A magnetic resonance imaging-based computational analysis of cerebral hemodynamics in patients with carotid artery stenosis
Introduction
Carotid artery stenosis (CAS) is a leading cause of stroke, accounting for 10–15% of ischemic strokes (1,2). Management of patients with CAS relies on assessment of the percentage of stenosis, which does not evaluate factors important in stroke pathophysiology such as plaque composition, the patency of cerebral collaterals, or the cerebral vascular flow reserve (3-5). Given the wide variability regarding the indications for carotid endarterectomy (CEA) in asymptomatic patients, lack of contemporary trials, and limitations of stenosis measurements, improved mechanisms of assessing stroke risk and CEA benefit are warranted.
Cerebral hemodynamics play a key role in the stroke risk of CAS (6). Strokes in patients with high grade stenosis, in combination with inadequate collateral pathways in the circle of Willis (CoW), are likely to have a hemodynamic etiology due to a critical flow reduction ipsilateral to the stenosis (7). Furthermore, cerebral hemodynamics may play a role in the prevention of symptomatic CAS, given that patent collaterals have been associated with a reduced risk of stroke and transient ischemic attack (TIA) (8-10). However, obtaining a quantitative description of cerebral hemodynamics with clinically available mechanisms is challenging. The primary imaging tools to measure cerebral blood flow clinically include transcranial doppler, which provides a velocity spectrum in selected locations, and 4D-flow magnetic resonance imaging (MRI), which yields a velocity field with limited spatial and temporal resolution. Measurements of intravascular pressure in the cervical and cerebral arteries are generally not available.
In contrast, patient-specific computational fluid dynamics (CFD) allows for characterization of complex hemodynamics and the ability to provide velocity and pressure data with a high spatial and temporal resolution. The capabilities of patient-specific CFD analysis to assess cerebral hemodynamics have been demonstrated for intracranial stenosis (11-13) and aneurysm (14-16). However, patient-specific calibration of cerebral blood flow models remains challenging due to the high anatomical variability of cerebral vasculature and the effects of cerebral autoregulation on cerebral blood flow. Previous CFD studies of cerebral hemodynamics have generally relied on modeling assumption about the flow distribution in the CoW (17,18), thereby significantly limiting the ability to capture the hemodynamic impact of stenosis, in particular the flow compensation in the CoW.
The aim of this study was to quantify cerebral hemodynamics using MRI-informed CFD and to provide novel hemodynamic information that may improve the understanding of stroke risk and identify patients who would benefit from CEA. We previously presented a novel method of calibrating CFD models patient-specifically using a combination of arterial spin labeling (ASL) MRI brain perfusion, phase-contrast (PC)-MRI flow, and cuff pressure data, which was validated in a small cohort of subjects (19). In this work, we demonstrated the feasibility of an MRI-informed CFD analysis to (I) assess differences in cerebral hemodynamics and collateral flow between two patients with high grade CAS; (II) evaluate hemodynamic changes following CEA; and (III) derive pressure metrics to identify hemodynamically significant CAS.
Methods
Two patients with CAS were recruited from the vascular surgery unit at the University of Michigan Hospital. The study was conducted in accordance with the Declaration of Helsinki (as revised in 2013). The study was approved by the Institutional Review Board of the University of Michigan (No. HUM00114275) and informed consent was taken from all individual participants.
Imaging data
Patients underwent an MRI study performed at 3T (MR750; GE Healthcare, Waukesha, WI). Patients 1 and 2 received MRI scans at 46 and 12 days preoperatively, respectively. Patient 2 received an additional MRI scan 56 days postoperatively. Anatomical information from the thoracic aorta to the CoW was acquired with a combination of 2D T1-weighted spoiled gradient echo MRI and 3D Time-of-Flight MRI. Additionally, preoperative neck and head computed tomography angiography (CTA) data was available for patient 2. Flow rates were obtained at the level of the ascending aorta and the main cervical arteries above the carotid bifurcation from PC-MRI. Brain tissue perfusion data were collected non-invasively using ASL. First, a standard non-selective labeling scheme was employed to acquire total perfusion maps of the brain tissue (20). Following, a vessel-selective labeling scheme was used to acquire the regional brain perfusion territories of the carotid and vertebral arteries (21,22). Cuff pressure was acquired in the right upper extremity while the patient remained in the supine position. Further details of the imaging protocol have been previously described (19).
MRI-informed computational modeling
MRI-informed CFD analysis of cervical and cerebral hemodynamics was performed using the validated open-source computational hemodynamics framework CRIMSON (23). Anatomical models of the large arteries from the aortic arch to the CoW were constructed by first segmenting 2D vessel contours along the centerline and second lofting an analytical representation of each vessel to create a combined 3D anatomical model (Figure S1). The preoperative model of patient 1 was reconstructed based on the anatomical MRI data. For the preoperative model of patient 2, the unsubtracted CTA data was used due to the higher spatial resolution. The postoperative anatomical model of patient 2 was created by updating the preoperative anatomical model in the region of the right carotid bifurcation based on the postoperative anatomical MRI data. Briefly, the postoperative MRI data was first co-registered to the preoperative CTA data in the region of the stenosis. The vessel segments of the common and internal carotid artery around the stenosis were then re-segmented based on the postoperative MRI and lofted to create a postoperative 3D model. 3D models were discretized using linear tetrahedral elements. All vessel walls were assumed rigid with a no-slip boundary condition. Inflow and outflow boundary conditions were prescribed at each model inlet and outlet (Figure S2). A volumetric flow waveform, reconstructed from PC-MRI, was imposed at the inflow of the ascending aorta. Each model outflow was coupled to a 3-element Windkessel lumped-parameter model, consisting of a proximal resistance Rp, a distal resistance Rd, and a compliance C, to capture the behavior of the distal vasculature. The Windkessel model parameters were iteratively calibrated to match the flow and pressure waveforms of the CFD model to the patient’s acquired hemodynamic data. Briefly, (I) Using the PC-MRI and ASL perfusion data, mean target flow rates were derived at each model outlet and the distal resistances Rd were tuned to match the CFD flow rates to the target values. (II) The resistance ratio Rp/Rd was manually adjusted to match the pulsatility of the CFD flow waveforms to the pulsatility of the PC-MRI waveforms above the carotid bifurcation. And (III), the total resistance and compliance of all windkessel models combined was adjusted to match the CFD model diastolic and systolic pressure at the right subclavian artery to the cuff pressure measurements. The final Windkessel parameters are provided in Table S1. A more detailed description of our patient-specific parameter tuning strategy can be found elsewhere (19).
Blood was modeled as an incompressible Newtonian fluid with a dynamic viscosity of 0.004 kg·m−1·s−1 and a density of 1,060 kg·m−3. Computations were performed with the CRIMSON Navier-Stokes Flow solver using 80 cores on a high-performance computing cluster. Simulations were run using a time step size of 0.1 ms until cycle-to-cycle periodicity was achieved, typically after 5 cardiac cycles. Velocity and pressure fields were extracted for the last cardiac cycle.
To visualize and quantify the blood supply from the cervical arteries to the CoW, a further post-processing analysis on the CFD data, known as Lagrangian particle tracking (LPT) was performed (24,25). Virtual boluses were created by seeding massless particles continuously at the root of each cervical artery and advecting the particles with the velocity field over multiple cardiac cycles. Particles were counted at each outlet over the last cardiac cycle.
Results
Patient history
Patient 1 [female, 55 years old with history of hypertension (HTN), hyperlipidemia (HLD), peripheral artery disease, chronic obstructive pulmonary disease, and previous left CEA] presented with an asymptomatic 70–99% stenosis of the proximal right internal carotid artery (RICA) based on duplex ultrasound (DUS) North American Symptomatic Carotid Endarterectomy Trial (NASCET) criteria [peak systolic velocity (PSV)/end-diastolic velocity (EDV) = 417/140 cm/s]. She had a compete CoW and the left internal carotid artery (LICA) was patent with no evidence of stenosis (PSV/EDV = 112/42 cm/s). She underwent CEA with patch angioplasty on the RICA. Her post-operative course was uncomplicated. Her post-operative duplex demonstrated a PSV/EDV of 123/50 cm/s. At one month follow-up the patient was on optimal medical therapy (OMT).
Patient 2 (male, 63 years old with history of HTN and HLD) presented with an asymptomatic 50–69% stenosis of the proximal RICA by velocities (PSV/EDV = 160/33 cm/s) but a 70–99% stenosis by image criteria on DUS and a hemodynamically insignificant stenosis of the proximal LICA based on DUS (PSV/EDV =97/41 cm/s). CTA data revealed an 80–90% and 60% stenosis in the proximal RICA and proximal LICA respectively with a complex high-grade plaque. The patient’s CoW was incomplete with a hypoplastic right P1 segment and an atretic distal right vertebral artery (RVA). Given the disease progression with an 80–90% RICA stenosis identified on CTA, CEA with patch angioplasty was performed on the RICA following the study and patency was confirmed by DUS (RICA: PSV/EDV =73/19 cm/s, LICA: PSV/EDV =84/28 cm/s) postoperatively. The patient tolerated the procedure well, which was complicated by a neck hematoma that was managed conservatively. At one month follow up the patient remained asymptomatic and was on OMT.
Anatomical models
The cross-section at maximum RICA stenosis revealed a remaining luminal diameter of 1.4 and 3.5 mm for patients 1 and 2, respectively. The lumen of the proximal RICA in patient 2 was fully restored following CEA (8.3 mm) (Figure 1).
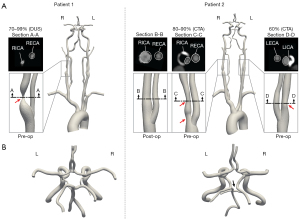
Preoperative hemodynamics
In patient 1, the severe RICA stenosis resulted in a reduction in mean flow () and pressure () when compared to the patent contralateral LICA (=3.25 mL/s versus =6.79 mL/s and =62.92 mmHg versus =87.72 mmHg, respectively) (Figure 2). In the CoW, the mean flow in the ipsilateral right middle cerebral artery (RMCA) was restored to the level of the contralateral left middle cerebral artery (LMCA) (=3.74 mL/s and =3.92 mL/s). However, the pressure difference between hemispheres remained (=47.62 mmHg and =74.91 mmHg). In contrast, the severe RICA stenosis in patient 2 resulted only in a minor flow reduction compared to the LICA with =4.00 mL/s and =5.25 mL/s and the mean pressure was comparable (=109.16 mmHg and =110.53 mmHg). In the CoW, the mean flows and pressures observed at the right and left MCA were comparable with =106.42 mmHg versus =106.37 mmHg and =2.50 mL/s versus =2.60 mL/s.
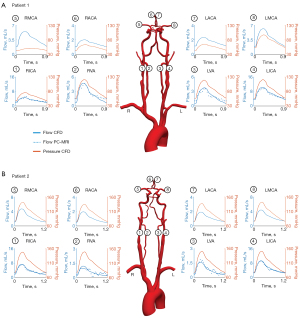
Patient 1 exhibited a large amount of flow compensation between hemispheres with the right anterior cerebral artery (RACA) (#2) being predominately supplied by the LICA (80.31%) (Figure 3). In contrast, patient 2 revealed only a small amount of collateral flow from the LICA to the RACA (20.05%). The MCAs (#3, #4) were predominately supplied by the ipsilateral ICA in both patients. Patient 1 revealed a rotational motion of vertebral flow in the basilar artery, resulting in dominant supply of the left posterior cerebral artery (LPCA) (#5) from the RVA and dominant supply of the right posterior cerebral artery (RPCA) (#6) from the left vertebral artery (LVA). The posterior territory of patient 2 was supplied ipsilaterally by the ICAs and the cerebellum was supplied by the LVA.
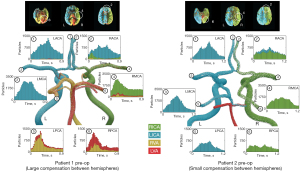
Postoperative hemodynamics
The preoperative collateral flow from the LICA to the RACA observed in patient 2 disappeared postoperatively (Figure 4). The flow direction in the anterior communicating artery was reversed, resulting in a minor flow contribution from the RICA to the left anterior cerebral artery (LACA) (7.24%). Following CEA in patient 2, changes in flow rates were small in both cervical and cerebral arteries with flow increasing by 8.1% in the RICA and by 13.4% in the LMCA postoperatively (Figure 5).
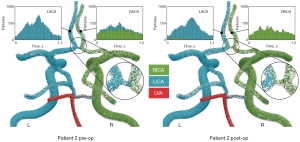
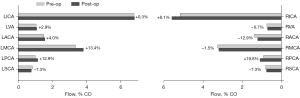
Pressure metrics
The hemodynamic impact of CAS was further assessed by calculating a mean pressure gradient over the stenosis as the difference in pressures 2 cm proximal and distal to the maximum narrowing (Figure 6). The preoperative analysis resulted in a significantly higher mean pressure gradient over the RICA stenosis of patient 1 with =26.3 mmHg compared to the RICA stenosis of patient 2 with =1.8 mmHg. Following CEA in patient 2, the mean pressure gradient over the RICA stenosis was reduced to =0.1 mmHg.
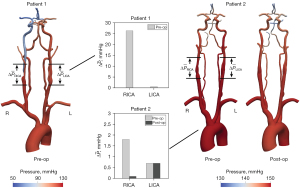
Discussion
Diagnosis and management of patients with CAS is centered around assessing the degree of stenosis. While reduced long-term stroke risk following carotid revascularization has been demonstrated in symptomatic patients with greater than 50% stenosis, the criteria and benefits of revascularization in asymptomatic patients are unclear (26-28). While contemporary guidelines recommend consideration of carotid artery revascularization for asymptomatic patients with greater than 70% stenosis (29), most clinicians reserve revascularization for cases greater than 80–90%. Thus, the question of how to best identify patients with asymptomatic CAS who would benefit from carotid revascularization remains. In the present study, we utilized a novel patient-specific MRI-informed CFD modeling strategy to provide quantitative insight into cerebral hemodynamics.
The preoperative comparison of two patients with high grade asymptomatic CAS showed significant differences in hemodynamics despite both patients having similar percentage of luminal narrowing. Patient 1 exhibited a large flow and pressure gradient in the RICA with a large amount of collateral flow compensation, while patient 2 demonstrated only minor reductions in the RICA. Differences in lumen diameter (1.4 mm for patient 1 and 3.5 mm for patient 2) may explain the differences in hemodynamics between patients in this study. These differences illustrate the variability in cerebral hemodynamics due to stenotic lesions, anatomical variations, patency of collaterals, and vascular flow reserve. While the peak velocities obtained from DUS revealed differences between the two patients, a comprehensive representation of the differences in flow, pressure, and blood supply is not available from DUS or CTA/magnetic resonance angiography (MRA). Interestingly, following CEA patient 2 had minor hemodynamic changes, with a small reduction in pressure gradient and increase in flow in the RICA thus highlighting that revascularization may not result in hemodynamic improvements. Although it is unclear how the hemodynamic differences noted in these two patients affected their stroke risk, these results highlight that patient-specific analyses provide a more comprehensive assessment of carotid artery lesions and their potential hemodynamic effects.
Due to its high sensitivity to the degree of luminal narrowing, the pressure gradient may be a better suited marker than anatomical or velocity-based metrics to identify hemodynamically significant stenotic lesions. In coronary artery disease, intervention based on pressure gradient-derived metrics (e.g., fractional flow reserve or FFR) has been shown superior to anatomic-derived metrics (30,31). While the use fractional flow has been proposed for CAS (6), studies so far have only established feasibility (12,32). Since pressure guidewire measurements in the cervical or intracranial arteries are generally unavailable, CFD analysis provides a non-invasive alternative. Liu et al. found a fractional flow of 0.88 to be a potential threshold to distinguish between severe and moderate degrees of stenosis based on CFD models of the carotid bifurcation informed by contrast-enhanced MRI and DUS data (33). Furthermore, CFD-derived pressure gradients in CAS have shown to correlate well with guidewire pressure measurements (11,34).
Distal to the stenosis, hemodynamics is further impacted by the flow compensatory capabilities of the cerebral vasculature. Digital subtraction angiography (DSA) is the gold standard for visualizing collateral flow and has been used to derive hemodynamic markers (e.g., bolus transit time) (35). ASL perfusion has been proposed as a non-invasive DSA alternative to assess the hemodynamic impact of CAS at the tissue level. ASL-derived markers (e.g., arterial transit time, cerebral blood volume, etc.) have shown to be good indicators of the hemodynamic severity of carotid stenosis (36,37). Vessel-selective ASL has further allowed to quantify collateral flow compensation (38).
The aim of our proposed modeling strategy is to quantitatively assess the hemodynamic impact of stenosis at the level of the stenosis, the CoW, and the tissue, thereby providing a more complete hemodynamic picture. However, one of the challenges in the cerebral vasculature is that the flow in each of the main arteries of the CoW is considerably controlled by the mechanisms of cerebral autoregulation in the distal vascular bed. Previous CFD modeling studies of cerebral blood flow in CAS patients have generally not accounted for these changes in the distal vasculature and instead have relied on idealized assumptions about the flow distribution in the CoW (17,18). Our proposed patient-specific model parameter tuning strategy allows to account for changes in the microvasculature and enables the quantification of collateral flow in the main pathways of the CoW additionally to capturing the pressure gradient over the stenosis.
This study was limited by the small sample size and is therefore intended as a feasibility study to demonstrate the capabilities of an MRI-informed CFD analysis to patient-specifically characterize cerebral hemodynamics rather than a replacement of gold standard diagnostic modalities. Furthermore, it remains unclear if hemodynamic differences in carotid arterial and intracranial flow are predictive of stroke risk and/or surgical outcomes. Given the limited number of patients in this study, we cannot account for the impact of factors associated with carotid artery disease (sex, tobacco use, HTN, HLD, etc.) which may be affecting the differences we are observing. Moreover, our study did not include an assessment of high risk/vulnerable plaques, which is associated with stroke risk and cerebrovascular events in symptomatic and asymptomatic patients (39,40). Furthermore, secondary collateral pathways (e.g., ophthalmic and leptomeningeal arteries), which have been shown to play an important role in stroke volume and outcomes (41,42), were not included in the CFD modeling.
In conclusion, we presented an MRI-informed CFD modeling strategy to characterize cerebral hemodynamics in patients with CAS. We demonstrated that our MRI-informed CFD analysis can provide detailed and quantitative information about hemodynamic impact of CAS and collateral flow compensation in the CoW. Future studies are needed to investigate the clinical impact of cerebrovascular hemodynamic differences and how they pertain to stroke risk and clinical management.
Acknowledgments
Funding: This work was supported by the Predoctoral Fellowship (Rackham Graduate School, University of Michigan) and the Edward B. Diethrich Professorship.
Footnote
Conflicts of Interest: All authors have completed the ICMJE uniform disclosure form (available at https://qims.amegroups.com/article/view/10.21037/qims-22-565/coif). The authors have no conflicts of interest to declare.
Ethical Statement: The authors are accountable for all aspects of the work in ensuring that questions related to the accuracy or integrity of any part of the work are appropriately investigated and resolved. The study was conducted in accordance with the Declaration of Helsinki (as revised in 2013). The study was approved by the Institutional Review Board of the University of Michigan (No. HUM00114275) and informed consent was taken from all individual participants.
Open Access Statement: This is an Open Access article distributed in accordance with the Creative Commons Attribution-NonCommercial-NoDerivs 4.0 International License (CC BY-NC-ND 4.0), which permits the non-commercial replication and distribution of the article with the strict proviso that no changes or edits are made and the original work is properly cited (including links to both the formal publication through the relevant DOI and the license). See: https://creativecommons.org/licenses/by-nc-nd/4.0/.
References
- Flaherty ML, Kissela B, Khoury JC, Alwell K, Moomaw CJ, Woo D, Khatri P, Ferioli S, Adeoye O, Broderick JP, Kleindorfer D. Carotid artery stenosis as a cause of stroke. Neuroepidemiology 2013;40:36-41. [Crossref] [PubMed]
-
.Lalla R Raghavan P Chaturvedi S Trends and controversies in carotid artery stenosis treatment. F1000Res 2020 . doi:.10.12688/f1000research.25922.1 - Liebeskind DS. Collateral circulation. Stroke 2003;34:2279-84. [Crossref] [PubMed]
- Saba L, Yuan C, Hatsukami TS, Balu N, Qiao Y, DeMarco JK, Saam T, Moody AR, Li D, Matouk CC, Johnson MH, Jäger HR, Mossa-Basha M, Kooi ME, Fan Z, Saloner D, Wintermark M, Mikulis DJ, Wasserman BA. Carotid Artery Wall Imaging: Perspective and Guidelines from the ASNR Vessel Wall Imaging Study Group and Expert Consensus Recommendations of the American Society of Neuroradiology. AJNR Am J Neuroradiol 2018;39:E9-E31. [Crossref] [PubMed]
- Gupta A, Chazen JL, Hartman M, Delgado D, Anumula N, Shao H, Mazumdar M, Segal AZ, Kamel H, Leifer D, Sanelli PC. Cerebrovascular reserve and stroke risk in patients with carotid stenosis or occlusion: a systematic review and meta-analysis. Stroke 2012;43:2884-91. [Crossref] [PubMed]
- Liebeskind DS, Feldmann E. Fractional Flow in Cerebrovascular Disorders. Interv Neurol 2013;1:87-99. [Crossref] [PubMed]
- Li Y, Li M, Zhang X, Yang S, Fan H, Qin W, Yang L, Yuan J, Hu W. Clinical features and the degree of cerebrovascular stenosis in different types and subtypes of cerebral watershed infarction. BMC Neurol 2017;17:166. [Crossref] [PubMed]
- Henderson RD, Eliasziw M, Fox AJ, Rothwell PM, Barnett HJ. Angiographically defined collateral circulation and risk of stroke in patients with severe carotid artery stenosis. North American Symptomatic Carotid Endarterectomy Trial (NASCET) Group. Stroke 2000;31:128-32. [Crossref] [PubMed]
- Hendrikse J, Hartkamp MJ, Hillen B, Mali WP, van der Grond J. Collateral ability of the circle of Willis in patients with unilateral internal carotid artery occlusion: border zone infarcts and clinical symptoms. Stroke 2001;32:2768-73. [Crossref] [PubMed]
- Bisschops RH, Klijn CJ, Kappelle LJ, van Huffelen AC, van der Grond J. Collateral flow and ischemic brain lesions in patients with unilateral carotid artery occlusion. Neurology 2003;60:1435-41. [Crossref] [PubMed]
- Liu J, Yan Z, Pu Y, Shiu WS, Wu J, Chen R, Leng X, Qin H, Liu X, Jia B, Song L, Wang Y, Miao Z, Wang Y, Liu L, Cai XC. Functional assessment of cerebral artery stenosis: A pilot study based on computational fluid dynamics. J Cereb Blood Flow Metab 2017;37:2567-76. [Crossref] [PubMed]
- Leng X, Scalzo F, Ip HL, Johnson M, Fong AK, Fan FS, Chen X, Soo YO, Miao Z, Liu L, Feldmann E, Leung TW, Liebeskind DS, Wong KS. Computational fluid dynamics modeling of symptomatic intracranial atherosclerosis may predict risk of stroke recurrence. PLoS One 2014;9:e97531. [Crossref] [PubMed]
- Leng X, Lan L, Ip HL, Abrigo J, Scalzo F, Liu H, et al. Hemodynamics and stroke risk in intracranial atherosclerotic disease. Ann Neurol 2019;85:752-64. [Crossref] [PubMed]
- Castro MA, Putman CM, Cebral JR. Computational fluid dynamics modeling of intracranial aneurysms: effects of parent artery segmentation on intra-aneurysmal hemodynamics. AJNR Am J Neuroradiol 2006;27:1703-9. [PubMed]
- Raschi M, Mut F, Byrne G, Putman CM, Tateshima S, Viñuela F, Tanoue T, Tanishita K, Cebral CFD JR. Int J Numer Method Biomed Eng 2012;28:214-28. [Crossref] [PubMed]
- Rayz VL, Boussel L, Acevedo-Bolton G, Martin AJ, Young WL, Lawton MT, Higashida R, Saloner D. Numerical simulations of flow in cerebral aneurysms: comparison of CFD results and in vivo MRI measurements. J Biomech Eng 2008;130:051011. [Crossref] [PubMed]
- Mukherjee D, Jani ND, Selvaganesan K, Weng CL, Shadden SC. Computational Assessment of the Relation Between Embolism Source and Embolus Distribution to the Circle of Willis for Improved Understanding of Stroke Etiology. J Biomech Eng 2016; [Crossref] [PubMed]
- Bockman MD, Kansagra AP, Shadden SC, Wong EC, Marsden AL. Fluid Mechanics of Mixing in the Vertebrobasilar System: Comparison of Simulation and MRI. Cardiovasc Eng Technol 2012;3:450-61. [Crossref]
- Schollenberger J, Osborne NH, Hernandez-Garcia L, Figueroa CA. A Combined Computational Fluid Dynamics and Arterial Spin Labeling MRI Modeling Strategy to Quantify Patient-Specific Cerebral Hemodynamics in Cerebrovascular Occlusive Disease. Front Bioeng Biotechnol 2021;9:722445. [Crossref] [PubMed]
- Alsop DC, Detre JA, Golay X, Günther M, Hendrikse J, Hernandez-Garcia L, Lu H, MacIntosh BJ, Parkes LM, Smits M, van Osch MJ, Wang DJ, Wong EC, Zaharchuk G. Recommended implementation of arterial spin-labeled perfusion MRI for clinical applications: A consensus of the ISMRM perfusion study group and the European consortium for ASL in dementia. Magn Reson Med 2015;73:102-16. [Crossref] [PubMed]
- Helle M, Norris DG, Rüfer S, Alfke K, Jansen O, van Osch MJ. Superselective pseudocontinuous arterial spin labeling. Magn Reson Med 2010;64:777-86. [Crossref] [PubMed]
- Schollenberger J, Figueroa CA, Nielsen JF, Hernandez-Garcia L. Practical considerations for territorial perfusion mapping in the cerebral circulation using super-selective pseudo-continuous arterial spin labeling. Magn Reson Med 2020;83:492-504. [Crossref] [PubMed]
- Arthurs CJ, Khlebnikov R, Melville A, Marčan M, Gomez A, Dillon-Murphy D, et al. CRIMSON: An open-source software framework for cardiovascular integrated modelling and simulation. PLoS Comput Biol 2021;17:e1008881. [Crossref] [PubMed]
- Di Achille P, Tellides G, Figueroa CA, Humphrey JD. A haemodynamic predictor of intraluminal thrombus formation in abdominal aortic aneurysms. Proc R Soc Lond Math Phys Eng Sci 2014;470:20140163.
- van Bakel TM, Arthurs CJ, van Herwaarden JA, Moll FL, Eagle KA, Patel HJ, Trimarchi S, Figueroa CA. A computational analysis of different endograft designs for Zone 0 aortic arch repair. Eur J Cardiothorac Surg 2018;54:389-96. [Crossref] [PubMed]
- Barnett HJM, Taylor DW, Haynes RB, Sackett DL, Peerless SJ, Ferguson GG, Fox AJ, Rankin RN, Hachinski VC, Wiebers DO, Eliasziw M. Beneficial effect of carotid endarterectomy in symptomatic patients with high-grade carotid stenosis. N Engl J Med 1991;325:445-53. [Crossref] [PubMed]
- MRC European Carotid Surgery Trial: interim results for symptomatic patients with severe (70-99%) or with mild (0-29%) carotid stenosis. European Carotid Surgery Trialists' Collaborative Group. Lancet 1991;337:1235-43. [Crossref] [PubMed]
- Mayberg MR, Wilson SE, Yatsu F, Weiss DG, Messina L, Hershey LA, Colling C, Eskridge J, Deykin D, Winn HR. Carotid endarterectomy and prevention of cerebral ischemia in symptomatic carotid stenosis. Veterans Affairs Cooperative Studies Program 309 Trialist Group. JAMA 1991;266:3289-94. [Crossref] [PubMed]
- AbuRahma AF, Avgerinos ED, Chang RW, Darling RC 3rd, Duncan AA, Forbes TL, Malas MB, Murad MH, Perler BA, Powell RJ, Rockman CB, Zhou W. Society for Vascular Surgery clinical practice guidelines for management of extracranial cerebrovascular disease. J Vasc Surg 2022;75:4S-22S. [Crossref] [PubMed]
- Tonino PA, De Bruyne B, Pijls NH, Siebert U, Ikeno F. van' t Veer M, Klauss V, Manoharan G, Engstrøm T, Oldroyd KG, Ver Lee PN, MacCarthy PA, Fearon WF; . Fractional flow reserve versus angiography for guiding percutaneous coronary intervention. N Engl J Med 2009;360:213-24. [Crossref] [PubMed]
- De Bruyne B, Fearon WF, Pijls NH, Barbato E, Tonino P, Piroth Z, et al. Fractional flow reserve-guided PCI for stable coronary artery disease. N Engl J Med 2014;371:1208-17. [Crossref] [PubMed]
- Miao Z, Liebeskind DS, Lo W, Liu L, Pu Y, Leng X, Song L, Xu X, Jia B, Gao F, Mo D, Sun X, Liu L, Ma N, Wang B, Wang Y, Wang Y. Fractional Flow Assessment for the Evaluation of Intracranial Atherosclerosis: A Feasibility Study. Interv Neurol 2016;5:65-75. [Crossref] [PubMed]
- Liu X, Zhang H, Ren L, Xiong H, Gao Z, Xu P, Huang W, Wu W. Functional assessment of the stenotic carotid artery by CFD-based pressure gradient evaluation. Am J Physiol Heart Circ Physiol 2016;311:H645-53. [Crossref] [PubMed]
- Zhang D, Xu P, Qiao H, Liu X, Luo L, Huang W, Zhang H, Shi C. Carotid DSA based CFD simulation in assessing the patient with asymptomatic carotid stenosis: a preliminary study. Biomed Eng Online 2018;17:31. [Crossref] [PubMed]
- Teng MMH, Chang FC, Lin CJ, Chiang L, Hong JS, Kao YH. Peritherapeutic Hemodynamic Changes of Carotid Stenting Evaluated with Quantitative DSA in Patients with Carotid Stenosis. AJNR Am J Neuroradiol 2016;37:1883-8. [Crossref] [PubMed]
- Schröder J, Heinze M, Günther M, Cheng B, Nickel A, Schröder T, Fischer F, Kessner SS, Magnus T, Fiehler J, Larena-Avellaneda A, Gerloff C, Thomalla G. Dynamics of brain perfusion and cognitive performance in revascularization of carotid artery stenosis. Neuroimage Clin 2019;22:101779. [Crossref] [PubMed]
- Di Napoli A, Cheng SF, Gregson J, Atkinson D, Markus JE, Richards T, Brown MM, Sokolska M, Jäger HR. Arterial Spin Labeling MRI in Carotid Stenosis: Arterial Transit Artifacts May Predict Symptoms. Radiology 2020;297:652-60. [Crossref] [PubMed]
- Richter V, Helle M, van Osch MJ, Lindner T, Gersing AS, Tsantilas P, Eckstein HH, Preibisch C, Zimmer C. MR Imaging of Individual Perfusion Reorganization Using Superselective Pseudocontinuous Arterial Spin-Labeling in Patients with Complex Extracranial Steno-Occlusive Disease. AJNR Am J Neuroradiol 2017;38:703-11. [Crossref] [PubMed]
- Altaf N, Daniels L, Morgan PS, Auer D, MacSweeney ST, Moody AR, Gladman JR. Detection of intraplaque hemorrhage by magnetic resonance imaging in symptomatic patients with mild to moderate carotid stenosis predicts recurrent neurological events. J Vasc Surg 2008;47:337-42. [Crossref] [PubMed]
- Porambo ME, DeMarco JK. MR imaging of vulnerable carotid plaque. Cardiovasc Diagn Ther 2020;10:1019-31. [Crossref] [PubMed]
- Miteff F, Levi CR, Bateman GA, Spratt N, McElduff P, Parsons MW. The independent predictive utility of computed tomography angiographic collateral status in acute ischaemic stroke. Brain 2009;132:2231-8. [Crossref] [PubMed]
- Liebeskind DS, Jahan R, Nogueira RG, Zaidat OO, Saver JL. Impact of collaterals on successful revascularization in Solitaire FR with the intention for thrombectomy. Stroke 2014;45:2036-40. [Crossref] [PubMed]