Concomitant functional impairment and reorganization in the linkage between the cerebellum and default mode network in patients with type 2 diabetes mellitus
Introduction
Type 2 diabetes mellitus (T2DM) is a global epidemic of chronic metabolic disease characterized by hyperglycemia and insulin resistance (1). Cognitive impairment has been gradually recognized as comorbidity and complication of T2DM (2,3), but the specific mechanism is still unclear. The brain is an organ with high metabolic and oxygen demands, a structure and function vulnerable to abnormal glucose metabolism (4). In recent years, resting-state functional magnetic resonance imaging (rs-fMRI) has provided an advanced noninvasive tool for investigating cerebral functional alterations and the neural basis of cognitive impairment in T2DM.
The interactions within the default mode network (DMN) and between the DMN and other brain systems play a critical role in maintaining fundamental cognitive functions (5). In the resting state, the DMN has high basic glucose metabolism and higher aerobic glycolysis than other brain networks. Furthermore, local glucose consumption is related to functional connectivity (FC) within the DMN (6,7). These characteristics make DMN (high-level cognitive network) more vulnerable to injury in T2DM. Compared with healthy controls (HCs), T2DM patients show impaired FC, topological configuration, and structural connectivity within the DMN and between DMN hub regions and other brain regions (8-12). Magnetization transfer imaging revealed that the biophysical integrity of macromolecular protein pools in the DMN hub region (posterior cingulate cortex) was selectively compromised in T2DM and related to diabetic hyperglycemia (13). A recent systematic review found that reduced DMN connectivity was a more consistent outcome in T2DM and that this change was associated with cognitive decline (14). These results suggest that DMN connectivity plays a critical role in the neuropathology of cognitive dysfunction in T2DM.
The cerebellum has traditionally been considered a stable area against the damage of diabetic hyperglycemia (15), but recent neuroimaging studies have challenged this view. Structural MR studies have shown that cerebellar gray matter (GM) is atrophic in patients with T2DM (16-18). Diffusion tensor imaging (DTI) studies have shown that the white matter microstructure of posterior cerebellar and cerebral-cerebellar white matter connections are decreased in T2DM patients (19,20). Furthermore, rs-fMRI studies have identified abnormal low-frequency fluctuation (ALFF) (21-23) and reduced integrity connectivity of the cerebellum (8), as well as wide cerebrum-cerebellum FC (24) in T2DM patients. In addition, our recent study reported that the negative FC between the DMN and the posterior cerebellum was increased in high plasma glucose participants compared to those with normal plasma glucose (25). Consequently, the cerebellum may be a sensitive region for damage by T2DM, and its abnormalities may play a role in abnormal brain circuits.
In recent years, the advanced functions of the cerebellum have gradually attracted the attention of researchers. Animal experiments and human neuroimaging studies have demonstrated that the cerebellum is involved in various cognition, emotion regulation, and reward processes (26,27). Several previous resting-state FC studies have shown that the cerebellum is involved in multiple high-level brain networks. Of these, crus I and lobule IX primarily participate in the DMN and have intrinsic connectivity with cerebral DMN regions (28-32). Recently, the relationship between changes in the resting-state FC pattern of the cerebellum-DMN and cognitive dysfunction in neuropsychiatric diseases has been widely explored (33-35). Although the structure and function of the cerebellum and DMN regions were altered in T2DM patients, the relationship between the cerebellum and DMN abnormalities has remained unclear. Herein, we chose the above crus I and lobule IX as seed regions to investigate alterations of resting-state FC between the cerebellum and DMN regions by seed-based FC analysis. We hypothesized that T2DM patients would show abnormal resting-state FC between the cerebellum and DMN regions.
Methods
Participants
In this study, we recruited 71 participants (34 HCs and 37 T2DM patients) from the Diabetes Clinic and Physical Examination Center of the Third Xiangya Hospital between 2015 and 2017. The inclusion criteria for all participants in this study were as follows: (I) aged 30–70 years; (II) education ≥6 years; (III) right-handedness. The exclusion criteria were as follows: (I) major medical illnesses such as cardiovascular disease, kidney disease, and tumors; (II) self-reported history of alcohol and other substance dependence, brain injury, major depression, or other neuropsychiatric diseases that could affect cognitive function; (III) severe hearing or visual impairment and contraindications for MRI examination; and (IV) Fazekas score of 2 or 3.
Based on the World Health Organization (WHO) diagnostic criteria for diabetes from 1999 (36), all participants were divided into either the T2DM or HC groups. All participants with T2DM received stable hypoglycemic treatment. The therapeutic agents used to treat them are shown in Table 1. Importantly, no participant self-reported having experienced mild or severe hypoglycemia. The study was conducted following the Declaration of Helsinki (as revised in 2013) and was approved by the Ethics Committee of the Third Xiangya Hospital of Central South University. All participants provided informed consent.
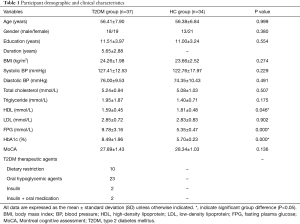
Full table
Procedure and measurements
Demographic data, disease history, and drug use were recorded in detail for each participant. Before MR scanning, blood samples were collected to measure fasting plasma glucose (FPG) levels, glycosylated hemoglobin (HbA1c), and serum lipids through standard laboratory analysis. In addition, we used the Montreal Cognitive Assessment (MoCA) scale to evaluate the global cognitive status of the 2 groups.
Image acquisition
All participants’ rs-fMRI images were obtained using an 8-channel head coil on a 1.5-T Siemens Avanto scanner (Siemens Healthineers, Erlangen, Germany). All participants were given earphones to reduce noise impact during the scan. Each participant was instructed to rest quietly with their eyes closed and to clear their mind of thought. For rs-fMRI, echo planar imaging (EPI) sequence was used to obtain functional images and the parameters as follows: repetition time (TR) =2,000 ms, echo time (TE) =40 ms, slice thickness =4 mm, slices =28, field of view (FOV) =240 mm × 240 mm, matrix =64×64, flip angle =90°, scan range =250, and scan time =506 s. To obtain brain structural images, high-resolution 3D T1WI scanning was chosen with follow parameters: TR/TE =1,900/2.93 ms, flip angle =15°, slice thickness =1.0 mm, slice gap =0 mm, FOV =240 mm × 240 mm, matrix =256×256, slices numbers =256, and scan time =423 s. All participants’ brain scans included the entire cerebellum.
Image preprocessing
We used RESTplus software to preprocess all images (37) in the MATLAB 2014a operating environment (MathWorks, Natick, MA, USA). The main procedure was employed as follows: (I) the first 10 time points were removed to achieve magnetic field saturation. (II) For slice-timing and realignment, participants with the excessive head movement were excluded based on the obtained realignment parameters (translation >1.5 mm or rotation >1.5° around any axis). (III) Then, structural images and functional images were co-registered. Structural images were segmented using the unified algorithm (38) and converted into the standard Montreal Neurosciences Institute (MNI) space to generate a series of parameters. Finally, functional images were normalized to the MNI space based on these parameters and resliced to 3×3×3 mm3 (4). A 6-mm Gaussian filter kernel was used for spatial smoothing (5). Several nuisance variables were regressed, including head motion parameters and global, white matter, and ventricular signals (6). Linear detrending and bandpass filter (0.01–0.08 Hz).
FC analysis
To assess the cerebellar intrinsic FC patterns in T2DM patients and HCs, we used the voxel-wise FC analysis in the RESTplus toolbox (36). In this study, we defined 3 seed regions of interest (ROIs), including the left crus I (MNI: −33, −76, −34), right crus I (MNI: 33, −76, −34), and lobule IX (MNI: 0, −55, −49) (Figure 1). These seed ROIs have previously shown intrinsic connectivity with cerebral DMN regions in schizophrenia (34) and healthy participants (28,31,39). In the RESTplus toolbox, the seed ROIs were defined as spheres centered on MNI coordinates with a radius of 6 mm. The correlation map for statistical analysis was obtained by calculating the Pearson correlation coefficient between seed ROIs and other whole-brain voxels, then performing Z transformation with Fisher’s r-to-z transform.
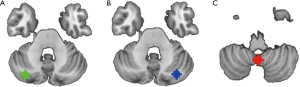
Statistical analysis
The demographic parameters and clinical variables were statistically analyzed using SPSS version 22 (SPSS Inc., IBM, Chicago, IL, USA). Independent sample t-tests and chi-square tests were used to compare differences between groups of variables (P<0.05 was considered a significant difference between groups). The FC statistical analysis within and between groups was carried out in a voxel-wise manner using SPM8 (40). A single-sample t-test was used for intragroup comparisons to obtain brain regions significantly correlated with seed ROIs in each group [voxels P<0.001, Gaussian random fields (GRF) correction]. After controlling for age and gender, the 2-sample t-test was used for between-group comparisons in the union mask obtained by the single-sample t-test to clarify the distribution of brain regions significantly related to the seed ROIs (voxels P<0.001, cluster P<0.05, GRF correction). Finally, we used Pearson correlation analyses to explore the correlation between variables and the z-value of FC with significant intergroup differences in the T2DM group.
Results
Participant characteristics
Detailed demographic characteristics and clinical variables of the 2 groups of participants are summarized in Table 1. Compared to HCs, the participants with T2DM showed higher FPG levels, HbA1c, and high-density lipoprotein (HDL) levels (all P<0.05). No significant differences in age, gender, education years, body mass index (BMI), systolic and diastolic blood pressure, triglyceride, total cholesterol, low-density lipoprotein (LDL), or MoCA (all P>0.05) were identified between groups.
FC results
In the HC group, 3 cerebellar seed ROIs were positively connected to DMN cerebral regions. These regions included the medial prefrontal cortex (mPFC), bilateral inferior parietal lobule (IPL), precuneus/posterior cingulate gyrus, and lateral temporal cortex, consistent with previous research results (28,32,41). In the T2DM group, the FC pattern of the 3 seed regions was similar to that of the HC group, but some FC was different.
Table 2 summarizes the MNI coordinates, cluster size, and t-value of brain regions exhibiting significantly increased and decreased FC with cerebellar seed ROIs in the T2DM group after corrected. Figure 2 presents the FC maps of cerebellar seed regions with significant intergroup differences. Specifically, participants with T2DM showed increased FC between the left crus I and right medial superior frontal gyrus (mSFG) and left mSFG compared to HCs (Figure 2A,B). Additionally, the FC between the right crus I and the left medial orbitofrontal cortex (mOFC) was also higher in T2DM patients than HCs (Figure 2C). Besides, T2DM patients showed significantly decreased FC between lobule IX and the right angular gyrus (ANG) compared to HCs (Figure 2D).
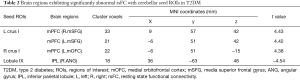
Full table
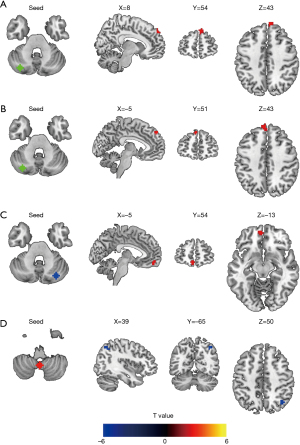
Correlation analyses
In the correlation analysis, we found that diabetes duration was positively correlated with FC between the left crus I and right mSFG (r=0.438, P=0.007, Figure 3). After controlling for age, gender, and education level, this correlation persisted (r=0.351, P=0.039). Moreover, no other significant correlations exsited among the T2DM patients.
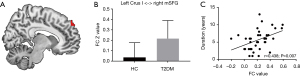
Discussion
This study aimed to investigate the intrinsic FC patterns between the cerebellum and the DMN in T2DM patients using a seed-based FC analysis method. We found increased FC between the cerebellum and anterior DMN (mPFC) and decreased FC between the cerebellum and posterior DMN (IPL) in T2DM patients compared with HCs. The increased FC between the cerebellum and mPFC (right mSFG) was positively correlated with diabetes duration. This changing pattern of impairment combined with compensation may be a marker of early brain damage in T2DM patients that can help us better understand the pathogenesis of brain dysfunction in T2DM.
More specifically, we found decreased FC between lobule IX and the right ANG in T2DM patients compared with HCs, suggesting that the functional interactivity of the cerebellum with the posterior DMN (IPL) was disrupted in T2DM patients. Multiple previous studies have revealed the abnormal morphological structure and cell metabolism of the cerebellum and IPL and abnormal structural connectivity between the cerebellum and parietal regions in patients with T2DM (16,19,42). These abnormalities provide a structural basis for discovering the decreased FC between the cerebellum and IPL and support our findings. Due to its unique anatomical location, the ANG is closely related to the input information integration of the occipital, temporal, and parietal lobes (43). A tasking fMRI study showed that the ANG plays an important role in the working memory of healthy participants (44). Working memory impairment is a common type of cognitive impairment in T2DM, and it has been shown to appear in the early stages of T2DM (45). Tasking fMRI also confirmed that the neural activity of the ANG was weakened during memory coding in T2DM patients (46). In addition, the cerebellum is involved in working memory, and cerebellar abnormalities impair working memory (47,48). Taken together, the decreased functional interactivity between the cerebellum and posterior DMN (right ANG) may be involved in the impairment of working memory in T2DM patients, but further studies are necessary for confirmation.
In this study, increased FC between the cerebellum and mPFC (left crus I-bilateral mSFG and right crus I-left mOFC) was also found in T2DM patients compared with HCs. Previous anatomical and neuroimaging studies have confirmed that the cerebellum interacts with the prefrontal lobe (such as the mPFC) through anatomical and functional connections in normal participants (31,49). This provides a basis for studying the FC pattern between the cerebellum and mPFC in T2DM patients. In contrast, a recent fMRI study observed decreased FC between the cerebellum and the prefrontal lobe in T2DM patients (24). We determined that the most likely explanation for these contradictory results was the inconsistent diabetes duration of the participants through further analysis. The average disease duration of the T2DM participants in this study was approximately 5.6 years, indicating that they were in the early stage of the disease. In our recent fMRI study, increased negative FC was found in early-stage T2DM (25). Other studies have shown that patients with early-stage type 1 diabetes also exhibit enhanced FC (50,51). This change may be related to the loss of local inhibitory neurons, causing increased activation of long-distance neurons (52). Another explanation is to define FC enhancement as functional reorganization, which is a compensatory mechanism in response to early structural damage (53,54). The mPFC, an important node of the anterior DMN (55), participates in emotional processing, executive control, memory, and decision making (56). The structure of the mPFC and cerebellum were damaged in T2DM patients (16,17,57,58). Therefore, increased anterior DMN connectivity with the cerebellum may serve as a compensatory mechanism. Before the onset of apparent cognitive deterioration, functional reorganization of networks already has started as a compensatory mechanism for slight decrements in cognitive performance among prediabetes and T2DM patients, and this functional reorganization increased over time in the early stage of T2DM but decreased in the later stage (59). Therefore, this compensatory may be why the patients with early stage T2DM in this study had higher connectivity than HCs. Besides, we found that the increase in FC between the left crus I and bilateral mSFG was positively correlated with diabetes duration. This indicated that the functional reorganization of the cerebellum with the anterior DMN became more obvious with the progression of the disease in early-stage T2DM patients. Taken together, the increased FC between the cerebellum and the anterior DMN might be used as a biomarker of early brain damage and provide a basis for the early diagnosis and prevention of cognitive impairment in patients with T2DM.
In correlation analysis, we did not find any relationship between diabetes-related biomedical measures (such as glycosylated hemoglobin and FPG) and FC changes. However, it should be noted that DMN connectivity exhibits an opposite change trend in different diabetes duration (25), which indicated inconsistent patterns of brain damage at different stages of T2DM. Therefore, we speculate that a single correlation model cannot describe the relationship between the changes of FC and diabetes-related variables in patients with varied diabetes duration, which may be the reason why a consistent correlation could not be found in this study. By trying the correlation analysis in 2 subgroups with different diabetes duration, we found diabetes-related variables (FPG and HbA1c) and the altered FC showed a strong correlation in early stage T2DM patients, but not in late-stage T2DM patients, which supports our speculation to some extent. However, the small number of participants with later stage T2DM in this study limited our subgroup study. Therefore, it is necessary to increase the sample size to investigate the changes in brain function of T2DM patients with different stages in future research, which may help to reveal better the pathological mechanism of brain damage in patients with T2DM.
Several limitations in the present study should be considered. First, it is difficult for a small sample cross-sectional study to reflect the universality of the results. Second, we did not consider the effect of hypoglycemic drugs, which may impact the interpretation of the results. In addition, the MR device used in this study had a low field strength, so the signal-to-noise ratio of images was lower than 3.0 T. Therefore, it is necessary to repeat or confirm our findings on data acquired with high field strength. Finally, we did not find a correlation with neuropsychological tests, which might be because our cognitive test was not sufficiently detailed; therefore, meticulous assessments of cognition in T2DM patients could be carried out in the future.
To conclude, concomitant functional impairment and reorganization in the linkage between the cerebellum and DMN in patients with T2DM (based on the results that the FC between the cerebellum and mPFC is increased and that the FC between the cerebellum and the IPL is decreased) may be a biomarker of early brain injury in T2DM patients that can help us better understand the pathogenesis of cognitive impairment in T2DM.
Acknowledgments
Funding: This work was supported by the Natural Science Foundation of Hunan Province (2019JJ50913 and 2020JJ8072).
Footnote
Conflicts of Interest: All authors have completed the ICMJE uniform disclosure form (available at http://dx.doi.org/10.21037/qims-21-41). The authors have no conflicts of interest to declare.
Ethical Statement: The authors are accountable for all aspects of the work in ensuring that questions related to the accuracy or integrity of any part of the work are appropriately investigated and resolved. The study was conducted following the Declaration of Helsinki (as revised in 2013) and was approved by the Medical Research Ethics Committee of the Third Xiangya Hospital of Central South University {2019-S381}. All participants provided written informed consent before the study.
Open Access Statement: This is an Open Access article distributed in accordance with the Creative Commons Attribution-NonCommercial-NoDerivs 4.0 International License (CC BY-NC-ND 4.0), which permits the non-commercial replication and distribution of the article with the strict proviso that no changes or edits are made and the original work is properly cited (including links to both the formal publication through the relevant DOI and the license). See: https://creativecommons.org/licenses/by-nc-nd/4.0/.
References
- Roden M, Shulman GI. The integrative biology of type 2 diabetes. Nature 2019;576:51-60. [Crossref] [PubMed]
- McCrimmon RJ, Ryan CM, Frier BM. Diabetes and cognitive dysfunction. Lancet 2012;379:2291-9. [Crossref] [PubMed]
- Cox DJ, Kovatchev BP, Gonder-Frederick LA, Summers KH, McCall A, Grimm KJ, Clarke WL. Relationships between hyperglycemia and cognitive performance among adults with type 1 and type 2 diabetes. Diabetes Care 2005;28:71-7. [Crossref] [PubMed]
- Dienel GA. Brain Glucose Metabolism: Integration of Energetics with Function. Physiol Rev 2019;99:949-1045. [Crossref] [PubMed]
- Uddin LQ, Kelly AM, Biswal BB, Castellanos FX, Milham MP. Functional connectivity of default mode network components: correlation, anticorrelation, and causality. Hum Brain Mapp 2009;30:625-37. [Crossref] [PubMed]
- Vaishnavi SN, Vlassenko AG, Rundle MM, Snyder AZ, Mintun MA, Raichle ME. Regional aerobic glycolysis in the human brain. Proc Natl Acad Sci U S A 2010;107:17757-62. [Crossref] [PubMed]
- Passow S, Specht K, Adamsen TC, Biermann M, Brekke N, Craven AR, Ersland L, Gruner R, Kleven-Madsen N, Kvernenes OH, Schwarzlmuller T, Olesen RA, Hugdahl K. Default-mode network functional connectivity is closely related to metabolic activity. Hum Brain Mapp 2015;36:2027-38. [Crossref] [PubMed]
- Yang SQ, Xu ZP, Xiong Y, Zhan YF, Guo LY, Zhang S, Jiang RF, Yao YH, Qin YY, Wang JZ, Liu Y, Zhu WZ. Altered Intranetwork and Internetwork Functional Connectivity in Type 2 Diabetes Mellitus with and without Cognitive Impairment. Sci Rep 2016;6:32980. [Crossref] [PubMed]
- Chen Y, Liu Z, Wang A, Zhang J, Zhang S, Qi D, Chen K, Zhang Z. Dysfunctional organization of default mode network before memory impairments in type 2 diabetes. Psychoneuroendocrinology 2016;74:141-8. [Crossref] [PubMed]
- Musen G, Jacobson AM, Bolo NR, Simonson DC, Shenton ME, McCartney RL, Flores VL, Hoogenboom WS. Resting-state brain functional connectivity is altered in type 2 diabetes. Diabetes 2012;61:2375-9. [Crossref] [PubMed]
- Tan X, Liang Y, Zeng H, Qin C, Li Y, Yang J, Qiu S. Altered functional connectivity of the posterior cingulate cortex in type 2 diabetes with cognitive impairment. Brain Imaging Behav 2019;13:1699-707. [Crossref] [PubMed]
- Li C, Zuo Z, Liu D, Jiang R, Li Y, Li H, Yin X, Lai Y, Wang J, Xiong K. Type 2 Diabetes Mellitus May Exacerbate Gray Matter Atrophy in Patients With Early-Onset Mild Cognitive Impairment. Front Neurosci 2020;14:856. [Crossref] [PubMed]
- Yang S, Wu M, Ajilore O, Lamar M, Kumar A. Metabolic Aberrations Impact Biophysical Integrity of Macromolecular Protein Pools in the Default Mode Network. Diabetes 2016;65:3464-72. [Crossref] [PubMed]
- Macpherson H, Formica M, Harris E, Daly RM. Brain functional alterations in Type 2 Diabetes - A systematic review of fMRI studies. Front Neuroendocrinol 2017;47:34-46. [Crossref] [PubMed]
- Heikkilä O, Makimattila S, Timonen M, Groop PH, Heikkinen S, Lundbom N. Cerebellar glucose during fasting and acute hyperglycemia in nondiabetic men and in men with type 1 diabetes. Cerebellum 2010;9:336-44. [Crossref] [PubMed]
- Redel JM, DiFrancesco M, Vannest J, Altaye M, Beebe D, Khoury J, Dolan LM, Lee G, Brunner H, Holland S, Brady C, Shah AS. Brain gray matter volume differences in obese youth with type 2 diabetes: a pilot study. J Pediatr Endocrinol Metab 2018;31:261-8. [Crossref] [PubMed]
- Roy B, Ehlert L, Mullur R, Freeby MJ, Woo MA, Kumar R, Choi S. Regional Brain Gray Matter Changes in Patients with Type 2 Diabetes Mellitus. Sci Rep 2020;10:9925. [Crossref] [PubMed]
- Zhang D, Shi L, Song X, Shi C, Sun P, Lou W, Wang D, Luo L. Neuroimaging endophenotypes of type 2 diabetes mellitus: a discordant sibling pair study. Quant Imaging Med Surg 2019;9:1000-13. [Crossref] [PubMed]
- Hsu JL, Chen YL, Leu JG, Jaw FS, Lee CH, Tsai YF, Hsu CY, Bai CH, Leemans A. Microstructural white matter abnormalities in type 2 diabetes mellitus: a diffusion tensor imaging study. Neuroimage 2012;59:1098-105. [Crossref] [PubMed]
- Fang P, An J, Tan X, Zeng LL, Shen H, Qiu S, Hu D. Changes in the cerebellar and cerebro-cerebellar circuit in type 2 diabetes. Brain Res Bull 2017;130:95-100. [Crossref] [PubMed]
- Wang ZL, Zou L, Lu ZW, Xie XQ, Jia ZZ, Pan CJ, Zhang GX, Ge XM. Abnormal spontaneous brain activity in type 2 diabetic retinopathy revealed by amplitude of low-frequency fluctuations: a resting-state fMRI study. Clin Radiol 2017;72:340.e1-340.e7. [Crossref] [PubMed]
- Xia W, Wang S, Sun Z, Bai F, Zhou Y, Yang Y, Wang P, Huang Y, Yuan Y. Altered baseline brain activity in type 2 diabetes: a resting-state fMRI study. Psychoneuroendocrinology 2013;38:2493-501. [Crossref] [PubMed]
- Wang CX, Fu KL, Liu HJ, Xing F, Zhang SY. Spontaneous brain activity in type 2 diabetics revealed by amplitude of low-frequency fluctuations and its association with diabetic vascular disease: a resting-state FMRI study. PLoS One 2014;9:e108883 [Crossref] [PubMed]
- Zhang D, Gao J, Yan X, Tang M, Zhe X, Cheng M, Chen W, Zhang X. Altered functional connectivity of brain regions based on a meta-analysis in patients with T2DM: A resting-state fMRI study. Brain Behav 2020;10:e01725 [Crossref] [PubMed]
- Liu H, Liu J, Peng L, Feng Z, Cao L, Liu H, Shen H, Hu D, Zeng LL, Wang W. Changes in default mode network connectivity in different glucose metabolism status and diabetes duration. Neuroimage Clin 2019;21:101629 [Crossref] [PubMed]
- Carta I, Chen CH, Schott AL, Dorizan S, Khodakhah K. Cerebellar modulation of the reward circuitry and social behavior. Science 2019;363:eaav0581 [Crossref] [PubMed]
- Wagner MJ, Luo L. Neocortex-Cerebellum Circuits for Cognitive Processing. Trends Neurosci 2020;43:42-54. [Crossref] [PubMed]
- Habas C, Kamdar N, Nguyen D, Prater K, Beckmann CF, Menon V, Greicius MD. Distinct cerebellar contributions to intrinsic connectivity networks. J Neurosci 2009;29:8586-94. [Crossref] [PubMed]
- Sang L, Qin W, Liu Y, Han W, Zhang Y, Jiang T, Yu C. Resting-state functional connectivity of the vermal and hemispheric subregions of the cerebellum with both the cerebral cortical networks and subcortical structures. Neuroimage 2012;61:1213-25. [Crossref] [PubMed]
- Buckner RL, Krienen FM, Castellanos A, Diaz JC, Yeo BT. The organization of the human cerebellum estimated by intrinsic functional connectivity. J Neurophysiol 2011;106:2322-45. [Crossref] [PubMed]
- Krienen FM, Buckner RL. Segregated fronto-cerebellar circuits revealed by intrinsic functional connectivity. Cereb Cortex 2009;19:2485-97. [Crossref] [PubMed]
- Filippini N, MacIntosh BJ, Hough MG, Goodwin GM, Frisoni GB, Smith SM, Matthews PM, Beckmann CF, Mackay CE. Distinct patterns of brain activity in young carriers of the APOE-epsilon4 allele. Proc Natl Acad Sci U S A 2009;106:7209-14. [Crossref] [PubMed]
- Luo X, Chen G, Jia Y, Gong J, Qiu S, Zhong S, Zhao L, Chen F, Lai S, Qi Z, Huang L, Wang Y. Disrupted Cerebellar Connectivity with the Central Executive Network and the Default-Mode Network in Unmedicated Bipolar II Disorder. Front Psychiatry 2018;9:705. [Crossref] [PubMed]
- Guo W, Liu F, Zhang Z, Liu G, Liu J, Yu L, Xiao C, Zhao J. Increased Cerebellar Functional Connectivity with the Default-Mode Network in Unaffected Siblings of Schizophrenia Patients at Rest. Schizophr Bull 2015;41:1317-25. [Crossref] [PubMed]
- Pagen LHG, van de Ven VG, Gronenschild E, Priovoulos N, Verhey FRJ, Jacobs HIL. Contributions of Cerebro-Cerebellar Default Mode Connectivity Patterns to Memory Performance in Mild Cognitive Impairment. J Alzheimers Dis 2020;75:633-47. [Crossref] [PubMed]
- Alberti KG, Zimmet PZ. Definition, diagnosis and classification of diabetes mellitus and its complications. Part 1: diagnosis and classification of diabetes mellitus provisional report of a WHO consultation. Diabet Med 1998;15:539-53. [Crossref] [PubMed]
- Jia XZ, Wang J, Sun HY, Zhang H, Liao W, Wang Z, Yan CG, Song XW, Zang YF. RESTplus: an improved toolkit for resting-state functional magnetic resonance imaging data processing. Science Bulletin 2019;64:953-4. [Crossref]
- Ashburner J, Friston KJ. Unified segmentation. Neuroimage 2005;26:839-51. [Crossref] [PubMed]
- Bernard JA, Seidler RD, Hassevoort KM, Benson BL, Welsh RC, Wiggins JL, Jaeggi SM, Buschkuehl M, Monk CS, Jonides J, Peltier SJ. Resting state cortico-cerebellar functional connectivity networks: a comparison of anatomical and self-organizing map approaches. Front Neuroanat 2012;6:31. [Crossref] [PubMed]
- Forman SD, Cohen JD, Fitzgerald M, Eddy WF, Mintun MA, Noll DC. Improved assessment of significant activation in functional magnetic resonance imaging (fMRI): use of a cluster-size threshold. Magn Reson Med 1995;33:636-47. [Crossref] [PubMed]
- Li W, Han T, Qin W, Zhang J, Liu H, Li Y, Meng L, Ji X, Yu C. Altered functional connectivity of cognitive-related cerebellar subregions in well-recovered stroke patients. Neural Plast 2013;2013:452439 [Crossref] [PubMed]
- Sinha S, Ekka M, Sharma U, P R, Pandey RM, Jagannathan NR. Assessment of changes in brain metabolites in Indian patients with type-2 diabetes mellitus using proton magnetic resonance spectroscopy. BMC Res Notes 2014;7:41. [Crossref] [PubMed]
- Seghier ML. The angular gyrus: multiple functions and multiple subdivisions. Neuroscientist 2013;19:43-61. [Crossref] [PubMed]
- Vatansever D, Manktelow AE, Sahakian BJ, Menon DK, Stamatakis EA. Angular default mode network connectivity across working memory load. Hum Brain Mapp 2017;38:41-52. [Crossref] [PubMed]
- Zhang Y, Lu S, Liu C, Zhang H, Zhou X, Ni C, Qin W, Zhang Q. Altered brain activation and functional connectivity in working memory related networks in patients with type 2 diabetes: An ICA-based analysis. Sci Rep 2016;6:23767. [Crossref] [PubMed]
- Wood AG, Chen J, Moran C, Phan T, Beare R, Cooper K, Litras S, Srikanth V. Brain Activation during Memory Encoding in Type 2 Diabetes Mellitus: A Discordant Twin Pair Study. J Diabetes Res 2016;2016:3978428 [Crossref] [PubMed]
- Desmond JE, Gabrieli JD, Wagner AD, Ginier BL, Glover GH. Lobular patterns of cerebellar activation in verbal working-memory and finger-tapping tasks as revealed by functional MRI. J Neurosci 1997;17:9675-85. [Crossref] [PubMed]
- Deverett B, Kislin M, Tank DW, Wang SS. Cerebellar disruption impairs working memory during evidence accumulation. Nat Commun 2019;10:3128. [Crossref] [PubMed]
- Strick PL, Dum RP, Fiez JA. Cerebellum and nonmotor function. Annu Rev Neurosci 2009;32:413-34. [Crossref] [PubMed]
- van Duinkerken E, Schoonheim MM, Sanz-Arigita EJ. RG IJ, Moll AC, Snoek FJ, Ryan CM, Klein M, Diamant M, Barkhof F. Resting-state brain networks in type 1 diabetic patients with and without microangiopathy and their relation to cognitive functions and disease variables. Diabetes 2012;61:1814-21. [Crossref] [PubMed]
- Saggar M, Tsalikian E, Mauras N, Mazaika P, White NH, Weinzimer S, Buckingham B, Hershey T, Reiss ALDiabetes Research in Children Network. (DirecNet). Compensatory Hyperconnectivity in Developing Brains of Young Children With Type 1 Diabetes. Diabetes 2017;66:754-62. [Crossref] [PubMed]
- de Haan W, Mott K, van Straaten EC, Scheltens P, Stam CJ. Activity dependent degeneration explains hub vulnerability in Alzheimer's disease. PLoS Comput Biol 2012;8:e1002582 [Crossref] [PubMed]
- Qi Z, Wu X, Wang Z, Zhang N, Dong H, Yao L, Li K. Impairment and compensation coexist in amnestic MCI default mode network. Neuroimage 2010;50:48-55. [Crossref] [PubMed]
- Schoonheim MM, Geurts JJ, Barkhof F. The limits of functional reorganization in multiple sclerosis. Neurology 2010;74:1246-7. [Crossref] [PubMed]
- Shirer WR, Ryali S, Rykhlevskaia E, Menon V, Greicius MD. Decoding subject-driven cognitive states with whole-brain connectivity patterns. Cereb Cortex 2012;22:158-65. [Crossref] [PubMed]
- Euston DR, Gruber AJ, McNaughton BL. The role of medial prefrontal cortex in memory and decision making. Neuron 2012;76:1057-70. [Crossref] [PubMed]
- Wang C, Fu K, Liu H, Xing F, Zhang S. Brain structural changes and their correlation with vascular disease in type 2 diabetes mellitus patients: a voxel-based morphometric study. Neural Regen Res 2014;9:1548-56. [Crossref] [PubMed]
- Moran C, Phan TG, Chen J, Blizzard L, Beare R, Venn A, Munch G, Wood AG, Forbes J, Greenaway TM, Pearson S, Srikanth V. Brain atrophy in type 2 diabetes: regional distribution and influence on cognition. Diabetes Care 2013;36:4036-42. [Crossref] [PubMed]
- van Bussel FC, Backes WH, van Veenendaal TM, Hofman PA, van Boxtel MP, Schram MT, Sep SJ, Dagnelie PC, Schaper N, Stehouwer CD, Wildberger JE, Jansen JF. Functional Brain Networks Are Altered in Type 2 Diabetes and Prediabetes: Signs for Compensation of Cognitive Decrements? The Maastricht Study. Diabetes 2016;65:2404-13. [Crossref] [PubMed]