Predictive value of magnetic resonance imaging radiomics-based machine learning for disease progression in patients with high-grade glioma
Introduction
High-grade glioma (HGG) is a type of central nervous system tumor with a high degree of malignancy. The standard treatment strategy for HGG is surgical resection followed by adjuvant chemoradiotherapy (1). The median progression-free survival is about 7 months, even when the standard treatment strategy is used (2,3). Moreover, the recurrence time of HGG varies significantly between individuals (3), and the recurrence sites are primarily located in or near the primary tumor area (4).
Studies have shown that increasing the prescribed dose of radiotherapy can reduce local recurrence and improve prognosis (5). However, doing so also results in increased doses to normal tissues. In addition, a tradeoff between the target dose and doses to normal tissue is necessary when the target area conflicts with the organs at risk. This tradeoff is dependent primarily on the judgment of the treating physician and represents a difficult medical decision for junior physicians without extensive experience. Therefore, it is necessary to develop therapeutic efficacy prediction models for patient stratification to assist in clinical decision-making in terms of dose increment, radiotherapy plan tradeoff, adjuvant chemoradiotherapy strategy, and follow-up strategy, among other factors (6-9).
Currently, there are 3 types of prognosis prediction methods for cancer patients. The first is based on basic clinical information such as the World Health Organization (WHO) grade (10), isocitrate dehydrogenase mutation (11-14), and genetic factors (15,16). In this method, the focus is mainly on determining whether clinical features have potential predictive value, which is achieved by comparing whether there is a statistical difference between various groups. The second method is to predict the prognosis of a patient based on quantitative parameters obtained from functional imaging such as positron emission tomography (PET), diffusion-weighted imaging (DWI), and magnetic resonance imaging (MRI) perfusion (17-21). This method has been demonstrated to have good predictive value but requires additional image acquisition and, therefore, additional medical resources. The third method is based on the use of radiomics and deep learning to build prediction models from manually or automatically extracted image features (8,22-26). In recent years, several studies have focused on determining the prognosis of patients with glioma based on radiomics or deep learning methods (8,9,17). However, the predictive value of MRI images for prognosis at different time points is still unclear, as is the predictive value of using various MRI sequences and different regions of interest (ROIs).
In this study, our goal was to develop and validate models to predict disease progression or recurrence after treatment of patients with HGG based on postoperative MRI radiomics, with a focus on clinical applicability in terms of the existing medical practice in our hospital and avoiding the need to collect additional images. The value of MRI images in predicting prognosis at different time points and the predictive value of various MRI sequences of different ROIs were also investigated. Finally, based on our findings, we evaluated the performance of the constructed model at patient stratification. We present the following article in accordance with the TRIPOD reporting checklist (available at https://qims.amegroups.com/article/view/10.21037/qims-22-459/rc).
Methods
Patients
The study was conducted in accordance with the Declaration of Helsinki (as revised in 2013). The study was reviewed and approved by the ethics committee of the West China Hospital. Written informed consent was waived owing to the retrospective nature of this study. In this retrospective study, data obtained from patients with HGG treated in the West China Hospital between June 2017 and April 2020 were reviewed. The inclusion criteria were as follows: (I) patients with HGG confirmed by pathology and (II) patients who underwent surgical resection of glioma followed by adjuvant chemoradiotherapy at the West China Hospital. The exclusion criteria were as follows: (I) patients who had been treated for recurrent glioma, (II) patients who had not received standard adjuvant temozolomide chemotherapy, (III) patients without radiotherapy planning MRI, (IV) patients without follow-up information or with incomplete information, and (V) patients with less than 18 months follow-up and no recurrence at the end of the data collection period. A total of 162 patients were enrolled in the study.
MRI imaging
Radiotherapy planning MRI was used for radiomics feature extraction in this study. All MRI images were collected using a GE 3.0-T MRI scanner (Discovery 750 W, GE Healthcare, Chicago, IL, USA) following surgical resection of the glioma and before adjuvant chemoradiotherapy. All MRI scans were performed at least 3 weeks after surgical resection. The MRI sequences included T1-weighted imaging (T1WI), contrast-enhanced T1-weighted imaging (CE-T1WI), T2-weighted imaging (T2WI), and fluid-attenuated inversion recovery (FLAIR) imaging.
Treatment and follow-up
All patients enrolled in this retrospective study had undergone surgical treatment and postoperative adjuvant temozolomide chemoradiotherapy. Follow-up data for all patients were accessed from electronic medical records. Disease progression or recurrence was determined upon meeting any of the following criteria (27): a >25% increase in the products of perpendicular diameters of enhancing lesions, a significant increase in T2WI/FLAIR nonenhancing lesions, any new lesions, and clear clinical deterioration not attributable to other causes apart from the tumor. Based on these criteria, all follow-up data (including images and follow-up records) for each patient were reviewed independently by 2 physicians to determine the time of disease progression. As for disputed points, an agreement was reached after discussion between the 2 physicians.
Radiomics feature extraction
The ROIs for radiomics feature extraction came from the target volumes for radiotherapy planning, including gross tumor volume (GTV) and clinical target volume (CTV). They were delineated by the physician on fused computed tomography (CT) and MRI images. The GTV encompasses any GTV remaining after maximum safe resection as well as the volume of the surgical cavity as determined by postoperative T2WI and FLAIR images (28). The CTV is an expansion of the GTV to account for subclinical diseases. It includes GTV plus 1–2 cm of margin for grade III tumors and up to 2–2.5 cm of margin for grade IV tumors (28). The examples for GTV and CTV are shown in Figure 1.
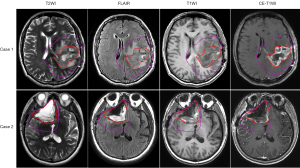
Generally, the signal intensity of MRI is affected by scanner and sequence parameters, which makes it difficult to quantitatively compare between different MRIs (29,30). To reduce the influence of these inconsistencies on the extracted radiomics features, the histogram normalization method (31) was used to normalize individual MRI images. All images were rescaled to 0–255, with prior points outside of the 1–99% intensity range excluded. Finally, all MRIs were resampled to a voxel size of 0.5 mm × 0.5 mm × 3 mm.
Based on each manually delineated ROI (GTV and CTV), radiomics features were automatically extracted from each MRI sequence (including T1WI, T2WI, CE-T1WI, and FLAIR) using PyRadiomics (32). Based on previous studies (29,33), the value of the bin width parameter was set to 6 to ensure that the number of bins was in an appropriate range. Laplacian of Gaussian (LoG) kernels with parameter values σ =1 and 3 mm were applied to strengthen various levels of texture features (34,35). A total of 107 radiomics features, including 14 shape features, 18 first-order features, and 75 texture features, were extracted from each original image, and 93 radiomics features were extracted from each LoG-transformed image (14 shape features were excluded). For each patient, a total of 2,344 radiomics features corresponding to the 4 MRI sequences and 2 ROIs were extracted.
Feature selection, prediction model, and performance evaluation
To build predictive models, the extracted radiomics features were combined with clinical features including WHO grade, sex, age, radiotherapy prescription dose, radiotherapy fraction, time interval from surgery to radiotherapy, and duration of radiotherapy. To make the features mutually comparable, each feature was normalized to have a mean value of 0 and a standard deviation of 1. To reduce interference from redundant and irrelevant radiomics features and to avoid overfitting, the Mann-Whitney U test and least absolute shrinkage and selection operator (LASSO) algorithm were used to perform feature selection (36). The Mann-Whitney U test was used for the preliminary filtering of features with P≤0.05, which was considered statistically significant, and the LASSO algorithm (37) was used to select the features that were ultimately used to build the predictive models.
The selected features were used to build machine learning models to predict the prognosis of patients with HGG with the goal of predicting whether disease progression or recurrence would be observed in the 6th, 9th, 12th, 15th, and 18th month after completion of radiotherapy. A total of 5 models were built using K-nearest neighbor (KNN), logistic regression, random forest (RF), support vector classification (SVC), and fully connected network (FCN) approaches. The first 4 models were built using the Scikit-learn package (38) while the last model was built using the PyTorch package (39). To build the FCN, we implemented 5 hidden layers with 64, 128, 256, 512, and 64 neurons each. The dropout strategy (40) was applied after each hidden layer to improve the generalizability of the models. Accordingly, the nonlinear fitting ability was promoted, and overfitting because of numerous parameters was alleviated.
Owing to the small sample size used in the study, the models were evaluated using 5×5-fold cross-validation, with 130 samples for the training set and 32 samples for the validation set in each fold. During each training phase, the data in the training set were divided into 2 parts based on 4-fold cross-validation, one of which was used for training the model and the other for model hyperparameter adjustment. Feature selection and model training were performed only on the training set, and the validation set was used only to evaluate the performance of the trained models. The receiver operating characteristic (ROC) curves and the areas under the ROC curves (AUCs) were calculated as comprehensive evaluation indices to evaluate the model performance in terms of sample sorting quality. This approach is suitable for obtaining robust evaluation results, particularly when the numbers of positive and negative samples are unbalanced.
To explore the prognostic prediction value of radiomics features extracted from different MRI sequences and ROIs, these radiomics features were used to build models separately, and their performance was compared. In addition, to explore the prognostic prediction value at different time points, models used to predict disease progression at the 6th, 9th, 12th, 15th, and 18th month after completion of radiotherapy were separately constructed and compared.
Finally, to evaluate the effectiveness of the predictive model in stratifying patients, the study patients were divided into high-risk and low-risk groups for recurrence and disease progression at the 12th month based on the predictive results. Considering 50% probability value as the cutoff value, the patients predicted to relapse were included in the high-risk group while the patients predicted to not relapse were included in the low-risk group.
Statistical methods
The log-rank test was used to compare Kaplan-Meier survival curves, and the DeLong test was used to compare the ROC curves of different models. All statistical tests were 2-sided with a statistical significance of P<0.05, and all analyses were performed using MedCalc version 20 (MedCalc Software, Ostend, Belgium).
Results
A total of 162 patients with primary HGG were enrolled in the study. The patient numbers used by the models (the number of samples used in the models) predicting disease progression and recurrence at the 6th, 9th, 12th, 15th, and 18th month were 162, 153, 145, 136, and 130, respectively. Among these, the number of cases of disease progression at the 6th, 9th, 12th, 15th, and 18th month (the number of positive samples) was 63, 71, 85, 98, and 103, respectively. All patients had undergone surgery followed by adjuvant chemoradiotherapy. The basic clinical information of the patients is summarized in Table 1.
Table 1
Clinical characteristic | Range | Count | Frequency |
---|---|---|---|
WHO grade | |||
WHO III | 58 | 36% | |
WHO IV | 104 | 64% | |
Gender | |||
Male | 97 | 60% | |
Female | 65 | 40% | |
Age (years) | |||
<20 | 5 | 3% | |
20–40 | 44 | 27% | |
40–60 | 84 | 52% | |
>60 | 29 | 18% | |
Prescription dose (Gy) | 58.5 (20–63.4) | ||
<60 | 26 | 16% | |
60 | 112 | 69% | |
>60 | 24 | 15% | |
Radiotherapy fractions | 29 (10–44) | ||
<30 | 21 | 13% | |
30 | 138 | 85% | |
>30 | 3 | 2% | |
Time interval from surgery to radiotherapy (days) | |||
<40 | 8 | 5% | |
40–90 | 133 | 82% | |
>90 | 21 | 13% | |
Duration of radiotherapy (days) | |||
<40 | 37 | 23% | |
40–45 | 105 | 65% | |
>45 | 20 | 12% |
WHO, World Health Organization.
The AUC for predicting disease progression and recurrence at the 6th, 9th, 12th, 15th, and 18th month after completing radiotherapy when all radiomics and clinical features were used for feature selection and model building is shown in Figure 2. Similar results were observed for models built using different machine learning methods, and the P value was between 0.07 and 0.84 when models were compared to each other using the DeLong test. The performance for predicting recurrence at the 12th, 15th, and 18th month was better than that at the 6th and 9th month. The DeLong test revealed that there was no significant difference between the models’ predictions for the 12th month and those for the 18th month (P=0.55 using the DeLong test), but there were significant differences between the models’ predictions for the 12th month and those for the 6th and 9th month (P<0.001 using the DeLong test). Since the models performed better for predicting disease progression at the 12th month and the positive and negative samples were relatively balanced for this task, we chose recurrence or disease progression at the 12th month as the prediction target in our subsequent analysis.
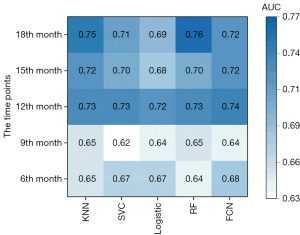
To evaluate the predictive value of single MRI sequences, radiomics features extracted from a single-sequence MRI (including GTV and CTV features) combined with clinical features were used for feature selection and model building. The AUC for predicting recurrence and disease progression at the 12th month after completing radiotherapy is shown in Figure 3. Models using radiomics features from only T2WI or FLAIR outperformed those from CE-T1WI and T1WI (P<0.001 using the DeLong test). In addition, the models combining radiomics features from T2WI and FLAIR outperformed those using radiomics features from any single MRI sequence and significantly outperformed those using features from all 4 MRI sequences (P<0.001 using the DeLong test), as illustrated in Figure 4.
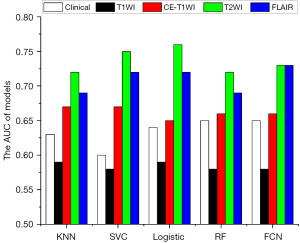
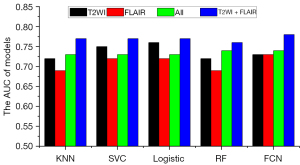
To evaluate the predictive value of radiomics features extracted from different ROIs, features extracted from the GTV and CTV were used separately for feature selection and model building. The AUC for predicting recurrence and disease progression at the 12th month after completion of radiotherapy is shown in Figure 5. Although the models using only CTV features outperformed those using GTV features (P<0.001 using the DeLong test), model performance did not improve when a combination of CTV and GTV features was used (P=0.544 using the DeLong test).
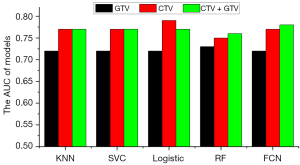
For insight into important features, feature selection was repeated 5 times in a 5-fold cross-validation of models using clinical features and CTV features, and the final number of features selected for the machine learning model construction ranged from 10 to 20. Five features were repeatedly selected in each feature selection, including a clinical feature (grad) and 4 radiomics features [high gray level zone emphasis in the gray level size zone matrix (GLSZM), long run high gray level emphasis in the gray level run length matrix (GLRLM), first-order range, and large dependence low gray level emphasis in the gray level dependence matrix (GLDM)]. The distribution of these feature values was significantly different among patients grouped by disease progression at the 12th month (Figure 6). Furthermore, the relationship between these selected CTV features and their corresponding GTV features is shown in Figure 7, and the Pearson correlation coefficients were found to be 0.78, 0.71, 0.52, and 0.48, respectively, for the high gray level zone emphasis, long run high gray level emphasis, large dependence low gray level emphasis, and first-order range features extracted from the GTV and CTV.
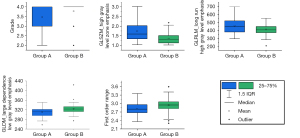
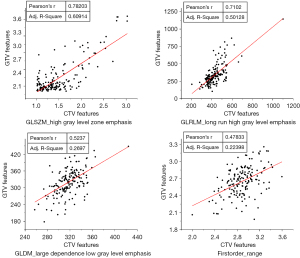
The AUCs for the KNN, SVC, logistic regression, RF, and FCN models built using clinical features alone were 0.63, 0.60, 0.64, 0.65, and 0.65, respectively. The AUCs for the KNN, SVC, logistic regression, RF, and FCN models built using radiomics features alone were 0.77, 0.76, 0.76, 0.75, and 0.77, respectively; combining clinical and radiomics features produced corresponding AUCs of 0.77, 0.77, 0.76, 0.76, and 0.78, respectively, for the KNN, SVC, logistic regression, RF, and FCN models. The predictive values of the radiomics features were significantly higher than those of the clinical features (P<0.001 using the DeLong test), and the addition of clinical features did not significantly improve the performance of any of the models. The ROC curves are shown in Figure 8A.
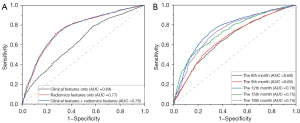
These results indicate that the radiomics features extracted from MRI images obtained before radiotherapy have good predictive value for the progression of HGG at 12 months after radiotherapy. The prediction models performed well when they were built using clinical and radiomics features extracted from T2WI and FLAIR (including GTV and CTV features). The AUCs of the best model were 0.70, 0.68, 0.78, 0.78, and 0.78, respectively, for predicting recurrence and disease progression at the 6th, 9th, 12th, 15th, and 18th month after completion of radiotherapy. The ROC curves are shown in Figure 8B.
To evaluate the effectiveness of the predictive model in stratifying patients, the study patients were divided into high-risk and low-risk groups for recurrence and disease progression at the 12th month based on the predictive results. Consequently, the high-risk group exhibited shorter disease-free survival compared to the low-risk group, as illustrated in Figure 9 (P<0.001 using the log-rank test). The median time to progression was 9.5 months for all patients, while the median time to progression was 5.1 and 18.3 months for the high-risk group and low-risk group, respectively. Additionally, 74%, 67%, and 40% of patients had progression-free survival longer than 6, 12, and 18 months in the low-risk group, respectively. In the high-risk group, 48%, 29%, and 8% of patients had progression-free survival longer than 6, 12, and 18 months, respectively.
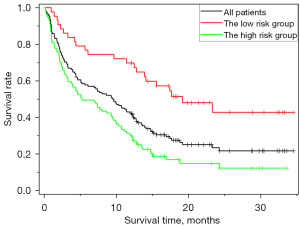
Discussion
In this study, the predictive value of MRI for disease progression and recurrence in patients with HGG was investigated using radiomics and machine learning. The influence of different MRI sequences, ROIs, and prediction time points on prediction results was also explored. The development and application of radiomics and machine learning approaches have enabled the extraction of data and image attributes from medical images to assist clinical practice. To this end, the medical images obtained in this study were used to predict patient outcomes to aid in clinical decision-making on factors including dose increment, radiotherapy plan tradeoff, adjuvant chemoradiotherapy strategy, and follow-up strategy.
To focus on the value of MRI in predicting patient outcomes, we assessed 5 machine learning models and obtained similar results for each model. Models for predicting disease progression and recurrence at the 12th, 15th, and 18th month after radiotherapy were superior to those for predicting disease progression at the 6th and 9th month. These results suggest that structural MRI information obtained between surgery and radiotherapy had significant predictive value in determining the long-term prognoses for patients with HGG.
Previously, Lundemann et al. (17) predicted the recurrence region of glioma based on PET, DWI, and dynamic contrast-enhanced imaging, and obtained a voxel-based prediction model with an AUC of 0.77. Tan et al. (9) analyzed CE-TIWI and FLAIR images for diagnosing HGG using radiomics and built models to predict overall survival (OS), obtaining a concordance index (C-index) of 0.76. Liu et al. (8) used a similar method to analyze T2WI images for low-grade glioma and obtained a C-index of 0.82. The AUC and C-index are indicators reflecting the sorting ability of models, with AUC constituting a special case of the C-index on the binary classification task, and both are comparable to a certain extent. In our study, the AUC of the best model was 0.78, which was similar to the results obtained in the above-mentioned research. Based on the results of our prediction models, patients could be easily stratified into 2 subgroups. The patients in the low-risk group had a significantly longer median time to progression than did those in the high-risk group. In general, our results indicate that postoperative structural MRI images alone have certain predictive value in forecasting disease progression in glioma patients.
Multiple MRI sequence types, including T1WI, CE-T1WI, T2WI, and FLAIR, were used in this study. Although these are all structural MRI sequence types, the results of this study indicate that T2WI and FLAIR images are more valuable in predicting HGG disease prognosis than are T1WI or CE-T1WI, possibly because slightly better tumor identification can be obtained from T2WI images than from T1WI or CE-T1WI images (8). Combining the radiomics features extracted from T2WI and FLAIR improved the prediction results, suggesting that T2WI and FLAIR provide complementary information for prognosis prediction. However, combining radiomics features extracted from all 4 MRI sequences degraded the prediction performance, possibly because using all of the radiomics features resulted in overfitting (41). Studies with significantly larger sample sizes are needed to confirm this.
Radiomics is an ROI-specific image analysis method. Takada et al. (42) demonstrated that the ROI range has an impact on the radiomics analysis results, with better results observed when the ROI is properly expanded based on the target area. In this study, we performed radiomics analysis based on both GTV and CTV and found that models built using CTV radiomics features outperformed those using GTV radiomics. Combining GTV and CTV features did not improve the performance of the models, as the CTV includes the GTV as well as potential lesion areas around the tumor. This may be because some GTV and CTV features are correlated and provide redundant information. Another possibility is that CTV features are more robust to ROI contouring errors compared to GTV features. Further research is needed to explore this.
Tsien et al. (5) demonstrated that increasing the prescription dose of radiotherapy can prolong the OS time. However, adding clinical features (including dose features) during model construction did not improve the performance of the models in this study, possibly because of the redundancy between clinical and radiomics features or the difference between the 2 studies in terms of the maximum prescribed doses. In this study, there were no significant differences in the prescribed doses: 69% of the patients were prescribed 60 Gy, and the maximum prescribed dose was 63.9 Gy. In Tsien et al.’s study, by contrast, there was a significant prescribed dose stratification with a maximum prescribed dose of 81 Gy. The predictive value of prescription doses for patient prognosis requires further study.
Despite its careful design, there were some limitations to this study. First, because this was a retrospective study, there were gaps in the details of the treatment course. The research was conducted based on information retained by the hospital information system. Furthermore, only 162 patients were enrolled in the study, and all were from the same hospital. Based on this limited data, cross-validation was used to evaluate our models and no external validation set was used. Thus, more data from multiple centers will be needed to verify the generalizability of the results. Finally, radiomics features were extracted using PyRadiomics without considering the influence of the radiomics software used.
Conclusions
In this study, it was shown that structural MRI obtained before radiotherapy can be used to predict disease progression or recurrence after treatment for HGG, with better results observed in the prediction of long-term outcomes. T2WI and FLAIR demonstrated greater predictive value than did T1WI and CE-T1WI in predicting the prognosis of HGG. Models using radiomics features extracted from the CTV outperformed those using features obtained from the GTV. The prediction models could effectively stratify patients with HGG, suggesting the usefulness of the proposed method as an aid in clinical radiotherapy plans and decision-making.
Acknowledgments
Funding: This work was supported by the National Natural Science Foundation of China (Nos. 81972848, 81472807, and 81803056) and the Science and Technology Department of Sichuan Province (No. 2021YFS0143).
Footnote
Reporting Checklist: The authors have completed the TRIPOD reporting checklist. Available at https://qims.amegroups.com/article/view/10.21037/qims-22-459/rc
Conflicts of Interest: All authors have completed the ICMJE uniform disclosure form (available at https://qims.amegroups.com/article/view/10.21037/qims-22-459/coif). The authors have no conflicts of interest to declare.
Ethical Statement: The authors are accountable for all aspects of the work in ensuring that questions related to the accuracy or integrity of any part of the work are appropriately investigated and resolved. The study was conducted in accordance with the Declaration of Helsinki (as revised in 2013). The study was approved by the institutional ethics committee of West China Hospital, and individual consent for this retrospective analysis was waived.
Open Access Statement: This is an Open Access article distributed in accordance with the Creative Commons Attribution-NonCommercial-NoDerivs 4.0 International License (CC BY-NC-ND 4.0), which permits the non-commercial replication and distribution of the article with the strict proviso that no changes or edits are made and the original work is properly cited (including links to both the formal publication through the relevant DOI and the license). See: https://creativecommons.org/licenses/by-nc-nd/4.0/.
References
- Stupp R, Dietrich PY, Ostermann Kraljevic S, Pica A, Maillard I, Maeder P, Meuli R, Janzer R, Pizzolato G, Miralbell R, Porchet F, Regli L, de Tribolet N, Mirimanoff RO, Leyvraz S. Promising survival for patients with newly diagnosed glioblastoma multiforme treated with concomitant radiation plus temozolomide followed by adjuvant temozolomide. J Clin Oncol 2002;20:1375-82. [Crossref] [PubMed]
- Aulakh S, DeDeo MR, Free J, Rosenfeld SS, Quinones-Hinojosa A, Paulus A, Manna A, Manochakian R, Chanan-Khan AA, Ailawadhi S. Survival trends in glioblastoma and association with treating facility volume. J Clin Neurosci 2019;68:271-4. [Crossref] [PubMed]
- Stupp R, Hegi ME, Mason WP, van den Bent MJ, Taphoorn MJ, Janzer RC, et al. Effects of radiotherapy with concomitant and adjuvant temozolomide versus radiotherapy alone on survival in glioblastoma in a randomised phase III study: 5-year analysis of the EORTC-NCIC trial. Lancet Oncol 2009;10:459-66. [Crossref] [PubMed]
- Minniti G, Amelio D, Amichetti M, Salvati M, Muni R, Bozzao A, Lanzetta G, Scarpino S, Arcella A, Enrici RM. Patterns of failure and comparison of different target volume delineations in patients with glioblastoma treated with conformal radiotherapy plus concomitant and adjuvant temozolomide. Radiother Oncol 2010;97:377-81. [Crossref] [PubMed]
- Tsien CI, Brown D, Normolle D, Schipper M, Piert M, Junck L, Heth J, Gomez-Hassan D, Ten Haken RK, Chenevert T, Cao Y, Lawrence T. Concurrent temozolomide and dose-escalated intensity-modulated radiation therapy in newly diagnosed glioblastoma. Clin Cancer Res 2012;18:273-9. [Crossref] [PubMed]
- Mizutani T, Magome T, Igaki H, Haga A, Nawa K, Sekiya N, Nakagawa K. Optimization of treatment strategy by using a machine learning model to predict survival time of patients with malignant glioma after radiotherapy. J Radiat Res 2019;60:818-24. [Crossref] [PubMed]
- Wu G, Shi Z, Chen Y, Wang Y, Yu J, Lv X, Chen L, Ju X, Chen Z. A sparse representation-based radiomics for outcome prediction of higher grade gliomas. Med Phys 2019;46:250-61. [Crossref] [PubMed]
- Liu X, Li Y, Qian Z, Sun Z, Xu K, Wang K, Liu S, Fan X, Li S, Zhang Z, Jiang T, Wang Y. A radiomic signature as a non-invasive predictor of progression-free survival in patients with lower-grade gliomas. Neuroimage Clin 2018;20:1070-7. [Crossref] [PubMed]
- Tan Y, Mu W, Wang XC, Yang GQ, Gillies RJ, Zhang H. Improving survival prediction of high-grade glioma via machine learning techniques based on MRI radiomic, genetic and clinical risk factors. Eur J Radiol 2019;120:108609. [Crossref] [PubMed]
- Louis DN, Perry A, Reifenberger G, von Deimling A, Figarella-Branger D, Cavenee WK, Ohgaki H, Wiestler OD, Kleihues P, Ellison DW. The 2016 World Health Organization Classification of Tumors of the Central Nervous System: a summary. Acta Neuropathol 2016;131:803-20. [Crossref] [PubMed]
- Wang HY, Tang K, Liang TY, Zhang WZ, Li JY, Wang W, Hu HM, Li MY, Wang HQ, He XZ, Zhu ZY, Liu YW, Zhang SZ. The comparison of clinical and biological characteristics between IDH1 and IDH2 mutations in gliomas. J Exp Clin Cancer Res 2016;35:86. [Crossref] [PubMed]
- Bralten LB, Kloosterhof NK, Balvers R, Sacchetti A, Lapre L, Lamfers M, Leenstra S, de Jonge H, Kros JM, Jansen EE, Struys EA, Jakobs C, Salomons GS, Diks SH, Peppelenbosch M, Kremer A, Hoogenraad CC, Smitt PA, French PJ. IDH1 R132H decreases proliferation of glioma cell lines in vitro and in vivo. Ann Neurol 2011;69:455-63. [Crossref] [PubMed]
- van den Bent MJ, Dubbink HJ, Marie Y, Brandes AA, Taphoorn MJ, Wesseling P, Frenay M, Tijssen CC, Lacombe D, Idbaih A, van Marion R, Kros JM, Dinjens WN, Gorlia T, Sanson M. IDH1 and IDH2 mutations are prognostic but not predictive for outcome in anaplastic oligodendroglial tumors: a report of the European Organization for Research and Treatment of Cancer Brain Tumor Group. Clin Cancer Res 2010;16:1597-604. [Crossref] [PubMed]
- Hartmann C, Meyer J, Balss J, Capper D, Mueller W, Christians A, Felsberg J, Wolter M, Mawrin C, Wick W, Weller M, Herold-Mende C, Unterberg A, Jeuken JW, Wesseling P, Reifenberger G, von Deimling A. Type and frequency of IDH1 and IDH2 mutations are related to astrocytic and oligodendroglial differentiation and age: a study of 1,010 diffuse gliomas. Acta Neuropathol 2009;118:469-74. [Crossref] [PubMed]
- Wu S, Qiao Q, Li G. A Radiosensitivity Gene Signature and XPO1 Predict Clinical Outcomes for Glioma Patients. Front Oncol 2020;10:871. [Crossref] [PubMed]
- Yang K, Jung SW, Shin H, Lim DH, Lee JI, Kong DS, Seol HJ, Kim ST, Nam DH. Cancer genetic markers according to radiotherapeutic response in patients with primary glioblastoma - Radiogenomic approach for precision medicine. Radiother Oncol 2019;131:66-74. [Crossref] [PubMed]
- Lundemann M, Munck Af Rosenschöld P, Muhic A, Larsen VA, Poulsen HS, Engelholm SA, Andersen FL, Kjær A, Larsson HBW, Law I, Hansen AE. Feasibility of multi-parametric PET and MRI for prediction of tumour recurrence in patients with glioblastoma. Eur J Nucl Med Mol Imaging 2019;46:603-13. [Crossref] [PubMed]
- Carceller F, Jerome NP, Fowkes LA, Khabra K, Mackinnon A, Bautista F, Marshall LV, Vaidya S, Mandeville H, Morgan V, Leach MO, Koh DM. Post-radiotherapy apparent diffusion coefficient (ADC) in children and young adults with high-grade gliomas and diffuse intrinsic pontine gliomas. Pediatr Hematol Oncol 2019;36:103-12. [Crossref] [PubMed]
- Chakhoyan A, Woodworth DC, Harris RJ, Lai A, Nghiemphu PL, Liau LM, Pope WB, Cloughesy TF, Ellingson BM. Mono-exponential, diffusion kurtosis and stretched exponential diffusion MR imaging response to chemoradiation in newly diagnosed glioblastoma. J Neurooncol 2018;139:651-9. [Crossref] [PubMed]
- Brynolfsson P, Nilsson D, Henriksson R, Hauksson J, Karlsson M, Garpebring A, Birgander R, Trygg J, Nyholm T, Asklund T. ADC texture--an imaging biomarker for high-grade glioma? Med Phys 2014;41:101903. [Crossref] [PubMed]
- Garrett MD, Yanagihara TK, Yeh R, McKhann GM, Sisti MB, Bruce JN, Sheth SA, Sonabend AM, Wang TJC. Monitoring Radiation Treatment Effects in Glioblastoma: FLAIR Volume as Significant Predictor of Survival. Tomography 2017;3:131-7. [Crossref] [PubMed]
- Choi YS, Ahn SS, Chang JH, Kang SG, Kim EH, Kim SH, Jain R, Lee SK. Machine learning and radiomic phenotyping of lower grade gliomas: improving survival prediction. Eur Radiol 2020;30:3834-42. [Crossref] [PubMed]
- Sun L, Zhang S, Chen H, Luo L. Brain Tumor Segmentation and Survival Prediction Using Multimodal MRI Scans With Deep Learning. Front Neurosci 2019;13:810. [Crossref] [PubMed]
- Zhong LZ, Fang XL, Dong D, Peng H, Fang MJ, Huang CL, He BX, Lin L, Ma J, Tang LL, Tian J. A deep learning MR-based radiomic nomogram may predict survival for nasopharyngeal carcinoma patients with stage T3N1M0. Radiother Oncol 2020;151:1-9. [Crossref] [PubMed]
- Shi L, Zhang Y, Nie K, Sun X, Niu T, Yue N, Kwong T, Chang P, Chow D, Chen JH, Su MY. Machine learning for prediction of chemoradiation therapy response in rectal cancer using pre-treatment and mid-radiation multi-parametric MRI. Magn Reson Imaging 2019;61:33-40. [Crossref] [PubMed]
- Ou J, Wu L, Li R, Wu CQ, Liu J, Chen TW, Zhang XM, Tang S, Wu YP, Yang LQ, Tan BG, Lu FL. CT radiomics features to predict lymph node metastasis in advanced esophageal squamous cell carcinoma and to discriminate between regional and non-regional lymph node metastasis: a case control study. Quant Imaging Med Surg 2021;11:628-40. [Crossref] [PubMed]
- Wen PY, Macdonald DR, Reardon DA, Cloughesy TF, Sorensen AG, Galanis E, Degroot J, Wick W, Gilbert MR, Lassman AB, Tsien C, Mikkelsen T, Wong ET, Chamberlain MC, Stupp R, Lamborn KR, Vogelbaum MA, van den Bent MJ, Chang SM. Updated response assessment criteria for high-grade gliomas: response assessment in neuro-oncology working group. J Clin Oncol 2010;28:1963-72. [Crossref] [PubMed]
- National Comprehensive Cancer Network. NCCN clinical practice guidelines in oncology: central nervous system cancers (Version 1.2017). 2017. Available online: https://www.nccn.org/guidelines/guidelines-detail?category=1&id=1425
- Bologna M, Corino V, Mainardi L. Technical Note: Virtual phantom analyses for preprocessing evaluation and detection of a robust feature set for MRI-radiomics of the brain. Med Phys 2019;46:5116-23. [Crossref] [PubMed]
- Yuan J, Xue C, Lo G, Wong OL, Zhou Y, Yu SK, Cheung KY. Quantitative assessment of acquisition imaging parameters on MRI radiomics features: a prospective anthropomorphic phantom study using a 3D-T2W-TSE sequence for MR-guided-radiotherapy. Quant Imaging Med Surg 2021;11:1870-87. [Crossref] [PubMed]
- Nyúl LG, Udupa JK, Zhang X. New variants of a method of MRI scale standardization. IEEE Trans Med Imaging 2000;19:143-50. [Crossref] [PubMed]
- van Griethuysen JJM, Fedorov A, Parmar C, Hosny A, Aucoin N, Narayan V, Beets-Tan RGH, Fillion-Robin JC, Pieper S, Aerts HJWL. Computational Radiomics System to Decode the Radiographic Phenotype. Cancer Res 2017;77:e104-7. [Crossref] [PubMed]
- Yip SS, Aerts HJ. Applications and limitations of radiomics. Phys Med Biol 2016;61:R150-66. [Crossref] [PubMed]
- Shayesteh SP, Alikhassi A, Fard Esfahani A, Miraie M, Geramifar P, Bitarafan-Rajabi A, Haddad P. Neo-adjuvant chemoradiotherapy response prediction using MRI based ensemble learning method in rectal cancer patients. Phys Med 2019;62:111-9. [Crossref] [PubMed]
- Dinapoli N, Barbaro B, Gatta R, Chiloiro G, Casà C, Masciocchi C, et al. Magnetic Resonance, Vendor-independent, Intensity Histogram Analysis Predicting Pathologic Complete Response After Radiochemotherapy of Rectal Cancer. Int J Radiat Oncol Biol Phys 2018;102:765-74. [Crossref] [PubMed]
- Huang J, Yao H, Li Y, Dong M, Han C, He L, Huang X, Xia T, Yi Z, Wang H, Zhang Y, He J, Liang C, Liu Z. Development and validation of a CT-based radiomics nomogram for preoperative prediction of tumor histologic grade in gastric adenocarcinoma. Chin J Cancer Res 2021;33:69-78. [Crossref] [PubMed]
- Tibshirani R. Regression shrinkage and selection via the lasso. Journal of the Royal Statistical Society: Series B (Methodological) 1996;58:267-88. [Crossref]
- Pedregosa F, Varoquaux G, Gramfort A, Michel V, Thirion B, Grisel O, Blondel M, Prettenhofer P, Weiss R, Dubourg V, Vanderplas J, Passos A, Cournapeau D, Brucher M, Perrot M, Duchesnay É. Scikit-learn: machine learning in Python. J Mach Learn Res 2011;12:2825-30.
- Paszke A, Gross S, Chintala S, Chanan G, Yang E, DeVito Z, Lin Z, Desmaison A, Antiga L, Lerer A. Automatic differentiation in PyTorch. 2017. Available online: https://pytorch.org/
- Srivastava N, Hinton G, Krizhevsky A, Sutskever I, Salakhutdinov R. Dropout: a simple way to prevent neural networks from overfitting. J Mach Learn Res 2014;15:1929-58.
- Babyak MA. What you see may not be what you get: a brief, nontechnical introduction to overfitting in regression-type models. Psychosom Med 2004;66:411-21. [PubMed]
- Takada A, Yokota H, Watanabe Nemoto M, Horikoshi T, Matsushima J, Uno T. A multi-scanner study of MRI radiomics in uterine cervical cancer: prediction of in-field tumor control after definitive radiotherapy based on a machine learning method including peritumoral regions. Jpn J Radiol 2020;38:265-73. [Crossref] [PubMed]