Dual-energy CT quantitative parameters for the differentiation of benign from malignant lesions and the prediction of histopathological and molecular subtypes in breast cancer
Introduction
Breast cancer is the most common malignancy in women. It is a heterogeneous disease with various subtypes and therapeutic responses (1). The determination of breast tumor status is not only crucial for reducing unnecessary biopsies of benign lesions but also for reducing the costs and morbidity associated with invasive biopsy procedures. Compared with invasive breast cancer, ductal carcinoma in situ (DCIS) has a good prognosis, and breast-conserving surgery and radiotherapy are frequently recommended as treatments (2). Among invasive non-special carcinomas, the luminal A subtype is highly sensitive to endocrine therapy and is associated with a better prognosis, whereas patients with the luminal B and human epidermal growth factor receptor 2 (HER2)-enriched subtypes tend to have poorer prognoses (3). The basal-like subtype has a tendency to relapse quickly and accounts for a large proportion of deaths after diagnosis (4). Differentiating DCIS and the luminal A subtype without invasive biopsy could spare many women from receiving intensive treatment, thereby preserving their quality of life.
Magnetic resonance imaging (MRI), ultrasonography, and mammography are key modalities for breast tumor diagnosis and the subsequent choice of appropriate therapy (5). MRI is highly sensitive to the detection of breast cancer and is the most sensitive technique for screening high-risk women and detecting contralateral or multifocal disease (6-8). However, concomitant limitations of MRI include a high false-positive rate and limited accessibility in developing countries (7,9). Ultrasonography is restricted by a high false-positive rate and operator dependence, while mammography is limited in dense breast (10), especially in Asian women.
In oncological imaging, dual-energy computed tomography (DECT) has promising clinical application for the characterization of tumors (11). Recently, DECT has attracted wide research interest for use in the differentiation of benign from malignant tumors, as well as histopathological and genotypic classification (12-17). So far, only a few studies (18-21) have evaluated breast lesion visibility by reconstructing images from DECT. A small-sample study (22) demonstrated that the iodine concentration was higher in invasive non-special carcinoma than in DCIS and benign tumors. However, more quantitative parameters and participants should be considered. To date, few studies have specifically investigated the diagnostic performance of DECT in the characterization of breast tumors.
We hypothesized that quantitative parameters derived from DECT could be used for the preoperative characterization of breast tumors. In our study, DECT was performed on participants with breast tumors to obtain the quantitative parameters. Our aim was to assess the diagnostic performance of DECT quantitative parameters in differentiating benign from malignant lesions and predicting histopathological and molecular subtypes in breast cancer patients.
Methods
Participant characteristics
Our prospective, single-center study was approved by the ethics committee of the Chongqing University Cancer Hospital (No. CZLS20200215-A) and written informed consent was obtained from all participants. From June to December 2019, 130 consecutive women were enrolled, with a median age of 53.2±10.01 years (range, 32–87 years). Participants’ imaging (mammography, ultrasonography, MRI) results were highly suspicious, or they had been pathologically diagnosed with breast cancer and were undergoing dual-phase contrast-enhanced DECT for the evaluation of potential lung metastasis or other underlying lung lesions. The inclusion criteria for patients were as follows: (I) a first diagnosis of breast tumor; (II) breast masses with the shortest diameter >1 cm that were visible on DECT enhanced images and within the field of view of DECT; (III) had not undergone biopsy within the 1 week prior to dual-energy CT screening; and (IV) no history of chemotherapy or radiation therapy in the breast space. The exclusion criteria were as follows: (I) impaired kidney function (glomerular filtration rate <30 mL/min); (II) severe contrast media allergy; (III) pregnancy; and (IV) an inability to provide informed consent for the CT examination.
We reviewed and recorded the histopathological diagnostic data from the hospital’s electronic medical records. All pathological data were obtained within the 2 weeks following CT examination. As described in the 2013 St. Gallen guidelines, the molecular subtypes of breast cancer are divided into four categories (23): (I) luminal A [estrogen receptor (ER) or progesterone receptor (PR)+, HER2−, and Ki67−]; (II) luminal B (ER or PR+, HER2−, and Ki67+, or ER or PR+, HER2+, and Ki67+); and (III) HER2-enriched (ER−, PR−, and HER2+); or (IV) basal-like (ER−, PR−, and HER2−).
Dual-energy CT image acquisition
Imaging data were acquired on a 2.5 generation dual-source CT unit (SOMATOM Drive, Siemens Healthineers, Forchheim, Germany). Automatic exposure control (CARE Dose 4D, Siemens Healthineers) was used for all scans. The scanner settings were as follows: collimation, 64×0.6 mm; rotation time, 0.28 s; pitch, 0.55; reference tube current time product, 71 mAs for the 100-kVp tube and 60 mAs for the Sn140-kVp tube; reformatted section thickness, 1.5 mm; reformatted section increment, 1.5 mm. All participants were scanned craniocaudally in the supine position. The non-enhanced scan was acquired first. For contrast-enhanced scanning, iodinated nonionic contrast media (Ioversol, 320 mg/mL iodine, HENGRUI Medicine, Jiangsu, China) was administered via the right or left ulnar vein using a dual-head injector at a dosage of 1.5 mL/kg and a flow rate of 2.5 mL/sec; this was followed by a bolus injection of 30 mL saline administered at the same flow rate. After injection, arterial phase scans were started using a bolus-tracking technique with a threshold of 100 Hounsfield units (HUs) in the descending aorta and an additional delay of 10 seconds. The delay time for venous phase scanning was 25 seconds after the end of arterial phase scanning.
Dual-energy CT quantitative parameters
DECT data were analyzed using viewer software on a syngo.via workstation (syngo.via VB20A, Dual Energy, Siemens Healthineers, Forchheim, Germany). Standard linear-blended images were reconstructed by applying a blending factor of 0.5 (M_0.5; 50% of the low kV and 50% of the high-kV spectrum) for attenuation (HU) measurements. Measurements of dual-energy quantitative parameters were performed by two radiologists (X.X.W, who has 6 years of experience in breast and chest diagnostic imaging, and X.F.Z, who has 2 years of experience in post-reconstruction imaging), who were blinded to the histopathologic results of the patients. A region of interest (ROI) as large as possible was placed on breast lesions, excluding any areas of obvious gross necrosis, calcification, or large vessels. The quantitative parameters of iodine concentration (in milligrams per cubed centimeter) and effective atomic number were divided by the iodine concentration and the effective atomic number of the aorta, respectively, to obtain the normalized iodine concentration (NIC) and the normalized effective atomic number (nZeff), respectively. The slope of the spectral HU curve (λHu in HU per kiloelectron-volt [keV]) was calculated as follows: λHu = (HU40keV − HU70keV)/30keV (24).
Statistical analysis
Statistical analyses were performed using commercially available statistical software (SPSS software, version 25.0; Armonk, US). To assess inter-observer agreement in the quantitative analysis, we used the intraclass correlation coefficient (ICC) with a two-way random model of consistency. In the univariate analysis, continuous data were compared using Welch’s t-test for normal distribution or using the Mann-Whitney U-test for non-normal distribution. Receiver operating characteristic (ROC) curve analysis was used to evaluate the diagnostic ability of DECT quantitative parameters. The sensitivity, specificity, and accuracy were calculated, and the optimal threshold was determined according to the Youden index. To determine the predictive diagnostic performance of DECT quantitative parameters in differentiating malignant lesions of the breast from benign lesions, multivariate logistic regression analysis was performed using the variables with a P value <0.05 from the univariate analysis. DECTOne-way analysis of variance (ANOVA) with Tukey’s post-hoc/nonparametric Kruskal-Wallis H test was used to analyze DECT quantitative parameters among the four molecular subtypes of breast cancer. The level of significance was defined as P<0.05.
Results
Participant characteristics
Of the 205 participants initially enrolled in this study, 75 participants were excluded because they had no or incomplete pathological information (n=23), had undergone a mass biopsy within 1 week prior to CT scanning (n=33), had a breast mass with an invisible/shortest diameter <1 cm (n=12), or had a breast mass that exceeded the field of view due to obesity (n=7). Finally, 130 participants (mean age, 53.2 ±10.01 years; age range, 32–87 years) were included in our study (Figure 1). No serious allergic events were observed. Among the 130 patients, 161 breast lesions were confirmed by pathology including 44 benign lesions and 117 malignant lesions. Of the malignant breast tumors, 86.3% (101 of 117) of participants had invasive non-special carcinoma, and 13.7% (16 of 117) of participants had DCIS. Among the patients with invasive non-special carcinoma, 22.8% (23 of 101) had the luminal A subtype, 50.4% (51 of 101) had the luminal B subtype, 12.9% (13 of 101) had the HER2-enriched subtype, and 13.9% (14 of 101) had the basal-like subtype. The demographic, clinical, and pathologic characteristics of the 130 women included in our study are shown in Table 1.
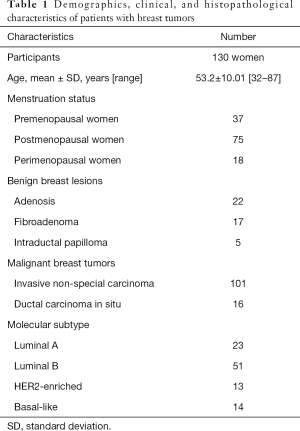
Full table
Differentiation between benign and malignant lesions of the breast
The median ICC for inter-observer variability in terms of the quantitative parameters was 0.941 (range, 0.801–0.979) for the arterial phase and 0.938 (range, 0.791–0.980) for the venous phase. The DECT quantitative parameters of benign and malignant lesions of the breast are shown in Table 2 and Figure 2, and the representative images are shown in Figure 3. In the arterial and venous phase, NICs, λHu, nZeff, and attenuation were higher in malignant lesions than in benign lesions (all P<0.001). ROC curve analysis (Table 3, Figure 4) showed that λHu in the venous phase had the best differential diagnostic capability, with an AUC of 0.90. The optimal threshold for distinguishing benign from malignant lesions of the breast was 1.740 HU/keV, which produced a sensitivity of 84.1% (37 of 44), a specificity of 86.3% (101 of 117), and an accuracy of 85.7% (138 of 161). Univariate logistic regression analysis showed that all of the DECT quantitative parameters were risk factors for predicting malignant lesions (all P<0.001, Table 4). Further multivariate logistic regression analysis (Table 4) showed that among these DECT quantitative parameters, arterial phase NIC and venous phase λHu were independent predictors of malignant lesions [arterial phase NIC odds ratio, 7.2; 95% confidence interval (CI): 1.9, 26.9; P=0.01; venous phase λHu odds ratio, 7.2; 95% CI: 1.2, 44.7; P=0.01].
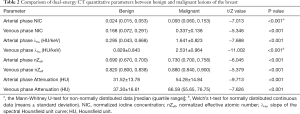
Full table
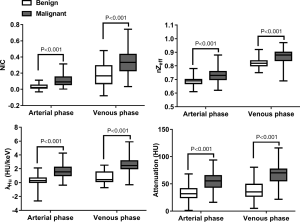
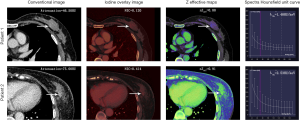
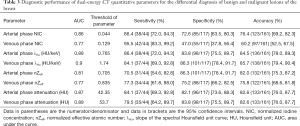
Full table
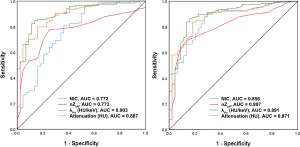
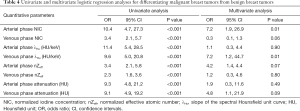
Full table
Differentiation between invasive non-special breast carcinoma and DCIS
NIC was higher in invasive non-special carcinoma than in DCIS (arterial phase, 0.113±0.066 vs. 0.037±0.039, P=0.02; venous phase, 0.353±0.137 vs. 0.237±0.075, P<0.001), as illustrated in Figure 5. The ROC curve of arterial phase NIC showed that the optimal threshold for distinguishing invasive non-carcinoma from DCIS was 0.034, which produced an AUC of 0.68, a sensitivity of 43.8% (7 of 16), a specificity of 87.1% (88 of 101), and an accuracy of 81.2% (95 of 117). Meanwhile, the ROC curve of venous phase NIC showed that the optimal threshold for distinguishing invasive non-carcinoma from DCIS was 0.285, which achieved an AUC of 0.77, a sensitivity of 75.0% (12 of 16), a specificity of 81.2% (82 of 101), and an accuracy of 80.3% (94 of 117). The combination of the arterial and venous phase NICs had an AUC of 0.77. No significant differences in arterial and venous phase λHu, nZeff, or attenuation were observed between invasive non-special carcinoma and DCIS (P=0.07–0.95).
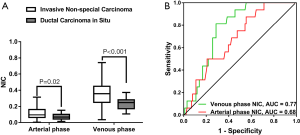
Differentiation between the luminal A and non-luminal A subtypes of invasive non-special breast carcinoma
The DECT quantitative parameters of the four molecular subtypes of breast cancer are detailed in Table 5. The luminal A subtype produced a lower venous phase NIC, and arterial and venous phase nZeffthan those in non-luminal A subtypes of invasive non-special breast carcinoma (adjusted P<0.001–0.02, Figure 6). No significant difference was observed between the luminal B, HER2-enriched, and basal-like subtypes (P=0.72–0.99). ROC curve analysis showed that nZeff in the arterial phase had the best differential diagnostic capability for distinguishing between the luminal A and non-luminal A subtypes; the optimal threshold of 0.715 corresponded to an AUC of 0.83, a sensitivity of 73.9% (17 of 23), a specificity of 78.2% (61 of 78), and an accuracy of 77.2% (78 of 101). However, the differential diagnostic capability of the combination of the three parameters (venous phase NIC, and arterial and venous phase nZeff) was better, with an AUC of 0.91, a sensitivity of 87.0% (20 of 23), a specificity of 78.2% (61 of 78), and an accuracy of 80.2% (81 of 101). No significant differences were observed in arterial phase NIC, arterial and venous phase λHu, or attenuation among the four molecular subtypes (P=0.05–0.83).
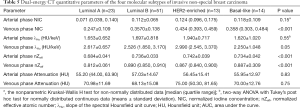
Full table
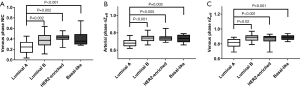
Discussion
The results of this study lend considerable support to the utility of DECT quantitative parameters for differentiating benign from malignant lesions and for predicting histopathological and molecular subtypes in women with breast cancer. These parameters were demonstrated to have high diagnostic accuracy, sensitivity, and specificity. DECT is widely used to characterize and differentiate tumors; however, at present, data on the diagnostic performance of DECT quantitative parameters for the characterization of breast tumors are limited. In this study, in the arterial and venous phase, NICs, λHu, nZeff, and attenuation were found to be higher in malignant lesions than in benign lesions (all P<0.001). Also, the NIC in both the arterial and venous phases was higher in invasive non-special carcinoma than in DCIS (both P<0.001). Compared with the non-luminal A subtype, the luminal A subtype produced a lower venous phase NIC, and arterial and venous phase nZeff (AUC of 0.91 for the combination of these three parameters). Our study showed that DECT quantitative parameters exhibit a promising diagnostic performance. It is worth noting that not all DECT quantitative parameters have high diagnostic efficiency for breast lesions differentiation.
This study found that DECT quantitative parameters are beneficial for the preoperative differentiation of benign from malignant breast lesions. In contrast-enhanced CT scans of known breast lesions, the morphology, enhancement pattern, and perfusion value can be used to differentiate benign from malignant lesions (25-27). A previous study (28) showed that vasculature remodeling or neoangiogenesis is an initiating event in the early stage of tumor development. Iodine distribution in the tissue is strongly related to local blood volume and vascular density (11). Therefore, NIC may be used as a surrogate imaging marker for assessing angiogenesis in breast cancer. λHu can provide quantitative information about tissue composition and has been shown to be able to distinguish iodine from soft tissues (29). Our findings are in line with the results of a recent study (24), which demonstrated that λHu was the single best parameter for the detection of metastatic sentinel lymph nodes in breast cancer.
According to the National Comprehension Cancer Network Guidelines (version 1.2019) for breast cancer, surgery of the sentinel lymph nodes is generally not recommended for DCIS (30). Therefore, differentiating DCIS from invasive carcinoma is crucial for clinical decision-making. A previous study (31) on the application of DECT in lung cancer reported that the NIC was higher in invasive pulmonary adenocarcinomas than in pre-invasive lesions. In our study, we found that NIC in both the arterial and venous phases was higher in invasive non-special carcinoma than in DCIS, which is consistent with the results of the most recently reported study (22). One interpretation of this result may be that invasive non-special carcinoma entails more underlying microvasculature and tumor angiogenesis than DCIS, which increases blood flow within the tumor (32), leading to a faster time to peak enhancement.
Presently, breast cancer is classified into molecular subtypes with distinct behaviors, responses to treatment, and outcomes. Neoadjuvant chemotherapy is less effective against luminal subtypes (hormone receptor+, HER2−), such as the luminal A subtype, than against other subtypes, despite the better overall prognosis and less recurrent (33). Thus, identifying the luminal A subtype is crucial for decision-making in clinical treatment. In our study, we found that the luminal A and non-luminal A subtypes can be distinguished by venous phase NIC, as well as arterial and venous phase nZeff. nZeff is a quantitative indicator of the compound atom of a compound or a mixture of various materials. So far, the exact clinical significance of nZeff is not clear, and nZeff can be used to distinguish between luminal A and non-luminal A subtypes in breast cancer has not been well established in previous studies.
Our study had some limitations that should be noted. Firstly, the participants were selected from a group with high suspicion of breast cancer at a single institution, which may have introduced bias, and fewer participants with benign tumors were included. Secondly, due to the short duration of this study and the low incidence of special pathological types of tumors, not all histopathological types of breast tumors were included. Thirdly, we excluded breast lesions with a shortest diameter <1 cm, mainly due to the fact that such size tumors are too small and the boundary is not clear, which may have affected the accurate delineation of the ROI. Fourthly, the sample size of this study is relatively small. Although the size of our study population assured reliability to interpret the outcomes according to the sample size calculation, large sample research is still needed to improve the statistical power in future studies. Finally, we could not distinguish between the luminal B, HER2-enriched, and basal-like subtypes using DECT quantitative parameters. Many studies (34,35) have shown that machine learning can significantly improve diagnostic efficiency. We hope to realize the application of machine learning after recruiting more participants in the future.
In conclusion, DECT quantitative parameters are a feasible and valuable noninvasive means of differentiating between benign and malignant lesions and predicting histopathological and molecular subtypes in patients with breast cancer. Further work is needed to validate our results in a larger prospective cohort.
Acknowledgments
The authors thank all volunteers who participated in the study and the staff of the Department of Radiology, Chongqing University Cancer Hospital, China, for their selfless and valuable assistance. We acknowledge the support of Xiaoyue Zhang from Siemens scientific research.
Funding: This study has received funding from the National Natural Science Foundation of China (Grant No. 82071883), the Combination Projects of Medicine and Engineering of the Fundamental Research Funds for the Central Universities in 2019 (Project No. 2019CDYGYB008), the Chongqing Key Medical Research Project of Combination of Science and Medicine (Grant No. 2019ZDXM007), and the 2019 SKY Imaging Research Fund of the Chinese International Medical Foundation (Project No. Z-2014-07-1912-10).
Footnote
Conflicts of Interest: All authors have completed the ICMJE uniform disclosure form (available at http://dx.doi.org/10.21037/qims-20-825). The authors have no conflicts of interest to declare.
Ethical Statement: This study was approved by the ethics committee of the Chongqing University Cancer Hospital (No. CZLS20200215-A), and informed consent was obtained from all patients. The authors are accountable for all aspects of the work in ensuring that questions related to the accuracy or integrity of any part of the work are appropriately investigated and resolved.
Open Access Statement: This is an Open Access article distributed in accordance with the Creative Commons Attribution-NonCommercial-NoDerivs 4.0 International License (CC BY-NC-ND 4.0), which permits the non-commercial replication and distribution of the article with the strict proviso that no changes or edits are made and the original work is properly cited (including links to both the formal publication through the relevant DOI and the license). See: https://creativecommons.org/licenses/by-nc-nd/4.0/.
References
- Haynes B, Sarma A, Nangia-Makker P, Shekhar MP. Breast cancer complexity: implications of intratumoral heterogeneity in clinical management. Cancer Metastasis Rev 2017;36:547-55. [Crossref] [PubMed]
- Cutuli B, Bernier J, Poortmans P. Radiotherapy in DCIS, an underestimated benefit? Radiother Oncol 2014;112:1-8. [Crossref] [PubMed]
- Arvold ND, Taghian AG, Niemierko A, Abi Raad RF, Sreedhara M, Nguyen PL, Bellon JR, Wong JS, Smith BL, Harris JR. Age, breast cancer subtype approximation, and local recurrence after breast-conserving therapy. J Clin Oncol 2011;29:3885-91. [Crossref] [PubMed]
- Huo D, Hu H, Rhie SK, Gamazon ER, Cherniack AD, Liu J, Yoshimatsu TF, Pitt JJ, Hoadley KA, Troester M, Ru Y, Lichtenberg T, Sturtz LA, Shelley CS, Benz CC, Mills GB, Laird PW, Shriver CD, Perou CM, Olopade OI. Comparison of Breast Cancer Molecular Features and Survival by African and European Ancestry in The Cancer Genome Atlas. JAMA Oncol 2017;3:1654-62. [Crossref] [PubMed]
- Suvannarerg V, Chitchumnong P, Apiwat W, Lertdamrongdej L, Tretipwanit N, Pisarnturakit P, Sitthinamsuwan P, Thiravit S, Muangsomboon K, Korpraphong P. Diagnostic performance of qualitative and quantitative shear wave elastography in differentiating malignant from benign breast masses, and association with the histological prognostic factors. Quant Imaging Med Surg 2019;9:386-98. [Crossref] [PubMed]
- Lehman CD, Isaacs C, Schnall MD, Pisano ED, Ascher SM, Weatherall PT, Bluemke DA, Bowen DJ, Marcom PK, Armstrong DK, Domchek SM, Tomlinson G, Skates SJ, Gatsonis C. Cancer yield of mammography, MR, and US in high-risk women: prospective multi-institution breast cancer screening study. Radiology 2007;244:381-8. [Crossref] [PubMed]
- Berg WA, Gutierrez L. NessAiver MS, Carter WB, Bhargavan M, Lewis RS, Ioffe OB. Diagnostic accuracy of mammography, clinical examination, US, and MR imaging in preoperative assessment of breast cancer. Radiology 2004;233:830-49. [Crossref] [PubMed]
- Zhang X, Wang D, Liu Z, Wang Z, Li Q, Xu H, Zhang B, Liu T, Jin F. The diagnostic accuracy of magnetic resonance imaging in predicting pathologic complete response after neoadjuvant chemotherapy in patients with different molecular subtypes of breast cancer. Quant Imaging Med Surg 2020;10:197-210. [Crossref] [PubMed]
- Brennan ME, Houssami N, Lord S, Macaskill P, Irwig L, Dixon JM, Warren RM, Ciatto S. Magnetic resonance imaging screening of the contralateral breast in women with newly diagnosed breast cancer: systematic review and meta-analysis of incremental cancer detection and impact on surgical management. J Clin Oncol 2009;27:5640-9. [Crossref] [PubMed]
- Kerlikowske K, Zhu W, Tosteson A, Sprague B, Tice J, Lehman C, Miglioretti D. Identifying women with dense breasts at high risk for interval cancer: a cohort study. Ann Intern Med 2015;162:673-81. [Crossref] [PubMed]
- Simons D, Kachelriess M, Schlemmer HP. Recent developments of dual-energy CT in oncology. Eur Radiol 2014;24:930-9. [Crossref] [PubMed]
- Srinivasan A, Parker RA, Manjunathan A, Ibrahim M, Shah GV, Mukherji SK. Differentiation of benign and malignant neck pathologies: preliminary experience using spectral computed tomography. J Comput Assist Tomogr 2013;37:666-72. [Crossref] [PubMed]
- Tawfik AM, Razek AA, Kerl JM, Nour-Eldin NE, Bauer R, Vogl TJ. Comparison of dual-energy CT-derived iodine content and iodine overlay of normal, inflammatory and metastatic squamous cell carcinoma cervical lymph nodes. Eur Radiol 2014;24:574-80. [Crossref] [PubMed]
- Deniffel D, Sauter A, Dangelmaier J, Fingerle A, Rummeny EJ, Pfeiffer D. Differentiating intrapulmonary metastases from different primary tumors via quantitative dual-energy CT based iodine concentration and conventional CT attenuation. Eur J Radiol 2019;111:6-13. [Crossref] [PubMed]
- Kaltenbach B, Wichmann JL, Pfeifer S, Albrecht MH, Booz C, Lenga L, Hammerstingl R, D'Angelo T, Vogl TJ, Martin SS. Iodine quantification to distinguish hepatic neuroendocrine tumor metastasis from hepatocellular carcinoma at dual-source dual-energy liver CT. Eur J Radiol 2018;105:20-4. [Crossref] [PubMed]
- Udare A, Walker D, Krishna S, Chatelain R, McInnes MD, Flood TA, Schieda N. Characterization of clear cell renal cell carcinoma and other renal tumors: evaluation of dual-energy CT using material-specific iodine and fat imaging. Eur Radiol 2020;30:2091-102. [Crossref] [PubMed]
- Li M, Zhang L, Tang W, Ma PQ, Zhou LN, Jin YJ, Qi LL, Wu N. Quantitative features of dual-energy spectral computed tomography for solid lung adenocarcinoma with EGFR and KRAS mutations, and ALK rearrangement: a preliminary study. Transl Lung Cancer Res 2019;8:401-12. [Crossref] [PubMed]
- Okada K, Matsuda M, Tsuda T, Kido T, Murata A, Nishiyama H, Nishiyama K, Yamasawa H, Kamei Y, Kurata M, Fukushima M, Kitazawa R, Mochizuki T. Dual-energy computed tomography for evaluation of breast cancer: value of virtual monoenergetic images reconstructed with a noise-reduced monoenergetic reconstruction algorithm. Jpn J Radiol 2020;38:154-64. [Crossref] [PubMed]
- Metin Y, Metin NO, Ozdemir O, Tasci F, Kul S. The role of low keV virtual monochromatic imaging in increasing the conspicuity of primary breast cancer in dual-energy spectral thoracic CT examination for staging purposes. Acta Radiol 2020;61:168-74. [Crossref] [PubMed]
- Bandaru RC, Rachegowda N. Efficacy Of ultrasonography and computed tomography in differentiating transudate from exudate in patients with pleural effusion. Imaging in Medicine 2018;10. [Crossref]
- Okamura Y, Yoshizawa N, Yamaguchi M, Kashiwakura I. Application of Dual-Energy Computed Tomography for Breast Cancer Diagnosis. International Journal of Medical Physics Clinical Engineering & Radiation Oncology 2016;05:288-97. [Crossref]
- Volterrani L, Gentili F, Fausto A, Pelini V, Megha T, Sardanelli F, Mazzei MA. Dual-Energy CT for Locoregional Staging of Breast Cancer: Preliminary Results. AJR Am J Roentgenol 2020;214:707-14. [Crossref] [PubMed]
- Goldhirsch A, Winer EP, Coates AS, Gelber RD, Piccart-Gebhart M, Thurlimann B, Senn HJ. Panel m. Personalizing the treatment of women with early breast cancer: highlights of the St Gallen International Expert Consensus on the Primary Therapy of Early Breast Cancer 2013. Ann Oncol 2013;24:2206-23. [Crossref] [PubMed]
- Zhang X, Zheng C, Yang Z, Cheng Z, Deng H, Chen M, Duan X, Mao J, Shen J. Axillary Sentinel Lymph Nodes in Breast Cancer: Quantitative Evaluation at Dual-Energy CT. Radiology 2018;289:337-46. [Crossref] [PubMed]
- Lin YP, Hsu HH, Ko KH, Chu CM, Chou YC, Chang WC, Chang TH. Differentiation of Malignant and Benign Incidental Breast Lesions Detected by Chest Multidetector-Row Computed Tomography: Added Value of Quantitative Enhancement Analysis. PLoS One 2016;11:e0154569. [Crossref] [PubMed]
- Prionas ND, Lindfors KK, Ray S, Huang SY, Beckett LA, Monsky WL, Boone JM. Contrast-enhanced dedicated breast CT: initial clinical experience. Radiology 2010;256:714-23. [Crossref] [PubMed]
- Cheng YG, Sun ZQ, Zhang HX, Mao GQ. An application study of low-dose computed tomography perfusion imaging (LDCTPI) in breast cancer and breast fibroadenoma. Journal of X-Ray Science and Technology 2018;26:681-90. [Crossref] [PubMed]
- Gao F, Li M, Ge X, Zheng X, Ren Q, Chen Y, Lv F, Hua Y. Multi-detector spiral CT study of the relationships between pulmonary ground-glass nodules and blood vessels. Eur Radiol 2013;23:3271-7. [Crossref] [PubMed]
- Boll DT, Patil NA, Paulson EK, Merkle EM, Nelson RC, Schindera ST, Roessl E, Martens G, Proksa R, Fleiter TR, Schlomka JP. Focal cystic high-attenuation lesions: characterization in renal phantom by using photon-counting spectral CT--improved differentiation of lesion composition. Radiology 2010;254:270-6. [Crossref] [PubMed]
- Jakub JW, Murphy BL, Gonzalez AB, Conners AL, Henrichsen TL, Maimone St, Keeney MG, McLaughlin SA, Pockaj BA, Chen B, Musonza T, Harmsen WS, Boughey JC, Hieken TJ, Habermann EB, Shah HN, Degnim AC. A Validated Nomogram to Predict Upstaging of Ductal Carcinoma in Situ to Invasive Disease. Ann Surg Oncol 2017;24:2915-24. [Crossref] [PubMed]
- Yang Y, Li K, Sun D, Yu J, Cai Z, Cao Y, Wu J. Invasive Pulmonary Adenocarcinomas Versus Preinvasive Lesions Appearing as Pure Ground-Glass Nodules: Differentiation Using Enhanced Dual-Source Dual-Energy CT. American Journal of Roentgenology 2019;213:W114-22. [Crossref] [PubMed]
- Li Y, Yang Z, Chen T, Chen H, Sun J, Lu Y. Peripheral lung carcinoma: Correlation of angiogenesis and first-pass perfusion parameters of 64-detector row CT. Lung Cancer 2008;61:44-53. [Crossref] [PubMed]
- Boughey JC, McCall LM, Ballman KV, Mittendorf EA, Ahrendt GM, Wilke LG, Taback B, Leitch AM, Flippo-Morton T, Hunt KK. Tumor biology correlates with rates of breast-conserving surgery and pathologic complete response after neoadjuvant chemotherapy for breast cancer: findings from the ACOSOG Z1071 (Alliance) Prospective Multicenter Clinical Trial. Ann Surg 2014;260:608-14; discussion 614-6. [Crossref] [PubMed]
- Reig B, Heacock L, Geras KJ, Moy L. Machine learning in breast MRI. J Magn Reson Imaging 2020;52:998-1018. [Crossref] [PubMed]
- Park EK, Lee KS, Seo BK, Cho KR, Woo OH, Son GS, Lee HY, Chang YW. Machine Learning Approaches to Radiogenomics of Breast Cancer using Low-Dose Perfusion Computed Tomography: Predicting Prognostic Biomarkers and Molecular Subtypes. Sci Rep 2019;9:17847. [Crossref] [PubMed]