Ultrasound-based prediction of preoperative core biopsy categories in solid breast tumor using machine learning
Introduction
Breast tumors are common across the globe and lower the quality of life of adult women. Histologically, breast tumors are classified as benign, malignant, or borderline. Each breast lesion can be treated using different methods, including such as biopsy, operation, and imaging follow-up (1,2).
Imaging follow-ups for breast tumors are the most common method and include magnetic resonance imaging (MRI), ultrasound (US), and mammography. These are considered important techniques for assessing breast lesions and preliminarily evaluating their pathological properties. However, these methods are not without disadvantages; mammography is not good at discovering a nodule in dense breast tissue (3), and MRI often has a high false-positive rate for malignant tumors and a high cost of operation (4). In contrast, US does not use radiation, costs less, and is widely available. Therefore, US is the preferred imaging method for evaluating breast nodules in Asian countries, where women tend to have denser breast tissue (5).
The American College of Radiology Breast Imaging Reporting and Data System (ACR BI-RADS) is the most commonly use classification tool in US. This system can predict the probability of malignancy and is suitable only for screening, nut it is not suitable for precise individual management for radiologists for two reasons. First, radiologists currently pay too much attention to probability in providing the BI-RADS category and often ignore the specific pathological type (e.g., mucinous cancer, sclerosing adenosis, or fibroadenoma). To some extent, the radiologists’ diagnostic accuracy and understanding of the specific pathological type will inevitably decrease in the future. Second, lesions treated with neoadjuvant therapy are classified into BI-RADS category 6 for the entire treatment period. At the moment, BI-RADS category is not capable of reflecting dynamic changes. Therefore, radiologists must master the diagnosis of specific pathological types, which can sometimes provide the necessary guidance for individualized clinical management.
There are many types of solid tumors of the breast, and it is very difficult for radiologists to be proficient in the detailed characteristics of each type of tumor. Core biopsy categories (CBCs) help to alleviate this difficulty. According to core biopsy reporting guidelines, all breast histological types can be classified into 5 categories (B1–B5) (6). CBCs can become the basis for building a new radiological reporting system to help radiologists master pathological types. Thus, machine learning should be applied to conduct CBC multiclassification.
Machine learning with many algorithms can automatically analyze and obtain rules from existing data and then use the rules to predict unknown data. Machine learning can also complete classification tasks, including in the areas of radiology, critical care medicine, and cardiology (7-9). Recent studies have shown that machine learning can be beneficial to the diagnosis and treatment of cancer, including imaging examinations for evaluating breast cancer (9-12). Sutton et al. indicated that a machine learning classifier combining MRI radiomics with molecular subtypes could accurately assess pathologic complete response after post-neoadjuvant chemotherapy for breast cancer (13). Wu et al. reported that machine learning achieved a high level of differentiation between triple-negative and non-triple-negative subtypes of breast cancer (14). We aimed to use machine learning to analyze the clinical and ultrasonic features for predicting CBCs and to aid in the development of a new US imaging reporting system for solid tumors of the breast. We present the following article in accordance with the TRIPOD reporting checklist (available at https://qims.amegroups.com/article/view/10.21037/qims-22-877/rc).
Methods
Participants
The study was conducted in accordance with the Declaration of Helsinki (as revised in 2013). This study was approved by the institutional review board of Guangdong Provincial People’s Hospital, Guangdong Academy of Medical Sciences. The informed consent requirement was waived due to the retrospective nature of the study. We acquired details of the pathological characteristics of breast nodules from histological reports. From March 1, 2019, to December 31, 2019, 1,082 female patients were included in the study (age range, 12–96 years; mean age ± standard deviation, 42.22±13.37 years). A total of 1,185 nodules (815 benign nodules and 370 malignant nodules) met the inclusion and exclusion criteria. All patients underwent US-guided core needle biopsies. Nodules were marked on the skin, and ultrasonic images were obtained in the electronic system.
The inclusion criteria were as follows: (I) nodules were clinically suspicious for breast cancer and underwent biopsy; (II) nodules were B1, B2, B3, or B5; (III) patients expressed a strong desire to be biopsied; and (IV) solid breast nodules were defined as having little or no anechoic components based on US images. The exclusion criteria were as follows: (I) lesions were metastatic tumors; (II) patients had undergone systemic hormone therapy or adjuvant chemotherapy; and (III) nodules were B4. (The B4 category was excluded, as it cannot provide a definite diagnosis or valuable information for clinical practice, and further processing is required to determine its pathological type.)
The workflow is shown in Figure 1.
Clinical characteristic acquisition, ultrasonic image acquisition, and interpretation
Clinical characteristics included age, height, weight, and body mass index (BMI). The images were obtained using a 14-MHz linear transducer (Toshiba Aplio 500, Toshiba, Tokyo, Japan). The nodules’ images were acquired in a standard manner. All images included at least 2 orthogonal planes (radial and antiradial planes or transverse and longitudinal planes). According to the ACR BI-RADS fifth edition classification criteria and a previous study (15), all images were analyzed retrospectively by two breast radiologists (reader 1 with 10 years’ experience and reader 2 with 5 years’ experience). The radiologists strictly recorded 15 ultrasonic features, as follows: orientation, shape, echogenic pattern, margin, posterior features, calcifications, vascularity grade, vascularity distribution, background echotexture of parenchyma (BEP), anteroposterior thickness of breast parenchyma (TBP), anteroposterior thickness ratio of breast parenchyma to tissue before pectoralis fascia (RPT), anteroposterior thickness ratio of breast parenchyma to mammary fat (RPF), lymph node metastasis, tumor size, and BI-RADS category. RPT and RPF were the adjusted parameters of TBP. They were acquired after TBP was corrected via thickness of tissue, pectoralis fascia, and fat. The detailed interpretations of all ultrasonic features are listed in Appendix 1. Two radiologists were blinded to the pathological characteristic but not to patient age. Intra- and interobserver agreements of 15 ultrasonic features were evaluated. For the records of each ultrasonic feature, any disagreements between the two radiologists were resolved by final consensus following discussion.
Core biopsy reporting categories (6)
According to histological examination, all lesions were classified into 5 categories based on clinical and ultrasonic characteristics:
- B1 lesion indicates normal tissue.
- B2 indicates benign lesion, including fibroadenomas, fibrocystic change, sclerosing adenosis, and duct ectasia as well as other nonparenchymal lesions such as abscesses and fat necrosis.
- B3 lesion indicates uncertain malignant potential, and this category mainly consists of lesions that may provide benign histology on core biopsy but either are known to show heterogeneity or to be associated with an increased risk of malignancy. The category includes atypical intraductal epithelial proliferations, flat epithelial atypia, lobular neoplasia, phyllodes tumors, papillary lesions, radial scars, mucocele-like lesions, and rare lesions.
- B4 indicates suspicious nodules (technical problems may have led to crushed or poorly fixed cores that contain probable carcinoma that cannot provide a definitive diagnosis). Thus, B4 lesions were excluded in this study.
- B5 indicates malignant nodules.
Statistical analysis
Statistical analysis was performed with SPSS version 22.0 (IBM Corp., Armonk, NY, USA). The statistical significance levels were two-sided, and a P value <0.05 was deemed to be statistically significant.
Multiple comparisons of the B1, B2, B3, and B5 categories
Multiple differences in all characteristics were assessed among the 4 categories. Continuous variables were compared using the least significant difference, whereas categorical variables were compared using the Bonferroni test.
Machine learning in characteristics analysis
RStudio version 1.1.463 (RStudio, The R Foundation for Statistical Computing, Vienna, Austria) was used as R software. Because there are many machine learning models, we chose the more common ones to complete the classification. The machine learning models included were random forest (RF), support vector machine (SVM), k-nearest-neighbor (KNN), multilayer perceptron (MLP), and ridge regression (RR). In operations, all cases were randomly split into training and validation cohorts based on the machine learning model. We achieved normalization for each feature in the data preprocessing steps. For the hyperparameters, we applied a simple tuning process instead of a detailed one since this study did not focus on the optimal hyperparameters. The hyperparameters used for each classifier are shown in Appendix 2.
Performance of machine learning
The maps of distribution were depicted for variables. The table of the validation cohort was used to predict the biopsied categories, including area under the curve (AUC) and accuracy. The receiver operating characteristic (ROC) was provided for every algorithm. According to the highest AUC, we determined the optimal algorithm, whose weight map was provided to show the importance of ultrasonic and clinical features.
Results
Clinical and ultrasonic characteristics
In our study, the proportions of the 4 categories were 4% (44/1,185) for the B1 group, 60% (714/1,185) for the B2 group, 5% (57/1,185) for the B3 group, and 31% (370/1,185) for the B5 group. The baseline clinical and ultrasonic characteristics are listed in Table 1. There were statistically significant differences in 17 features (P<0.05) but not for echo pattern or BEP (P>0.05). The horizontal bar graphs of categorical variables are shown in Figure 2, the histograms of continuous variables are shown in Figure 3, and multiple comparisons of clinical and ultrasonic characteristics are shown in Appendix 3.
Table 1
Features | B1 (n=44) | B2 (n=714) | B3 (n=57) | B5 (n=370) | P |
---|---|---|---|---|---|
Age (years), mean ± SD | 47.55±11.46 | 36.82±11.34 | 42.45±8.73 | 52.02±11.92 | <0.001 |
Height (cm), mean ± SD | 158.68±4.67 | 158.93±4.82 | 159.02±5.26 | 157.67±5.07 | 0.001 |
Weight (kg), mean ± SD | 55.71±8.08 | 53.96±7.68 | 56.34±7.91 | 57.89±8.14 | <0.001 |
BMI (kg/m2), mean ± SD | 22.12±2.98 | 21.37±2.95 | 22.31±3.25 | 23.28±3.09 | <0.001 |
Echo pattern, n [%] | 0.050 | ||||
Hyperechoic | 0 [0] | 0 [0] | 0 [0] | 0 [0] | |
Complex cystic and solid | 0 [0] | 5 [1] | 1 [2] | 2 [1] | |
Hypoechoic | 27 [61] | 332 [46] | 19 [33] | 152 [41] | |
Isoechoic | 0 [0] | 14 [2] | 3 [5] | 11 [3] | |
Heterogeneous | 17 [39] | 363 [51] | 34 [60] | 205 [55] | |
Shape, n [%] | <0.001 | ||||
Oval | 17 [39] | 182 [25] | 12 [21] | 18 [5] | |
Round | 1 [2] | 11 [2] | 0 [0] | 2 [1] | |
Irregular | 26 [59] | 521 [73] | 45 [79] | 350 [95] | |
Margin, n [%] | <0.001 | ||||
Circumscribed | 11 [25] | 222 [31] | 11 [19] | 17 [5] | |
Indistinct | 17 [39] | 115 [16] | 14 [25] | 47 [13] | |
Angular | 14 [32] | 353 [49] | 27 [47] | 167 [45] | |
Microlobulated | 2 [5] | 24 [3] | 5 [9] | 139 [38] | |
Orientation, n [%] | <0.001 | ||||
Parallel | 37 [84] | 648 [91] | 47 [82] | 267 [72] | |
Not parallel | 7 [16] | 66 [9] | 10 [18] | 103 [28] | |
Posterior feature, n [%] | <0.001 | ||||
No posterior feature | 15 [34] | 85 [12] | 9 [16] | 20 [5] | |
Enhancement sound | 4 [9] | 75 [11] | 8 [14] | 21 [6] | |
Shadowing | 13 [30] | 161 [23] | 10 [18] | 63 [17] | |
Combined pattern | 12 [27] | 393 [55] | 30 [53] | 266 [72] | |
Calcification, n [%] | <0.001 | ||||
Within a mass | 4 [9] | 72 [10] | 6 [11] | 119 [32] | |
Outside of a mass | 0 [0] | 3 [0] | 0 [0] | 0 [0] | |
Intraductal calcifications | 0 [0] | 1 [0] | 0 [0] | 1 [0.3] | |
None | 40 [91] | 638 [89] | 51 [89] | 250 [68] | |
Vascularity distribution, n [%] | <0.001 | ||||
Absent | 31 [70] | 260 [36] | 15 [26] | 44 [12] | |
Vessels in rim | 6 [14] | 128 [18] | 15 [26] | 35 [10] | |
Internal | 7 [16] | 326 [46] | 27 [48] | 291 [78] | |
Vascularity grade, n [%] | <0.001 | ||||
Grade I | 31 [70] | 260 [36] | 14 [25] | 45 [12] | |
Grade II | 8 [18] | 271 [38] | 28 [49] | 107 [29] | |
Grade III | 4 [9] | 126 [18] | 13 [23] | 140 [38] | |
Grade IV | 1 [2] | 57 [8] | 2 [4] | 78 [21] | |
Lymph node metastasis reported, n [%] | <0.001 | ||||
No | 43 [98] | 689 [96] | 55 [96] | 255 [69] | |
Yes | 1 [2] | 25 [4] | 2 [4] | 115 [31] | |
BEP, n [%] | 0.281 | ||||
Homogenous | 10 [23] | 148 [21] | 11[19] | 60 [16] | |
Inhomogeneous | 34 [77] | 566 [79] | 46 [81] | 310 [84] | |
BI-RADS category, n [%] | <0.001 | ||||
3 | 2 [5] | 36 [5] | 3 [5] | 1 [0] | |
4A | 15 [34] | 135 [19] | 10 [18] | 7 [2] | |
4B | 15 [34] | 335 [47] | 12 [21] | 37 [10] | |
4C | 11 [25] | 166 [23] | 23 [40] | 96 [26] | |
5 | 1 [2] | 42 [6] | 9 [16] | 229 [62] | |
Tumor size (mm), mean ± SD | 11.76±6.50 | 17.91±9.39 | 18.03±7.90 | 24.46±11.66 | <0.001 |
TBP (mm), mean ± SD | 7.73±3.21 | 8.12±2.93 | 7.75±2.49 | 9.06±4.47 | <0.001 |
RPT, mean ± SD | 0.51±0.14 | 0.52±0.14 | 0.52±0.13 | 0.48±0.21 | 0.004 |
RPF, mean ± SD | 1.96±1.97 | 1.93±1.75 | 1.86±1.23 | 1.53±1.72 | 0.004 |
SD, standard deviation; BMI, body mass index; BEP, background echotexture of parenchyma; BI-RADS, Breast Imaging Reporting and Data System; TBP, anteroposterior thickness of breast parenchyma; RPT, thickness ratio of breast parenchyma to tissue before pectoralis fascia; RPF, thickness ratio of breast parenchyma to mammary fat.
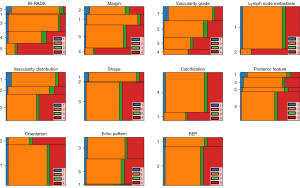
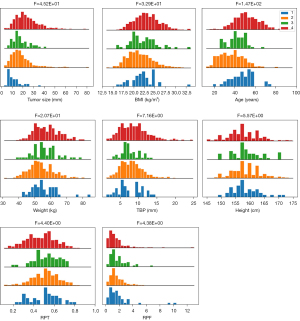
Performance of machine learning
All nodules were split into a training cohort (829 nodules) and a validation cohort (356 nodules). In the validation cohort, the ROCs of 5 algorithms are plotted in Figure 4. The prediction results, AUC, and accuracy of the 5 algorithms are listed in Table 2.
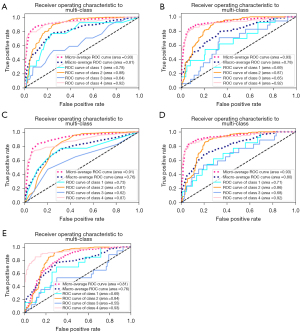
Table 2
Model | HIS | PRE | |||||
---|---|---|---|---|---|---|---|
B1 | B2 | B3 | B5 | AUC | Accuracy | ||
RF | B1 | 0 | 12 | 0 | 1 | 0.78 | 0.82 |
B2 | 0 | 204 | 0 | 11 | 0.88 | ||
B3 | 0 | 16 | 0 | 1 | 0.64 | ||
B5 | 0 | 23 | 0 | 88 | 0.92 | ||
SVM | B1 | 0 | 13 | 0 | 0 | 0.69 | 0.81 |
B2 | 0 | 204 | 0 | 11 | 0.87 | ||
B3 | 0 | 15 | 0 | 2 | 0.65 | ||
B5 | 0 | 27 | 0 | 84 | 0.92 | ||
KNN | B1 | 0 | 13 | 0 | 0 | 0.73 | 0.77 |
B2 | 0 | 210 | 0 | 5 | 0.81 | ||
B3 | 0 | 16 | 0 | 1 | 0.62 | ||
B5 | 0 | 46 | 0 | 65 | 0.87 | ||
MLP | B1 | 1 | 10 | 0 | 2 | 0.71 | 0.80 |
B2 | 1 | 198 | 0 | 16 | 0.86 | ||
B3 | 1 | 15 | 0 | 1 | 0.68 | ||
B5 | 0 | 25 | 0 | 86 | 0.92 | ||
RR | B1 | 0 | 10 | 0 | 3 | 0.69 | 0.81 |
B2 | 0 | 203 | 0 | 12 | 0.84 | ||
B3 | 0 | 16 | 0 | 1 | 0.55 | ||
B5 | 0 | 24 | 0 | 87 | 0.93 |
AUC, area under the curve; HIS, actual histological result; PRE, predicted histological result; RF, random forest; SVM, support vector machine; KNN, k-nearest-neighbor; MLP, multilayer perceptron; RR, ridge regression.
According to the above results, we found that RF was the best algorithm for predicting CBCs. The AUCs were 0.78, 0.88, 0.64, and 0.92, for B1, B2, B3, and B5, respectively. In summary, RF showed the best predictive ability with an accuracy of 0.82. Since each rank variable was treated as an independent variable in the categorical variables, up to 45 features were included in RF. Because the top 10 variables play a major role in building the model, the weight map of these variables is shown in Figure 5.
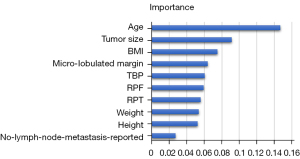
Discussion
Our study demonstrated relationships between several clinical and almost all US features with biopsy categories of solid tumors of the breast. Based on clinical and US features, machine learning models were used to predict CBC. This is in contrast to a previous study, in which MRI features of B2, B3, and B5 lesions were analyzed in only 61 cases and focused solely on the distribution of MRI features (16). Another study investigated several clinical and US features of 102 B3 lesions but did not analyze in detail the relationship between B3 lesions and each feature of the BI-RADS lexicon (17). To the best of our knowledge, there are no previous studies that have used machine learning models to predict CBC based on US features.
Ultrasonic terminologies of BI-RADS are used to depict the morphology and function of breast tumors. However, each feature can exist in both benign and malignant tumors. Hypoecho, calcification, and abundant vascularity have been reported in benign and malignant tumors (18-20); a similar phenomenon was found in our study (Table 1). Benign and malignant lesions cannot be accurately predicted with a single US feature or clinical feature. Therefore, a model that assesses the characteristics of breast lesions is needed.
Machine learning is beneficial for building a predictive model and plays an important role in radiological studies (9,21). In our study, we used 5 machine learning models to analyze US and clinical features and to conduct multiclassification for predicting CBC. Our study showed outstanding performance of the 5 machine learning models in predicting the B2 and B5 lesions, but they could only barely predict B1 or B3 lesions (Table 2). We tried to retrain the RF model by overrepresenting B1 and B3 within the same class. However, there was no significant improvement using this approach (Appendix 4).
Similar to previous ultrasonic studies (22,23), our study found that RF showed the best overall performance among the 5 machine learning models (Table 2). RF contains multiple decision trees, and the output category is determined by the mode of the category output by the individual tree. Therefore, RF is advantageous for conducting the classification. According to the weight map of RF, we found that the top 10 features were age, tumor size, BMI, microlobulated margin, TBP, RPF, RPT, weight, height, and no reported lymph node metastasis.
Age is not included in the risk stratification of ACR US BI-RADS, but it influences the doctor’s determination. Increasing age is closely related to a higher incidence of breast cancer worldwide (24,25). Ordinarily, tumor size is not considered to be a risk factor in US BI-RADS. Nevertheless, based on the tumor biological behavior, the faster the tumor size increases, the higher the possibility of malignancy. BMI showed a strong ability to predict CBC. BMI was highest in the B5 category, and a higher BMI indicated a greater likelihood for malignancy. It has long been recognized that overweight and obese individuals are at increased risk of postmenopausal breast cancer, notably hormone receptor-positive cancers (26). In our study, increased age and higher BMI were associated with a higher CBC.
Lesions with microlobulated margins are likely to be classified as malignant (27). The greater desmoplastic reaction leads to noncircumscribed margins, including microlobulated margins (28). As the parameters of breast parenchyma, TBP, RPF, and RPT performed well in our predictive task. The performance of TBP was very strong, and the TBP of the B5 category was the greatest. The interaction of tumor cells and host stromal cells is associated with breast cancer, and the parenchymal stromal cells play an important role in the formation and development of breast cancer, acting as a matrix to promote tumor growth (29,30). A small number of ultrasonic studies found that thick breast parenchyma promotes the occurrence of breast cancer (31-34). Similar to previous studies (35,36), our study found that the reported lymphatic metastasis was able to predict malignancy.
Our study was not without limitation. First, although RF was determined as the optimal machine learning for CBC, it missed 13 B5 and B2 lesions. As expert mathematicians further perfect the RF, we will try to use the latest RF algorithm to improve the accuracy of CBC prediction in the future. Second, the sample size was small, particularly in the B1 and B3 categories. A larger data set is needed to validate the robustness of RF and to build the application to automatically predict the preoperative biopsy category. Third, the study did not integrate other clinical risk factors due to the inherent incompleteness of the retrospective study data. Prospective studies with complete data sets (i.e., those including family history, serological, and menopausal period) should be carried out. Last but not the least, the subjective analysis of some ultrasonic features led to inevitable deviation. Consequently, objective parameters (i.e., radiomics features and contrast-enhanced US) should be used to improve this model.
Conclusions
Based on clinical and ultrasonic features, we used machine learning models to predict the CBCs of solid breast tumors. Of the 5 models, RF was the optimal machine learning model, as it had the highest accuracy of 0.82. Especially for B2 and B5, RF performed well, and its AUCs were 0.88 and 0.92, respectively. In practice, our study can aid in clinically precise individualized management with BI-RADS and can also assist in developing a new US imaging reporting system for solid breast tumors.
Acknowledgments
We thank all the study participants and the medical teams who contributed to this study.
Funding: This work was supported by the Guangdong Medical Science and Technology Research Fund (Nos. A2022225, B2022089, and C2018001).
Footnote
Reporting Checklist: The authors have completed the TRIPOD reporting checklist. Available at https://qims.amegroups.com/article/view/10.21037/qims-22-877/rc
Conflicts of Interest: All authors have completed the ICMJE uniform disclosure form (available at https://qims.amegroups.com/article/view/10.21037/qims-22-877/coif). The authors have no conflicts of interest to declare.
Ethical Statement: The authors are accountable for all aspects of the work in ensuring that questions related to the accuracy or integrity of any part of the work are appropriately investigated and resolved. The study was conducted in accordance with the Declaration of Helsinki (as revised in 2013). This study was approved by the institutional review board of Guangdong Provincial People’s Hospital, Guangdong Academy of Medical Sciences. The informed consent requirement was waived due to the nature of the retrospective study.
Open Access Statement: This is an Open Access article distributed in accordance with the Creative Commons Attribution-NonCommercial-NoDerivs 4.0 International License (CC BY-NC-ND 4.0), which permits the non-commercial replication and distribution of the article with the strict proviso that no changes or edits are made and the original work is properly cited (including links to both the formal publication through the relevant DOI and the license). See: https://creativecommons.org/licenses/by-nc-nd/4.0/.
References
- Neal L, Sandhu NP, Hieken TJ, Glazebrook KN, Mac Bride MB, Dilaveri CA, Wahner-Roedler DL, Ghosh K, Visscher DW. Diagnosis and management of benign, atypical, and indeterminate breast lesions detected on core needle biopsy. Mayo Clin Proc 2014;89:536-47. [Crossref] [PubMed]
- Rungruang B, Kelley JL 3rd. Benign breast diseases: epidemiology, evaluation, and management. Clin Obstet Gynecol 2011;54:110-24. [Crossref] [PubMed]
- Yap YS, Lu YS, Tamura K, Lee JE, Ko EY, Park YH, Cao AY, Lin CH, Toi M, Wu J, Lee SC. Insights Into Breast Cancer in the East vs the West: A Review. JAMA Oncol 2019;5:1489-96. [Crossref] [PubMed]
- Sardanelli F, Boetes C, Borisch B, Decker T, Federico M, Gilbert FJ, et al. Magnetic resonance imaging of the breast: recommendations from the EUSOMA working group. Eur J Cancer 2010;46:1296-316. [Crossref] [PubMed]
- Shen S, Zhou Y, Xu Y, Zhang B, Duan X, Huang R, Li B, Shi Y, Shao Z, Liao H, Jiang J, Shen N, Zhang J, Yu C, Jiang H, Li S, Han S, Ma J, Sun Q. A multi-centre randomised trial comparing ultrasound vs mammography for screening breast cancer in high-risk Chinese women. Br J Cancer 2015;112:998-1004. [Crossref] [PubMed]
- Lee A, Anderson N, Carder P, Cooke J, Deb R, Ellis IO, Howe M, Jenkins JA, Knox F, Stephenson T. Guidelines for non-operative diagnostic procedures and reporting in breast cancer screening. London: The Royal College of Pathologists, 2016.
- Narula S, Shameer K, Salem Omar AM, Dudley JT, Sengupta PP. Machine-Learning Algorithms to Automate Morphological and Functional Assessments in 2D Echocardiography. J Am Coll Cardiol 2016;68:2287-95. [Crossref] [PubMed]
- Nanayakkara S, Fogarty S, Tremeer M, Ross K, Richards B, Bergmeir C, Xu S, Stub D, Smith K, Tacey M, Liew D, Pilcher D, Kaye DM. Characterising risk of in-hospital mortality following cardiac arrest using machine learning: A retrospective international registry study. PLoS Med 2018;15:e1002709. [Crossref] [PubMed]
- Zhang B, Tian J, Pei S, Chen Y, He X, Dong Y, Zhang L, Mo X, Huang W. Thyroid 2019;29:858-67. [Crossref] [PubMed]
- Achilonu OJ, Fabian J, Bebington B, Singh E, Eijkemans MJC, Musenge E. Predicting Colorectal Cancer Recurrence and Patient Survival Using Supervised Machine Learning Approach: A South African Population-Based Study. Front Public Health 2021;9:694306. [Crossref] [PubMed]
- Yu KH, Lee TM, Yen MH, Kou SC, Rosen B, Chiang JH, Kohane IS. Reproducible Machine Learning Methods for Lung Cancer Detection Using Computed Tomography Images: Algorithm Development and Validation. J Med Internet Res 2020;22:e16709. [Crossref] [PubMed]
- Bitencourt AGV, Gibbs P, Rossi Saccarelli C, Daimiel I, Lo Gullo R, Fox MJ, Thakur S, Pinker K, Morris EA, Morrow M, Jochelson MS. MRI-based machine learning radiomics can predict HER2 expression level and pathologic response after neoadjuvant therapy in HER2 overexpressing breast cancer. EBioMedicine 2020;61:103042. [Crossref] [PubMed]
- Sutton EJ, Onishi N, Fehr DA, Dashevsky BZ, Sadinski M, Pinker K, Martinez DF, Brogi E, Braunstein L, Razavi P, El-Tamer M, Sacchini V, Deasy JO, Morris EA, Veeraraghavan H. A machine learning model that classifies breast cancer pathologic complete response on MRI post-neoadjuvant chemotherapy. Breast Cancer Res 2020;22:57. [Crossref] [PubMed]
- Wu T, Sultan LR, Tian J, Cary TW, Sehgal CM. Machine learning for diagnostic ultrasound of triple-negative breast cancer. Breast Cancer Res Treat 2019;173:365-73. [Crossref] [PubMed]
- Lin X, Zhuang S, Yang S, Lai D, Chen M, Zhang J. Development and internal validation of a conventional ultrasound-based nomogram for predicting malignant nonmasslike breast lesions. Quant Imaging Med Surg 2022;12:5452-61. [Crossref] [PubMed]
- Meucci R, Pistolese Chiara A, Perretta T, Vanni G, Portarena I, Manenti G, Ryan Colleen P, Castrignanò A, Di Stefano C, Ferrari D, Lamacchia F, Pellicciaro M, Materazzo M, Buonomo Oreste C. MR imaging-guided vacuum assisted breast biopsy: Radiological-pathological correlation and underestimation rate in pre-surgical assessment. Eur J Radiol Open 2020;7:100244. [Crossref] [PubMed]
- Giuliani M, Rinaldi P, Rella R, D'Angelo A, Carlino G, Infante A, Romani M, Bufi E, Belli P, Manfredi R. A new risk stratification score for the management of ultrasound-detected B3 breast lesions. Breast J 2018;24:965-70. [Crossref] [PubMed]
- Kim YR, Kim HS, Kim HW. Are Irregular Hypoechoic Breast Masses on Ultrasound Always Malignancies?: A Pictorial Essay. Korean J Radiol 2015;16:1266-75. [Crossref] [PubMed]
- Grigoryev M, Thomas A, Plath L, Durmus T, Slowinski T, Diekmann F, Fischer T. Detection of microcalcifications in women with dense breasts and hypoechoic focal lesions: comparison of mammography and ultrasound. Ultraschall Med 2014;35:554-60. [Crossref] [PubMed]
- Park AY, Kwon M, Woo OH, Cho KR, Park EK, Cha SH, Song SE, Lee JH, Cha J, Son GS, Seo BK. A Prospective Study on the Value of Ultrasound Microflow Assessment to Distinguish Malignant from Benign Solid Breast Masses: Association between Ultrasound Parameters and Histologic Microvessel Densities. Korean J Radiol 2019;20:759-72. [Crossref] [PubMed]
- Lu CF, Hsu FT, Hsieh KL, Kao YJ, Cheng SJ, Hsu JB, Tsai PH, Chen RJ, Huang CC, Yen Y, Chen CY. Machine Learning-Based Radiomics for Molecular Subtyping of Gliomas. Clin Cancer Res 2018;24:4429-36. [Crossref] [PubMed]
- Destrempes F, Trop I, Allard L, Chayer B, Garcia-Duitama J, El Khoury M, Lalonde L, Cloutier G. Added Value of Quantitative Ultrasound and Machine Learning in BI-RADS 4-5 Assessment of Solid Breast Lesions. Ultrasound Med Biol 2020;46:436-44. [Crossref] [PubMed]
- Wildeboer RR, Mannaerts CK, van Sloun RJG, Budäus L, Tilki D, Wijkstra H, Salomon G, Mischi M. Automated multiparametric localization of prostate cancer based on B-mode, shear-wave elastography, and contrast-enhanced ultrasound radiomics. Eur Radiol 2020;30:806-15. [Crossref] [PubMed]
- Fan L, Strasser-Weippl K, Li JJ, St Louis J, Finkelstein DM, Yu KD, Chen WQ, Shao ZM, Goss PE. Breast cancer in China. Lancet Oncol 2014;15:e279-89. [Crossref] [PubMed]
- Hu Y, Yang Y, Gu R, Jin L, Shen S, Liu F, Wang H, Mei J, Jiang X, Liu Q, Su F. Does patient age affect the PPV(3) of ACR BI-RADS Ultrasound categories 4 and 5 in the diagnostic setting? Eur Radiol 2018;28:2492-8. [Crossref] [PubMed]
- Jiralerspong S, Goodwin PJ. Obesity and Breast Cancer Prognosis: Evidence, Challenges, and Opportunities. J Clin Oncol 2016;34:4203-16. [Crossref] [PubMed]
- Aho M, Irshad A, Ackerman SJ, Lewis M, Leddy R, Pope TL, Campbell AS, Cluver A, Wolf BJ, Cunningham JE. Correlation of sonographic features of invasive ductal mammary carcinoma with age, tumor grade, and hormone-receptor status. J Clin Ultrasound 2013;41:10-7. [Crossref] [PubMed]
- Irshad A, Leddy R, Pisano E, Baker N, Lewis M, Ackerman S, Campbell A. Assessing the role of ultrasound in predicting the biological behavior of breast cancer. AJR Am J Roentgenol 2013;200:284-90. [Crossref] [PubMed]
- Fidler IJ, Poste G. The "seed and soil" hypothesis revisited. Lancet Oncol 2008;9:808. [Crossref] [PubMed]
- Li H, Mendel KR, Lan L, Sheth D, Giger ML. Digital Mammography in Breast Cancer: Additive Value of Radiomics of Breast Parenchyma. Radiology 2019;291:15-20. [Crossref] [PubMed]
- Hou XY, Niu HY, Huang XL, Gao Y. Correlation of Breast Ultrasound Classifications with Breast Cancer in Chinese Women. Ultrasound Med Biol 2016;42:2616-21. [Crossref] [PubMed]
- Kim WH, Lee SH, Chang JM, Cho N, Moon WK. Background echotexture classification in breast ultrasound: inter-observer agreement study. Acta Radiol 2017;58:1427-33. [Crossref] [PubMed]
- Chang RF, Hou YL, Lo CM, Huang CS, Chen JH, Kim WH, Chang JM, Bae MS, Moon WK. Quantitative analysis of breast echotexture patterns in automated breast ultrasound images. Med Phys 2015;42:4566-78. [Crossref] [PubMed]
- Liu Z, Li Z, Qu J, Zhang R, Zhou X, Li L, Sun K, Tang Z, Jiang H, Li H, Xiong Q, Ding Y, Zhao X, Wang K, Liu Z, Tian J. Radiomics of Multiparametric MRI for Pretreatment Prediction of Pathologic Complete Response to Neoadjuvant Chemotherapy in Breast Cancer: A Multicenter Study. Clin Cancer Res 2019;25:3538-47. [Crossref] [PubMed]
- Jiang T, Su W, Zhao Y, Li Q, Huang P. Non-invasive prediction of lymph node status for patients with early-stage invasive breast cancer based on a morphological feature from ultrasound images. Quant Imaging Med Surg 2021;11:3399-407. [Crossref] [PubMed]
- Zheng X, Yao Z, Huang Y, Yu Y, Wang Y, Liu Y, Mao R, Li F, Xiao Y, Wang Y, Hu Y, Yu J, Zhou J. Deep learning radiomics can predict axillary lymph node status in early-stage breast cancer. Nat Commun 2020;11:1236. [Crossref] [PubMed]