Preoperative 18F-FDG PET/CT radiomics analysis for predicting HER2 expression and prognosis in gastric cancer
Introduction
Gastric cancer (GC) incurs a heavy global health burden. In 2018, there were over 1,000,000 new cases and about 783,000 deaths from GC, making it the fifth leading cause of cancer death worldwide (1). Furthermore, Eastern Asian countries had the highest incidence (2). In recent decades, molecular targeted therapies have revolutionized the standard treatment of some tumors (3,4). As the first approved targeted drug for human epidermal growth factor receptor 2 (HER2)-positive GC patients, trastuzumab marked a significant turning point in the first-line therapy of GC. It has significantly improved the prognosis of GC patients (5,6), but accurate assessment of HER2 status is critical to identifying suitable patients for targeted treatment. In clinical practice, immunohistochemistry (IHC) or fluorescence in situ hybridization (FISH) testing is mainly conducted with biopsy or surgical specimens. However, HER2 expression is typically heterogeneous and dynamic (7). Moreover, inadequate tumor tissue from biopsies may also lead to inaccurate results. These issues emphasize the necessity to predict the status of HER2 through a non-invasive approach.
The high recurrence after adjuvant chemotherapy is another problem faced by GC patients. The results on the prognosis of GC patients after adjuvant chemotherapy have varied among several clinical trials (8-10). According to one clinical trial, the overall survival (OS) of the adjuvant chemotherapy group was moderately improved compared to the surgery-only group (78% vs. 69%), indicating that some GC patients do not benefit from adjuvant chemotherapy (10). Additionally, previous studies (11,12) have shown significant variation in prognosis in patients with the same clinical stage and similar treatment strategies, which implies that more prognostic information and understanding of the biological heterogeneity of GC are required for precise treatment. Consequently, exploring the risk of recurrence and the potential benefit of chemotherapy in GC patients has been a topic in individualized treatment. Some studies have demonstrated that several biomarkers can predict the prognosis of GC patients (13,14), but most of the reported markers lack reproducibility, and limited biological information of tumors is involved.
Numerous studies have shown the predictive value of 18F-flurodeoxyglucose positron emission tomography/computed tomography (18F-FDG PET/CT) in GC staging, identifying metastases, and therapeutic evaluation (15-17). Moreover, the relationship between 18F-FDG uptake and HER2 mutation status has also been validated (18,19). In recent years, radiomics (20,21) has emerged as a high-throughput image analysis process that extracts a high dimension of quantitative imaging features and characterizes the related tumor phenotype (18). It is also considered a promising research field because it is non-invasive. Many radiomics predictive models have shown great promise in the diagnosis and treatment of multiple cancers (22-24), and some studies have investigated the value of CT-based radiomics in detecting metastasis and predicting early recurrence in GC (25-27).
To our knowledge, the predictive value of 18F-FDG PET/CT radiomics in GC has not been extensively investigated. Therefore, we retrospectively collected the pretreatment 18F-FDG PET/CT data of patients with GC with the aim of confirming the high diagnostic performance of preoperative 18F-FDG PET/CT-based radiomics analysis in differentiating HER2 positive (HER2+) expression from HER2 negative (HER2−) expression and for prognosis prediction. This could be considered as a non-invasive treatment decision support system that potentially can assist physicians in decision making for optimal therapeutic strategies. We present the following article in accordance with the TRIPOD reporting checklist (available at https://qims.amegroups.com/article/view/10.21037/qims-22-148/rc).
Methods
Patients
The study was conducted in accordance with the Declaration of Helsinki (as revised in 2013). It was approved by the Ethics Committee of Fudan University Shanghai Cancer Center (No. 1909207-14-1910), and individual consent for this retrospective analysis was waived. We retrospectively reviewed patients with GC (n=90) who underwent total or partial radical gastrectomy and adjuvant chemotherapy at Fudan University Shanghai Cancer Hospital between January 2016 and December 2017. Patients were followed up until March 2019. Tumor-node-metastasis (TNM) staging was conducted according to the American Joint Committee on Cancer TNM Staging Manual, 8th edition (28). The inclusion criteria were as follows: (I) patient diagnosed with GC by surgically resected specimens; (II) HER2 IHC staining performed with primary GC tissue; (III) available clinical data such as sex, age, tumor size, and TNM staging; and (IV) available 18F-FDG PET/CT scan data before treatment.
For HER2 IHC analysis, the surgical tissue samples were formalin-fixed and paraffin-embedded before IHC staining using the HercepTestTM Kit (Dako, Glostrup, Denmark) according to the manufacturer’s instructions. An HER2+ status was defined as IHC 3+, IHC 2+, or FISH-positive, and IHC 0 or IHC 1 was considered HER2− (29). Additionally, progression-free survival (PFS) was used to evaluate the prognosis of the patients, which was defined as time to progression or death after the surgery.
All enrolled patients were followed up for at least 1 year, which included serum tumor marker testing, abdominopelvic imaging [CT or magnetic resonance imaging (MRI)] or gastroscopy. Disease progression referred to recurrence of the primary tumor, new metastasis, or at least 20% increase in the sum of the diameters of lesions after chemotherapy according to the Response Evaluation Criteria in Solid Tumors (RECIST 1.1). Based on the findings, the enrolled patients were divided into disease-progression and non-disease-progression groups.
18F-FDG PET/CT scan and image analysis
18F-FDG PET/CT scans were performed with a whole-body PET/CT scanner (Biograph 16 HR; Siemens Medical Systems, Erlangen, Germany). Patients fasted for at least 6 hours, and blood glucose levels had to be <140 mg/dL before the injection of 18F-FDG (3.7 MBq/kg). Scanning was started approximately 1 hour later. CT images were acquired first, with a low-dose technique (120 kV, 140 mA, 5-mm slice thickness), and then positron emission tomography (PET) scanning with the following parameters: 2–3 min/bed with Gaussian-filter iterative reconstruction method (iterations 4; subsets 8; image size 168) for the reconstruction. Two experienced nuclear medicine physicians evaluated the PET/CT images, and the tumor parameters were measured using a computer platform (Syngo; Siemens, Knoxville, TN, USA), including maximum standardized uptake value (SUVmax), metabolic tumor volume (MTV40%, MTV2.5), and total lesion glycolysis (TLG40%, TLG2.5), according to published articles (18).
Medical image segmentation
The volumes of interest (VOIs) in the tumor were segmented slice-by-slice using open-source ITK-SNAP software (version 3.6; http://www.itksnap.org/pmwiki/pmwiki.php) (30) by 2 attending physicians from the Department of Nuclear Medicine with >5 years’ experience. In the case of a difference of opinion, another attending physician with >15 years’ experience adjudicated the decision. All physicians were unaware of the pathological results. Since the 2 imaging modalities were co-registered, only the VOIs of the PET images were hand-segmented and then correlated to the CT images through coordinate transform and nearest neighbor interpolation. This interpolation function selected the value of the nearest point without considering other neighboring values. Moreover, because the CT images had a much higher resolution than the PET images, this interpolation function guaranteed accuracy and efficiency without intensive computation. The radiologists still verified the generated VOIs of each CT image to ensure reliability.
Radiomic feature extraction
The radiomics workflow for predicting the HER2 expression and prognosis is summarized in Figure 1, comprising 4 principal stages: radiomic feature extraction, representative feature selection, predictive model construction, and statistical analysis. Before extracting the high-quantitative features, we applied SUV normalization of the PET images based on the body weights and injection doses of the patients, followed by a discretization process with a fixed bin width of 0.25, and for the CT images, features were computed based on a resampled voxel dimensions of 2×2×2 mm3 and a 25 bin width calculated on Hounsfield units (HU) (31). Other parameters were set to the default value provided by pyradiomics (31). For feature extraction, there were 1,050 high-quantitative imaging features extracted from VOIs delineated on the PET and CT images. Radiomic features included 4 major groups: shape features (n=14), statistical features (n=18), texture features (n=56), and higher-order statistical features [consisting of 370 Laplacian of Gaussian (LoG)-filtered features, and 592 wavelet-filtered features]. Shape features describe the geometric properties of the focused VOIs, such as the surface area. Statistical features describe the intensity distribution histogram of the image voxels. Texture features describe the statistical inter-relationships among neighboring voxels that provide the spatial arrangement via multiple matrices such as the gray-level co-occurrence matrix (GLCM) and gray-level run-length matrix (GLRLM). The fractal features identify repetitive or non-repetitive patterns and highlight details at different coarseness levels of texture patterns by applying filters or mathematical transforms such as wavelet transformation and LoG. The radiomic feature extraction process was implemented through the scikit-learn (sklearn) package (31) and PyRadiomics (2.0.1) (31) in Python version 3.6.4, which are a machine learning library and an open-source Python package compliant with the instructions of the Image Biomarker Standardization Initiative reference manual (32), respectively.
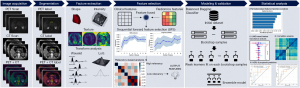
Feature selection
We fused the extracted 2,100 high-quantitative imaging features with 28 clinical features and 30 clinical features for HER2 expression and the prognosis prediction, respectively, before implementing the feature selection. The feature selection strategy was designed to reduce the high dimensionality of the feature space and mined the embedded pattern based on the outcome-driven machine learning model. As illustrated in Figure 2A, we applied a sequential combination of multivariate and univariate selection on each fused feature pool. Additionally, feature selection was applied on different fused feature pools for different classification tasks (Figure 2B). For multivariate feature selection, the sequential forward feature selection (SFS) method was applied to automatically select the optimal feature set based on the prediction performance. The cardinality of the feature subset started incrementing from 1 and incremented by 1 until all combinations of a user-defined subset length were covered. The combination that maximized 10-fold cross-validation performance was chosen in each iteration, primarily accuracy and the area under curve (AUC). The performance of SFS for HER2 and prognosis prediction is shown in Figure 3. Next, univariate selection was used to select the final discriminative feature set through a redundancy-based analysis, including analyzing the statistical correlation among selected features and comparing the performance after eliminating each statistically correlated feature.
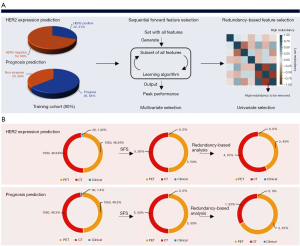
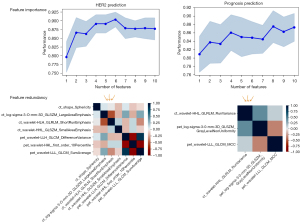
Modeling and validation
The patients were split into training and independent validation datasets using a stratified method based on an 8:2 ratio. We then introduced an ensemble classifier—a balanced bagging classifier as the overall framework for HER2 and prognosis prediction because the class ratio for the initial dataset was slightly imbalanced. We also applied the Adaboost classifier as the base classifier in the balanced bagging approach due to its superior performance within the balanced bagging compared with others, which improved the stability and accuracy of the algorithms. Since the final prediction was generated through voting from multiple base classifiers (Adaboost) within the ensemble model, it reduced the variance and prevented the model from overfitting. To validate the robustness and stability of each predictive model, 5-fold cross-validation on the training set and independent validation methods were utilized. The performance of the model was primarily evaluated by accuracy, sensitivity, specificity, and AUC. The positive and negative predictive values were also computed through the confusion matrix.
Statistical analysis
Statistical analysis contained the feature importance ranking, the contribution of CT features and PET features to the final prediction, and correlation analysis among the selected features for each task with the clinical features. We applied different methods to evaluate the feature importance based on different scales to enhance the reliability of the selected features. The feature importance ranking was implemented continuously, and randomly permuted and scored each variable to evaluate the representative value of selected features through the scale of the whole testing dataset, and the local interpretable model-agnostic explanations (LIME) model was implemented to evaluate the contribution of CT and PET features to the final prediction through its derived coefficients based on the scale of each patient in the testing dataset (33). As for the correlation analysis, Mann-Whitney U tests were used to assess the difference between patients in the training and independent validation cohorts. The Pearson method was used for evaluating the correlation between the selected radiomic features and the clinical features. All statistical analyses were implemented using Python package version 3.6.4, and P<0.05 (2-sided) was considered statistically significant.
Results
Patient characteristics
From January 2016 to December 2017, 90 patients with diagnosed GC were enrolled in this retrospective study. The training cohort comprised 46 males and 26 females (age range 22–86 years, median 61 years), and the validation cohort included 11 males and 7 females (age range 47–79 years, median 63 years). Histopathological analyses revealed adenocarcinoma in 58 patients (64.4%), signet ring cell carcinoma (SRCC) in 10 patients (11.1%), and mixed adenocarcinoma in 22 patients (24.4%). GC patients with HER2+ expression accounted for 31.1% (n=28), and the median PFS was 7.9 months. There was a significant difference between HER2+ and HER2− patients in terms of SUVmax (P=0.04), but no obvious difference was found in MTV, TLG, and different prognosis (P>0.05). We also found that 36.7% (n=33) of the patients had a poor prognosis after further follow-up. There were no statistically significant differences in category, age, sex, and clinical features (histopathological type, Lauren subtype, differentiation, etc.) between the training and validation cohorts (P>0.05). More details of the demographic and clinical characteristics of the enrolled patients are displayed in Table 1.
Table 1
Characteristics | Training cohort (n=72) | External validation cohort (n=18) | P |
---|---|---|---|
Age, median [range] | 61 [22–86] | 63 [47–79] | 0.15 |
Gender, n (%) | 0.42 | ||
Male | 46 (63.9) | 11 (61.1) | |
Female | 26 (36.1) | 7 (38.9) | |
Histopathological type, n (%) | 0.13 | ||
Adenocarcinoma | 48 (66.7) | 10 (55.6) | |
Signet ring cell carcinoma | 9 (12.5) | 1 (5.6) | |
Mixed adenocarcinoma | 15 (20.8) | 7 (38.9) | |
Lauren type, n (%) | |||
Intestinal type | 25 (34.7) | 8 (44.4) | 0.20 |
Diffuse type | 25 (34.7) | 6 (33.3) | |
Mixed type | 22 (30.6) | 4 (22.2) | |
Differentiation, n (%) | 0.25 | ||
Low | 37 (51.4) | 9 (50.0) | |
Middle-low | 25 (34.7) | 4 (22.2) | |
Middle | 10 (13.9) | 3 (16.7) | |
High | 0 (0.0) | 2 (11.1) | |
HER2 expression, n (%) | 0.41 | ||
HER2 positive | 22 (30.6) | 6 (33.3) | |
HER2 negative | 50 (69.4) | 12 (66.7) | |
PFS, n (%) | 0.42 | ||
Progress | 46 (63.9) | 11 (61.1) | |
Non-progress | 26 (36.1) | 7 (38.9) | |
SUVmax, mean (standard deviation) | 8.17 (5.14) | 7.06 (3.54) | 0.37 |
40% MTV, mean (standard deviation) | 36.94 (30.37) | 36.52 (33.11) | 0.41 |
40% TLG, mean (standard deviation) | 312.29 (1,301.66) | 127.86 (92.19) | 0.42 |
P<0.05 is considered statistically significant. HER2, human epidermal growth factor receptor 2; PFS, progression-free survival; SUVmax, maximum standardized uptake value; MTV, metabolic volume; TLG, total lesion glycolysis.
Results of feature selection
For the task of predicting HER2 expression, the fused feature pool contained 2,100 radiomic features extracted from PET and CT images and 28 clinical-biological features. There were 5 PET features, and 5 CT features selected through multivariate selection based on the feature importance ranking (Figure 3), and the final discriminative feature set comprising 4 CT features and 3 PET features was selected in univariate selection through redundancy analysis (Figure 3) for model construction. For the prognosis prediction task, 5 PET features and 5 CT features were selected from the fused feature pool composed of 2,100 radiomic features and 30 clinical-biological features during multivariate selection (Figure 3), and after redundancy-based analysis, only 1 CT feature and 2 PET features remained.
Performance of the radiomics signature
The performance of the selected radiomics signatures for each prediction task is displayed in Figure 4. The ensemble model established with 7 radiomic features to predict HER2 expression achieved 83.3% accuracy (sensitivity, 84.6%; specificity, 80%; AUC, 72.2%). The predicted accuracy for HER2+ and HER2− expression is shown in Figure 4C. As for prognosis prediction, the receiver operating characteristic (ROC) curves of the radiomics signature reached 75.3% (Figure 4B). Moreover, the predictive model for prognosis also showed superior performance with an accuracy of 77.8% (sensitivity, 81.8%; specificity, 71.4%; AUC, 75.3%). This model achieved 81.8% and 71.4% accuracy for predicting each target class (progression vs. non-progression, respectively). More importantly, based on the sensitivity and specificity values for each prediction task, there was no sign of bias within the model. The detailed performance of the radiomics signatures for each prediction task is summarized in Table 2.
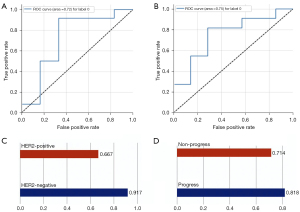
Table 2
Evaluation | Accuracy (SD) | AUC (SD) | Sensitivity (SD) | Specificity (SD) |
---|---|---|---|---|
HER2 | 83.3% (4.09%) | 72.2% (3.61%) | 84.6% (5.44%) | 80% (7.34%) |
Prognosis | 77.8% (4.53%) | 75.3% (4.13%) | 81.8% (6.88%) | 71.4% (6.32%) |
SD, standard deviation; AUC, area under curve; CI, confidence interval; HER2, human epidermal growth factor receptor 2.
Feature analysis and interpretation
There were 7 features selected to predict HER2 expression, including 4 CT features (2 wavelet decomposed features, 1 LoG decomposed feature, 1 shape feature) and 3 wavelet decomposed PET features. According to the feature importance ranking shown in Figure 5A, all the wavelet decomposed features from both the CT and PET images contributed positively to the final prediction, suggesting a significant role of wavelet decomposed features in the prediction of HER2 expression. Moulin claimed that the significance of wavelet decomposed features could be interpreted from both the mathematical and psychophysics perspective (34). From the mathematical standpoint, the regularity and vanishing moment properties of its filter affect the ability to efficiently represent typical images by affecting the shape of the bias functions, whereas from the psychophysical perspective, multiscale and multi-orientation decompositions were indeed natural and efficient for visual information processing (34). We also computed the overall PET and CT feature contribution to the final prediction in the validation set using the LIME model (33) (Figure 5B), and the results aligned with the feature importance ranking that CT features contributed more than PET features. Therefore, based on the model performance and the literature support, the reasonableness of the selected features could be justified, and their application might improve diagnostic performance in distinguishing HER2+ expression from HER2− expression. There were 3 features selected for prognosis prediction, comprising 2 PET features and 1 CT feature, but only 2 of them (wavelet decomposed CT feature and LoG decomposed PET feature) were considered to contribute to the prediction task based on the importance feature score shown in Figure 5C. To further analyze the reason for this, a correlation analysis of the 3 selected features and the clinical features that were defaulted to be effective in the diagnosis of the prognosis was conducted. The wavelet decomposed CT feature was statistically correlated to infiltration depth (P=0.017), SUV (P=2e-3), and TLG (P<1e-5). The LoG decomposed PET feature was only statistically correlated to the histopathological type (P=0.017), and the wavelet decomposed PET feature did not correlate to any of the clinical features. This result could be a proper interpretation of the feature importance ranking shown in Figure 5C. As the importance score for the LoG decomposed PET feature weighted more than the CT feature, we might infer that the value of histopathological type was superior to infiltration depth, SUV, and TLG.
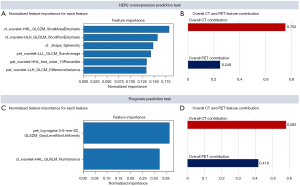
Discussion
In recent years, the necessity and urgency to establish a non-invasive and accurate method to provide comprehensive information about heterogeneous tumors have been emphasized. In this study, we have shown in a large cohort of GC patients that 18F-FDG PET/CT radiomics enabled distinction of different HER2 expressions (positive vs. negative), and different prognosis prediction (progression vs. non-progression) with an accuracy of 83.3% and 77.8%, respectively. The present radiomics signature promises to provide an efficient, objective, and non-invasive method for obtaining phenotypic information and potentially assisting clinical decision-making.
We firstly investigated the relationship between 18F-FDG uptake and HER2 expression, and found a significance difference in the SUVmax between HER2+ and HER2− patients. Similarly, previous studies have demonstrated that HER2+ GC has a higher SUVmax than HER2− GC (18,35), and 18F-FDG accumulation might help predict the HER2 expression of GC. However, the thresholds varied considerably across studies. Unfortunately, there was no significant difference in MTV and TLG between HER2+ and HER2− expression (P>0.05) in our study. The possible reason may be that the pathological type of some patients in our study was SRCC, which often shows low-to-no 18F-FDG uptake. Therefore, large-scale clinical trials are required to further investigate the value of 18F-FDG uptake in predicting HER2 expression in GC.
Radiomics can extract much more information from medical images and provide more complete characterization of the tumors beyond clinical parameters (i.e., SUVmax, MTV, and TLG). Some studies have established a radiomics nomogram to predict HER2 status in GC using CT data (36,37). To achieve this, they established and validated a radiomics nomogram for HER2 status prediction through a multivariable logistic regression method incorporating the radiomics signatures and carcinoembryonic antigen (CEA) levels. The radiomics nomogram achieved an AUC of 0.771 [95% confidence interval (CI): 0.607–0.934] in their validation cohort. Since the multivariable regression involved multiple data variables for analysis and the authors tried to find a formula that could explain how factors within variables respond simultaneously to change in others, the model did not have much scope for smaller datasets (38). In our study, we introduced an ensemble model (balanced bagging classifier), which was not limited by data size and effectively prevented the risk of overfitting. This ensemble model achieved outstanding performance with an accuracy of 83.3% (sensitivity, 84.6%; specificity, 80%; AUC, 72.2%) for HER2 expression prediction. We also found that the selected CT features had higher feature importance than the selected PET features, for the following possible reasons: (I) PET images can only provide information from the highlighted area where gray-level information is rare; (II) compared with PET images, CT images have better resolution, and structure information can be extracted from plenty of gray levels.
Early prediction of the poor outcome of patients could assist in advanced selection of additional treatments. Many studies have elucidated that some parameters of pretreatment 18F-FDG uptake (i.e., SUVmax, MTV, or TLG) are independent prognostic predictors for GC patients (39). Unfortunately, there was no statistical difference of SUVmax, MTV, or TLG between patients with different prognoses, and the reason for this finding might be associated with different pathological types and TNM stages, as some histopathological types do not show high 18F-FDG uptake. Furthermore, 18F-FDG uptake is only a reflection of glucose metabolism and it is difficult to assess the complex pathophysiological behavior of tumors from a single factor. By comparison, radiomics analysis can offer more comprehensive information about the tumor, which may demonstrate intratumoral heterogeneity and its invasiveness.
Jiang et al. reported that a radiomics signature based on 19 CT features might be helpful in identifying GC patients at higher risk of recurrence (40). It remains an intractable challenge to interpret the associations between biological processes and radiomic features. In our study, we selected 3 features to establish the predictive model, and correlation analysis indicated that these features were statistically correlated to infiltration depth (P=0.017), SUV (P=2e-3), and TLG (P<1e-5), which are strongly associated with tumor invasion. Our study also revealed that most of the selected features were from the wavelet decomposed images with different combinations of low-pass and high-pass filters. For now, it remains difficult to elucidate the effectiveness of radiomic features on chemotherapy outcome and the prognosis of patients. A reasonable explanation could be that radiomic features reflected intratumor heterogeneity and genomic pathways, as many studies have validated that radiomic features might offer helpful information on the tumor’s molecular profile and subsequent treatment strategies (41,42).
Conclusions
We demonstrated the superior diagnostic performance of 18F-FDG PET/CT radiomics in differentiating HER2+ expression from HER2− expression and prognosis prediction in patients with GC. The high diagnostic performance justified the feasibility of applying the models to provide individualized predictive information non-invasively to supplement conventional methods of clinical decision-making in patients with GC.
Acknowledgments
Funding: This research was partially funded by the National Natural Science Foundation of China (Nos. 82001866, 81971648) and Shanghai Sailing Program (No. 20YF1408400).
Footnote
Reporting Checklist: The authors have completed the TRIPOD reporting checklist. Available at https://qims.amegroups.com/article/view/10.21037/qims-22-148/rc
Conflicts of Interest: All authors have completed the ICMJE uniform disclosure form (available at https://qims.amegroups.com/article/view/10.21037/qims-22-148/coif). The authors have no conflicts of interest to declare.
Ethical Statement:
Open Access Statement: This is an Open Access article distributed in accordance with the Creative Commons Attribution-NonCommercial-NoDerivs 4.0 International License (CC BY-NC-ND 4.0), which permits the non-commercial replication and distribution of the article with the strict proviso that no changes or edits are made and the original work is properly cited (including links to both the formal publication through the relevant DOI and the license). See: https://creativecommons.org/licenses/by-nc-nd/4.0/.
References
- Bray F, Ferlay J, Soerjomataram I, Siegel RL, Torre LA, Jemal A. Global cancer statistics 2018: GLOBOCAN estimates of incidence and mortality worldwide for 36 cancers in 185 countries. CA Cancer J Clin 2018;68:394-424. [Crossref] [PubMed]
- Global, regional, and national incidence, prevalence, and years lived with disability for 354 diseases and injuries for 195 countries and territories, 1990-2017: a systematic analysis for the Global Burden of Disease Study 2017. Lancet 2018;392:1789-858. [Crossref] [PubMed]
- Hirsch FR, Scagliotti GV, Mulshine JL, Kwon R, Curran WJ Jr, Wu YL, Paz-Ares L. Lung cancer: current therapies and new targeted treatments. Lancet 2017;389:299-311. [Crossref] [PubMed]
- Meric-Bernstam F, Johnson AM, Dumbrava EEI, Raghav K, Balaji K, Bhatt M, Murthy RK, Rodon J, Piha-Paul SA. Advances in HER2-Targeted Therapy: Novel Agents and Opportunities Beyond Breast and Gastric Cancer. Clin Cancer Res 2019;25:2033-41. [Crossref] [PubMed]
- Bang YJ, Van Cutsem E, Feyereislova A, Chung HC, Shen L, Sawaki A, Lordick F, Ohtsu A, Omuro Y, Satoh T, Aprile G, Kulikov E, Hill J, Lehle M, Rüschoff J, Kang YK. Trastuzumab in combination with chemotherapy versus chemotherapy alone for treatment of HER2-positive advanced gastric or gastro-oesophageal junction cancer (ToGA): a phase 3, open-label, randomised controlled trial. Lancet 2010;376:687-97. [Crossref] [PubMed]
- Oh DY, Bang YJ. HER2-targeted therapies - a role beyond breast cancer. Nat Rev Clin Oncol 2020;17:33-48. [Crossref] [PubMed]
- Roviello G, Aprile G, D'Angelo A, Iannone LF, Roviello F, Polom K, Mini E, Catalano M. Human epidermal growth factor receptor 2 (HER2) in advanced gastric cancer: where do we stand? Gastric Cancer 2021;24:765-79. [Crossref] [PubMed]
- Jiang Y, Li T, Liang X, Hu Y, Huang L, Liao Z, Zhao L, Han Z, Zhu S, Wang M, Xu Y, Qi X, Liu H, Yang Y, Yu J, Liu W, Cai S, Li G. Association of Adjuvant Chemotherapy With Survival in Patients With Stage II or III Gastric Cancer. JAMA Surg 2017;152:e171087. [Crossref] [PubMed]
- Cheong JH, Yang HK, Kim H, Kim WH, Kim YW, Kook MC, et al. Predictive test for chemotherapy response in resectable gastric cancer: a multi-cohort, retrospective analysis. Lancet Oncol 2018;19:629-38. [Crossref] [PubMed]
- Noh SH, Park SR, Yang HK, Chung HC, Chung IJ, Kim SW, Kim HH, Choi JH, Kim HK, Yu W, Lee JI, Shin DB, Ji J, Chen JS, Lim Y, Ha S, Bang YJ. Adjuvant capecitabine plus oxaliplatin for gastric cancer after D2 gastrectomy (CLASSIC): 5-year follow-up of an open-label, randomised phase 3 trial. Lancet Oncol 2014;15:1389-96. [Crossref] [PubMed]
- Paoletti X, Oba K, Burzykowski T, Michiels S, Ohashi Y, Pignon JP, Rougier P, Sakamoto J, Sargent D, Sasako M, Van Cutsem E, Buyse M. Benefit of adjuvant chemotherapy for resectable gastric cancer: a meta-analysis. JAMA 2010;303:1729-37. [Crossref] [PubMed]
- Coccolini F, Nardi M, Montori G, Ceresoli M, Celotti A, Cascinu S, Fugazzola P, Tomasoni M, Glehen O, Catena F, Yonemura Y, Ansaloni L. Neoadjuvant chemotherapy in advanced gastric and esophago-gastric cancer. Meta-analysis of randomized trials. Int J Surg 2018;51:120-7. [Crossref] [PubMed]
- Jiang Y, Zhang Q, Hu Y, Li T, Yu J, Zhao L, Ye G, Deng H, Mou T, Cai S, Zhou Z, Liu H, Chen G, Li G, Qi X. ImmunoScore Signature: A Prognostic and Predictive Tool in Gastric Cancer. Ann Surg 2018;267:504-13. [Crossref] [PubMed]
- Zhu X, Tian X, Sun T, Yu C, Cao Y, Yan T, Shen C, Lin Y, Fang JY, Hong J, Chen H. GeneExpressScore Signature: a robust prognostic and predictive classifier in gastric cancer. Mol Oncol 2018;12:1871-83. [Crossref] [PubMed]
- Findlay JM, Antonowicz S, Segaran A, El Kafsi J, Zhang A, Bradley KM, Gillies RS, Maynard ND, Middleton MR. Routinely staging gastric cancer with (18)F-FDG PET-CT detects additional metastases and predicts early recurrence and death after surgery. Eur Radiol 2019;29:2490-8. [Crossref] [PubMed]
- Perlaza P, Ortín J, Pagès M, Buxó E, Fernández-Esparrach G, Colletti PM, Rubello D, Mayoral M, Sánchez N, Ruiz C, Ginés A, Fuster D. Should 18F-FDG PET/CT Be Routinely Performed in the Clinical Staging of Locally Advanced Gastric Adenocarcinoma? Clin Nucl Med 2018;43:402-10. [Crossref] [PubMed]
- Kim SJ, Cho YS, Moon SH, Bae JM, Kim S, Choe YS, Kim BT, Lee KH. Primary Tumor 18F-FDG Avidity Affects the Performance of 18F-FDG PET/CT for Detecting Gastric Cancer Recurrence. J Nucl Med 2016;57:544-50. [Crossref] [PubMed]
- Park JS, Lee N, Beom SH, Kim HS, Lee CK, Rha SY, Chung HC, Yun M, Cho A, Jung M. The prognostic value of volume-based parameters using (18)F-FDG PET/CT in gastric cancer according to HER2 status. Gastric Cancer 2018;21:213-24. [Crossref] [PubMed]
- Sun X, Xiao Z, Chen G, Han Z, Liu Y, Zhang C, Sun Y, Song Y, Wang K, Fang F, Wang X, Lin Y, Xu L, Shao L, Li J, Cheng Z, Gambhir SS, Shen B. A PET imaging approach for determining EGFR mutation status for improved lung cancer patient management. Sci Transl Med 2018;10:eaan8840. [Crossref] [PubMed]
- Lambin P, Rios-Velazquez E, Leijenaar R, Carvalho S, van Stiphout RG, Granton P, Zegers CM, Gillies R, Boellard R, Dekker A, Aerts HJ. Radiomics: extracting more information from medical images using advanced feature analysis. Eur J Cancer 2012;48:441-6. [Crossref] [PubMed]
- Attanasio S, Forte SM, Restante G, Gabelloni M, Guglielmi G, Neri E. Artificial intelligence, radiomics and other horizons in body composition assessment. Quant Imaging Med Surg 2020;10:1650-60. [Crossref] [PubMed]
- Verma V, Simone CB 2nd, Krishnan S, Lin SH, Yang J, Hahn SM. The Rise of Radiomics and Implications for Oncologic Management. J Natl Cancer Inst 2017; [Crossref] [PubMed]
- Bi WL, Hosny A, Schabath MB, Giger ML, Birkbak NJ, Mehrtash A, Allison T, Arnaout O, Abbosh C, Dunn IF, Mak RH, Tamimi RM, Tempany CM, Swanton C, Hoffmann U, Schwartz LH, Gillies RJ, Huang RY, Aerts HJWL. Artificial intelligence in cancer imaging: Clinical challenges and applications. CA Cancer J Clin 2019;69:127-57. [Crossref] [PubMed]
- Lambin P, Leijenaar RTH, Deist TM, Peerlings J, de Jong EEC, van Timmeren J, Sanduleanu S, Larue RTHM, Even AJG, Jochems A, van Wijk Y, Woodruff H, van Soest J, Lustberg T, Roelofs E, van Elmpt W, Dekker A, Mottaghy FM, Wildberger JE, Walsh S. Radiomics: the bridge between medical imaging and personalized medicine. Nat Rev Clin Oncol 2017;14:749-62. [Crossref] [PubMed]
- Liu S, He J, Liu S, Ji C, Guan W, Chen L, Guan Y, Yang X, Zhou Z. Radiomics analysis using contrast-enhanced CT for preoperative prediction of occult peritoneal metastasis in advanced gastric cancer. Eur Radiol 2020;30:239-46. [Crossref] [PubMed]
- Huang YQ, Liang CH, He L, Tian J, Liang CS, Chen X, Ma ZL, Liu ZY. Development and Validation of a Radiomics Nomogram for Preoperative Prediction of Lymph Node Metastasis in Colorectal Cancer. J Clin Oncol 2016;34:2157-64. [Crossref] [PubMed]
- Zhu Y, Zhou Y, Zhang W, Xue L, Li Y, Jiang J, Zhong Y, Wang S, Jiang L. Value of quantitative dynamic contrast-enhanced and diffusion-weighted magnetic resonance imaging in predicting extramural venous invasion in locally advanced gastric cancer and prognostic significance. Quant Imaging Med Surg 2021;11:328-40. [Crossref] [PubMed]
- Amin MB, Edge SB, Greene FL, Byrd DR, Brookland RK, Washington MK, et al. AJCC Cancer Staging Manual (8th edition). Springer International Publishing: American Joint Commission on Cancer; 2017.
- Banerji U, van Herpen CML, Saura C, Thistlethwaite F, Lord S, Moreno V, Macpherson IR, Boni V, Rolfo C, de Vries EGE, Rottey S, Geenen J, Eskens F, Gil-Martin M, Mommers EC, Koper NP, Aftimos P. Trastuzumab duocarmazine in locally advanced and metastatic solid tumours and HER2-expressing breast cancer: a phase 1 dose-escalation and dose-expansion study. Lancet Oncol 2019;20:1124-35. [Crossref] [PubMed]
- Yushkevich PA, Piven J, Hazlett HC, Smith RG, Ho S, Gee JC, Gerig G. User-guided 3D active contour segmentation of anatomical structures: significantly improved efficiency and reliability. Neuroimage 2006;31:1116-28. [Crossref] [PubMed]
- van Griethuysen JJM, Fedorov A, Parmar C, Hosny A, Aucoin N, Narayan V, Beets-Tan RGH, Fillion-Robin JC, Pieper S, Aerts HJWL. Computational Radiomics System to Decode the Radiographic Phenotype. Cancer Res 2017;77:e104-7. [Crossref] [PubMed]
- Zwanenburg A, Leger S, Vallières M, Löck S. Image biomarker standardisation initiative. arXiv 2016. arXiv preprint arXiv:1612.07003.
- Ribeiro MT, Singh S, Guestrin C. "Why should i trust you?" Explaining the predictions of any classifier. Proceedings of the 22nd ACM SIGKDD international conference on knowledge discovery and data mining; 2016:1135-44.
- Moulin P. Multiscale image decompositions and wavelets. The essential guide to image processing. Academic Press; 2009:123-42.
- Chen R, Zhou X, Liu J, Huang G. Relationship Between 18F-FDG PET/CT Findings and HER2 Expression in Gastric Cancer. J Nucl Med 2016;57:1040-4. [Crossref] [PubMed]
- Wang N, Wang X, Li W, Ye H, Bai H, Wu J, Chen M, Contrast-Enhanced CT. Parameters of Gastric Adenocarcinoma: Can Radiomic Features Be Surrogate Biomarkers for HER2 Over-Expression Status? Cancer Manag Res 2020;12:1211-9. [Crossref] [PubMed]
- Li Y, Cheng Z, Gevaert O, He L, Huang Y, Chen X, Huang X, Wu X, Zhang W, Dong M, Huang J, Huang Y, Xia T, Liang C, Liu Z. A CT-based radiomics nomogram for prediction of human epidermal growth factor receptor 2 status in patients with gastric cancer. Chin J Cancer Res 2020;32:62-71. [Crossref] [PubMed]
- Alexopoulos EC. Introduction to multivariate regression analysis. Hippokratia 2010;14:23-8. [PubMed]
- Marcus C, Subramaniam RM. PET/Computed Tomography and Precision Medicine: Gastric Cancer. PET Clin 2017;12:437-47. [Crossref] [PubMed]
- Jiang Y, Wang W, Chen C, Zhang X, Zha X, Lv W, Xie J, Huang W, Sun Z, Hu Y, Yu J, Li T, Zhou Z, Xu Y, Li G. Radiomics Signature on Computed Tomography Imaging: Association With Lymph Node Metastasis in Patients With Gastric Cancer. Front Oncol 2019;9:340. [Crossref] [PubMed]
- Jiang Y, Wang H, Wu J, Chen C, Yuan Q, Huang W, Li T, Xi S, Hu Y, Zhou Z, Xu Y, Li G, Li R. Noninvasive imaging evaluation of tumor immune microenvironment to predict outcomes in gastric cancer. Ann Oncol 2020;31:760-8. [Crossref] [PubMed]
- Rossi G, Barabino E, Fedeli A, Ficarra G, Coco S, Russo A, Adamo V, Buemi F, Zullo L, Dono M, De Luca G, Longo L, Dal Bello MG, Tagliamento M, Alama A, Cittadini G, Pronzato P, Genova C. Radiomic Detection of EGFR Mutations in NSCLC. Cancer Res 2021;81:724-31. [Crossref] [PubMed]