Pericoronary adipose tissue attenuation assessed by dual-layer spectral detector computed tomography is a sensitive imaging marker of high-risk plaques
Introduction
Vascular inflammation is a critical factor in atherosclerotic plaque formation and in the triggering of plaque rupture leading to acute coronary syndrome (ACS) (1,2). Pericoronary adipose tissue (PCAT) is a metabolically and immunologically active fat depot encasing coronary arteries with no fascial barrier (3), and it plays an important role in the process of atherosclerosis (4). PCAT can function as a sensor of coronary inflammation (5,6), as it can be infiltrated by vascular inflammation, which suppresses adjacent adipose cell differentiation, resulting in changes in tissue composition that are detectable on coronary computed tomography angiography (CTA) images (7,8). Recent work by Antonopoulos et al. demonstrated that the pericoronary fat attenuation index (FAI), a novel imaging biomarker measured using coronary CTA, could help detect biopsy result-proven vascular inflammation in patients with coronary artery disease (CAD) undergoing cardiac surgery (9). Other researches have shown that PCAT attenuation is associated with vascular inflammation and plaque characteristics, and that vascular inflammation may cause morphological changes in PCAT, simultaneously influencing plaque stability (10-12). Therefore, PCAT attenuation may be a promising non-invasive imaging marker for assessing the risk of high-risk plaque rupture. However, previous studies assessed PCAT attenuation using conventional polychromatic CT, which has a limited capability for material differentiation due to the absorption of polychromatic X-rays by tissues.
In recent years, dual-layer spectral detector computed tomography (SDCT) has emerged as a tool with the potential to improve the detection of tissue characteristics (13,14). It can provide virtual mono-energetic and effective atomic images, and has a good ability to discriminate between tissue components. To our knowledge, few studies have used SDCT to investigate PCAT attenuation. Therefore, we aimed to assess PCAT attenuation using SDCT and to explore an imaging marker derived from SDCT to improve high-risk plaque identification in patients at high risk of developing ACS.
Methods
Study population
This retrospective study was approved by the ethics committee of Shengjing Hospital of China Medical University (No. 2020PS231K). Consecutive patients who presented to the emergency department with chest pain and who underwent coronary CTA using SDCT were enrolled from August 2018 to January 2020. Informed consent was not required due to the retrospective nature of the study. The exclusion criteria were as follows: aged <18 years (n=30); absence of noncalcified or mixed noncalcified plaque on CT images (n=251); anatomic variations of the heart or coronary arteries (n=12); a known malignancy or infectious disease (n=44); previous myocardial infarction or surgery (n=69); and poor image quality or limited/minimal PCAT for analysis (n=19). “Cases” were defined as patients with high-risk plaque and “controls” were patients without high-risk plaque. The flowchart of patient enrollment and the study design are shown in Figure 1.
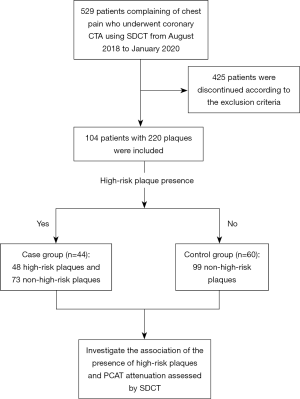
Coronary CTA examination and image reconstruction
All CT acquisitions were performed using a 64-slice SDCT (IQon Spectral CT, Philips Healthcare, Best, The Netherlands). Prior to CT examination, patients with a heart rate ≥70 bpm were administered an oral β-receptor blocker (25–50 mg; Metoprolol Succinate sustained-release tablets, AstraZeneca, Sweden) to reduce and stabilize their heart rate. The coronary CTA scan was acquired using a prospectively electrocardiogram-triggered protocol (‘Step & Shoot Cardiac’). Bolus tracking with iodinated contrast 0.8 mL/kg (Iodixanol, 270 mg/mL, GE Healthcare, Ireland) was used, and flushing with 20 mL saline was carried out at a flow rate of 4.5 mL/s (<80 kg) or 5 mL/s (≥ 80 kg), with the coronary CTA scan acquired 6 seconds after a threshold of 110 Hounsfield Unit (HU) had been reached in the descending aorta. The coronary CTA scan parameters were as follows: the tube voltage was set to 120 kVp; tube current-automatic exposure control (dose right index =13) was used to provide a balance between image quality and radiation dose; field of view =250 mm; tube rotation time =0.27 s; detector collimation =64 × 0.625 mm; matrix =512 × 512; slice thickness =0.9 mm; and increment =0.45 mm. The scan trigger was centered around 78% of the R-R interval, with a ±3% buffer used.
Raw data were reconstructed into: (I) conventional polychromatic images using iterative model reconstruction (IMR; Cardiac Routine Level 1, Philips Healthcare); (II) effective atomic number images; and (III) virtual mono-energetic images (VMI; energy level at 40 keV and 70 keV) using spectral iterative reconstruction (Spectral Level 4, Philips Healthcare). The reconstructed images were transferred to the processing workstations. All studies were reviewed, and for each subject, we selected the cardiac phase exhibiting the least coronary motion artifacts for further analysis.
Epicardial adipose tissue and PCAT analysis
The epicardial adipose tissue (EAT) were analyzed using a semi-automated software (cardiac risk assessment version 1.2.0, Siemens Healthineer, Germany), and an attenuation threshold of −190 to −30 HU was applied to identify adipose tissue voxels in polychromatic images (15,16). Regions of interest (ROIs) were automatically drawn on every scan, tracing the contour of the epicardium using the pulmonary artery bifurcation as the superior limit and left ventricular apex as the inferior limit of the heart (17). If necessary, interpolated contours were manually adjusted. The EAT volume and mean attenuation were recorded in cubic centimeters (cc) and HU, respectively.
As a part of the EAT, PCAT is defined as the adipose tissue voxels located within a distance from the outer vessel wall that is equal to the diameter of the respective vessel (9). PCAT attenuation was measured on conventional images and VMI of 40 keV and 70 keV using the syngo.via software (version VB20, Siemens Healthineer, Forcheim, Germany). Based on the earlier work and investigation of PCAT attenuation (18), the attenuation thresholds of –280 to –40 HU and –220 to –30 HU were respectively applied to identify adipose tissue voxels on 40 and 70 keV VMI. On the spectral attenuation curve of adipose tissue, the mean attenuation on 40–70 keV VMI rose evidently before flattening out above 70 keV (Figure 2). Therefore, in order to highlight the spectral attenuation characteristics of adipose tissue, we selected the range of 40–70 keV to calculate the slope of the spectral attenuation curve. The effective atomic number of PCAT was assessed on 2-dimensional multiplanar reformatted (MPR) effective atomic number images using IntelliSpace Portal 6.5 (Philips Healthcare, Best, The Netherlands). ROIs on MPR images were selected from 5 different views, and the average value of effective atomic number was calculated. On effective atomic number images, ROIs of the same size with accurate co-localization were achieved using the adjacent anatomical structure as a reference and comparative views of conventional images. The mean PCAT attenuation (in HU) assessed by polychromatic images (CTpoly), VMI of 40 keV (CT40 keV) and 70 keV (CT70 keV), the slope of the spectral attenuation curve (λHU) which was calculated as: λHU = (CT40 keV − CT70 keV)/30, and the mean effective atomic number of PCAT (Zeff) were recorded.
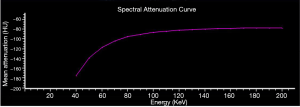
To study the reproducibility of the PCAT attenuation measurements, images for 30 randomly selected subjects were independently measured by 2 radiologists with 11 and 10 years of experience in cardiac imaging, respectively. The radiologists were blinded to the original measurements. The intraclass correlation coefficient (ICC) between the 2 radiologists was calculated.
Coronary plaque analysis
Plaque characteristics were analyzed on polychromatic images by an investigator using the software (IntelliSpace Portal 6.5, Philips Healthcare); the investigator was blinded to the EAT and PCAT attenuation measurements. All coronary segments with a lumen diameter ≥2 mm were analyzed (10,19); however, only noncalcified plaques and mixed noncalcified plaques characteristics were measured, because high-risk plaques are mainly considered to be plaques with spotty calcification or without calcification. Plaques were defined as structures >1 mm2 within or adjacent to the coronary artery lumen that could be clearly distinguished from the vessel lumen and the surrounding adipose tissue (20). The boundaries of the lesion were defined by the proximal and distal non-diseased tissue with an absence of atherosclerotic changes.
The software automatically determined the length, the maximum cross-sectional area of the plaque, total plaque volume, plaque burden, remodeling index, the mean attenuation of the plaque, the low-attenuation percentage of the plaque, the intermediate-attenuation percentage of the plaque, the high-attenuation percentage of the plaque, and diameter stenosis of the lesion. If necessary, corrections were made manually. The plaque burden (in %) was determined as: plaque burden=(plaque area/vessel area) ×100 (21), and the remodeling index was calculated as the ratio of the vessel area of the lesion over the proximal normal arterial vessel area. The following cut-off values were used: low-attenuation (<30 HU), intermediate-attenuation (31–130 HU), and high-attenuation (>131 HU) (10). High-risk plaques were defined as those with at least 2 of the following features: positive remodeling; low density (<30 HU); spotty calcification; or a “napkin-ring” sign (22). Plaques fulfilling at least 2 of these features were classified as non-high-risk plaques.
Statistical analysis
Statistical analyses were performed using Statistical Package for Social Sciences (SPSS) statistics software (version 21.0, IBM). The Shapiro-Wilk test was used to test continuous variables for normal distribution; non-normally distributed variables were presented as the median (25th to 75th percentile) and normally distributed variables were presented as the mean ± standard deviation. Categorical variables were presented as counts (percentages). Continuous variables were compared by using an unpaired t-test, and categorical variables were compared by using the χ2 test. Stepwise-backward logistic regression analysis was performed to identify the presence of high-risk plaque. Due to the existence of individual heterogeneity, adjustment to the mean attenuation of EAT was performed on logistic regression analysis. Optimal thresholds were obtained from a receiver operator characteristic (ROC) curve analysis at the point at which the Youden J statistic (J = sensitivity + specificity − 1) was the highest. A 2-tailed P value of <0.05 was considered to be statistically significant.
Results
Study population information
Among the 44 patients in the case group, there were 48 high-risk plaques and 73 non-high-risk plaques, while among the 60 patients in the control group, 99 non-high-risk plaques were detected. The baseline information of the patients is shown in Table 1. Except for highest-grade stenosis (P=0.049), no significant differences were observed in baseline data between the case and control groups, including in the mean EAT attenuation (all P>0.05).
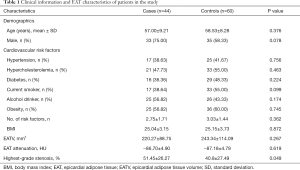
Full table
Plaque anatomical characteristics
Among the 48 high-risk plaques in the case group, 25 (52.08%) were low-density plaques, 43 (89.58%) exhibited positive remodeling, 14 (29.17%) had spotty calcification, and 18 (37.50%) had a napkin-ring sign. As shown in Table 2, high-risk plaques possessed a larger remodeling index, lower CT attenuation, a higher percentage of low-attenuation components, and a lower percentage of intermediate-attenuation components (all P<0.05).
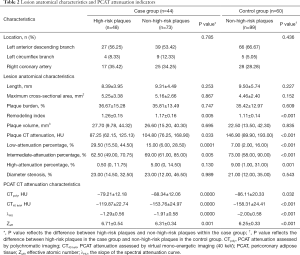
Full table
Association between PCAT attenuation and the presence of high-risk plaques
The intraclass correlation (ICC) for the measurement of PCAT attenuation was 0.816 (P<0.05), showing excellent reproducibility. The attenuation of PCAT surrounding high-risk plaques was higher than that surrounding non-high-risk plaques in both the case and control groups (all P<0.05). Examples of PCAT attenuation surrounding high-risk plaques and non-high-risk plaques are shown in Figure 3.
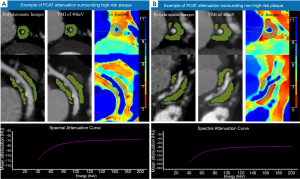
Univariate analysis revealed that the anatomical characteristics related to high-risk plaques included plaque CT attenuation [odds ratio (OR) 0.989; 95% CI: 0.983–0.995; P=0.01], the percentage of intermediate-attenuation in plaque (OR 0.962; 95% CI: 0.943–0.981; P<0.001) and the percentage of high-attenuation in plaque (OR 0.970; 95% CI: 0.945–0.996; P=0.023). The remodeling index and the percentage of low-attenuation in plaque were excluded in the analysis, because they are identifying features of high-risk plaques. Then, after adjustment for the mean attenuation of EAT, multivariate logistic regression analyses including each of the PCAT attenuation indicators (CT40 keV, CTpoly, λHU, and Zeff) and the high-risk plaque characteristics described above were performed. This revealed that the intermediate- and high-attenuation percentages, and all the PCAT attenuation indicators were related to the presence of high-risk plaque (shown in Table 3).
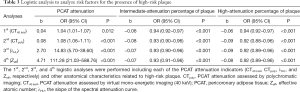
Full table
The area under the receiver operating characteristic (ROC) curve (AUC) of CTpoly, CT40 keV, Zeff, and λHU for the identification of high-risk plaques was 0.687, 0.883, 0.806, and 0.807, respectively. The spectral indicators derived from SDCT, especially CT40 keV, performed better than those derived from polychromatic CT. Based on our data, a cut-off of −120.60 HU for CT40 keV achieved an accuracy of 90% for detecting high-risk plaques (Figure 4, Table 4).
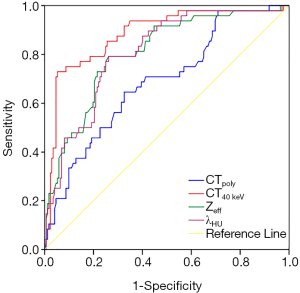
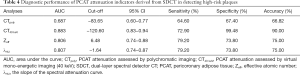
Full table
Discussion
This study found that PCAT surrounding high-risk plaques had higher attenuation than that surrounding non-high-risk plaques and that high PCAT attenuation assessed by VMI at 40 keV (≥−120.60 HU) could be the best imaging marker for identifying high-risk plaques, with an accuracy of 90%.
Some investigations of the association of PCAT attenuation with culprit lesions in ACS patients have suggested that PCAT attenuation is an important prognostic indicator. Antonopoulos et al. were the first to develop the imaging metric FAI, which can non-invasively detect coronary inflammation by mapping the spatial changes of perivascular fat attenuation, and found that PCAT attenuation around culprit lesions was increased by 8.76±2.87 HU compared to that proximal to the lesion (9). The same investigators then performed a post-hoc analysis of 3,912 patients across 2 prospectively recruited cohorts (11). They found that PCAT attenuation around the right coronary artery could be a representative biomarker of global coronary inflammation and that high PCAT attenuation was an indicator of increased cardiac mortality and could guide early targeted prevention. Hedgire et al. demonstrated that perivascular adipose tissue stranding (defined as irregular obscuration of PCAT) could be a helpful predictor of culprit lesions and a risk marker in ACS patients (12). Recently, Goeller et al. (23) evaluated PCAT attenuation around the proximal right coronary artery in relation to plaque changes in a stable CAD cohort followed for at least 1 year. They demonstrated that progression of the non-calcified plaque burden is associated with an increase in PCAT attenuation. In other words, PCAT attenuation could provide important information regarding plaque metabolic activity and high PCAT attenuation may be a risk factor for cardiovascular disease. In our study, the PCAT surrounding high-risk plaques showed higher attenuation than that surrounding non-high-risk plaques. The underlying mechanism may be that high-risk atherosclerotic plaque is highly inflamed and is always accompanied by greater adventitial inflammation than that seen in non-high-risk plaque (24). Inflammatory cells then infiltrate the surrounding perivascular space (25), causing adipose tissue edema or inflammation and thus contributing to higher attenuation. Another possible mechanism is that vascular inflammation sends paracrine signals to PCAT to prevent lipid accumulation in adipocytes, leading to increased PCAT attenuation (9); therefore, the increased vascular inflammation existing in high-risk plaques could in turn lead to higher PCAT attenuation. We observed high PCAT attenuation to be an independent risk factor for high-risk plaques. By establishing cut-offs to identify high-risk plaques using ROC curves generated from polychromatic and spectral images, we showed that PCAT attenuation derived from 40 keV VMI (CT40 keV) possessed a relatively higher AUC and accuracy compared to other indicators because of its higher sensitivity in detecting differences in adipose attenuation. Using a cut-off of −120.60 HU, CT40 keV could distinguish high-risk plaques with an accuracy of 90% and a specificity of 99.48%.
To our knowledge, this is the first study reporting on PCAT attenuation (but not EAT) measured by SDCT, which has emerged as a new CT technology and has the potential to analyze tissue composition using monochromatic evaluation and material decomposition. It is well known that X-ray at low energy has a dominant photoelectric effect, which increases the X-ray absorption difference between materials and produces superior contrast, resulting in an evident change in HU value when approaching lower energies (26). The inflammation caused by a high-risk lesion caused adipose tissue edema and less lipid accumulation, resulting in a difference in the attenuation coefficient on images, and VMI at lower energy could detect the difference more sensitively and capture the existence of inflammation indirectly. We found a CT40 keV to be the most sensitive imaging indicator for detecting differences in PCAT density and discriminating high-risk plaques. A recent study also suggested that differences in adipose tissue density could be better differentiated using low-energy VMI (18). The authors compared EAT and other visceral fat attenuation (measurements were performed on VMI at 40, 70, and 120 keV) in patients using dual-energy CT and found that different regional fat deposits had distinctive regional attenuation values on VMI at lower energy levels; this result supports the findings of the present study. Furthermore, SDCT is a novel spectral CT technology which can provide polychromatic images and a variety of spectral reconstructions from the same examination using standard CT protocols, allowing for retrospective spectral imaging analysis (27). SDCT can also be used to obtain VMI at low energy levels with low levels of noise and a high contrast-to-noise ratio (CNR), because the dual-layer detector can facilitate the exploitation of anti-correlated noise suppression due to perfectly matched raw-data and effectively suppresses image noise (26,28). Therefore, low-energy VMI, such as 40 keV, based on SDCT could be well used in clinical practice. Furthermore, coronary CTA using SDCT could become a part of the routine clinical examination for patients with coronary atherosclerosis by virtue of its sensitivity for the detection of pathological changes in PCAT.
However, limitations exist in this study. Firstly, this is a single-center cross-sectional study investigating a small population, and patients with incomplete clinical data were excluded, which introduced bias. Secondly, intravascular ultrasound data were not available as a reference standard for high-risk plaques. Finally, the PCAT attenuation thresholds still need to be validated in an external cohort.
Conclusions
PCAT surrounding high-risk plaques showed higher attenuation than that surrounding non-high-risk plaques, which has been associated with coronary artery inflammation. The metrics derived from SDCT, especially CT40 keV, showed higher discriminatory power for detecting the changes in PCAT attenuation than polychromatic CT. Therefore, PCAT attenuation assessed by VMI at 40 keV based on SDCT may serve as a novel imaging marker of plaque vulnerability offering some early warning information for clinic.
Acknowledgments
The authors would like to thank Mani Vembar, Hui Yao, and Zheng Jia from Philips for their assistance with CT technology and Yang Yu from Siemens for his assistance with data measurement.
Funding: This study was granted by the National Natural Science Foundation of China (Grant No.82071920, 81901741), the Key Research & Development Plan of Liaoning Province (No. 2020JH2/10300037), and the 345 Talent Project in the Shengjing Hospital of China Medical University.
Footnote
Conflicts of Interest: All authors have completed the ICMJE uniform disclosure form (available at http://dx.doi.org/10.21037/qims-20-860). The authors have no conflicts of interest to declare.
Ethical Statement: The study was conducted in accordance with the Declaration of Helsinki. This retrospective study was approved by the ethics committee of Shengjing Hospital of China Medical University (No. 2020PS231K) without the need for informed consent.
Open Access Statement: This is an Open Access article distributed in accordance with the Creative Commons Attribution-NonCommercial-NoDerivs 4.0 International License (CC BY-NC-ND 4.0), which permits the non-commercial replication and distribution of the article with the strict proviso that no changes or edits are made and the original work is properly cited (including links to both the formal publication through the relevant DOI and the license). See: https://creativecommons.org/licenses/by-nc-nd/4.0/.
References
- Antoniades C, Antonopoulos AS, Deanfield J. Imaging residual inflammatory cardiovascular risk. Eur Heart J 2020;41:748-58. [PubMed]
- Wolf D, Ley K. Immunity and inflammation in atherosclerosis. Circ Res 2019;124:315-27. [Crossref] [PubMed]
- Patel VB, Shah S, Verma S, Oudit GY. Epicardial adipose tissue as a metabolic transducer: role in heart failure and coronary artery disease. Heart Fail Rev 2017;22:889-902. [Crossref] [PubMed]
- Antoniades C, Shirodaria C. Detecting coronary inflammation with perivascular fat attenuation imaging: Making sense from perivascular attenuation maps. JACC Cardiovasc Imaging 2019;12:2011-4. [Crossref] [PubMed]
- Lin A, Dey D, Wong DTL, Nerlekar N. Perivascular adipose tissue and coronary atherosclerosis: from biology to imaging phenotyping. Curr Atheroscler Rep 2019;21:47. [Crossref] [PubMed]
- Ohyama K, Matsumoto Y, Amamizu H, Uzuka H, Nishimiya K, Morosawa S, Hirano M, Watabe H, Funaki Y, Miyata S, Takahashi J, Ito K, Shimokawa H. Association of coronary perivascular adipose tissue inflammation and drug-eluting stent-induced coronary hyperconstricting responses in pigs: F-fluorodeoxyglucose positron emission tomography imaging study. Arterioscler Thromb Vasc Biol 2017;37:1757-64. [Crossref] [PubMed]
- Wang J, Chen D, Cheng XM, Zhang QG, Peng YP, Wang LJ, He SQ, Gong JB. Influence of phenotype conversion of epicardial adipocytes on the coronary atherosclerosis and its potential molecular mechanism. Am J Transl Res 2015;7:1712-23. [PubMed]
- Crewe C, An YA, Scherer PE. The ominous triad of adipose tissue dysfunction: inflammation, fibrosis, and impaired angiogenesis. J Clin Invest 2017;127:74-82. [Crossref] [PubMed]
- Antonopoulos AS, Sanna F, Sabharwal N, Thomas S, Oikonomou EK, Herdman L, Margaritis M, Shirodaria C, Kampoli AM, Akoumianakis I, Petrou M, Sayeed R, Krasopoulos G, Psarros C, Ciccone P, Brophy CM, Digby J, Kelion A, Uberoi R, Anthony S, Alexopoulos N, Tousoulis D, Achenbach S, Neubauer S, Channon KM, Antoniades C. Detecting human coronary inflammation by imaging perivascular fat. Sci Transl Med 2017;9:eaal2658. [Crossref] [PubMed]
- Goeller M, Achenbach S, Cadet S, Kwan AC, Commandeur F, Slomka PJ, Gransar H, Albrecht MH, Tamarappoo BK, Berman DS, Marwan M, Dey D. Pericoronary adipose tissue computed tomography attenuation and high-risk plaque characteristics in acute coronary syndrome compared with stable coronary artery disease. JAMA Cardiol 2018;3:858-63. [Crossref] [PubMed]
- Oikonomou EK, Marwan M, Desai MY, Mancio J, Alashi A, Hutt Centeno E, Thomas S, Herdman L, Kotanidis CP, Thomas KE, Griffin BP, Flamm SD, Antonopoulos AS, Shirodaria C, Sabharwal N, Deanfield J, Neubauer S, Hopewell JC, Channon KM, Achenbach S, Antoniades C. Non-invasive detection of coronary inflammation using computed tomography and prediction of residual cardiovascular risk (the CRISP CT study): a post-hoc analysis of prospective outcome data. Lancet 2018;392:929-39. [Crossref] [PubMed]
- Hedgire S, Baliyan V, Zucker EJ, Bittner DO, Staziaki PV, Takx RAP, Scholtz JE, Meyersohn N, Hoffmann U, Ghoshhajra B. Perivascular epicardial fat stranding at coronary ct angiography: a marker of acute plaque rupture and spontaneous coronary artery dissection. Radiology 2018;287:808-15. [Crossref] [PubMed]
- Ma Q, Hu J, Yang W, Hou Y. Dual-layer detector spectral CT versus magnetic resonance imaging for the assessment of iron overload in myelodysplastic syndromes and aplastic anemia. Jpn J Radiol 2020;38:374-81. [Crossref] [PubMed]
- Bai X, Gao P, Zhang D, Zhang S, Liang J, Lu X, Sui B. Plaque burden assessment and attenuation measurement of carotid atherosclerotic plaque using virtual monoenergetic images in comparison to conventional polyenergetic images from dual-layer spectral detector CT. Eur J Radiol 2020;132:109302. [Crossref] [PubMed]
- Hell MM, Achenbach S, Schuhbaeck A, Klinghammer L, May MS, Marwan M. CT-based analysis of pericoronary adipose tissue density: Relation to cardiovascular risk factors and epicardial adipose tissue volume. J Cardiovasc Comput Tomogr 2016;10:52-60. [Crossref] [PubMed]
- Tamarappoo B, Dey D, Shmilovich H, Nakazato R, Gransar H, Cheng VY, Friedman JD, Hayes SW, Thomson LE, Slomka PJ, Rozanski A, Berman DS. Increased pericardial fat volume measured from noncontrast CT predicts myocardial ischemia by SPECT. JACC Cardiovasc Imaging 2010;3:1104-12. [Crossref] [PubMed]
- Liu Z, Wang S, Wang Y, Zhou N, Shu J, Stamm C, Jiang M, Luo F. Association of epicardial adipose tissue attenuation with coronary atherosclerosis in patients with a high risk of coronary artery disease. Atherosclerosis 2019;284:230-6. [Crossref] [PubMed]
- Rodriguez-Granillo GA, Capunay C, Deviggiano A, De Zan M, Carrascosa P. Regional differences of fat depot attenuation using non-contrast, contrast-enhanced, and delayed-enhanced cardiac CT. Acta Radiol 2019;60:459-67. [Crossref] [PubMed]
- Kitagawa T, Yamamoto H, Sentani K, Takahashi S, Tsushima H, Senoo A, Yasui W, Sueda T, Kihara Y. The relationship between inflammation and neoangiogenesis of epicardial adipose tissue and coronary atherosclerosis based on computed tomography analysis. Atherosclerosis 2015;243:293-9. [Crossref] [PubMed]
- Leber AW, Knez A, Becker A, Becker C, von Ziegler F, Nikolaou K, Rist C, Reiser M, White C, Steinbeck G, Boekstegers P. Accuracy of multidetector spiral computed tomography in identifying and differentiating the composition of coronary atherosclerotic plaques: a comparative study with intracoronary ultrasound. J Am Coll Cardiol 2004;43:1241-7. [Crossref] [PubMed]
- Versteylen MO, Kietselaer BL, Dagnelie PC, Joosen IA, Dedic A, Raaijmakers RH, Wildberger JE, Nieman K, Crijns HJ, Niessen WJ, Daemen MJ, Hofstra L. Additive value of semiautomated quantification of coronary artery disease using cardiac computed tomographic angiography to predict future acute coronary syndrome. J Am Coll Cardiol 2013;61:2296-305. [Crossref] [PubMed]
- Lu MT, Park J, Ghemigian K, Mayrhofer T, Puchner SB, Liu T, Fleg JL, Udelson JE, Truong QA, Ferencik M, Hoffmann U. Epicardial and paracardial adipose tissue volume and attenuation - Association with high-risk coronary plaque on computed tomographic angiography in the ROMICAT II trial. Atherosclerosis 2016;251:47-54. [Crossref] [PubMed]
- Goeller M, Tamarappoo BK, Kwan AC, Cadet S, Commandeur F, Razipour A, Slomka PJ, Gransar H, Chen X, Otaki Y, Friedman JD, Cao JJ, Albrecht MH, Bittner DO, Marwan M, Achenbach S, Berman DS, Dey D. Relationship between changes in pericoronary adipose tissue attenuation and coronary plaque burden quantified from coronary computed tomography angiography. Eur Heart J Cardiovasc Imaging 2019;20:636-43. [Crossref] [PubMed]
- Moreno PR, Purushothaman KR, Fuster V, O'Connor WN. Intimomedial interface damage and adventitial inflammation is increased beneath disrupted atherosclerosis in the aorta: Implications for plaque vulnerability. Circulation 2002;105:2504-11. [Crossref] [PubMed]
- Henrichot E, Juge-Aubry CE, Pernin A, Pache JC, Velebit V, Dayer JM, Meda P, Chizzolini C, Meier CA. Production of chemokines by perivascular adipose tissue: a role in the pathogenesis of atherosclerosis? Arterioscler Thromb Vasc Biol 2005;25:2594-9. [Crossref] [PubMed]
- Ehn S, Sellerer T, Muenzel D, Fingerle AA, Kopp F, Duda M, Mei K, Renger B, Herzen J, Dangelmaier J, Schwaiger BJ, Sauter A, Riederer I, Renz M, Braren R, Rummeny EJ, Pfeiffer F, Noël PB. Assessment of quantification accuracy and image quality of a full-body dual-layer spectral CT system. J Appl Clin Med Phys 2018;19:204-17. [Crossref] [PubMed]
- Goo HW, Goo JM. Dual-energy CT: New horizon in medical imaging. Korean J Radiol 2017;18:555-69. [Crossref] [PubMed]
- Huang X, Gao S, Ma Y, Lu X, Jia Z, Hou Y. The optimal monoenergetic spectral image level of coronary computed tomography (CT) angiography on a dual-layer spectral detector CT with half-dose contrast media. Quant Imaging Med Surg 2020;10:592-603. [Crossref] [PubMed]