The application value of a vendor-specific deep learning image reconstruction algorithm in “triple low” head and neck computed tomography angiography
Introduction
The head and neck computed tomography angiography (CTA) is a noninvasive imaging modality that can clearly show the arterial vessels and their branches originating from the aortic arch (AA) to the head and is thus often used to evaluate vascular stenosis, occlusion, aneurysm, calcification of the head and neck arteries, or stroke (1,2). Despite its excellent capability to display anatomical structures, there remain concerns about radiation safety and contrast nephropathy (3). Therefore, reducing the radiation dose, contrast dosage, and injection rate while maintaining the image quality have attracted a widespread research attention. Use of low-voltage techniques is one of the most effective means to achieving the “triple low” (low radiation dose, low contrast dosage, and low contrast injection rate) target in CTA. Due to the poorer penetration ability of low-energy X-rays, low-voltage computed tomography (CT) results in greater noise in images. Iterative reconstruction algorithms, such as volume-based adaptive statistical iterative reconstruction (ASIR-V) (4), have been developed to reconstruct images in low-voltage scans to reduce image noise (5,6). However, images reconstructed by iterative algorithms often show texture degradation and excessive smoothing when the strength of the iterative reconstruction is significantly increased (7-9); this may increase the risk of missed diagnosis, thus reducing the potential of iterative algorithms in facilitating further dose optimization in clinical applications.
Recently, image reconstruction algorithms based on the deep convolutional neural networks, such as deep learning image reconstruction (DLIR), which can improve the spatial resolution and detection capability without the loss of noise texture (10,11), have been introduced and gradually applied in clinical research. Previous studies have found that DLIR is effective in improving the detectability of low-contrast and small-diameter lesions (12-15) under low-dose conditions in different clinical CTA applications. In this study, we extended the clinical application of DLIR to the head and neck. Specifically, we aimed to evaluate the ability of DLIR combined with a low-tube voltage technique to reduce the radiation dose, contrast dosage, and contrast injection rate in head and neck CTA. We present this article in accordance with the STROBE reporting checklist (available at https://qims.amegroups.com/article/view/10.21037/qims-23-1602/rc).
Methods
Phantom experiment
To set an appropriate contrast dose reduction percentage in clinical application, a phantom experiment was first conducted. In this experiment, the contrast (iohexol, 350 mgI/mL; GE HealthCare, Shanghai, China) was diluted into solutions of different concentrations using saline (6, 8, 10, 12, 14, 16, 18, 20, and 22 mgI/mL) and sealed in plastic containers, which were numbered in the order of concentration from lowest to highest (i.e., 1–9). The nine plastic containers were fixed counterclockwise in a cylindrical phantom with a diameter of 20 cm and scanned with a 256-row CT scanner (Revolution Apex CT, GE HealthCare) at different tube voltages (70, 80, 100, and 120 kVp), and the images were reconstructed using ASIR-V at a level of 50% (ASIR-V50%), as shown in Figure 1. Additional scanning parameters can be found in Table S1. Circular regions of interest (ROIs; area 50 mm2) concentric with each plastic container were placed to measure the CT values in the cylinders to analyze the relationship between the CT values of different concentrations and tube voltages.
Clinical study
Participants
This prospective study was approved by the Ethics Committee of Taizhou Hospital of Zhejiang Province (No. K202306136) and was conducted in accordance with the Declaration of Helsinki (as revised in 2013). Informed consent form was obtained from each patient. This study prospectively and consecutively recruited adult patients requiring head and neck CTA in Taizhou Hospital of Zhejiang Province from December 2022 to March 2023. Patients were excluded if they met any of the following criteria: (I) body mass index (BMI) ≤18 or ≥25 kg/m2 and (II) allergy to iodine contrast agents or with renal insufficiency. Finally, 100 patients were enrolled in the study (Figure 2) and divided randomly and evenly into two groups.
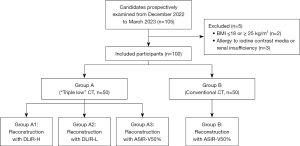
Imaging examination
The scans of all patients were completed on a 256-row CT scanner (Revolution Apex CT, GE Healthcare). The scan parameters are listed in Table 1. CT scans were performed in the natural supine position, with patients maintaining calm breathing. The scan ranged from the AA to the top of the skull, and the bolus-tracking technique was used, with the ROI set in the descending aorta (ROI area 15–20 mm2) under a trigger threshold of 100 Hounsfield units (HU) and a delay time of 3 s. Nonionic contrast agent was injected with a 20-G intravenous needle and a high-pressure syringe (CT Motion, Ulrich Medical, Shanghai, China) through the right median cubital vein. The total volume of contrast injection in group A (low-dose group) was determined as follows: contrast injection volume (mL) = body weight (kg) ×0.5 mL/kg. The injection rate (mL/s) was calculated as the total volume (mL) divided by 8 (s). Meanwhile, the total volume of contrast injection (mL) in group B (conventional group) was calculated as follows: body weight (kg) ×0.8 mL/kg. The injection rate was 4–5 mL/s.
Table 1
Parameters | Group A | Group B |
---|---|---|
Tube voltage (kVp) | 70 | 100 |
Tube current (mA) | 100–1,300* | 100–1,300* |
Noise index | 6 | 6 |
Rotation time (seconds) | 0.5 | 0.5 |
Detector collimation (mm) | 128×0.625 | 128×0.625 |
Slice thickness/interval (mm) | 0.625/0.625 | 0.625/0.625 |
Pitch | 0.992:1 | 0.992:1 |
*, automatic tube current modulation. Group A, triple-low group; Group B, conventional group.
Images in group A were reconstructed with DLIR at high weighting (DLIR-H), DLIR at low weighting (DLIR-L), and ASIR-V50%, which were noted as A1, A2, and A3, respectively. Images in group B were reconstructed with ASIR-V50%. All images were transferred to an Advantage Workstation 4.7 (GE Healthcare) for generation of maximal intensity projection (MIP), multi planar reformation (MPR), curved planar reformation (CPR), and volume rendering (VR) images.
Image quality evaluation
The CT attenuations and standard deviations (SDs) of the sternocleidomastoid muscle (SCM) at the level of hypopharynx and epiglottis and the cerebral white matter (WM) at the level of splenium of corpus callosum were measured using ROIs whose areas were 10 and 40 mm2, respectively, with the SDs of the SCM and WM were taken as the background noise of the neck and head images, respectively. In addition, ROIs with the areas of 50, 10, 2, 2, and 1 mm2 were placed on the blood vessels of the AA, beginning of the internal carotid artery (ICA; ICA-C1), equivalent vertebral artery (VA), ICA-C4, and the M1 of middle cerebral artery (MCA-M1), respectively, to measure their CT attenuations and SDs, with any vascular plaque being avoided. The signal-to-noise ratio (SNR) was calculated as follows: SNR = CTvessel/SDvessel. Meanwhile, the contrast-to-noise ratio (CNR) of the head (CNRhead) and neck (CNRneck) was calculated, respectively, as follows: CNRhead = (CTvessel − CTWM)/SDWM; CNRneck = (CTvessel − CTSCM)/SDSCM.
The sharpness of images was evaluated with the edge rise slope (ERS) (16-18) at ICA-C1 (neck) and ICA-C4 (head) running in the axial plane. ERS was defined as the ratio of the CT value difference between the last dip and the first peak on the rapid rising CT value curve divided by the distance between the two points (Figure S1). Larger ERSs indicated sharper edges. The start point of the CT value curve was set to the center of the vessel, and the end point was set at a low CT value outside the vessel. Measurements were performed using code written in Python 3 (Python Software Foundation).
The subjective quality of images was assessed blindly by two radiologists (one senior radiologist with 10 years of experience and one junior radiologist with 5 years of experience in imaging the head and neck). The images were evaluated on a five-point Likert scale as follows: 2–5 points = acceptable image mass fractions; 5= excellent image quality, clear anatomical details, sharp vessel edges with high contrast, and no artifacts; 4= very good image quality, relatively clear anatomical structures and details, smooth vessel edges with high contrast, and slight artifacts; 3= good image quality, majority of clear anatomical structures, smoother vessel edges with moderate contrast, and moderate artifacts; 2= moderate image quality, majority of anatomical structures deemed diagnostically adequate with poor contrast and moderate artifacts; and 1= poor image quality, unclear anatomical structures, anatomical detail insufficient for detection, poor contrast, and severe artifacts. In cases of disagreement, the final score in was negotiated between the two readers.
Radiation dose
The CT dose index volume (CTDIvol; mGy) was used to reflect the slice-averaged dose over the entire spiral scanning. The dose-length product (DLP; mGy·cm) was used to evaluate the total radiation dose received by the participant after undergoing a single CT exposure scan. DLP is calculated as follows: DLP = CTDIvol × L (with L being the length of the scan along the Z-axis). CTDIvol and DLP were recorded by the scanner after each scan. The effective radiation dose (ED; mSv) was defined as the product of the DLP and a conversion factor of 0.0031 mSv/(mGy·cm) (19) for the adult head and neck. Both DLP and ED were used to assess the radiation dose of each patient.
Statistical analysis
All data in this study were analyzed using the SPSS version 26.0 statistical software (IBM Corp., Armonk, New York, USA). Continuous variables are expressed as the mean ± SD and were tested for normality using the Shapiro-Wilk test. Nonparametric variables are expressed as the median with interquartile range or via frequency distribution tables. Normally distributed continuous variables were compared using one-way analysis of variance with least significant difference (LSD) correction (four-group comparison) and the Student’s t-test (four-group comparison). Nonnormally distributed continuous and nonparametric variables were compared using the Kruskal-Wallis one-way analysis of variance (ANOVA) test with Bonferroni correction (four-group comparison) and the Mann-Whitney test (two-group comparison). The Fisher exact test was used to compare categorical variables. The kappa statistic was used to test the interrater consistency (a κ value over 0.8 was considered excellent, between 0.61 and 0.8 was considered good, and less than 0.6 was considered poor). A P value less than 0.05 was considered statistically significant. The sample size in this study was calculated with G*Power software (20).
Results
Phantom study
Figure 3 shows the distribution curves of CT values at different tube voltages with different concentrations of contrast agent. Under the same contrast concentration, the CT value decreased with the increase of tube voltage; under the same tube voltage, the CT value increased with the increase of contrast concentration.
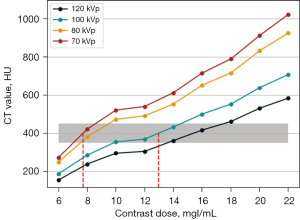
The CT value after enhancement is required to be in the range of 350–450 HU for routine diagnostic needs, and in this study, the median (400 HU) was chosen as the reference value. The contrast concentration to satisfy a CT value of 400 HU calculated by linear interpolation at 70 kVp was 7.71 mgI/mL, and the corresponding contrast concentration at 100 kVp was 12.96 mgI/mL (the red dashed line in Figure 3). Therefore, for a CT value of 400 HU, the contrast agent concentration required at 70 kVp was about 60% that at 100 kVp. This ratio was used to select the contrast injection protocol in the clinical study.
Clinical study
Participants
A total of 100 patients were included, 53 of whom were male and 47 were female, with a mean age of 65.74±13.39 years, body weight of 56.69±6.62 kg, and BMI of 21.77±1.79 kg/m2. There was no difference in patient age, BMI, or gender between the two patient groups (age: A vs. B, P=0.31; BMI: A vs. B, P=0.46; gender: A vs. B, P=0.40) (Table 2). A total of 44 patients had stenosis, including 11 with mild stenosis, 12 with moderate stenosis, 11 with severe stenosis, and 10 with vascular occlusion, and there was no statistically significant difference in stenosis between the two groups (P=0.39). Compared with group B, who underwent the standard dose protocol, group A showed significant reductions in contrast dosage, injection rate, and radiation of 36.09%, 20.88%, and 47.80%, respectively (contrast dosage: A vs. B, P<0.001; injection rate: A vs. B, P<0.001; radiation: A vs. B, P<0.001) (Table 2).
Table 2
Patient characteristics | Group A | Group B | P value |
---|---|---|---|
Age (years) | 66.72±12.55 | 64.76±14.25 | 0.31 |
Gender (male:female) | 26:24 | 27:23 | 0.40 |
Weight (kg) | 56.91±8.04 | 56.26±4.89 | 0.38 |
BMI (kg/m2) | 21.80±2.07 | 21.73±1.47 | 0.46 |
Contrast dosage (mL) | 28.76±4.00 | 45.00±3.83 | <0.001 |
Injection rate (mL/s) | 3.60±0.50 | 4.55±0.36 | <0.001 |
CTDIvol (mGy) | 5.70±0.41 | 10.85±0.62 | <0.001 |
DLP (mGy·cm) | 230.52±23.35 | 437.31±34.90 | <0.001 |
ED (mSv) | 0.71±0.07 | 1.36±0.11 | <0.001 |
Arterial stenosis | 0.39 | ||
Not narrowed | 27 | 29 | |
Mild stenosis | 3 | 8 | |
Moderate stenosis | 6 | 6 | |
Severe stenosis | 9 | 2 | |
Occlusion | 5 | 5 |
Data are presented as mean ± standard deviation or number. Group A: triple-low group; Group B: conventional group. BMI, body mass index; CTDIvol, computed tomography dose index volume; DLP, dose-length product; ED, effective dose.
Objective evaluation
The degree of noise differed significantly across the four reconstruction image groups (SCM: P<0.001; WM: P<0.001), with the lowest being in group A, followed by groups B, A2, and A3 (Figure 4). There were statistically significant differences between any two groups on a variety of noise measures (SDA1-SCMvs. SDA2-SCM, P<0.001; SDA1-SCMvs. SDA3-SCM, P<0.001; SDA1-SCMvs. SDB-SCM, P<0.001; SDA2-SCMvs. SDA3-SCM, P<0.001; SDA2-SCMvs. SDB-SCM, P=0.045; SDA3-SCMvs. SDB-SCM, P<0.001; SDA1-WMvs. SDA2-WM, P<0.001; SDA1-WMvs. SDA3-WM, P<0.001; SDA1-WMvs. SDB-WM, P<0.001; SDA2-WMvs. SDA3-WM, P<0.001; SDA2-WMvs. SDB-WM, P<0.001; SDA3-WMvs. SDB-WM, P=0.002). In the neck, the image noise in group A1 was 35.3%, 39.1%, and 28.7% lower than that of groups A2, A3, and B, respectively; meanwhile, for the head, the noise was 29.9%, 32.9%, and 21.0%, lower, respectively.
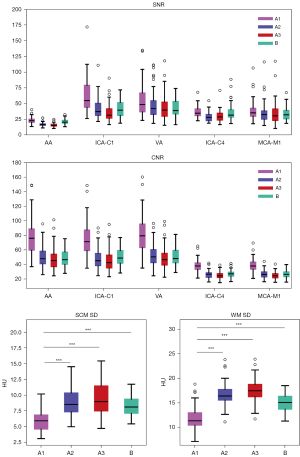
CNRs were highest in group A1, with all vessels showing statistically significant differences from the other groups (CNRA1-AAvs. CNRA2-AA, P<0.001; CNRA1-AAvs. CNRA3-AA, P<0.001; CNRA1-AAvs. CNRB-AA, P<0.001; CNRA1-ICA-C1vs. CNRA2-ICA-C1, P<0.001; CNRA1-ICA-C1vs. CNRA3-ICA-C1, P<0.001; CNRA1-ICA-C1vs. CNRB-ICA-C1, P<0.001; CNRA1-VAvs. CNRA2-VA, P<0.001; CNRA1-VAvs. CNRA3-VA, P<0.001; CNRA1-VAvs. CNRB-VA, P<0.001; CNRA1-ICA-C4vs. CNRA2-ICA-C4, P<0.001; CNRA1-ICA-C4vs. CNRA3-ICA-C4, P<0.001; CNRA1-ICA-C4vs. CNRB-ICA-C4, P<0.001; CNRA1-MCA-M1vs. CNRA2-MCA-M1, P<0.001; CNRA1-MCA-M1vs. CNRA3-MCA-M1, P<0.001; CNRA1-MCA-M1vs. CNRB-MCA-M1, P<0.001). All CNRs in group A2 were significantly higher than those in group A3 (CNRA2-AAvs. CNRA3-AA, P<0.001; CNRA2-ICA-C1vs. CNRA3-ICA-C1, P<0.001; CNRA2-VAvs. CNRA3-VA, P<0.001; CNRA2-ICA-C4vs. CNRA3-ICA-C4, P<0.001; CNRA2-MCA-M1vs. CNRA3-MCA-M1, P<0.001), but none were statistically different from those in group B (CNRA2-AAvs. CNRB-AA, P=0.68; CNRA2-ICA-C1vs. CNRB-ICA-C1, P=0.39; CNRA2-VAvs. CNRB-VA, P=0.52; CNRA2-ICA-C4vs. CNRB-ICA-C4, P=0.35; CNRA2-MCA-M1vs. CNRB-MCA-MI, P=0.95). SNRs were highest in group A1 for all vessels, except for MCA-M1, and were statistically different from those in group A2 and group A3 (SNRA1-AAvs. SNRA2-AA, P<0.001; SNRA1-AAvs. SNRA3-AA, P<0.001; SNRA1-ICA-C1vs. SNRA2-ICA-C1, P<0.001; SNRA1-ICA-C1vs. SNRA3-ICA-C1, P<0.001; SNRA1-VAvs. SNRA2-VA, P<0.001; SNRA1-VAvs. SNRA3-VA, P<0.001; SNRA1-ICA-C4vs. SNRA2-ICA-C4, P<0.001; SNRA1-ICA-C4vs. SNRA3-ICA-C4, P<0.001).
ERSs in the head and neck were statistically different among the four groups (ICA-C1: P<0.001; ICA-C4: P=0.001), with the ERSs declining from largest to the smallest in the A2, A1, A3, and B groups, respectively. There was no statistically significant difference in ERS among the A1, A2, and A3 groups (ERSA1-ICA-C1vs. ERSA2-ICA-C1, P=0.78; ERSA1-ICA-C1vs. ERSA3-ICA-C1, P=0.58; ERSA2-ICA-C1vs. ERSA3-ICA-C1, P=0.40; ERSA1-ICA-C4vs. ERSA2-ICA-C4, P=0.76; ERSA1-ICA-C4vs. ERSA3-ICA-C4, P=0.59; ERSA2-ICA-C4vs. ERSA3-ICA-C4, P=0.39), whereas these groups all had significantly higher ERSs compared to group B (ERSA1-ICA-C1vs. ERSB-ICA-C1, P<0.001; ERSA2-ICA-C1vs. ERSB-ICA-C1, P<0.001; ERSA3-ICA-C1vs. ERSB-ICA-C1, P=0.001; ERSA1-ICA-C4vs. ERSB-ICA-C4, P=0.001; ERSA2-ICA-C4vs. ERSB-ICA-C4, P<0.001; ERSA3-ICA-C4vs. ERSB-ICA-C4, P=0.004), as shown in Table 3.
Table 3
Locations | A1 | A2 | A3 | B | P value |
---|---|---|---|---|---|
CT (HU) | |||||
AA | 487.52±65.13b,d | 487.77±65.09a,d | 487.57±64.95d | 450.46±55.03a,b,c | 0.005 |
ICA-C1 | 470.41±63.98 | 469.92±63.94 | 469.37±64.12 | 461.49±60.56 | 0.88 |
VA | 506.95±74.56b,c,d | 505.66±74.40a,c,d | 497.10±73.90a,b,d | 463.32±62.79a,b,c | 0.01 |
ICA-C4 | 475.62±68.32c,d | 475.52±68.22c,d | 468.82±66.66a,b,d | 439.28±62.20a,b,c | 0.02 |
MCA-M1 | 484.28±71.68c,d | 483.59±71.64c,d | 470.42±71.77a,b,d | 432.10±63.01a,b,c | <0.001 |
SD | |||||
SCM | 5.84±1.71b,c,d | 9.02±2.45a,c,d | 9.58±2.72a,b,d | 8.19±1.53a,b,c | <0.001 |
WM | 11.82±2.37b,c,d | 16.87±2.39a,c,d | 17.62±2.41a,b,d | 14.96±1.92a,b,c | <0.001 |
SNR | |||||
AA | 23.22±5.29b,c | 16.98±3.26a,c,d | 15.87±2.91a,b,d | 21.72±4.17b,c | <0.001 |
ICA-C1 | 63.50±26.81b,c,d | 43.47±17.53a,c | 36.66±15.00a,b | 41.64±12.85a | <0.001 |
VA | 55.28±26.29b,c,d | 47.55±23.44a | 45.69±22.23a | 43.19±13.85a | 0.04 |
ICA-C4 | 36.15±8.98b,c | 28.93±7.31a,d | 29.81±9.08a,d | 35.78±13.76b,c | <0.001 |
MCA-M1 | 38.67±15.96 | 34.52±17.36 | 34.98±19.31 | 34.43±11.30 | 0.51 |
CNR | |||||
AA | 78.26±23.98b,c,d | 51.62±15.52a,c | 47.71±15.43a,b | 49.05±11.55a | <0.001 |
ICA-C1 | 75.18±23.30b,c,d | 48.16±14.31a,c | 45.76±14.94a,b | 50.41±11.81a | <0.001 |
VA | 82.03±25.88b,c,d | 52.44±15.88a,c | 48.94±16.28a,b | 50.62±12.04a | <0.001 |
ICA-C4 | 37.80±8.89b,c,d | 26.07±5.28a,c | 24.60±5.05a,b,d | 27.10±5.55a,c | <0.001 |
MCA-M1 | 38.53±9.04b,c,d | 26.52±5.27a,c | 24.64±5.02a,b | 26.59±5.43a | <0.001 |
ERS | |||||
ICA-C1 | 59.89±11.57d | 60.53±11.73d | 58.49±11.35d | 49.76±12.69a,b,c | <0.001 |
ICA-C4 | 60.13±12.01d | 60.91±12.04d | 58.78±11.57d | 51.49±13.59a,b,c | 0.001 |
Data are presented as mean ± standard deviation. a, statistical significance with A1, P<0.05; b, statistical significance with A2, P<0.05; c, statistical significance with A3, P<0.05; d, statistical significance with B, P<0.05. A1: triple-low group with DLIR-H; A2: triple-low group with DLIR-L; A3: triple-low group with ASIR-V50%; B: conventional group with ASIR-V50%. CT, computed tomography; HU, Hounsfield units; AA, aortic arch; ICA-C1, internal carotid artery beginning segment; VA, vertebral artery; ICA-C4, internal carotid artery C4 segment; MCA-M1, middle cerebral artery M1 segment; SD, standard deviation; SCM, sternocleidomastoid muscle; WM, white matter; SNR, signal-to-noise ratio; CNR, contrast-to-noise ratio; ERS, edge rising slope; DLIR, deep learning image reconstruction; DLIR-H, DLIR at high weighting; DLIR-L, DLIR at low weighting; ASIR-V50%, volume-based adaptive statistical iterative reconstruction with 50% weighting.
Subjective evaluation
The highest subjective image quality score (SIQS) was found in group A1, followed by A2, B, and A3 (Figure 5). Statistical differences in SIQS were found between all groups (SIQSA1vs. SIQSA2, P<0.001; SIQSA1vs. SIQSA3, P<0.001; SIQSA1vs. SIQSB, P<0.001; SIQSA2vs. SIQSA3, P=0.001; SIQSA2vs. SIQSB, P=0.02) except between A3 and B. The κ values ranged from 0.88 to 1, indicating excellent agreement between the two readers (Table 4 and Figure 6).
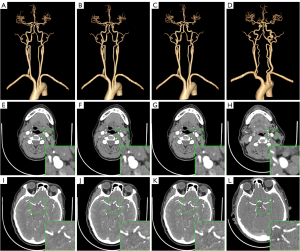
Table 4
SIQS | A1 | A2 | A3 | B | |||||||
---|---|---|---|---|---|---|---|---|---|---|---|
R1 | R2 | R1 | R2 | R1 | R2 | R1 | R2 | ||||
2 | 0 | 0 | 0 | 1 | 1 | 1 | 0 | 1 | |||
3 | 1 | 2 | 16 | 15 | 27 | 27 | 24 | 24 | |||
4 | 31 | 28 | 29 | 28 | 22 | 22 | 26 | 25 | |||
5 | 18 | 20 | 5 | 6 | 0 | 0 | 0 | 0 | |||
Kappa score | 0.88 | 0.93 | 1.00 | 0.92 |
A1: triple-low group with DLIR-H; A2: triple-low group with DLIR-L; A3: triple-low group with ASIR-V50%; B: conventional group with ASIR-V50%. SIQS, subjective image quality score; R1, reader 1; R2, reader 2; DLIR, deep learning image reconstruction; DLIR-H, DLIR at high weighting; DLIR-L, DLIR at low weighting; ASIR-V50%, volume-based adaptive statistical iterative reconstruction with 50% weighting.
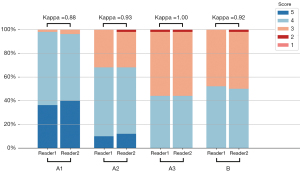
Discussion
In our study, we investigated the value of using DLIR in combination with a 70-kVp dosage to reduce radiation and contrast doses in CTA in the head and neck. Our results demonstrated that compared with a conventional scan protocol based on 100-kVp ASIR-V50%, a 70-kVp DLIR-H protocol reduced the radiation dose, contrast dosage, and contrast injection rate by 47.80%, 36.09%, and 20.88%, respectively.
IR algorithms, such as ASIR-V, have been developed to reduce image noise, especially in low-dose conditions. Typically, IR algorithms run at a moderate intensity to balance noise reduction with image resolution or diagnostic confidence (21,22), which limits its potential for dose reduction. To address this problem, deep learning-based image reconstruction algorithms have been developed, such as DLIR (a commercially available product). DLIR is based on the deep convolutional neural network (DNN), with high-dose filtered back projection (FBP) reconstruction images as the target data and low-dose CT projection as the input data. The ground truth target images used to train the DNN algorithm are obtained using high-dose FBP CT projections under ideal data acquisition conditions, typically from high-dose phantom scan data or clinical data. In this design, the images generated by DLIR can avoid the effects of potential oversmoothing of IR and have similar spatial resolution and noise texture properties to those of FBP images, but with significant reduction in noise. DLIR provides three selectable reconstruction strength levels (low, medium, and high) to control the amount of noise reduction (11). These strength levels have been developed to be better suited for different clinical practice and radiation dose requirements.
Multiple previous works have demonstrated the feasibility and advantages of low-tube voltage scans. Wang et al. (23) reported a radiation dose reduction of 50% when the tube voltage was decreased from 10 to 80 kVp in cerebral CTA. Annoni et al. (24) found the radiation dose reduced by 86% when the tube voltage was decreased from 100 to 80 kVp in carotid CTA. In our study on CTA of the head and neck, the radiation dose reduced by 47.80% when the tube voltage was decreased from 100 to 70 kVp, which is consistent with a previous study by Chen et al. (25). The differences in dose reduction observed between our study and others may be due to differences in multisite scanning or noise indices. Specifically, in this preliminary study, we used a much smaller noise index (noise index =6) than that of the above studies, which might have resulted in a higher radiation dose.
With the use of DLIR-H, the images obtained at the tube voltage of 70 kVp had lower background noise, which was reduced by 28.7% for the head and 21.0% for the neck compared to the images obtained with ASIR-V50% at a tube voltage of 100 kVp. The CNRs and the SNRs of the images in the “triple low” group with DLIR-H were better or equal to the conventional group with ASIR-V50%. The mean ERS was significantly higher on the DLIR images than the ASiR-V50% images both in head and neck, which is line with the findings of Tatsugami et al. (18). Consequently, our study demonstrated that even with a tube voltage reduced to 70 kVp, the reconstructed image could still meet the diagnostic needs after the application of DLIR-H reconstruction. The significantly reduced image noise in the 70-kVp DLIR-H group suggests the potential for further dose reduction. Compared to DLIR-L, DLIR-H is more capable of reducing noise. In this study, the SNR and CNR of the images reconstructed with DLIR-H were higher than those reconstructed with DLIR-L, which is consistent with previous work (13,17,18); moreover, the ERS of DLIR-H was slightly lower than that of DLIR-L, but not significantly so. CNR and SNR are fairly critical to quality CTA, but some loss of spatial resolution is tolerable.
Additionally, unlike other previous work on the head and neck, we set a customized contrast injection protocol based on the patient’s weight. The contrast dosage at 70 kVp was determined according to a phantom experiment. In the phantom experiment, the required contrast concentration for 70 kVp was about 60% of that for 100 kVp. In addition, considering individual patient differences, we set a certain redundancy for the clinical study, so the total amount of contrast at 70 kVp was set to 62.5% of that at 100 kVp, slightly higher than the determined 60%. We found that the contrast dosage in the low-voltage group was 28.76±4.00 mL and that the injection rate was 3.60±0.50 mL/s, which were significantly lower than those of the conventional protocol group. Combined with the use of lower tube voltage, the lower contrast dosage and injection protocol can provide even higher quality images than can conventional scanning, optimize the examination protocol for older adult patients with vasoconstriction or vascular fragility, and reduce the risk of medication in patients, further expanding the applicability of head and neck CTA.
Some limitations to this study should be noted. (I) This study focused on the comparison of image quality, radiation dose, and contrast dosage in head and neck CTA examination without assessing the accuracy of imaging diagnostic efficacy with digital subtraction angiography as the gold standard. (II) Due to ethical consideration, we did not perform a within-subject comparison of different tube voltages of the same individual. (III) Patients with BMI values out of the normal range were excluded from this study. Future studies will be conducted to examine the efficacy and efficiency of this CTA protocol for a wide range of patients.
Conclusions
The use of the 70-kVp tube voltage combined with the DLIR-H reconstruction algorithm the neck and head CTA not only reduced the examination radiation dose, contrast dosage, and contrast injection rate but also improved the image quality as compared to the conventional imaging protocol of 100 kVp and ASIR-V reconstruction.
Acknowledgments
Funding: This study was supported by
Footnote
Reporting Checklist: The authors have completed the STROBE reporting checklist. Available at https://qims.amegroups.com/article/view/10.21037/qims-23-1602/rc
Conflicts of Interest: All authors have completed the ICMJE uniform disclosure form (available at https://qims.amegroups.com/article/view/10.21037/qims-23-1602/coif). S.Z. is an employee of GE HealthCare China. The other authors have no conflicts of interest to declare.
Ethical Statement:
Open Access Statement: This is an Open Access article distributed in accordance with the Creative Commons Attribution-NonCommercial-NoDerivs 4.0 International License (CC BY-NC-ND 4.0), which permits the non-commercial replication and distribution of the article with the strict proviso that no changes or edits are made and the original work is properly cited (including links to both the formal publication through the relevant DOI and the license). See: https://creativecommons.org/licenses/by-nc-nd/4.0/.
References
- Kwee RM. Systematic review on the association between calcification in carotid plaques and clinical ischemic symptoms. J Vasc Surg 2010;51:1015-25. [Crossref] [PubMed]
- Brown DL, Hoffman SN, Jacobs TL, Gruis KL, Johnson SL, Chernew ME. CT angiography is cost-effective for confirmation of internal carotid artery occlusions. J Neuroimaging 2008;18:355-9. [Crossref] [PubMed]
- Davenport MS, Perazella MA, Yee J, Dillman JR, Fine D, McDonald RJ, Rodby RA, Wang CL, Weinreb JC. Use of Intravenous Iodinated Contrast Media in Patients with Kidney Disease: Consensus Statements from the American College of Radiology and the National Kidney Foundation. Radiology 2020;294:660-8. [Crossref] [PubMed]
- Fan J, Yue M, Melnyk R. Benefits of ASiR-V* Reconstruction for Reducing Patient Radiation Dose and Preserving Diagnostic Quality in CT Exams. GE Healthcare; 2020.
- Alkadhi H, Leschka S. Radiation dose of cardiac computed tomography - what has been achieved and what needs to be done. Eur Radiol 2011;21:505-9. [Crossref] [PubMed]
- Greffier J, Frandon J, Larbi A, Beregi JP, Pereira F. CT iterative reconstruction algorithms: a task-based image quality assessment. Eur Radiol 2020;30:487-500. [Crossref] [PubMed]
- Mileto A, Guimaraes LS, McCollough CH, Fletcher JG, Yu L. State of the Art in Abdominal CT: The Limits of Iterative Reconstruction Algorithms. Radiology 2019;293:491-503. [Crossref] [PubMed]
- Park CJ, Kim KW, Lee HJ, Kim MJ, Kim J. Contrast-Enhanced CT with Knowledge-Based Iterative Model Reconstruction for the Evaluation of Parotid Gland Tumors: A Feasibility Study. Korean J Radiol 2018;19:957-64. [Crossref] [PubMed]
- Geyer LL, Schoepf UJ, Meinel FG, Nance JW Jr, Bastarrika G, Leipsic JA, Paul NS, Rengo M, Laghi A, De Cecco CN. State of the Art: Iterative CT Reconstruction Techniques. Radiology 2015;276:339-57. [Crossref] [PubMed]
- Benz DC, Benetos G, Rampidis G, von Felten E, Bakula A, Sustar A, Kudura K, Messerli M, Fuchs TA, Gebhard C, Pazhenkottil AP, Kaufmann PA, Buechel RR. Validation of deep-learning image reconstruction for coronary computed tomography angiography: Impact on noise, image quality and diagnostic accuracy. J Cardiovasc Comput Tomogr 2020;14:444-51. [Crossref] [PubMed]
- Hsieh J, Liu E, Nett B, Tang J, Thibault JB, Sahney S. A new era of imagereconstruction: TrueFidelity™: Technical white paper on deep learning image reconstruction. GE Healthcare; 2019.
- Wang M, Fan J, Shi X, Qin L, Yan F, Yang W. A deep-learning reconstruction algorithm that improves the image quality of low-tube-voltage coronary CT angiography. Eur J Radiol 2022;146:110070. [Crossref] [PubMed]
- Li W, Diao K, Wen Y, Shuai T, You Y, Zhao J, Liao K, Lu C, Yu J, He Y, Li Z. High-strength deep learning image reconstruction in coronary CT angiography at 70-kVp tube voltage significantly improves image quality and reduces both radiation and contrast doses. Eur Radiol 2022;32:2912-20. [Crossref] [PubMed]
- Zhu L, Ha R, Machida H, Shi X, Wang F, Chen K, Chen D, Cao Y, Shen Y, Yang L. Image quality of coronary CT angiography at ultra low tube voltage reconstructed with a deep-learning image reconstruction algorithm in patients of different weight. Quant Imaging Med Surg 2023;13:3891-901. [Crossref] [PubMed]
- Sun J, Li H, Li J, Cao Y, Zhou Z, Li M, Peng Y. Performance evaluation of using shorter contrast injection and 70 kVp with deep learning image reconstruction for reduced contrast medium dose and radiation dose in coronary CT angiography for children: a pilot study. Quant Imaging Med Surg 2021;11:4162-71. [Crossref] [PubMed]
- Suzuki S, Machida H, Tanaka I, Ueno E. Vascular diameter measurement in CT angiography: comparison of model-based iterative reconstruction and standard filtered back projection algorithms in vitro. AJR Am J Roentgenol 2013;200:652-7. [Crossref] [PubMed]
- Cao L, Liu X, Qu T, Cheng Y, Li J, Li Y, Chen L, Niu X, Tian Q, Guo J. Improving spatial resolution and diagnostic confidence with thinner slice and deep learning image reconstruction in contrast-enhanced abdominal CT. Eur Radiol 2023;33:1603-11. [Crossref] [PubMed]
- Tatsugami F, Higaki T, Nakamura Y, Yu Z, Zhou J, Lu Y, Fujioka C, Kitagawa T, Kihara Y, Iida M, Awai K. Deep learning-based image restoration algorithm for coronary CT angiography. Eur Radiol 2019;29:5322-9. [Crossref] [PubMed]
- McCollough C, Cody D, Edyvean S, Geise R, Gould B, Keat N, Huda W, Judy P, Kalender W, McNitt-Gray M, Morin R, Payne T, Stern S, Rothenberg L, Shrimpton P, Timmer J, Wilson C. Report No. 096 - The Measurement, Reporting, and Management of Radiation Dose in CT. American Association of Physicists in Medicine; 2008.
- Faul F, Erdfelder E, Buchner A, Lang AG. Statistical power analyses using G*Power 3.1: tests for correlation and regression analyses. Behav Res Methods 2009;41:1149-60. [Crossref] [PubMed]
- Stocker TJ, Leipsic J, Hadamitzky M, Chen MY, Rubinshtein R, Deseive S, Heckner M, Bax JJ, Kitagawa K, Marques H, Schmermund A, Silva C, Mahmarian J, Kang JW, Grove EL, Lesser J, Massberg S, Hausleiter J. Application of Low Tube Potentials in CCTA: Results From the PROTECTION VI Study. JACC Cardiovasc Imaging 2020;13:425-34. [Crossref] [PubMed]
- Pauchard B, Higashigaito K, Lamri-Senouci A, Knebel JF, Berthold D, Verdun FR, Alkadhi H, Schmidt S. Iterative Reconstructions in Reduced-Dose CT: Which Type Ensures Diagnostic Image Quality in Young Oncology Patients? Acad Radiol 2017;24:1114-24. [Crossref] [PubMed]
- Wang X, Zhu C, Li J, Degnan AJ, Jiang T, Lu J. Knowledge-based iterative model reconstruction: Comparative image quality with low tube voltage cerebral CT angiography. Medicine (Baltimore) 2018;97:e11514. [Crossref] [PubMed]
- Annoni AD, Montorsi P, Andreini D, Pontone G, Mancini ME, Muscogiuri G, Formenti A, Mushtaq S, Trabattoni P, Dainese L, Pepi M. Submillisievert CT angiography for carotid arteries using wide array CT scanner and latest iterative reconstruction algorithm in comparison with previous generations technologies: Feasibility and diagnostic accuracy. J Cardiovasc Comput Tomogr 2019;13:41-7. [Crossref] [PubMed]
- Chen CW, Chen PA, Chou CC, Fu JH, Wang PC, Hsu SH, Lai PH. Combination of Adaptive Statistical Iterative Reconstruction-V and Lower Tube Voltage During Craniocervical Computed Tomographic Angiography Yields Better Image Quality with a Reduced Radiation Dose. Acad Radiol 2019;26:e233-40. [Crossref] [PubMed]