Investigating the association between ground-glass nodules glucose metabolism and the invasive growth pattern of early lung adenocarcinoma
Introduction
Lung adenocarcinoma is the most common and aggressive subtype of lung cancer, with the highest heterogeneity (1). Ground-glass nodules (GGNs) are generally considered to be an indolent subtype of early lung adenocarcinoma, which has a specific follow-up and treatment strategy (2). According to the new classification of lung adenocarcinoma proposed in 2011 by the International Association for the Study of Lung Cancer, the American Thoracic Society, and the European Respiratory Society (IASLC/ATS/ERS) (3), as well as the World Health Organization’s Classification of Lung Tumors in 2015 (4), lepidic predominant pulmonary lesions (including adenocarcinoma in situ, minimally invasive adenocarcinoma (INV), and non-mucinous lepidic predominant adenocarcinoma) often exhibit a better prognosis. This type of pulmonary lesion rarely exhibits mediastinal lymph node metastasis (2), and the 5-year disease-free survival rate can reach 93.8% (5). In contrast, invasive lung adenocarcinoma (acinar, papillary, micropapillary, and solid predominant INV) has a 5-year disease-free survival rate of 40–85% (5,6). Therefore, accurate and non-invasive identification of the histopathological subtypes and growth patterns of early lung adenocarcinoma can improve the prediction of patient prognosis and help design optimal management and treatment plans for GGNs.
Previous studies (7-9) have attempted to use the conventional computed tomography (CT) morphological parameters of GGNs (such as lesion size, morphology, boundary, CT attenuation value, solid component ratio, etc.) to predict the invasive growth pattern of early lung adenocarcinoma; however, the efficacy of this approach was unsatisfactory. A meta-analysis (10) found that the sensitivity and specificity of using CT image features to evaluate the invasiveness of GGNs were only 0.41–0.52 and 0.56–0.63, and the area under the receiver operating characteristic (ROC) curve (AUC) was only 0.60–0.67. Our previous study also confirmed that early lung adenocarcinoma pathological growth pattern could not be identified by conventional CT morphological parameters alone (11).
Altered metabolism is a hallmark of cancer. 18F-flurodeoxyglucose (18F-FDG) is a glucose analog, and its standard uptake value (SUV) in FDG positron emission tomography (PET) is closely related to the proliferation, invasion, progression, and metastasis of malignant tumors (12). Previous studies have shown that the GGN-based early lung adenocarcinoma often exhibits low or no FDG uptake (13), and its incidence of lymph node or distant metastases is low (2,14). Thus, FDG PET has limited application for identifying GGN-based early lung adenocarcinoma. However, our previous study found that the glucose metabolism level of GGNs (such as SUVmax) in FDG PET could predict the invasiveness of early lung adenocarcinoma, with an AUC of 0.628 (11). These results raised questions regarding the association between GGN glucose metabolism and the pathological growth pattern of early lung adenocarcinoma, the strength of the association, as well as the existence of this association after adjusting for confounding factors (such as patient clinical data, CT image features of GGNs, etc.) and stratification analysis. To address these questions, we carried out this retrospective cohort study of 18F-FDG PET/CT to explore the association between GGN glucose metabolism and early lung adenocarcinoma invasiveness.
Methods
Clinical data
We retrospectively analyzed patients who underwent PET/CT and high-resolution computed tomography (HRCT) imaging due to lung nodules and received surgery at our hospital from November 2011 to March 2020. The surgical pathological classification was based on the IASLC/ATS/ERS lung adenocarcinoma classification standard (3). According to previous literature, the enrolled patients were divided into a lepidic predominant pulmonary lesions (LPL) group and an INV group (15).
The inclusion criteria were as follows: (I) imaging manifestation was GGN; (II) GGN diameter ≤3 cm; (III) PET/CT and focal continuous HRCT imaging were performed preoperatively; (IV) the surgery and PET/CT, HRCT imaging were completed within 1 month; (V) all lesions were surgically removed, and the pathological data was complete; (VI) the nodules were confirmed to be carcinoma in situ or stage IA adenocarcinoma by postoperative pathological examination; and (VII) patients with no history of severe liver disease, diabetes, or tumor. The exclusion criteria were as follows: (I) poor image quality or the lesions were difficult to measure; and (II) patients who had previously received anti-tumor treatment.
Patient information, including age, gender, smoking status, imaging characteristics, quantitative analysis results of PET/CT and HRCT, and postoperative pathology, were recorded. The study protocol followed the Declaration of Helsinki’s tenets and was approved by the Ethics committee of our hospital [(2018) KD 013]. The study flowchart is shown in Figure 1.
Imaging equipment and methods
The Germany Siemens Biograph mCT [64] PET/CT scanner (Siemens Medical Solutions, Hoffman Estates, Illinois, USA) was used for 18F-FDG PET/CT imaging. The imaging agent was 18F-FDG (JYAMS PET Research and Development Ltd, Nanjing, China, radiochemical purity>95%). The patients were fasted for at least 6 hours before scanning, and 18F-FDG (3.70–5.18 MBq/kg) was injected intravenously after fasting blood glucose examination. The patients underwent a PET/CT scan after resting for 45–60 minutes in a quiet, warm, and dark environment.
CT scanning utilized CareDose 4D technology (the tube current was automatically adjusted during the CT scan according to body shape, anatomical structure, and tissue density), with tube voltage 100 kV, screw pitch 0.8, bulb tube single layer rotation time 0.5 s, and layer thickness 5 mm. PET scanning used three-dimensional mode; the scanning range was from the skull base to the upper femur, and the acquisition time was 2 min/bed. The Syngo TureD system (Siemens, Germany) was used for image reconstruction.
HRCT scanning was performed immediately after PET/CT scanning with the patient holding their breath, and the scan area was the location of lung nodules. The scanning parameters were as follows: voltage 140 kV, tube current 64 mAs, rotation time 0.5 s, pitch 0.6, and slice thickness 1.0 mm. The reconstruction algorithms were as follows: B70f very sharp and B41f medium +; matrix, 512×512. The CT window was set as follows: lung window width 1,200 HU, window level −600 HU; mediastinal window width 350 HU, window level 40 HU.
Image analysis
The SUVmax of the GGNs was used as the quantitative index of PET images. The measurement of GGN SUVmax was performed on the PET/CT (lung window) fusion image. We selected a circular region of interest (ROI) that completely covered the nodule, and the maximum SUVmax was measured layer by layer. When measuring the SUVmax, the image was magnified two times to reduce measurement errors, and the averaged value measured by two nuclear physicians was recorded.
The HRCT qualitative indicators included the following: GGN number (single, multiple), location [peripheral (2/3 area outside the lung on cross-sectional CT image), center (1/3 area inside the lung on cross-sectional CT image)], type [pure ground-glass nodules (pGGN), mixed ground-glass nodules (mGGN)], edge (smooth, lobulated), shape (circular/quasi-circular, irregular), bronchial sign, vacuole sign, pleural depression sign, and vessel convergence sign.
The HRCT quantitative indicators included the following: (I) GGN diameter (DGGN, the long diameter of the largest cross-section of the nodule on lung window); (II) the diameter of the solid component in the GGNs (Dsolid, the long diameter of the largest cross-section of the solid component on lung window); (III) the proportion of solid components (consolidation/tumor ratio (CTR), the ratio of Dsolid to DGGN); (IV) the average Hounsfield Unit of ground-glass components in the GGNs (CTGGO, the average value was measured three times at different lung window levels using a circular ROI. Solid components, blood vessels, and the dilated bronchus and vacuoles inside the nodule were avoided); (V) the average Hounsfield Unit of normal lung parenchyma (CTLP, the average Hounsfield Unit of normal lung tissue around the GGNs. The average value was measured three times at different lung window levels using a circular ROI); and (VI) ΔCTGGO-LP (the difference between CTGGO and CTLP). When measuring the above indicators, the images were magnified 3–8 times to reduce measurement errors, and all data were recorded as the averaged measurement from two radiologists. Representative cases are shown in Figure 2.
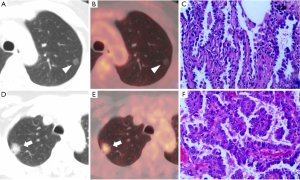
Statistical analysis
Statistical analysis was performed using R3.4.3 (http://www.R-project.org). Normally distributed data were expressed as mean ± SD, non-normally distributed data were expressed as median P50 (P25, P75), and counting data/categorical variables were expressed as number or percentage. The intraclass correlation coefficient was used to analyze the consistency between two observers’ measurements. The chi-square test (categorical variable), single-factor analysis of variance (normally distributed continuous variable), and Kruskal-Wallis test (skew continuous variable) were used to analyze the differences between groups.
Univariate logistic regression analysis was used to calculate the relationship between the imaging characteristics of GGNs with different glucose metabolism levels and INV. Generalized linear models with a logit link were used to test whether the GGN glucose metabolism level (SUVmax) was an independent risk factor for INV. Exact and asymptotic methods were used to calculate unadjusted and adjusted estimates, respectively. Covariates were included as potential confounders in the final models if they changed the estimates of the SUVmax on INV by more than 10% or were significantly associated with INV (P<0.10). Generalized additive models were used to test whether there was a non-linear relationship between INV and the SUVmax. This model helped to find non-linear relationships and determine whether there was a threshold effect.
In the stratified analysis and the interaction test, we assessed whether the following categorical variables had an impact on the correlation between the SUVmax and INV: GGN number (single vs. multiple), type (pGGN vs. mGGN), shape (round/quasi-round vs. irregular), edge type (smooth vs. lobulated), bronchial sign (no vs. yes), vacuole sign (no vs. yes), pleural depression sign (no vs. yes), DGGN (≤20 vs. >20 mm), and CTR (≤0.5 vs. >0.5). P<0.05 was considered statistically significant.
Results
Clinical and pathological data of included patients
A total of 156 patients were enrolled in the study, including 45 males and 111 females, with an average age of 61.4±8.9 years. There were 25 smokers, 98 cases with a single GGN, and 58 cases with multiple GGNs. The two observers had consistent measurements on the image characteristics and quantitative analysis of the GGNs (SUVmax, DGGN, Dsolid, CTR, CTGGO, and ΔCTGGO-LP), with intraclass correlation coefficient values of 0.955–0.999 and P<0.001. The pathology of 177 GGNs was confirmed postoperatively: there were 50 LPL cases, including five in situ carcinomas, 16 micro-INVs, and 29 lepidic predominant adenocarcinomas; as well as 127 INV cases, including 105 acinar types, 18 papillary types, and four micropapillary or solid types.
The HRCT imaging characteristics and pathological growth patterns of GGNs with different metabolic levels
According to the SUVmax tertiles, the 177 GGNs were divided into three groups: Tertile 1 (0.4–1.3), Tertile 2 (1.4–2.8), and Tertile 3 (2.9–11.7), which contained 55, 60, and 62 GGNs, respectively. A comparison of the HRCT imaging characteristics and pathological growth patterns between these groups is shown in Table 1. The number and position of the GGNs were not statistically different between the groups (P>0.05); while the type, shape, edge, bronchial sign, vacuole sign, pleural depression sign, vessel convergence sign, DGGN, Dsolid, CTR, CTGGO, and ΔCTGGO-LP were markedly different between the groups (P<0.05). In this study, INV accounted for 71.8% of the total GGN cohort. As the SUVmax increased from Tertile 1 to Tertile 3, the INV proportion also gradually increased from 52.7% (Tertile 1), to 73.3% (Tertile 2), and to 87.1% (Tertile 3); the differences between the groups were significant (P<0.001).
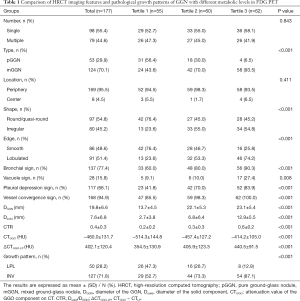
Full table
Association analysis between FDG PET/HRCT features of GGNs and the INV risk
After adjusting for age, gender, smoking history, and fasting blood glucose, we used the postoperative pathology of the GGNs as the dependent variable (INV: Y=1) and 16 GGN imaging features, including SUVmax, as the independent variables to perform single-factor logistic regression analysis. The results showed that GGN type, shape, edge, bronchial sign, vacuole sign, pleural depression sign, vessel convergence sign, DGGN, Dsolid, CTR, CTR grouping (0.5), CTGGO, ΔCTGGO-LP, and SUVmax were all possibly relevant factors for INV (P<0.10). The detailed data are shown in Table 2.
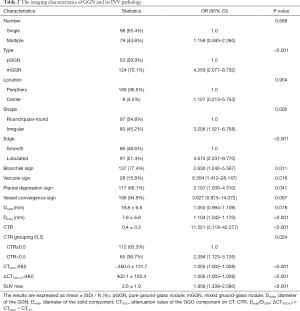
Full table
Multivariate regression analysis on the effect of the SUVmax on INV
To determine the association between GGN SUVmax and INV, we adjusted the potential confounding factors and performed multivariate regression analysis. The unadjusted covariate (non-adjusted) was equivalent to single-factor logistic regression analysis (only adjusted for age, gender, smoking history, and fasting blood glucose); the preliminarily adjusted covariate equation (Adjust I) adjusted seven covariates (age, gender, smoking history, fasting blood glucose, Dsolid, CTR, and ΔCTGGO-LP); and the fully adjusted covariate equation (Adjust II) adjusted 14 covariates (age, gender, smoking history, fasting blood glucose, GGN type, shape, edge, bronchial sign, vacuole sign, pleural depression sign, vessel convergence sign, Dsolid, CTR, and ΔCTGGO-LP).
We observed that an increase in the continuous variable, SUVmax, magnified the INV risk in regression equations with unadjusted, preliminarily adjusted (Adjust I), and fully adjusted (Adjust II) covariates (odd ratios (ORs) were 1.858, 1.520, and 1.399, respectively; P<0.001, 0.029, 0.093; see Table 3). For the three SUVmax tertiles, the increasing trend of the SUVmax was notably associated with an increased INV risk in the regression equations with unadjusted, preliminarily adjusted, and fully adjusted covariates (ORs were 2.112, 1.678, and 1.739, respectively; P=0.001, 0.026 and 0.036, respectively; see Table 3). Ultimately, Adjust I was used as Adjust II may have exhibited a potential statistical inefficiency (see Table 3).
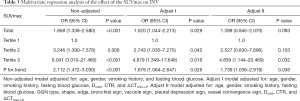
Full table
Curve fitting
Generalized additive models were used to test the association between GGN SUVmax and INV. The results showed that, after adjusting the covariates (age, gender, smoking history, fasting blood glucose, Dsolid, CTR, and ΔCTGGO-LP), the INV risk gradually increased with the increase of the SUVmax. The two were approximately linearly and positively associated (degree of freedom: 1.000, P=0.018, Figure 3).
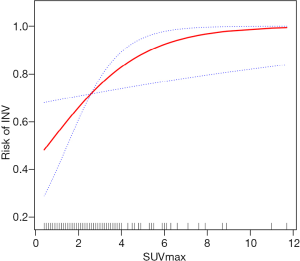
Stratification analysis and interactive effect
Stratification analysis and interaction tests were used to assess whether factors were affecting the association between GGN SUVmax and INV risk. The results showed that GGN number (single vs. multiple), type (pGGN vs. mGGN), shape (round/quasi-round vs. irregular), edge type (smooth vs. lobulated), bronchial sign (no vs. yes), vacuole sign (no vs. yes), pleural depression sign (no vs. yes), DGGN (≤20 vs. >20 mm), and CTR (≤0.5 vs. >0.5) did not significantly alter the association between the SUVmax and INV risk (the range of P for interaction was 0.129–0.909) (Figure 4).
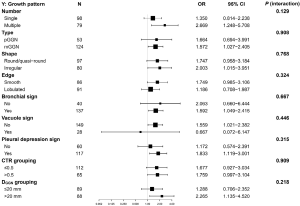
Discussion
As a semi-quantitative index in FDG PET, the SUVmax is widely used in the diagnosis, staging, treatment efficacy monitoring, and follow-up of lung cancer (16) and the construction of various clinical diagnosis and prediction models (17). Previous studies (11,13,18) have reported that the SUVmax of INV (acinar, papillary, micropapillary, solid-predominant INV) is significantly higher than that of lepidic predominant pulmonary lesions; however, the relationship between the SUVmax and invasiveness has not been fully elucidated. In this study, after adjusting for the confounding factors and performing stratified analysis and curve fitting, we found an approximately linear and positive association between the SUVmax and INV, and the risk of INV gradually increased with the increase of the SUVmax.
Numerous studies (8,19-22) have found that the morphological characteristics and quantitative parameters of lung GGN HRCT are related to INV. Consistent with these previous reports, our study also found that when the lung GGN was mGGN, the INV risk increased with the increase in GGN diameter, proportion of solid components (Dsolid, CTR), density of ground-glass components, as well as the positivity in bronchial sign, vacuole sign, pleural depression sign, and vessel convergence sign. However, the correlation between HRCT imaging features of lung GGNs and INV is not absolute, and its efficacy in predicting INV is insufficient (11). The reason for this might be that although the solid components observable by HRCT are likely to be the invasive components in histopathological examinations (in mGGN), they may also be benign or fibrous scars containing interstitial infiltrating components (23). Even in pGGN, there is still a considerable proportion (more than 40%) exhibiting invasive growth patterns (acinar or papillary predominant INV), as confirmed by postoperative pathological results (24).
The GGN size is also an important factor affecting the SUVmax (11,13,25); the larger the GGN diameter, the higher the SUVmax. Dsolid and CTR indicate the size and proportion of solid components in GGNs; the more solid components and fewer ground-glass components in the GGNs, the higher the SUVmax. The SUVmax of mGGN is significantly higher than that of pGGN (14), and the SUVmax of GGNs with CTR >0.5 is markedly higher than those with CTR ≤0.5 (26). The Hounsfield Unit reflects the number and density of cells in the lesion; the higher the Hounsfield Unit, the greater the density, the higher the number of abnormal cells per unit space, and the more FDG uptake. When GGNs are accompanied by aggressive CT imaging features (such as vacuole sign, pleural depression sign, vessel convergence sign, etc.), the SUVmax is significantly higher than those without such signs. Therefore, many HRCT morphological characteristics and quantitative parameters of lung GGNs are related to INV and the SUVmax, which constituted the confounding factors in our study of the association between the SUVmax and INV. Previous studies were more focused on the traditional influencing factors of the SUVmax, such as fasting time, fasting blood glucose, PET reconstruction parameters, body mass index, etc. (27). Our study is the first to systematically exclude the confounding interference from the qualitative and quantitative parameters of lung GGN HRCT, and faithfully clarify the relationship between the SUVmax and INV.
As a characteristic of malignant tumors, tumor cells can obtain energy through anaerobic glycolysis in an aerobic environment. Thus, there is usually intermittent hypoxia in tumor tissues, which induces the expression of glucose transmembrane transporter-1 (28) to increase glucose uptake. Moreover, the glucose metabolism level of GGN-based early lung adenocarcinoma is often related to the expression of glycolysis-related genes, tumor immune microenvironment (29), Ki-67 expression (30), and mutations in epidermal growth factor receptor (EGFR), tumor protein 53 (TP53), and kirsten rat sarcoma viral oncogene (KRAS) (31). The increase of lung GGN SUVmax indicates a poor prognosis and a high-risk of malignancy (32). Since we used different PET scanners, the SUVmax was not a universal value. However, the increase of GGN SUVmax still indicated the invasive growth pattern of tumor cells and poor prognosis. This relationship provides an important basis for risk stratification, clinical management, and surgical methods of GGNs (wedge/segment resection or lobectomy), especially for multi-GGNs. Clarification of the association between GGN SUVmax and INV also provides scientific evidence for the construction of clinical diagnosis and prognosis prediction models and the control of confounding factors.
Limitations
This study has several limitations that should be noted. Firstly, this was a single-center retrospective study. There were only four micropapillary or solid-predominant lung adenocarcinomas and an excessive number of acinar predominant types (105/177, 59.3%). The INV group could not be further divided into acinar + papillary-predominant and micropapillary + solid-predominant subgroups for analysis. Secondly, this study did not include information about the prognosis of patients with different GGN growth patterns. Thus, the relationship between the SUVmax and the prognosis of GGNs with different growth patterns was not established.
Conclusions
In summary, this study retrospectively analyzed patients who underwent PET/CT examination and surgical resection due to persistent lung GGNs, which were confirmed as early lung adenocarcinoma via postoperative pathology examination. After fully adjusting for the confounding and interaction factors, we found an approximately linear and positive association between the SUVmax and INV, and the risk of INV gradually increased with the increase of the SUVmax. This finding provides a scientific basis for constructing clinical diagnosis and prognosis prediction models and the control of confounding factors.
Acknowledgments
Funding: This study was supported by Key Laboratory of Changzhou High-tech Research Project (Grant No. CM20193010) and Changzhou Sci&Tech Program (Grant No. CJ20180022).
Footnote
Conflicts of Interest: All authors have completed the ICMJE uniform disclosure form (available at http://dx.doi.org/10.21037/qims-20-1189). The authors have no conflicts of interest to declare.
Ethical Statement: The authors are accountable for all aspects of the work in ensuring that questions related to the accuracy or integrity of any part of the work are appropriately investigated and resolved. The study was conducted in accordance with the Declaration of Helsinki (as revised in 2013). This study was approved by the ethics committee of the Third Affiliated Hospital of Soochow University for retrospective analysis [(2018) KD 013]. The requirement for individual consent for this retrospective analysis was waived.
Open Access Statement: This is an Open Access article distributed in accordance with the Creative Commons Attribution-NonCommercial-NoDerivs 4.0 International License (CC BY-NC-ND 4.0), which permits the non-commercial replication and distribution of the article with the strict proviso that no changes or edits are made and the original work is properly cited (including links to both the formal publication through the relevant DOI and the license). See: https://creativecommons.org/licenses/by-nc-nd/4.0/.
References
- Devarakonda S, Morgensztern D, Govindan R. Genomic alterations in lung adenocarcinoma. Lancet Oncol 2015;16:e342-51. [Crossref] [PubMed]
- Zhang Y, Fu F, Chen H. Management of ground-glass opacities in the lung cancer spectrum. Ann Thorac Surg 2020;110:1796-804. [Crossref] [PubMed]
- Travis WD, Brambilla E, Noguchi M, Nicholson AG, Geisinger KR, Yatabe Y, Beer DG, Powell CA, Riely GJ, Van Schil PE, Garg K, Austin JH, Asamura H, Rusch VW, Hirsch FR, Scagliotti G, Mitsudomi T, Huber RM, Ishikawa Y, Jett J, Sanchez-Cespedes M, Sculier JP, Takahashi T, Tsuboi M, Vansteenkiste J, Wistuba I, Yang PC, Aberle D, Brambilla C, Flieder D, Franklin W, Gazdar A, Gould M, Hasleton P, Henderson D, Johnson B, Johnson D, Kerr K, Kuriyama K, Lee JS, Miller VA, Petersen I, Roggli V, Rosell R, Saijo N, Thunnissen E, Tsao M, Yankelewitz D. International association for the study of lung cancer/american thoracic society/european respiratory society international multidisciplinary classification of lung adenocarcinoma. J Thorac Oncol 2011;6:244-85. [Crossref] [PubMed]
- Travis WD, Brambilla E, Nicholson AG, Yatabe Y, Austin JHM, Beasley MB, Chirieac LR, Dacic S, Duhig E, Flieder DB, Geisinger K, Hirsch FR, Ishikawa Y, Kerr KM, Noguchi M, Pelosi G, Powell CA, Tsao MS, Wistuba I, Panel WHO. The 2015 World Health Organization Classification of Lung Tumors: Impact of Genetic, Clinical and Radiologic Advances Since the 2004 Classification. J Thorac Oncol 2015;10:1243-60. [Crossref] [PubMed]
- Nakamura H, Saji H, Shinmyo T, Tagaya R, Kurimoto N, Koizumi H, Takagi M. Close association of IASLC/ATS/ERS lung adenocarcinoma subtypes with glucose-uptake in positron emission tomography. Lung Cancer 2015;87:28-33. [Crossref] [PubMed]
- Gong J, Liu JY, Hao W, Nie SD, Zheng B, Wang SP, Peng WJ. A deep residual learning network for predicting lung adenocarcinoma manifesting as ground-glass nodule on CT images. Eur Radiol 2020;30:1847-55. [Crossref] [PubMed]
- Zhang Y, Shen Y, Qiang JW, Ye JD, Zhang J, Zhao RY. HRCT features distinguishing pre-invasive from invasive pulmonary adenocarcinomas appearing as ground-glass nodules. Eur Radiol 2016;26:2921-8. [Crossref] [PubMed]
- Aherne EA, Plodkowski AJ, Montecalvo J, Hayan S, Zheng J, Capanu M, Adusumilli PS, Travis WD, Ginsberg MS. What CT characteristics of lepidic predominant pattern lung adenocarcinomas correlate with invasiveness on pathology? Lung Cancer 2018;118:83-9. [Crossref] [PubMed]
- Liang J, Xu XQ, Xu H, Yuan M, Zhang W, Shi ZF, Yu TF. Using the CT features to differentiate invasive pulmonary adenocarcinoma from pre-invasive lesion appearing as pure or mixed ground-glass nodules. Br J Radiol 2015;88:20140811 [Crossref] [PubMed]
- Dai J, Yu G, Yu J. Can CT imaging features of ground-glass opacity predict invasiveness? A meta-analysis. Thorac Cancer 2018;9:452-8. [Crossref] [PubMed]
- Shao X, Niu R, Jiang Z, Shao X, Wang Y. Role of PET/CT in Management of Early Lung Adenocarcinoma. AJR Am J Roentgenol 2020;214:437-45. [Crossref] [PubMed]
- Takenaka T, Yano T, Ito K, Morodomi Y, Miura N, Kawano D, Shoji F, Abe K, Honda H, Maehara Y. Biological significance of the maximum standardized uptake values on positron emission tomography in non-small cell lung cancer. J Surg Oncol 2009;100:688-92. [Crossref] [PubMed]
- Son BY, Cho S, Yum SW, Kim K, Jheon S. The maximum standardized uptake value of preoperative positron emission tomography/computed tomography in lung adenocarcinoma with a ground-glass opacity component of less than 30 mm. J Surg Oncol 2018;117:451-6. [Crossref] [PubMed]
- Kim TJ, Park CM, Goo JM, Lee KW. Is there a role for FDG PET in the management of lung cancer manifesting predominantly as ground-glass opacity? AJR Am J Roentgenol 2012;198:83-8. [Crossref] [PubMed]
- Alpert JB, Rusinek H, Ko JP, Dane B, Pass HI, Crawford BK, Rapkiewicz A, Naidich DP. Lepidic Predominant Pulmonary Lesions (LPL): CT-based Distinction From More Invasive Adenocarcinomas Using 3D Volumetric Density and First-order CT Texture Analysis. Acad Radiol 2017;24:1604-11. [Crossref] [PubMed]
- Wang Y, Zhao N, Wu ZB, Pan N, Shen XJ, Liu T, Wei F, You J, Xu WG, Ren XB. New insight on the correlation of metabolic status on F-FDG PET/CT with immune marker expression in patients with non-small cell lung cancer. Eur J Nucl Med Mol Imaging 2020;47:1127-36. [Crossref] [PubMed]
- Kawakita N, Toba H, Kawakami Y, Takizawa H, Bando Y, Otuska H, Matsumoto D, Takashima M, Tsuboi M, Yoshida M, Kondo K, Tangoku A. Use of a prognostic risk score that aggregates the FDG-PET/CT SUVmax, tumor size, and histologic group for predicting the prognosis of pStage I lung adenocarcinoma. Int J Clin Oncol 2020;25:1079-89. [Crossref] [PubMed]
- Sun XY, Chen TX, Chang C, Teng HH, Xie C, Ruan MM, Lei B, Liu L, Wang LH, Yang YH, Xie WH. SUVmax of FDG PET/CT Predicts Histological Grade of Lung Adenocarcinoma. Acad Radiol 2021;28:49-57. [Crossref] [PubMed]
- Cohen JG, Reymond E, Lederlin M, Medici M, Lantuejoul S, Laurent F, Arbib F, Jankowski A, Moreau-Gaudry A, Ferretti GR. Differentiating pre- and minimally invasive from invasive adenocarcinoma using CT-features in persistent pulmonary part-solid nodules in Caucasian patients. Eur J Radiol 2015;84:738-44. [Crossref] [PubMed]
- Qi L, Lu WW, Yang L, Tang W, Zhao SJ, Huang Y, Wu N, Wang JW. Qualitative and quantitative imaging features of pulmonary subsolid nodules: differentiating invasive adenocarcinoma from minimally invasive adenocarcinoma and preinvasive lesions. J Thorac Dis 2019;11:4835-46. [Crossref] [PubMed]
- Gao F, Li M, Zhang ZW, Xiao L, Zhang GZ, Zheng XP, Hua YQ, Li JY. Morphological classification of pre-invasive lesions and early-stage lung adenocarcinoma based on CT images. Eur Radiol 2019;29:5423-30. [Crossref] [PubMed]
- Zhu Y, Hou D, Lan M, Sun X, Ma X. A comparison of ultra-high-resolution CT target scan versus conventional CT target reconstruction in the evaluation of ground-glass-nodule-like lung adenocarcinoma. Quant Imaging Med Surg 2019;9:1087-94. [Crossref] [PubMed]
- Travis WD, Asamura H, Bankier AA, Beasley MB, Detterbeck F, Flieder DB, Goo JM, MacMahon H, Naidich D, Nicholson AG, Powell CA, Prokop M, Rami-Porta R, Rusch V, van Schil P, Yatabe Y, International Association for the Study of Lung Cancer Staging and Prognostic Factors Committee and Advisory Board Members. The IASLC Lung Cancer Staging Project: Proposals for Coding T Categories for Subsolid Nodules and Assessment of Tumor Size in Part-Solid Tumors in the Forthcoming Eighth Edition of the TNM Classification of Lung Cancer. J Thorac Oncol 2016;11:1204-23.
- Fu F, Zhang Y, Wen Z, Zheng D, Gao Z, Han H, Deng L, Wang S, Liu Q, Li Y, Shen L, Shen X, Zhao Y, Zhao Z, Ye T, Xiang J, Zhang Y, Sun Y, Hu H, Chen H. Distinct Prognostic Factors in Patients with Stage I Non-Small Cell Lung Cancer with Radiologic Part-Solid or Solid Lesions. J Thorac Oncol 2019;14:2133-42. [Crossref] [PubMed]
- Song SH, Ahn JH, Lee HY, Lee G, Choi JY, Kang J, Kim EY, Han J, Kwon OJ, Lee KS, Kim HK, Choi YS, Kim J, Shim YM. Prognostic impact of nomogram based on whole tumour size, tumour disappearance ratio on CT and SUVmax on PET in lung adenocarcinoma. Eur Radiol 2016;26:1538-46. [Crossref] [PubMed]
- Eriguchi D, Shimada Y, Imai K, Furumoto H, Okano T, Masuno R, Matsubayashi J, Kajiwara N, Ohira T, Ikeda N. Predictive accuracy of lepidic growth subtypes in early-stage adenocarcinoma of the lung by quantitative CT histogram and FDG-PET. Lung Cancer 2018;125:14-21. [Crossref] [PubMed]
- Ko KH, Hsu HH, Huang TW, Gao HW, Cheng CY, Hsu YC, Chang WC, Chu CM, Chen JH, Lee SC. Predictive value of 18F-FDG PET and CT morphologic features for recurrence in pathological stage IA non-small cell lung cancer. Medicine (Baltimore) 2015;94:e434 [Crossref] [PubMed]
- Macheda ML, Rogers S, Best JD. Molecular and cellular regulation of glucose transporter (GLUT) proteins in cancer. J Cell Physiol 2005;202:654-62. [Crossref] [PubMed]
- Mitchell KG, Amini B, Wang Y, Carter BW, Godoy MCB, Parra ER, Behrens C, Villalobos P, Reuben A, Lee JJ, Weissferdt A, Moran CA, Fujimoto J, Sepesi B, Walsh GL, Vaporciyan AA, Hofstetter WL, William WN, Gibbons DL, Wang J, et al. 18F-fluorodeoxyglucose positron emission tomography correlates with tumor immunometabolic phenotypes in resected lung cancer. Cancer Immunol Immunother 2020;69:1519-34. [Crossref] [PubMed]
- Watanabe K, Nomori H, Ohtsuka T, Naruke T, Ebihara A, Orikasa H, Yamazaki K, Uno K, Kobayashi T, Goya T. [F-18]Fluorodeoxyglucose positron emission tomography can predict pathological tumor stage and proliferative activity determined by Ki-67 in clinical stage IA lung adenocarcinomas. Jpn J Clin Oncol 2006;36:403-9. [Crossref] [PubMed]
- Li Y, Li X, Li H, Zhao Y, Liu Z, Sun K, Zhu X, Qi Q, An B, Shen D, Li R, Liu T, Mi J, Wang L, Yang F, Bai F, Wang J. Genomic characterisation of pulmonary subsolid nodules: mutational landscape and radiological features. Eur Respir J 2020;55:1901409 [Crossref] [PubMed]
- Lee HY, Lee SW, Lee KS, Jeong JY, Choi JY, Kwon OJ, Song SH, Kim EY, Kim J, Shim YM. Role of CT and PET Imaging in Predicting Tumor Recurrence and Survival in Patients with Lung Adenocarcinoma: A Comparison with the International Association for the Study of Lung Cancer/American Thoracic Society/European Respiratory Society Classification of Lung Adenocarcinoma. J Thorac Oncol 2015;10:1785-94. [Crossref] [PubMed]