Diagnostic value of dynamic 18F-FDG PET/CT imaging in non-small cell lung cancer and FDG hypermetabolic lymph nodes
Introduction
Lung cancer is one of the leading causes of cancer-related deaths worldwide, accounting for approximately 22% of total annual cancer deaths (1). Adenocarcinoma (ADC) and squamous cell carcinoma (SCC) are the most common subtypes of lung cancer (2), prone to hilar and mediastinal lymph node metastases. Evidently, improved treatment and prognosis (3) have recently become a clinical research hotspot. Considering that ADC and SCC have different treatment options, accurate identification of the pathological types is essential for appropriate treatment and prognosis. Positron emission tomography-computed tomography (PET/CT) combines the anatomical accuracy of CT with details of molecular metabolism from PET. It has become an imaging method of choice for molecular targets in the extensive biochemical process of the quantitative assessment of tumor physiology in vivo (4), thereby providing an important basis for differential diagnosis and efficacy evaluation of tumors (5). Static 2-deoxy-2-[18F]-fluoro-D-glucose (18F-FDG) PET/CT imaging and semi-quantitative evaluation (especially standard uptake values; SUV) are often used in clinical practice, especially for tumor diagnosis and staging. However, the static 18F-FDG PET/CT is only a display of the cumulative results after 60 min of tracer injection, ignoring the intermediate processes, namely delivery of the tracer by blood flow, its exchange between blood vessels and tissues, and its binding and separation from the target (6). SUV, an important diagnostic indicator of PET imaging, is a standard for drug activity and weight given and is a relative measurement value (7,8) that is influenced by many factors related to physical effects, hardware and software system specifications, tracer dynamics, motion, scanning protocol design, and current image-derived PET metrics limitations (4). Concurrently, the SUV average varies according to the voxels included in the average; therefore, it is sensitive to ROI definitions and subject to intra-observer and inter-observer variation (9). Due to the considerable overlap between SUV measurements for malignant and benign lesions, there are limited quantitative measures, such as SUVs, that can be used to interpret PET scans (9). Therefore, early dynamic 18F-FDG PET/CT imaging has increasingly become a novel method for quantitative evaluation in clinical oncology. It describes the spatial and temporal distribution of 18F-FDG from the time of injection, reflects the early blood perfusion and metabolism in real-time, distinguishes free 18F-FDG from bound 18F-FDG-6-PO4, and reveals the pattern of tracer activity over time. Through the application of a dynamic model of dynamic PET imaging in the later stage, functional parameters of tissues and organs, such as blood flow volume and material transport rate can be obtained (10). For dynamic PET imaging, Karakatsanis et al. (2-13) proposed a new four-dimensional (4D) whole-body PET acquisition protocol, promoting the transition from a traditional static SUV to a dynamic SUV for the first time. They demonstrated the clinical feasibility of multi-bed dynamic PET imaging, providing better tumor quantification. Studies have shown a difference in glucose metabolism between ADC and SCC. SCC has a higher metabolic rate than ADC, while vascularization, blood perfusion, and transport rate may be better in ADC than in SCC (14-16). Furthermore, PET/CT texture analysis has shown good diagnostic efficacy in differentiating non-small cell lung cancer (NSCLC) tissue types (17,18). The current study aimed to combine early dynamic 18F-FDG PET/CT imaging and texture analysis to diagnose NSCLC and its FDG hypermetabolic lymph nodes, providing effective information for the differential diagnosis of NSCLC and its metastatic lymph nodes. We present the following article in accordance with the STARD reporting checklist (available at https://qims.amegroups.com/article/view/10.21037/qims-22-725/rc).
Methods
Patients
The study was conducted in accordance with the Declaration of Helsinki (as revised in 2013). The study was approved by the Ethics Committee of Shengjing Hospital, Affiliated with the China Medical University (No. 2020PS683K) and informed consent was taken from all the patients. From October 2020 to November 2021, patients who made an active appointment for PET/CT examination were randomly enrolled by referring to previous imaging data and clinical information. Prospective patients who had applied for clinical applications of whole-body 18F-FDG PET/CT and had agreed to participate in early dynamic 18F-FDG PET/CT scans were included. Inclusion criteria were as follows: (I) first diagnosis of a primary lung tumor; (II) no surgery or any related treatment; (III) possibility of obtaining surgical pathology results and follow-up blood glucose. Exclusion criteria were as follows: (I) inability to complete the 18F-FDG PET/CT scan; (II) fasting blood glucose >8.0 mmol/L. Three patients were excluded, including one with large-cell lung cancer, one with small-cell lung cancer, and one with tuberculosis. Finally, patients with pathologically diagnosed SCC (16 cases) and ADC (18 cases) were included in this study, along with 43 FDG hypermetabolic lymph nodes with confirmed surgical pathology results (19 malignant lymph nodes and 24 benign lymph nodes). Table 1 summarizes the clinical characteristics of the 34 patients under study.
Table 1
Characteristics | SCC | ADC | P value | All lesions |
---|---|---|---|---|
Number of tumors [%] | 16 [47] | 18 [52] | 34 | |
Sex, n [%] | 0.02 | |||
Male | 3 [9] | 11 [32] | 14 | |
Female | 13 [38] | 7 [21] | 20 | |
Age (years), mean ± SD [range] | 61.5±8.3 [49–78] | 61.6±7.3 [51–74] | 0.98 | 61.5±7.7 [49–78] |
Smoking history, n [%] | 0.16 | |||
Yes | 7 [21] | 13 [30] | 20 | |
No | 9 [26] | 5 [15] | 14 | |
Tumor size (mm), mean ± SD [range] | 41.5±21.0 [19–99] | 30.4±10.7 [15–56] | 0.05 | 35.6±16.5 [15–99] |
T stage, n [%] | ||||
T1a | 1 [3] | 4 [12] | 5 | |
T1b | 3 [9] | 5 [15] | 8 | |
T2a | 7 [21] | 5 [15] | 12 | |
T2b | 2 [6] | 2 | ||
T3 | 3 [9] | 2 [6] | 5 | |
T4 | 2 [6] | 2 | ||
N stage, n [%] | ||||
N0 | 7 [21] | 7 [21] | 14 | |
N1 | 2 [6] | 11 [32] | 13 | |
N2 | 4 [13] | 4 | ||
N3 | 3 [9] | 3 | ||
NX | – | |||
TNM stage (7th edition), n [%] | 0.48 | |||
Stage I | 4 [13] | 7 [21] | 11 | |
Stage III | 12 [35] | 11 [32] | 23 | |
FDG hypermetabolic lymph nodes, n [%] | 16 [37] | 27 [63] | 43 | |
Superior mediastinal nodes (2R, 4R, 2L, 4L) | 3 [7] | 5 [12] | 8 | |
Aortic nodes (5, 6) | 4 [9] | 9 [21] | 13 | |
Inferior mediastinal nodes (7, 8R, 9R, 9L) | 7 [16] | 9 [21] | 16 | |
Pulmonary nodes (10R, 11R, 12R, 13R, 10L, 11L, 12L, 13L) | 2 [5] | 4 [9] | 6 | |
Malignant lymph nodes, n [%] | 4 [9] | 15 [35] | 19 | |
Benign lymph nodes, n [%] | 12 [28] | 12 [28] | 24 |
SCC, squamous cell carcinoma; ADC, adenocarcinoma.
Data collection
Each patient, placed in a supine position, underwent a 40-min early dynamic 18F-FDG PET/CT scan of the chest, followed by a whole-body static 18F-FDG PET/CT scan using the Discovery Elite PET/CT (GE Healthcare, Waukesha, United States). Before the examination, all patients fasted for more than 6 h and rested for at least 20 min in a quiet environment. First, a very low-current CT localization scan (120 kV, 80–120 mA, with a tube-rotation time of 1.0 s per rotation) was performed to locate the lung cancer and lymph nodes in the scanner field. Then, 1.0 mL of 4.44 MBq/kg of 18F-FDG, which was obtained using the MINItrace II cyclotron (GE Healthcare, USA) and FX positron drug chemical synthesizer (GE Healthcare), was injected into the median cubital vein of the patient. At the moment of dosing, chest dynamic 18F-FDG PET image acquisition was initiated, and the Ordered Subsets Expectation Maximization (OSEM) was used to reconstruct the acquired list mode (List) data. The frame protocol was 12×5 s, 3×360 s, and 3×420 s. After performing dynamic imaging, patients were advised to rest comfortably in the waiting room. Sixty minutes after injection, standard whole-body 18F-FDG PET/CT imaging was performed from the apex of the skull to the proximal thigh. Before the whole-body 18F-FDG PET/CT scan, a second low-dose CT scan (120 kV, 80–120 mA, with a tube-rotation time of 1.0 s per rotation) was performed to correct for the attenuation from the apex of the skull to the proximal thigh. Thereafter, 18F-FDG PET images of the whole body were collected in six to seven beds, in approximately 90 s per bed. The data acquisition process is shown in Figure 1. Early chest dynamics and standard whole-body images were acquired in three-dimensional (3D) mode, attenuation correction was performed using the attenuation map derived from CT, and a 3D iterative algorithm (OSEM) was used for reconstruction.
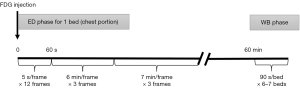
Data analysis
Image analysis and modeling of 18F-FDG PET/CT were performed under the supervision of three experienced nuclear medicine physicians (Dr. Li., a physician with 3 years of experience in nuclear medicine diagnosis; Dr. Shu., a physician with 20 years of experience in nuclear medicine diagnosis; Dr. Jun., a physician with 25 years of experience in nuclear medicine diagnosis), who were unaware of the clinical, pathological, and other imaging manifestations associated with uptake by the primary tumor and lymph node for FDG assessment. If the results of the three doctors differed, they discussed the results until a consensus was reached. The GE Advantage Workstation01 workstation was used to semi-automatically place the volume of interest (VOI) on the primary tumor and lymph nodes using a threshold of 40% of the maximum pixel value on static PET/CT images for SUV measurement and analysis. Lymph nodes with SUVmax >2.5 were considered FDG-high uptake lymph nodes. Dynamic PET/CT images were processed by PET Dynamic software (GE Healthcare, Beijing, China). First, the PET Dynamic software automatically searched for the thoracic aorta to obtain the arterial input function (AIF) and time activity curve (TAC). ITK-SNAP software was used to delineate lesions and lymph nodes in CT images of dynamic PET/CT imaging by two nuclear medicine physicians (Dr. Li. and Dr. Shu., separately). When encountering lesions or lymph nodes that were complicated in shape, had calcified necrosis, or were indistinguishable from blood vessels and esophagus, three nuclear medicine physicians (Dr. Li., Dr. Shu., and Dr. Jun.) discussed and delineated the VOI together. Finally, the two groups of VOI were compared for similarity, yielding a Dice coefficient between 0.83 and 0.91. The final dynamic PET image was fused and calibrated with CT to obtain the VOI of the dynamic PET image. The fusion and registration were rigid registration, which can automatically register the PET image to the CT image through software. By determining the area-weighted average of the mean metabolic activity in each primary lesion and lymph node, the ROI of all sections of the dynamic PET image and TAC of the primary lesion and lymph node could be obtained. Kinetic modeling used PET Dynamic software (GE Healthcare) for voxel-based analysis based on the VOI. Patlak graphical analysis and irreversible two-tissue compartment model (2TC-3k) were used to analyze the data. Patlak graphical analysis produced the relevant first-order characteristic parameters of net phosphorylation rate Ki and blood volume V (19-22). 2TC-3k, as shown in Figure 2, produced the related first-order characteristic parameters of transport rates (K1, k2, and k3) and blood volume fraction (VB).

Statistical analysis
MedCalc v20.0.3 and SPSS 26.0 were used for statistical analysis. The description of continuous variables in the data was expressed as mean ± standard deviation. Comparison between groups of parameters was analyzed by an independent sample t-test for normally distributed data or the Mann-Whitney U-test for skewed distribution data. The categorical variables were tested by the chi-square test. Binary logistic regression analysis was used to obtain the best-predicted probability of each kinetic parameter. The fit of the model was assessed by the Hosmer-Lemeshow test (a test used to judge the goodness of fit of a model, whose function is to show the degree of agreement between the fitted and the observed values). Receiver operating characteristic (ROC) curve analysis and the DeLong test were used to obtain the test efficacy of each kinetic parameter for comparative analysis. P<0.05 was considered a statistically significant difference.
Results
Patient and clinicopathological characteristics
Pathological diagnosis of patients was based on surgery (n=18), bronchofibroscopy (n=8), and CT-guided biopsy (n=8), which were completed 1–5 days after PET/CT imaging; post-operative pathology confirmed 27 FDG hypermetabolic lymph nodes derived from adenocarcinoma and 16 SCC-derive FDG hypermetabolic lymph nodes. The specific selection process is shown in Figure 3. None of the participants experienced any adverse reactions due to the increased scanning time.
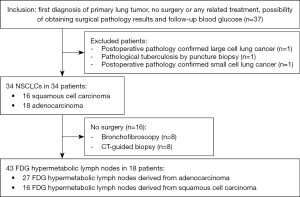
Diagnostic efficacy of each parameter and kinetic model for NSCLC
The lesions were pathologically confirmed in 18 cases of ADC and 16 cases of SCC. Figure 4 shows the difference between the static PET parameter SUV and the statistically significant dynamic PET parameter of ADC and SCC. The static PET parameters in SCC, SUVmax (14.73±6.56 vs. 11.03±6.85, P=0.12), and SUVmean (8.96±4.04 vs. 6.70±4.33, P=0.13), were higher than those in ADC, although the difference was not statistically significant.
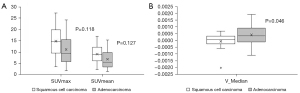
In contrast, the dynamic PET parameter, V_Median (−0.0001±0.00063 vs. 0.0004±0.0007, P=0.04), was significantly lower in SCC than in ADC. With the help of binary logistic regression analysis, the first-order characteristic parameters, Ki, V, K1, k2, k3, and VB, of SCC and ADC were selected to generate the prediction probability of lesions. The Hosmer-Lemeshow test showed the predictive probability models of K1, k3, and V to have good predictive performance in the differential diagnosis of squamous adenocarcinoma (Table 2). ROC curve analysis showed K1 to have the best diagnostic power, with an area under the curve (AUC) of 0.91, sensitivity of 0.83, and specificity of 0.94 (Figure 5); the DeLong test showed that the differences in AUC among the three models were not statistically significant (Table 2).
Table 2
Model | Chi-square value | Hosmer-Lemeshow’s P | AUC (95% confidence interval) | Sensitivity | Specificity | Accuracy | DeLong’s P |
---|---|---|---|---|---|---|---|
K1 | 7.11 | 0.53 | 0.91 (0.82–1) | 0.83 | 0.94 | 0.88 | 0.49 |
k3 | 5.84 | 0.67 | 0.85 (0.73–0.98) | 0.72 | 0.88 | 0.79 | 0.73 |
V | 8.13 | 0.42 | 0.89 (0.78–1) | 0.78 | 0.88 | 0.82 | 0.64 |
AUC, area under the curve.
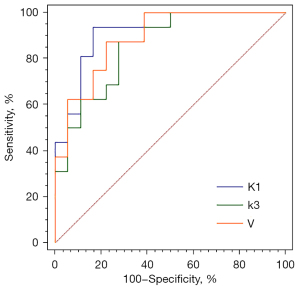
Diagnostic efficacy of each parameter and kinetic model for hypermetabolic lymph nodes
FDG hypermetabolic lymph nodes, with SUVmax >2.5 in static PET and confirmed by surgical pathology, including 19 malignant lymph nodes and 24 benign lymph nodes, were selected. The size (0.85±0.31 vs. 0.70±0.24, P=0.07) of malignant lymph nodes was slightly larger than that of benign lymph nodes; however, the difference between the two was not statistically significant. Figure 6 shows the differences in static PET parameters, SUV, and statistically significant dynamic PET parameters between benign and malignant lymph nodes. The static PET parameters, SUVmax (7.32±2.61 vs. 5.97±2.10, P=0.07) and SUVmean (4.37±1.65 vs. 3.49±1.21, P=0.06) of malignant lymph nodes were higher than those of benign lymph nodes, although the difference was not statistically significant; however, the dynamic PET parameters of malignant lymph nodes, k3_Entropy (6.4×10−4±5.5×10−4vs. 3.5×10−4±2.9×10−4, P=0.03), and VB_Entropy (8.0×10−4±6.0×10−4vs. 4.1×10−4±3.0×10−4, P=0.003) were significantly higher than those of benign lymph nodes. Ki_Uniformity (0.999918±6.4×10−5vs. 0.999960±2.5×10−5, P=0.005) and K1_Uniformity (0.999938±5.8×10−5vs. 0.999965±3.0×10−5, P=0.03) in malignant lymph nodes were significantly lower than in benign lymph nodes, and the difference was statistically significant. With the aid of binary logistic regression analysis, the first-order feature parameters, Ki, V, K1, k2, k3, and VB, of benign and malignant lymph nodes were selected to generate the predicted probability of lymph nodes. The Hosmer-Lemeshow test showed the predictive probability models of Ki, VB, and k3 to have good predictive performance for the differential diagnosis of benign and malignant lymph nodes (Table 3). ROC curve analysis showed Ki to have the best diagnostic power, with an AUC of 0.87, sensitivity of 0.79, and specificity of 0.84 (Figure 7). The DeLong test showed that differences in the AUC of the three models were not statistically significant (Table 3).
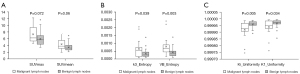
Table 3
Model | Chi-square value | Hosmer-Lemeshow’s P | AUC (95% confidence interval) | Sensitivity | Specificity | Accuracy | DeLong’s P |
---|---|---|---|---|---|---|---|
Ki | 3.72 | 0.88 | 0.87 (0.76–0.97) | 0.79 | 0.84 | 0.81 | 0.49 |
VB | 5.59 | 0.69 | 0.82 (0.69–0.95) | 0.74 | 0.83 | 0.79 | 0.57 |
k3 | 9.13 | 0.34 | 0.85 (0.73–0.98) | 0.83 | 0.90 | 0.86 | 0.87 |
AUC, area under the curve; VB, blood volume fraction.
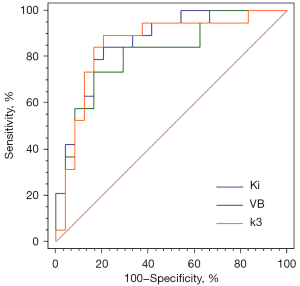
Discussion
Cancer management has entered the era of precision medicine. Precision medicine relies on validated biomarkers and a series of related technologies to classify patients according to their possible disease risk, prognosis, and/or treatment response. Early and accurate diagnosis of lung cancer subtypes is particularly important. PET/CT is a mature fusion-based functional imaging technology that can perform non-invasive tumor assessment for classification, staging, efficacy, and prognostic evaluation. However, its utility in the differential diagnosis of ADC and SCC is limited to interpreting images in a conventional way.
In this study, we used dynamic 18F-FDG PET/CT imaging and modeling to obtain transport rates (Ki, K1, k2, k3) and blood volume (V, VB) related to target-specific molecules/metabolic processes, further verifying the diagnostic utility of dynamic PET/CT in NSCLC. Unlike the semi-quantitative evaluation of single time-point measurement of conventional clinical 18F-FDG PET/CT imaging (6), dynamic 18F-FDG PET/CT is a multi-time-point measurement after injection, which can capture the whole process of 18F-FDG uptake and distribution. It retrieves quantitative evaluation indicators, such as transport rate and blood volume, through kinetic modeling to distinguish between ADC, SCC, and benign or malignant FDG hypermetabolic lymph nodes, providing objective imaging evidence for clinical treatment plans and prognostic evaluation. In addition, we included the texture parameter (23,24) as an indicator based on the quantitative evaluation of dynamic PET/CT. Combining the two, 18F-FDG PET/CT kinetic modeling yielded the first-order texture parameters based on transport rate and blood volume, thereby suggesting a theoretical basis and technical support for early, accurate, and individualized treatment.
Although there was no statistically significant difference in the SUV value of static PET between ADC and SCC, the overall trend showed that, compared to ADC, SCC had a higher SUV, similar to the results of previous studies (2,15). Schurbiers et al. (15) suggested that this could be because ADC is involved in glycolysis under normoxic conditions, whereas SCC is in a diffusion-restricted hypoxia state, resulting in a very high anaerobic glycolysis rate. In addition, our dynamic PET parameter, V_Median, and the logistic model, constructed by the first-order texture parameters of K1, k3, and V, could distinguish between ADC and SCC well, similar to the results of Meijer et al. (25). The transport rate and blood volume in ADC and SCC significantly differed. Compared to that in ADC, the k3 and VB in SCC had greater heterogeneity. Wang et al. (26) also demonstrated the advantages of dynamic PET imaging in assessing the metabolic heterogeneity of primary tumors and lymph nodes in patients with NSCLC. This could be because, compared to the high perfusion and low glucose phosphorylation rate in ADC, SCC had a higher glycolysis rate and less vascularization (27). In addition, due to the histopathological differences between ADC and SCC in metabolic transporters and enzymes (15), liquefactive necrosis can often be observed in SCC (28), thereby rendering the whole tumor of SCC to have higher metabolic heterogeneity, with increased glucose transport and phosphorylation in the area of reduced blood volume. Moreover, the study showed that compared to the high degree of confusion across malignant lymph nodes, benign lymph nodes demonstrated better uniformity. The logistic model constructed by the first-order texture parameters Ki, k3, and VB, showed appreciable efficiency in the differential diagnosis of benign and malignant lymph nodes. Previous studies had initially confirmed the importance of dynamic 18F-FDG PET/CT imaging for diagnosing chest lymph nodes (29,30). This may be due to the rapid growth of malignant lesions, increased demand for glucose, presence of a large number of new capillary networks, immature vascular wall, increased predisposition to necrosis, cyst degeneration, bleeding, other complex conditions, and poor uniformity (31), which resulted in differences in the kinetic parameters.
Our study has several limitations. First, the manual AIF VOI definition of the thoracic aorta may introduce inter- and intra-observer variability. However, Kramer et al. (32) conducted a study on the reproducibility of 18F-FDG indicators in NSCLC, and the results showed that test and retest values of all uptake measurements in whole-body static 18F-FDG PET/CT dual scan, performed within 3 days, were highly correlated. In addition, de Geus-Oei et al. (33) found the glucose metabolism rate based on AIFs and that based on continuous arterial sampling (reference standard) to be highly correlated [intra-group correlation coefficient (ICC); ascending aorta 0.98, left ventricle 0.94, and abdominal aorta 0.96]; therefore, the use of AIF was accurate and could be considered as a clinical alternative to arterial blood sampling. We used the thoracic aorta as the AIF instead of the heart, as the heart is not fully imaged in all dynamic scans. Second, since dynamic PET is an unconventional clinical examination item, the data acquisition method is cumbersome, and the patient’s cooperation degree was higher, the sample size included in the study was small. However, it should be noted that existing reports studied the simpler version of dynamic PET scan mode, showing good feasibility and accuracy, which makes dynamic PET imaging suitable for clinical application (34,35). Samimi et al. (35) confirmed that 5 minutes of dynamic PET imaging combined with 3 minutes of static PET imaging could accurately and robustly estimate the parameters of the two-tissue compartment model. In future studies, the sample size should be increased by recruiting more patients to verify the currently obtained conclusions. Third, since this is a prospective study, there is no relevant experience reference before this, and the data collection in the experiment is as close to the actual clinical collection scheme as possible. In addition, the specific collection conditions of the patients participating in the data collection were considered in the design; hence, the collection time was relatively flexible in the design of the experiment, especially in the early stage. Nonetheless, the instability of the Patlak and 2TC-3K models reduces the accuracy and reliability of the parameters. In future studies, we aim to refer to the data segmentation protocol of Häggström et al. (36) and adopt a robust direct 4D parametric image reconstruction algorithm to reduce unnecessary bias (37-39), in order to explore the aspects that the current research could not fully elucidate. In addition, although we assumed that the kinetics of the FDG study are irreversible, the possibility of non-negligible FDG uptake reversibility to some extent cannot be ruled out. If the model ignores their effects, the Ki and k3 parameters in these regions will be underestimated (40,41). Therefore, we plan to collect more samples in future and apply more models, including the reversible atrioventricular model and other parameters of the unorganized atrioventricular model, such as the fractal dimension (42,43). Based on previous studies, the estimation error of model 2TC-3K is usually larger than that of Patlak (19,20,44). However, the positive results we have obtained so far are mainly 2TC-3K. We have combined first-order texture parameters, which will need to be verified by enrolling more patients. Moreover, FDG low-metabolic lymph nodes may also be included in the study to discuss the diagnostic sensitivity of dynamic 18F-FDG PET/CT. In addition, the lack of external verification in this study limited the universality of the results to a certain extent. Finally, although the primary lesions and lymph nodes of the study were calibrated by three nuclear medicine physicians, distinguishing across some lesions, lymph nodes, and blood vessels was difficult and could introduce errors in the measurement results. Furthermore, due to the relatively small lymph nodes and a large number of interference items in the location, the artifact issue was not paid attention to; consequently, registration correction was performed, following which corresponding research was conducted to focus on this point and improve it. In addition, there were several cases of patients with ADC who exhibited weak FDG uptake, which could not be delineated and modeled. Tumor lesions or lymph nodes that were too small for kinetic modeling were also excluded.
With the increase in lung cancer screening, many small lesions have become easier to detect at an early stage. Therefore, a smaller size and smarter delineation-modeling tool would provide an important direction for future research.
Conclusions
In conclusion, dynamic 18F-FDG PET/CT imaging has certain clinical significance for the classification of NSCLC and the differential diagnosis of lymph nodes, thereby helping clinicians improve the histopathological diagnosis of lung cancer in a non-invasive manner.
Acknowledgments
Funding: None.
Footnote
Reporting Checklist: The authors have completed the STARD reporting checklist. Available at https://qims.amegroups.com/article/view/10.21037/qims-22-725/rc
Conflicts of Interest: All authors have completed the ICMJE uniform disclosure form (available at https://qims.amegroups.com/article/view/10.21037/qims-22-725/coif). YW is an employee of Beijing General Electric Company. The other authors have no conflicts of interest to declare.
Ethical Statement: The authors are accountable for all aspects of the work in ensuring that questions related to the accuracy or integrity of any part of the work are appropriately investigated and resolved. The study was conducted in accordance with the Declaration of Helsinki (as revised in 2013). The study was approved by the Ethics Committee of Shengjing Hospital, Affiliated with the China Medical University (No. 2020PS683K) and informed consent was taken from all the patients.
Open Access Statement: This is an Open Access article distributed in accordance with the Creative Commons Attribution-NonCommercial-NoDerivs 4.0 International License (CC BY-NC-ND 4.0), which permits the non-commercial replication and distribution of the article with the strict proviso that no changes or edits are made and the original work is properly cited (including links to both the formal publication through the relevant DOI and the license). See: https://creativecommons.org/licenses/by-nc-nd/4.0/.
References
- Siegel RL, Miller KD, Fuchs HE, Jemal A. Cancer Statistics, 2021. CA Cancer J Clin 2021;71:7-33. [Crossref] [PubMed]
- Hyun SH, Ahn MS, Koh YW, Lee SJ. A Machine-Learning Approach Using PET-Based Radiomics to Predict the Histological Subtypes of Lung Cancer. Clin Nucl Med 2019;44:956-60. [Crossref] [PubMed]
- Wang BY, Huang JY, Chen HC, Lin CH, Lin SH, Hung WH, Cheng YF. The comparison between adenocarcinoma and squamous cell carcinoma in lung cancer patients. J Cancer Res Clin Oncol 2020;146:43-52. [Crossref] [PubMed]
- Zaidi H, Karakatsanis N. Towards enhanced PET quantification in clinical oncology. Br J Radiol 2018;91:20170508. [Crossref] [PubMed]
- Furlow B. PET-CT Cancer Imaging. Radiol Technol 2018;90:149CT-70CT.
- Yang DM, Palma D, Louie A, Malthaner R, Fortin D, Rodrigues G, Yaremko B, Laba J, Gaede S, Warner A, Inculet R, Lee TY. Assessment of tumour response after stereotactic ablative radiation therapy for lung cancer: A prospective quantitative hybrid (18) F-fluorodeoxyglucose-positron emission tomography and CT perfusion study. J Med Imaging Radiat Oncol 2019;63:94-101. [Crossref] [PubMed]
- Devriese J, Beels L, Maes A, Van De Wiele C, Gheysens O, Pottel H. Review of clinically accessible methods to determine lean body mass for normalization of standardized uptake values. Q J Nucl Med Mol Imaging 2016;60:1-11. [PubMed]
- Keyes JW Jr. SUV: standard uptake or silly useless value? J Nucl Med 1995;36:1836-9. [PubMed]
- Adams MC, Turkington TG, Wilson JM, Wong TZ. A systematic review of the factors affecting accuracy of SUV measurements. AJR Am J Roentgenol 2010;195:310-20. [Crossref] [PubMed]
- Zaker N, Kotasidis F, Garibotto V, Zaidi H. Assessment of Lesion Detectability in Dynamic Whole-Body PET Imaging Using Compartmental and Patlak Parametric Mapping. Clin Nucl Med 2020;45:e221-31. [Crossref] [PubMed]
- Karakatsanis NA, Lodge MA, Zhou Y, Mhlanga J, Chaudhry MA, Tahari AK. Dynamic multi-bed FDG PET imaging: feasibility and optimization. 2011 IEEE Nuclear Sciences Symposium Conference Record, p. 3863.
- Karakatsanis NA, Lodge MA, Tahari AK, Zhou Y, Wahl RL, Rahmim A. Dynamic whole-body PET parametric imaging: I. Concept, acquisition protocol optimization and clinical application. Phys Med Biol 2013;58:7391-418. [Crossref] [PubMed]
- Karakatsanis NA, Lodge MA, Zhou Y, Wahl RL, Rahmim A. Dynamic whole-body PET parametric imaging: II. Task-oriented statistical estimation. Phys Med Biol 2013;58:7419-45. [Crossref] [PubMed]
- Koh YW, Lee SJ, Park SY. Differential expression and prognostic significance of GLUT1 according to histologic type of non-small-cell lung cancer and its association with volume-dependent parameters. Lung Cancer 2017;104:31-7. [Crossref] [PubMed]
- Schuurbiers OC, Meijer TW, Kaanders JH, Looijen-Salamon MG, de Geus-Oei LF, van der Drift MA, van der Heijden EH, Oyen WJ, Visser EP, Span PN, Bussink J. Glucose metabolism in NSCLC is histology-specific and diverges the prognostic potential of 18FDG-PET for adenocarcinoma and squamous cell carcinoma. J Thorac Oncol 2014;9:1485-93. [Crossref] [PubMed]
- Zhang J, Chen L, Chen Y, Wang W, Cheng L, Zhou X, Wang J. Tumor vascularity and glucose metabolism correlated in adenocarcinoma, but not in squamous cell carcinoma of the lung. PLoS One 2014;9:e91649. [Crossref] [PubMed]
- Bianconi F, Palumbo I, Fravolini ML, Chiari R, Minestrini M, Brunese L, Palumbo B. Texture Analysis on [18F]FDG PET/CT in Non-Small-Cell Lung Cancer: Correlations Between PET Features, CT Features, and Histological Types. Mol Imaging Biol 2019;21:1200-9. [Crossref] [PubMed]
- Lee H, Kim H, Choi YS, Pyo HR, Ahn MJ, Choi JY. Prognostic Significance of Pseudotime from Texture Parameters of FDG PET/CT in Locally Advanced Non-Small-Cell Lung Cancer with Tri-Modality Therapy. Cancers (Basel) 2022.
- Fahrni G, Karakatsanis NA, Di Domenicantonio G, Garibotto V, Zaidi H. Does whole-body Patlak (18)F-FDG PET imaging improve lesion detectability in clinical oncology? Eur Radiol 2019;29:4812-21. [Crossref] [PubMed]
- Zhuang M, Karakatsanis NA, Dierckx RAJO, Zaidi H. Quantitative Analysis of Heterogeneous [18F]FDG Static (SUV) vs. Patlak (Ki) Whole-body PET Imaging Using Different Segmentation Methods: a Simulation Study. Mol Imaging Biol 2019;21:317-27. [Crossref] [PubMed]
- Patlak CS, Blasberg RG, Fenstermacher JD. Graphical evaluation of blood-to-brain transfer constants from multiple-time uptake data. J Cereb Blood Flow Metab 1983;3:1-7. [Crossref] [PubMed]
- Patlak CS, Blasberg RG. Graphical evaluation of blood-to-brain transfer constants from multiple-time uptake data. Generalizations. J Cereb Blood Flow Metab 1985;5:584-90. [Crossref] [PubMed]
- Wang L, Li T, Hong J, Zhang M, Ouyang M, Zheng X, Tang K. (18)F-FDG PET-based radiomics model for predicting occult lymph node metastasis in clinical N0 solid lung adenocarcinoma. Quant Imaging Med Surg 2021;11:215-25. [Crossref] [PubMed]
- Shen H, Chen L, Liu K, Zhao K, Li J, Yu L, Ye H, Zhu W. A subregion-based positron emission tomography/computed tomography (PET/CT) radiomics model for the classification of non-small cell lung cancer histopathological subtypes. Quant Imaging Med Surg 2021;11:2918-32. [Crossref] [PubMed]
- Meijer TWH, de Geus-Oei LF, Visser EP, Oyen WJG, Looijen-Salamon MG, Visvikis D, Verhagen AFTM, Bussink J, Vriens D. Tumor Delineation and Quantitative Assessment of Glucose Metabolic Rate within Histologic Subtypes of Non-Small Cell Lung Cancer by Using Dynamic (18)F Fluorodeoxyglucose PET. Radiology 2017;283:547-59. [Crossref] [PubMed]
- Wang D, Zhang X, Liu H, Qiu B, Liu S, Zheng C, Fu J, Mo Y, Chen N, Zhou R, Chu C, Liu F, Guo J, Zhou Y, Zhou Y, Fan W, Liu H. Assessing dynamic metabolic heterogeneity in non-small cell lung cancer patients via ultra-high sensitivity total-body [18F]FDG PET/CT imaging: quantitative analysis of [18F]FDG uptake in primary tumors and metastatic lymph nodes. Eur J Nucl Med Mol Imaging 2022;49:4692-4704. [Crossref] [PubMed]
- Vriens D, Disselhorst JA, Oyen WJ, de Geus-Oei LF, Visser EP. Quantitative assessment of heterogeneity in tumor metabolism using FDG-PET. Int J Radiat Oncol Biol Phys 2012;82:e725-31. [Crossref] [PubMed]
- Tomori Y, Yamashiro T, Tomita H, Tsubakimoto M, Ishigami K, Atsumi E, Murayama S. CT radiomics analysis of lung cancers: Differentiation of squamous cell carcinoma from adenocarcinoma, a correlative study with FDG uptake. Eur J Radiol 2020;128:109032. [Crossref] [PubMed]
- Shinya T, Otomi Y, Kubo M, Kinoshita M, Takechi K, Uyama N, Yamanaka M, Terazawa K, Toba H, Bando Y, Otsuka H, Harada M. Preliminary clinical assessment of dynamic (18)F-fluorodeoxyglucose positron emission tomography/computed tomography for evaluating lymph node metastasis in patients with lung cancer: a prospective study. Ann Nucl Med 2019;33:414-23. [Crossref] [PubMed]
- Yamanaka M, Shinya T, Otomi Y, Kubo M, Arai Y, Toba H, Bando Y, Otsuka H, Harada M. Semiquantitative assessment of fluorodeoxyglucose uptake in primary tumours on dynamic PET/computed tomography for lymph node metastasis evaluation in patients with lung cancer: a prospective study. Nucl Med Commun 2020;41:1189-98. [Crossref] [PubMed]
- Digumarthy SR, Padole AM, Lo Gullo R, Singh R, Shepard JO, Kalra MK. CT texture analysis of histologically proven benign and malignant lung lesions. Medicine (Baltimore) 2018;97:e11172. [Crossref] [PubMed]
- Kramer GM, Frings V, Hoetjes N, Hoekstra OS, Smit EF, de Langen AJ, Boellaard R. Repeatability of Quantitative Whole-Body 18F-FDG PET/CT Uptake Measures as Function of Uptake Interval and Lesion Selection in Non-Small Cell Lung Cancer Patients. J Nucl Med 2016;57:1343-9. [Crossref] [PubMed]
- de Geus-Oei LF, Visser EP, Krabbe PF, van Hoorn BA, Koenders EB, Willemsen AT, Pruim J, Corstens FH, Oyen WJ. Comparison of image-derived and arterial input functions for estimating the rate of glucose metabolism in therapy-monitoring 18F-FDG PET studies. J Nucl Med 2006;47:945-9. [PubMed]
- Strauss LG, Pan L, Cheng C, Haberkorn U, Dimitrakopoulou-Strauss A. Shortened acquisition protocols for the quantitative assessment of the 2-tissue-compartment model using dynamic PET/CT 18F-FDG studies. J Nucl Med 2011;52:379-85. [Crossref] [PubMed]
- Samimi R, Kamali-Asl A, Geramifar P, van den Hoff J, Rahmim A. Short-duration dynamic FDG PET imaging: Optimization and clinical application. Phys Med 2020;80:193-200. [Crossref] [PubMed]
- Häggström I, Axelsson J, Schmidtlein CR, Karlsson M, Garpebring A, Johansson L, Sörensen J, Larsson A. A Monte Carlo study of the dependence of early frame sampling on uncertainty and bias in pharmacokinetic parameters from dynamic PET. J Nucl Med Technol 2015;43:53-60. [Crossref] [PubMed]
- Karakatsanis NA, Casey ME, Lodge MA, Rahmim A, Zaidi H. Whole-body direct 4D parametric PET imaging employing nested generalized Patlak expectation-maximization reconstruction. Phys Med Biol 2016;61:5456-85. [Crossref] [PubMed]
- Karakatsanis NA, Casey ME, Knesaurek K, Fayad ZA, Kostakoglu L. SUV/Patlak-4D whole-body PET/CT dynamic and parametric imaging: clinical demonstration and validation of SUV synthesis from dynamic passes. In 2017 IEEE Nuclear Science Symposium and Medical Imaging Conference (NSS/MIC) p. 1.
- Hu J, Panin V, Smith AM, Spottiswoode B, Shah V, von Gall CC. Design and implementation of automated clinical whole body parametric PET with continuous bed motion. IEEE Transactions on Radiation and Plasma Medical Sciences 2020;4:696-707. [Crossref]
- Messa C, Choi Y, Hoh CK, Jacobs EL, Glaspy JA, Rege S, Nitzsche E, Huang SC, Phelps ME, Hawkins RA. Quantification of glucose utilization in liver metastases: parametric imaging of FDG uptake with PET. J Comput Assist Tomogr 1992;16:684-9. [Crossref] [PubMed]
- Torizuka T, Tamaki N, Inokuma T, Magata Y, Sasayama S, Yonekura Y, Tanaka A, Yamaoka Y, Yamamoto K, Konishi J. In vivo assessment of glucose metabolism in hepatocellular carcinoma with FDG-PET. J Nucl Med 1995;36:1811-7. [PubMed]
- Karakatsanis NA, Zhou Y, Lodge MA, Casey ME, Wahl RL, Zaidi H, Rahmim A. Quantitative whole-body parametric PET imaging incorporating a generalized Patlak model. 2013 IEEE Nuclear Science Symposium and Medical Imaging Conference (2013 NSS/MIC) p. 1.
- Karakatsanis NA, Zhou Y, Lodge MA, Casey ME, Wahl RL, Zaidi H, Rahmim A. Generalized whole-body Patlak parametric imaging for enhanced quantification in clinical PET. Phys Med Biol 2015;60:8643-73. [Crossref] [PubMed]
- Feng T, Zhao Y, Shi H, Li H, Zhang X, Wang G, Price PM, Badawi RD, Cherry SR, Jones T. Total-Body Quantitative Parametric Imaging of Early Kinetics of (18)F-FDG. J Nucl Med 2021;62:738-44. [Crossref] [PubMed]