospective analysis. The study was conducted in accordance with the Declaration of Helsinki (as revised in 2013). None of the patients had any contraindications to CEM examination.
The inclusion criteria were as follows: (I) patients with suspected breast lesions after physical examination or ultrasound and (II) patients who were referred for CEM as part of diagnostic imaging. The exclusion criteria were as follows: (I) patients lacking histopathological confirmation of the final diagnoses of the suspicious lesions; (II) patients with missing data; (III) patients with a history of breast surgery, breast radiotherapy, chemotherapy or hormone treatment within 1 year prior to CEM examination; (IV) patients with poor image quality; and (V) patients with no lesions detected on any CEM image (Figure 1).
CEM examination
All CEM examinations in both research centers were performed using Senographe Essential mammography units (GE Healthcare, Buc, France) and followed the same examination protocol (Figure 2). In brief, all patients received intravenous injections of iodinated contrast material (Iohexol, 300–350 mg I/mL; Beilu Pharmaceutical Co., Ltd., Beijing, China) at a dose of 1.5 mL/kg and a rate of 3 mL/s. Two minutes after the start of injection, a pair of HE and LE images were obtained consecutively during a single breast compression. Image acquisition was performed first from the CC view and then the MLO view of the suspicious breast, followed by the CC view and the MLO view of the less suspicious breast. Afterwards, the HE and LE images were automatically recombined to generate the DES images. No severe allergic reaction to the contrast material was found in this study.
CEM image interpretation by the radiologists
All of the CEM images were reviewed and interpreted by two independent radiologists with 5 years of experience interpreting CEM images to obtain the clinical CEM features. The radiologists were blinded to the medical history and histopathological results of the patients. In the case of a discrepancy, the final decision was made in consensus.
Because there are no standardized criteria for CEM image interpretation at present (6,16), the images were interpreted according to the 5th edition of the BI-RADS Atlas (22). Since LE images appear similar to conventional mammography (23,24), the BI-RADS Mammography lexicon was consulted to evaluate the LE images with respect to the following information: breast composition (a, b, c or d), type of suspicious lesions (mass, calcification, asymmetry, or architectural distortion), presence or absence of suspicious skin/nipple findings or suspicious axillary adenopathy. The DES images were analyzed in accordance with the BI-RADS MRI lexicon concerning the type of enhancing lesions (focal, mass or nonmass), internal enhancement pattern (homogeneous, heterogeneous, rim enhancement, internal septations, clumped or clustered ring), and degree of background parenchymal enhancement (minimal, mild, moderate or marked). The degree of lesion enhancement (no, slight, moderate or intense enhancement) was also evaluated. Furthermore, the largest diameters of the lesions were measured independently by two radiologists with 1 year of experience interpreting CEM images. The mean values of the lesion diameters were considered as the final lesion diameters. The age of the patient was also analyzed as a clinical feature since it is a generally accepted risk factor for breast cancer. The list of clinical features is shown in Table S1.
Reference standards
The standard of reference was the histopathologic diagnosis obtained by biopsy or surgery within 2 weeks after CEM examination. Benign cases were defined as lesions that did not contain any carcinoma in situ or invasive components. Malignant cases were defined as lesions that contain any invasive components or ductal carcinoma in situ.
Lesion delineation and feature extraction
The contours of the lesions were manually delineated with ITK-SNAP (version 3.6; www.itksnap.org) (25) by one radiologist with 1 year of experience interpreting CEM images. Another senior radiologist with 8 years of experience interpreting CEM images reviewed all the lesion contours and made necessary modification. The two radiologists were not informed of the histopathological results of the lesions. For each lesion, a total of 6 regions-of-interests (ROIs) were delineated on the HE, LE and DES images in the CC and MLO views. The lesion delineation criteria are listed in Table 1. Two weeks later, the two radiologists randomly selected 30 patients and repeated the segmentation procedure to assess reproducibility of manual segmentation by using the intraclass correlation coefficient (ICC) (25). The features with ICCs greater than 0.75 were considered to indicate good agreement and were kept in the datasets for the radiomics feature selection step.
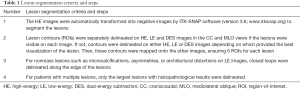
Full table
Before feature extraction, gray-level discretization was performed to discretize all the images to 256 gray levels. The image resampling step was omitted because the voxels were isotropic in-plane. Analysis Kit software (version 3.2.0; GE healthcare) (26,27) was used to extract the radiomics features. For each ROI, a total of 387 features, including 42 histogram features and 345 texture features, were obtained (Table S2).
Radiomics feature selection
The data from Center 1 were used as training set to select features, train the models, and perform internal validation. The data from Center 2 were used as testing set (2:1) to perform external testing and visualize receiver operating characteristic (ROC) curves.
For radiomics feature selection, a 3-step selection strategy (20) was utilized to avoid model overfitting and potential bias in the prediction outcome. First, the Mann-Whitney U test was used to compare the values of all the features between the benign and malignant groups, and to preliminarily screen the features which were related to distinguishing the benign and malignant lesions. All features were ranked by the P value from the Mann-Whiney U test in increasing order, and the top 800 (approximately 30%) were selected for the next step. Second, the Pearson correlation coefficient between each pair of features (r) was calculated as a selection tool to remove highly correlated features, which may share similar information for prediction. If a pair of features with |r|>0.85 was found, the feature with the higher P value was removed. Third, all remaining features were further selected by utilizing Boruta’s approach, which is based on the Random Forest (RF) algorithm. Boruta’s approach performs a top-down search by comparing the importance of original attributes with randomly achievable importance (28) and is recommended for the analysis of high-dimensional datasets (29). Features with significantly better performance are considered ‘Confirmed’. This step was to help select the features which were important in building a classification model. All the ‘Confirmed’ features from Boruta’s approach were used as the final selected features.
Statistical analysis
All statistical analyses were performed using R software (version 3.6.3; www.r-project.org). The Mann-Whitney U test was used to compare continuous features between the benign and malignant groups, and the chi-square test (or Fisher’s exact test if any cell count was less than 5) was used to compare categorical features. The final selected radiomics features and all clinical features were used to build a radiomics RF model and a clinical RF model, respectively. The RF models (30) were built by using the randomForest package with all default settings except for ntree =1,000. Following the approach of Breiman (30), the Mean Decrease Accuracy (MDA) and Mean Decrease Gini (MDG) coefficient (31), were used to rank the importance of the features from the RF algorithm results. Then, a combined logistic regression model was built by including the predicted probabilities from both RF models. The area under the ROC curve (AUC) and 95% confidence interval (CI) were calculated to compare the performance of each model run with the testing set. The ROC curve plot was used to visualize the results, and error bars which represented 95% CI of the combined models’ sensitivities and specificities were provided when needed. The DeLong test (32) was used to compare different AUCs. The accuracy, sensitivity and specificity of the models for the testing set were also calculated by selecting the optimal threshold based on Youden index.
To further compare the performance and stability of the models, an internal validation was performed by randomly splitting the training set into internal-training and internal-validation sets with the ratio of 2:1 for 100 iterations. Since our goal was to compare the performance, we used Monte Carlo cross-validation with re-training in the analysis to ensure more confidence at cost of an acceptable small bias (33). The mean AUCs were then calculated to compare the performance of the models. The Kruskal-Wallis test was used to compare overall differences among the mean AUCs, and the Mann-Whitney U test was used to make pairwise comparisons between AUC pairs of interest. Bonferroni correction was used for multiple comparisons. A P value less than 0.050 was considered to be statistically significant in this study.
Results
Patient characteristics and clinical features of CEM
The patient characteristics and clinical features from their CEM images are given in Table 2. Based on the inclusion criteria, a total of 334 patients were included in the study. As shown in Figure 1, after excluding 108 patients amongst whom 81 lacked histopathological results, a total of 226 patients with 226 lesions (benign lesions: 101/226, 44.7%; malignant lesions: 125/226, 55.3%) were included in the following analysis. The mean age of all the patients was 47.4±10.1 years (range, 22–70 years).
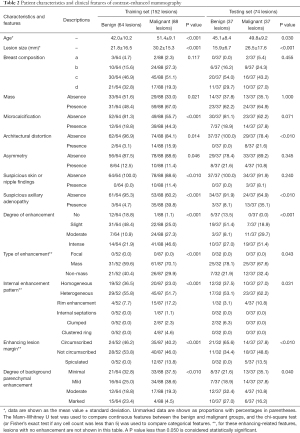
Full table
Radiomics feature selection
The intra-observer ICC range was 0.802 to 0.934 for radiomics features extracted twice by the same radiologist. The inter-observer ICC of feature extraction obtained by the two radiologists ranged from 0.789 to 0.910. The results showed good reproducibility of radiomics feature extraction. The results of the radiomics feature selection using Boruta’s approach is shown in Figure 3 and Table S3. A total of 41 radiomics features (shown as green boxes) were selected to construct the radiomics RF model.
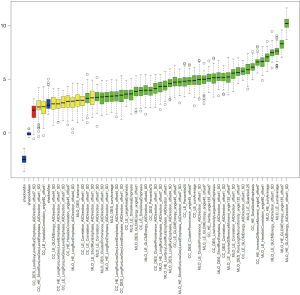
Model construction
First, all of the clinical CEM features and the 41 selected radiomics features were used to construct the binary (benign vs. malignant) clinical and radiomics classification models, respectively. The values of MDA and MDG coefficient, which were used to rank the importance of the features from the RF algorithm, are shown in Figure 4A (for the clinical features) and Figure 4B (for the radiomics features). In Figure 4A, the features of age, enhancing lesion margin, mean lesion size, and degree of enhancement are highly ranked in terms of MDA for lesion classification. Of note, these features also rank highly in terms of MDG coefficient (still in the top 5 ranking), which indicates that they may have an important contribution to the classification of breast lesions. Similarly, in Figure 4B, after ranking the selected radiomics features by MDA and MDG coefficient, the top 5 ranked factors were mainly Gray-level Cooccurrence Matrix Energy features and sumAverage features of HE and LE images.
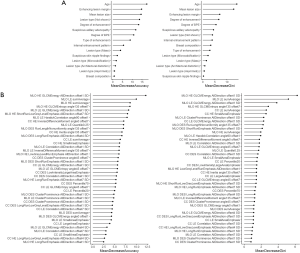
After constructing the clinical and radiomics models, a combined logistic regression model incorporating the clinical and radiomics features of CEM was built. The ROC curves of the clinical, radiomics and combined RF models obtained with the testing set are shown in Figure 5. After incorporating the clinical and radiomics features of CEM, combined model showed an improvement of the classification performance, with an AUC of 0.964 (95% CI: 0.918–1.000), over both the radiomics RF model (AUC =0.947; 95% CI: 0.891–0.997, adjusted P=0.074) and the clinical RF model (AUC =0.882; 95% CI: 0.803–0.962, adjusted P=0.078). The accuracy, sensitivity and specificity of the three models are shown in Table 3. The combined model demonstrated the highest values of the performance measures, with a diagnostic accuracy of 94.6%.


Full table
The mean AUCs of the clinical, radiomics and combined models obtained from the 100 rounds of internal validation process are shown in Figure 6 and Table 4. The combined model still achieved the highest mean AUC of 0.934±0.030, which was significantly higher than that of the radiomics RF model (mean AUC =0.921±0.031; adjusted P<0.001) and that of the clinical RF model (mean AUC =0.907±0.036; adjusted P<0.001).
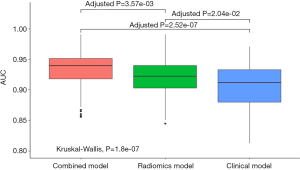

Full table
Discussion
Our results showed that a model incorporating both clinical and radiomics features of CEM can achieve better classification results than model constructed by either clinical or radiomics features.
To the best of our knowledge, this is the first study to combine the BI-RADS lexicon-based clinical image features and radiomics features of CEM for the classification of breast lesions, and the results are encouraging. CEM has shown promising diagnostic value, with reported summary AUCs between 0.93–0.96 (8,34) in two meta-analyses. The sensitivity of CEM has been reported to be quite satisfactory even when interpreted by nonexperienced human readers (35). However, like breast MRI, the specificity of CEM is limited and can be affected by the experience of the readers. Therefore, we sought to explore whether we could further improve the diagnostic performance of CEM by including some objective quantitative indexes, namely, radiomics features, which were extracted by specific computing algorithms.
Several studies have proposed new methods for diagnosing breast lesions using CEM images. Perek et al. (14) sought to improve the diagnostic specificity for breast lesions without compromising sensitivity by constructing multimodal networks that incorporated the BI-RADS descriptors as additional inputs to classification neural networks. The results showed that by combining image features, the performance of the network was improved at a sensitivity of 100% and a specificity of 66%. Similarly, the performance of the radiomics model was also improved after adding the BI-RADS descriptors in our study. Some studies proposed a computer-aided diagnostic system (10-12) in which only radiomics features of CEM were employed to aid in diagnosis, and the resulting AUCs were 0.85–0.95. In our study, the combined CEM model achieved an AUC of 0.964 (95% CI: 0.918–1.000) for the testing set and a mean AUC of 0.934 for the internal validation set, which shows the potential of improving classification performance by adding clinical CEM features to the radiomics models. Our results indicate that different modalities (BI-RADS textural lexicons and pixel-based radiomics features) may complement each other and contribute to a more precise classification. In particular, two computer-aided CEM-based diagnostic systems developed by Fanizzi et al. (10) and Patel et al. (11) showed increases in specificity of at least 8% with respect to the performance of human reader. Consistent with their results, the combined model in our study also demonstrated an increase in specificity compared with that reported in the literature [pooled specificity: 66–84% (8,9)], which could be further improved by incorporating more lesion or patient characteristics in the future. This may provide ideas and evidence for the future application of artificial intelligence-computer aided diagnostic tools for CEM to automatically extract both clinical and radiomics CEM features for the diagnosis of breast lesions.
In our study, the radiologists evaluated the CEM images with the help of the BI-RADS lexicons for mammography and MRI. In fact, BI-RADS assessments have been incorporated in artificial intelligence data as measures of the likelihood of malignancy in breast imaging studies (36). However, in our study, some second-level image features, such as mass shape or calcification morphology, were not incorporated in the clinical model because the sample sizes of some of these feature categories were small, which would have affected the robustness of the models. This may partly explain why the performance of the clinical model in our study was not as high as that previously reported in studies on clinical CEM features. As Kamal et al. (15,16) stated, other morphological and enhancement features can be helpful in differentiating breast lesions. Therefore, further studies with larger sample sizes, including other clinical features, are warranted.
The methods of lesion delineation adopted by different studies on CEM images are not completely consistent. Most studies segmented the lesions in both LE and DES images (21,25,37), while others segmented only in DES images (38,39). In addition, some studies delineated the lesions in LE or DES images and mapped the lesion ROIs to the matched DES or LE images (11,12). In this study, we segmented the lesions in HE, LE and DES images in both CC and MLO views, in an attempt to make the best use of all the image information. Furthermore, we used ICC to evaluate the reproducibility of radiomics feature extraction process. This method was adopted by several studies (21,25,26). Our results showed that the radiomics features demonstrated satisfactory reproducibility of manual segmentation, which was in line with a previous study (40).
Our study had several limitations. First, except for patient age, we did not include other clinical patient characteristics, such as family history, or genomic characteristics, into the clinical or the combined model. By incorporating these features into the models, the performance of both models may be further improved. Second, this is a retrospective study with a relatively small dataset. The small sample size in the testing cohort may have an impact on the validation of the model. We must acknowledge that misclassification of a small number of lesions in the testing set may result in relatively large differences of AUC, accuracy, sensitivity or specificity. Therefore, we performed 100 rounds of internal validation to further confirm our results. Despite the promising results of our study, a larger prospective study is needed to prove the performance of the models in this study. Third, the proportion of patients with malignant breast lesions in this study was higher than that in clinical practice, which may cause potential patient selection bias. However, a balanced dataset is also important in the radiomics analysis, especially in training the classification model. Forth, a manual segmentation method was employed in this study. Although favorable intra- and inter-observer ICCs were obtained, the automated or semi-automated segmentation method may have higher stability and is less time-consuming. Fifth, the modeling process was performed on a single vendor system. The results of the study may not generalize well to other CEM systems.
In conclusion, incorporating the clinical and radiomics features of CEM may achieve better classification results for breast lesions than using clinical or radiomics features alone. Our procedure may form the basis of a new diagnostic scheme in future computer-aided or artificial intelligence realms with CEM or serve as a helpful adjunct for radiologists in interpreting CEM images.
Acknowledgments
The authors are thankful to Boran Pang, MD, for providing technical support and inspiration in experimental design. Permission was obtained from him.
Funding: We also acknowledge funding and support from the Clinical Research Plan of SHDC (SHDC2020CR2008A), the National Natural Science Foundation of China (NSFC 82071878), Shanghai Science and Technology Foundation (19DZ1930502), Shanghai Anticancer Association EYAS PROJECT (SACA-CY20B01), and Shanghai Anticancer Association FLIGHT PROJECT (SACA-AX-201903).
Footnote
Conflicts of Interest: All authors have completed the ICMJE uniform disclosure form (available at https://dx.doi.org/10.21037/qims-21-103). SD is an employee of General Electric (GE) Healthcare (Shanghai, China). The other authors have no conflicts of interest to declare.
Ethical Statement: The authors are accountable for all aspects of the work in ensuring that questions related to the accuracy or integrity of any part of the work are appropriately investigated and resolved. The study was conducted in accordance with the Declaration of Helsinki (as revised in 2013). The Institutional Review Board of each center approved this study. The patient written informed consent was waived for this retrospective analysis.
Open Access Statement: This is an Open Access article distributed in accordance with the Creative Commons Attribution-NonCommercial-NoDerivs 4.0 International License (CC BY-NC-ND 4.0), which permits the non-commercial replication and distribution of the article with the strict proviso that no changes or edits are made and the original work is properly cited (including links to both the formal publication through the relevant DOI and the license). See: https://creativecommons.org/licenses/by-nc-nd/4.0/.
References
- Lewin JM, Isaacs PK, Vance V, Larke FJ. Dual-energy contrast-enhanced digital subtraction mammography: feasibility. Radiology 2003;229:261-8. [Crossref] [PubMed]
- Dromain C, Balleyguier C, Muller S, Mathieu MC, Rochard F, Opolon P, Sigal R. Evaluation of Tumor Angiogenesis of Breast Carcinoma Using Contrast-Enhanced Digital Mammography. AJR Am J Roentgenol 2006;187:W528-37 [Crossref] [PubMed]
- Mori M, Akashi-Tanaka S, Suzuki S, Daniels MI, Watanabe C, Hirose M, Nakamura S. Diagnostic accuracy of contrast-enhanced spectral mammography in comparison to conventional full-field digital mammography in a population of women with dense breasts. Breast Cancer 2017;24:104-10. [Crossref] [PubMed]
- Dromain C, Thibault F, Diekmann F, Fallenberg EM, Jong RA, Koomen M, Hendrick RE, Tardivon A, Toledano A. Dual-energy contrast-enhanced digital mammography: initial clinical results of a multireader, multicase study. Breast Cancer Res 2012;14:R94. [Crossref] [PubMed]
- Lobbes MBI, Smidt ML, Houwers J, Tjan-Heijnen VC, Wildberger JE. Contrast enhanced mammography: Techniques, current results, and potential indications. Clin Radiol 2013;68:935-44. [Crossref] [PubMed]
- Ghaderi KF, Phillips J, Perry H, Lotfi P, Mehta TS. Contrast-enhanced Mammography: Current Applications and Future Directions. Radiographics 2019;39:1907-20. [Crossref] [PubMed]
- Zamora K, Allen E, Hermecz B. Contrast mammography in clinical practice: Current uses and potential diagnostic dilemmas. Clin Imaging 2021;71:126-35. [Crossref] [PubMed]
- Zhu X, Huang JM, Zhang K, Xia LJ, Feng L, Yang P, Zhang MY, Xiao W, Lin HX, Yu YH. Diagnostic Value of Contrast-Enhanced Spectral Mammography for Screening Breast Cancer: Systematic Review and Meta-analysis. Clin Breast Cancer 2018;18:e985-95. [Crossref] [PubMed]
- Xiang W, Rao H, Zhou L. A meta-analysis of contrast-enhanced spectral mammography versus MRI in the diagnosis of breast cancer. Thorac Cancer 2020;11:1423-32. [Crossref] [PubMed]
- Fanizzi A, Losurdo L, Basile TMA, Bellotti R, Bottigli U, Delogu P, Diacono D, Didonna V, Fausto A, Lombardi A, Lorusso V, Massafra R, Tangaro S, La Forgia D. Fully Automated Support System for Diagnosis of Breast Cancer in Contrast-Enhanced Spectral Mammography Images. J Clin Med 2019;8:891. [Crossref] [PubMed]
- Patel BK, Ranjbar S, Wu T, Pockaj BA, Li J, Zhang N, Lobbes M, Zhang B, Mitchell JR. Computer-aided diagnosis of contrast-enhanced spectral mammography: A feasibility study. Eur J Radiol 2018;98:207-13. [Crossref] [PubMed]
- Danala G, Patel B, Aghaei F, Heidari M, Li J, Wu T, Zheng B. Classification of Breast Masses Using a Computer-Aided Diagnosis Scheme of Contrast Enhanced Digital Mammograms. Ann Biomed Eng 2018;46:1419-31. [Crossref] [PubMed]
- Fusco R, Vallone P, Filice S, Granata V, Petrosino T, Rubulotta MR, Setola SV, Maio F, Raiano C, Raiano N, Siani C, Di Bonito M, Sansone M, Botti G, Petrillo A. Radiomic features analysis by digital breast tomosynthesis and contrast-enhanced dual-energy mammography to detect malignant breast lesions. Biomed Signal Proces 2019;53:101568 [Crossref]
- Perek S, Kiryati N, Zimmerman-Moreno G, Sklair-Levy M, Konen E, Mayer A. Classification of contrast-enhanced spectral mammography (CESM) images. Int J Comput Assist Radiol Surg 2019;14:249-57. [Crossref] [PubMed]
- Mohamed Kamal R, Hussien Helal M, Wessam R, Mahmoud Mansour S, Godda I, Alieldin N. Contrast-enhanced spectral mammography: Impact of the qualitative morphology descriptors on the diagnosis of breast lesions. Eur J Radiol 2015;84:1049-55. [Crossref] [PubMed]
- Kamal RM, Helal MH, Mansour SM, Haggag MA, Nada OM, Farahat IG, Alieldin NH. Can we apply the MRI BI-RADS lexicon morphology descriptors on contrast-enhanced spectral mammography? Br J Radiol 2016;89:20160157 [Crossref] [PubMed]
- Knogler T, Homolka P, Hoernig M, Leithner R, Langs G, Waitzbauer M, Pinker K, Leitner S, Helbich TH. Application of BI-RADS Descriptors in Contrast-Enhanced Dual-Energy Mammography: Comparison with MRI. Breast Care (Basel) 2017;12:212-6. [Crossref] [PubMed]
- Gillies RJ, Kinahan PE, Hricak H. Radiomics: Images Are More than Pictures, They Are Data. Radiology 2016;278:563-77. [Crossref] [PubMed]
- Aerts HJWL, Velazquez ER, Leijenaar RTH, Parmar C, Grossmann P, Carvalho S, Bussink J, Monshouwer R, Haibe-Kains B, Rietveld D, Hoebers F, Rietbergen MM, Leemans CR, Dekker A, Quackenbush J, Gillies RJ, Lambin P. Decoding tumour phenotype by noninvasive imaging using a quantitative radiomics approach. Nat Commun 2014;5:4006. [Crossref] [PubMed]
- Liu Z, Wang S, Dong D, Wei J, Fang C, Zhou X, Sun K, Li L, Li B, Wang M, Tian J. The Applications of Radiomics in Precision Diagnosis and Treatment of Oncology: Opportunities and Challenges. Theranostics 2019;9:1303-22. [Crossref] [PubMed]
- Mao N, Yin P, Li Q, Wang Q, Liu M, Ma H, Dong J, Che K, Wang Z, Duan S, Zhang X, Hong N, Xie H. Radiomics nomogram of contrast-enhanced spectral mammography for prediction of axillary lymph node metastasis in breast cancer: a multicenter study. Eur Radiol 2020;30:6732-9. [Crossref] [PubMed]
- D'Orsi CJ, Sickles EA, Mendelson EB, Morris EA. ACR BI-RADS® atlas: breast imaging reporting and data system. Reston, VA: American College of Radiology; 2013.
- Mark A. Francescone, Maxine S. Jochelson, D. David Dershaw, Janice S. Sung, Mary C. Hughes, Junting Zheng, Chaya Moskowitz, Morris EA. Low energy mammogram obtained in contrast-enhanced digital mammography (CEDM) is comparable to routine full-field digital mammography (FFDM). Eur J Radiol 2014;83:1350-5. [Crossref]
- Lalji UC, Jeukens CR, Houben I, Nelemans PJ, van Engen RE, van Wylick E, Beets-Tan RG, Wildberger JE, Paulis LE, Lobbes MB. Evaluation of low-energy contrast-enhanced spectral mammography images by comparing them to full-field digital mammography using EUREF image quality criteria. Eur Radiol 2015;25:2813-20. [Crossref] [PubMed]
- Lin F, Wang Z, Zhang K, Yang P, Ma H, Shi Y, Liu M, Wang Q, Cui J, Mao N, Xie H. Contrast-Enhanced Spectral Mammography-Based Radiomics Nomogram for Identifying Benign and Malignant Breast Lesions of Sub-1 cm. Front Oncol 2020;10:573630 [Crossref] [PubMed]
- Hu X, Ye W, Li Z, Chen C, Cheng S, Lv X, Weng W, Li J, Weng Q, Pang P, Xu M, Chen M, Ji J. Non-invasive evaluation for benign and malignant subcentimeter pulmonary ground-glass nodules (≤1 cm) based on CT texture analysis. Br J Radiol 2020;93:20190762 [Crossref] [PubMed]
- Chu H, Lin X, He J, Pang P, Fan B, Lei P, Guo D, Ye C. Value of MRI Radiomics Based on Enhanced T1WI Images in Prediction of Meningiomas Grade. Acad Radiol 2021;28:687-93. [Crossref] [PubMed]
- Kursa M, Rudnicki W. Feature Selection with Boruta Package. J Stat Softw 2010;36:1-13. [Crossref]
- Degenhardt F, Seifert S, Szymczak S. Evaluation of variable selection methods for random forests and omics data sets. Brief Bioinform 2019;20:492-503. [Crossref] [PubMed]
- Breiman L. Random Forests. Mach Learn 2001;45:5-32. [Crossref]
- Hong H, Xiaoling G, Hua Y. editors. Variable selection using Mean Decrease Accuracy and Mean Decrease Gini based on Random Forest. 2016 7th IEEE International Conference on Software Engineering and Service Science (ICSESS); 2016.
- DeLong ER, DeLong DM, Clarke-Pearson DL. Comparing the areas under two or more correlated receiver operating characteristic curves: a nonparametric approach. Biometrics 1988;44:837-45. [Crossref] [PubMed]
- Burman P. A Comparative Study of Ordinary Cross-Validation, v-Fold Cross-Validation and the Repeated Learning-Testing Methods. Biometrika 1989;76:503-14. [Crossref]
- Tagliafico AS, Bignotti B, Rossi F, Signori A, Sormani MP, Valdora F, Calabrese M, Houssami N. Diagnostic performance of contrast-enhanced spectral mammography: Systematic review and meta-analysis. Breast 2016;28:13-9. [Crossref] [PubMed]
- van Nijnatten TJA, Smidt ML, Goorts B, Samiei S, Houben I, Kok EM, Wildberger JE, Robben SGF, Lobbes MBI. Can high school students help to improve breast radiologists in detecting missed breast cancer lesions on full-field digital mammography? J Cancer 2019;10:765-71. [Crossref] [PubMed]
- Mendelson EB. Artificial Intelligence in Breast Imaging: Potentials and Limitations. AJR Am J Roentgenol 2019;212:293-9. [Crossref] [PubMed]
- La Forgia D, Fanizzi A, Campobasso F, Bellotti R, Didonna V, Lorusso V, Moschetta M, Massafra R, Tamborra P, Tangaro S, Telegrafo M, Pastena MI, Zito A. Radiomic Analysis in Contrast-Enhanced Spectral Mammography for Predicting Breast Cancer Histological Outcome. Diagnostics (Basel) 2020;10:708. [Crossref] [PubMed]
- Marino MA, Pinker K, Leithner D, Sung J, Avendano D, Morris EA, Jochelson M. Contrast-Enhanced Mammography and Radiomics Analysis for Noninvasive Breast Cancer Characterization: Initial Results. Mol Imaging Biol 2020;22:780-7. [Crossref] [PubMed]
- Marino MA, Leithner D, Sung J, Avendano D, Morris EA, Pinker K, Jochelson MS. Radiomics for Tumor Characterization in Breast Cancer Patients: A Feasibility Study Comparing Contrast-Enhanced Mammography and Magnetic Resonance Imaging. Diagnostics (Basel) 2020;10:492. [Crossref] [PubMed]
- Lee M, Woo B, Kuo MD, Jamshidi N, Kim JH. Quality of Radiomic Features in Glioblastoma Multiforme: Impact of Semi-Automated Tumor Segmentation Software. Korean J Radiol 2017;18:498-509. [Crossref] [PubMed]