Development and internal validation of a conventional ultrasound-based nomogram for predicting malignant nonmasslike breast lesions
Introduction
An increasing number of nonmasslike (NML) breast lesions that do not adhere to the fifth edition of the Breast Imaging Reporting and Data System (BI-RADS) criteria of a mass are being identified due to the recent advances in ultrasound (US) technology (1). It is challenging to differentiate benign from malignant NML breast lesions on conventional US alone because of their wide spectrum of pathologic changes and the lack of specific US features (2). BI-RADS provides diagnostic efficiency in the interpreting and reporting of breast masses by US with a thorough evaluation of shape, orientation, margin, echo pattern, posterior features, calcifications, and associated features of breast lesions (3). However, BI-RADS might be less effective in the differential diagnosis of breast NML lesions because both benign and malignant lesions have irregular shapes and non-circumscribed margins. Some US features, including calcification, flow signal, location, number of lesions, and architectural distortion, have been reported as potentially related to malignant NML breast lesions (4). However, these studies have only provided radiologists with information about certain factors associated with a higher risk of malignant lesions, and they have had limited predictive power and application in clinical practice.
Recently, it was reported that automated breast volume scanning, shear-wave elastography, and contrast-enhanced ultrasonography could improve the diagnostic efficiency of US in identifying malignant NML breast lesions, to some extent (5-7). However, the requirement of expensive specialized equipment and additional operator training have largely limited their application in the primary screening of breast lesions. A comprehensive, simplified, and effective tool that provides a quantified, individualized risk prediction of malignant NML breast lesions on the basis of conventional US-based features is strongly needed for US physicians making a preliminary diagnosis. Consequently, we aimed to develop a conventional, US feature–based nomogram for the prediction of malignant NML breast lesions with adequate diagnosis efficiency to enable NML breast lesion differential diagnosis by radiologists immediately after US examination. We present the following article in accordance with the STARD reporting checklist (available at https://qims.amegroups.com/article/view/10.21037/qims-22-378/rc) (8).
Methods
Participants
The present study was conducted in accordance with the Declaration of Helsinki (as revised in 2013). The study design was approved by the Institutional Review Board of the Guangdong Province Hospital of Chinese Medicine, and individual consent for retrospective analysis was waived.
Consecutive patients were identified from the electronic medical records of our hospital if they met the following criteria: (I) female aged ≥18 years and (II) diagnosed with NML breast lesions by US screening from June 1st, 2017, to April 17th, 2020. The exclusion criteria were the following: (I) patients with a previous history of surgery, radiotherapy, or chemotherapy in the involved breast; (II) pregnant or breastfeeding women; (III) patients without intact US data; (IV) patients without an exact pathology.
Data collection
Information on age, clinical symptoms, and pathology of the NML breast lesions of enrolled patients were retrospectively collected from the database. Clinical symptoms included pain, bloody discharge, self-detected breast mass, and other related chief complaints. All the results of pathology were obtained by biopsy or surgical resection.
Image interpretation
All US examinations were conducted with a high-resolution US unit (LOGIQ E9; GE Medical, Milwaukee, WI, USA). The US images were independently interpreted by 2 experienced radiologists who were blinded to the pathology. Interpretations were made by senior doctors when the radiologists’ opinions differed. US characteristics included the number of lesions, location of lesions classified by quadrant, maximum diameter of lesions, orientation (parallel and non-parallel) of lesions, stripelike hypo-echoic lines within lesions, echo patterns, posterior features, calcification, and flow signals graded by Adler’s classification. According to the recommendation of a previous study (2), the echo pattern of a lesion was classified as parallel to ductal-like structures, a non–ductal-like hypo-echoic area, or an area of architectural distortion.
Adler’s classification was used to assess the grade of detected vascularity. Vascularity was categorized from Grade 0 to Grade 3, depending on the amount of vascularity. Grade 0 was regarded as absent vascularity. Minimal (Grade 1) flow was generally regarded as an area of 1 or 2 pixels containing flow (<0.1 cm in diameter). When a certain number of small vessels and/or a main vessel was seen, clinicians graded the vascularity as moderate (Grade 2). Marked (Grade 3) vascularity was rated when 4 or more vessels were visualized (9).
BI-RADS assessment categories were also obtained by corresponding radiologists. According to the BI-RADS Atlas Fifth Edition of the American College of Rheumatology (ACR) (10), the lexicon of the BI-RADS classification of breast lesions includes shape, orientation, margin, echo pattern, posterior features, calcifications, and associated features. However, for breast NML lesions, both benign and malignant lesions have irregular shape and a non-circumscribed margin. Thus, the evaluation of shape and margin was not included in the present study.
Sample size
No gold-standard approach is currently available for the calculation of the sample size requirements of risk prediction models. However, it is widely accepted that at least 10 events per candidate variable for the logistic regression analysis are needed for the derivation of a risk prediction model (11). Thus, at least 120 patients were required for our study, as 12 candidate variables were included in the multivariable regression analysis.
Statistical analysis
Continuous data are expressed as means ± standard deviations or medians with interquartile ranges, while categorical data are presented as percentages and counts. Prediction models for the binary outcomes were developed via multivariable logistic regression. Clinical symptoms and the number of lesions were transformed into binary variables. Candidate variables with a P value <0.1 after multivariable logistic analysis in the screening step were included in the nomogram. The relative importance of each predictor in the model was determined by subtracting the degrees of freedom from the Wald chi-square value (12). Odds ratios and 95% confidence intervals were also calculated for each variable. To further investigate the potential influence caused by multicollinearity of the candidate variables, principal component analysis (PCA) and variance inflation factor (VIF) of the multivariable logistic analysis were completed through calculations using R version 3.5.0 (R Foundation for Statistical Computing) with a specific package (“car”).
Following the instruction of a previous tutorial paper (11), R version 3.5.0 and R package “rms” were used for the development and validation of our nomogram. One thousand bootstrap samples were drawn to correct the bias and were used for internal validation. Referring to previous studies (13,14), we obtained predicted probabilities for the original sample based on each estimated model of bootstrap and concordance index (C-index) calculation. The bias-corrected C-index was defined as the average of these bootstrap C-indices, representing the ability to distinguish between patients who experience an event from those who do not (12). The C-index was measured on a scale of 0.5 (no better than chance) to 1 (perfect discrimination) (14). Overall accuracy and calibration were visualized by comparing predicted versus actual probabilities, including a bias correction for overfitting (13,14). The prediction performance of the nomogram was also quantified using the receiver operating characteristic (ROC) analysis with the area under the curve (AUC).
As previously described (15), a decision curve analysis (DCA) was performed to obtain the final ranges for threshold probabilities and to compare the clinical benefits of the nomogram and BI-RADS using the “rmda” package. A P value of ≤0.05 was set as the significance level. Statistical analysis was performed using SPSS v. 20.0 (IBM Corp., Armonk, NY, USA) and R software programs.
Results
Clinical and pathologic characteristics
As shown in Figure 1, 229 patients were included in the present study after exclusion and follow-up. The mean (or percentage) for each candidate variable of the benign lesion group, the malignant lesion group, and the entire data set are shown in Table 1. Significant differences in age, clinical symptoms, stripelike hypo-echoic lines, echo pattern, calcification, and Adler’s classification were found between the benign and the malignant lesion groups. We found that calcification was much less common in benign cases (64.2% vs. 73.4%; P<0.001). The malignant rate of NML lesions in the entire cohort was 31.0%. Details of pathology are shown in Table 2, and representative images of both benign and malignant NML breast lesions are shown in Figure 2. More details on the baseline characteristics, including age, clinical symptoms, origin of the participants, and the US-based BI-RADS classification, are provided in Table S1.
Table 1
Items | Total (n=229) | Benign lesion (n=158) | Malignant lesion (n=71) | P value |
---|---|---|---|---|
Age (years) | 44.3±11.0 | 42.1±10.3 | 49.1±11.2 | <0.001 |
Symptoms | 45 (19.7) | 38 (24.1) | 7 (9.9) | 0.012 |
Number of the lesions (>1) | 50 (21.8) | 37 (23.4) | 13 (18.3) | 0.387 |
Location of the lesions | 0.644 | |||
Upper outer quadrant | 91 (39.7) | 66 (41.8) | 25 (35.2) | |
Other quadrants | 63 (27.5) | 42 (26.6) | 21 (29.6) | |
≥2 quadrants | 75 (32.8) | 50 (31.6) | 25 (35.2) | |
Diameters of the lesions (mm) | 30.0±22.4 | 28.9±23.2 | 32.4±20.3 | 0.279 |
Orientation (Parallel) | 205 (89.5) | 144 (91.1) | 61 (85.9) | 0.233 |
Stripelike hypo-echoic lines | 161 (70.3) | 118 (74.7) | 43 (60.6) | 0.031 |
Echo pattern | 0.004 | |||
Parallel to ductal-like structures | 65 (28.4) | 39 (24.7) | 26 (36.6) | |
Non–ductal-like hypo-echoic area | 111 (48.5) | 73 (46.2) | 38 (53.5) | |
Architectural distortion | 53 (23.1) | 46 (29.1) | 7 (9.9) | |
Posterior features | 150 (65.5) | 104 (65.8) | 46 (64.8) | 0.879 |
Calcification | 147 (64.2) | 116 (73.4) | 31 (43.7) | <0.001 |
Other features* | 204 (89.1) | 140 (88.6) | 64 (90.1) | 0.731 |
Adler classification | <0.001 | |||
Level 0 | 119 (52.0) | 96 (60.8) | 23 (32.4) | |
Level 1 | 53 (23.1) | 37 (23.4) | 16 (22.5) | |
Level 2 | 31 (13.5) | 10 (6.3) | 21 (29.6) | |
Level 3 | 26 (11.4) | 15 (9.5) | 11 (15.5) |
Numbers are presented as mean ± standard deviation or n (%). *, other features included skin thickening, skin contraction, and edema.
Table 2
Items | N |
---|---|
Benign lesions | |
Fibrocystic breast disease | 65 |
Chronic mastitis | 41 |
Cyclomastopathy | 23 |
Intraductal papilloma | 11 |
Fibroadenoma | 10 |
Sclerosing adenosis | 4 |
Atypical breast intraductal hyperplasia | 3 |
Hematoma | 1 |
Malignant lesions | |
Invasive ductal carcinoma | 35 |
Ductal carcinoma in situ | 28 |
Invasive lobular carcinoma | 3 |
Invasive ductal carcinoma + micropapillary carcinoma | 2 |
Paget disease | 2 |
Invasive mucinous carcinoma | 1 |
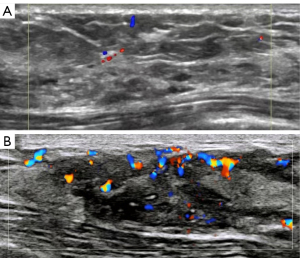
Predictors of malignant NML breast lesions
Six variables, including age, clinical symptoms, echo pattern, calcification, orientation, and Adler’s classification, were included to develop the nomogram according to the results of the multivariable logistic regression analysis. The odds ratios with 95% confidence intervals of the 6 selected variables are available in Table 3. The relative predictive power of each selected variable is provided in Figure 3, which shows age having the largest influence. Results of the PCA and VIF are shown in Table S2, Table S3, respectively.
Table 3
Variables (ref/unit) | Coefficient | Standard error | P value | OR | 95% confidence interval |
---|---|---|---|---|---|
Age (years) | 0.081 | 0.02 | 0 | 1.084 | 1.043–1.126 |
Symptoms (n) | 1.014 | 0.513 | 0.048 | 2.756 | 1.008–7.537 |
Number of lesions (>1) | 0.468 | 0.489 | 0.338 | 1.597 | 0.612–4.167 |
Location of lesions (Upper outer quadrant) | 0.528 | ||||
Other quadrants | −0.396 | 0.445 | 0.373 | 0.673 | 0.281–1.61 |
≥2 quadrants | 0.059 | 0.476 | 0.901 | 1.061 | 0.418–2.695 |
Diameters of lesions (mm) | 0.012 | 0.01 | 0.254 | 1.012 | 0.992–1.033 |
Orientation (Parallel) | 1.187 | 0.618 | 0.055 | 3.278 | 0.976–11.014 |
Striped hypo-echoic lines (n) | 0.097 | 0.581 | 0.867 | 1.102 | 0.353–3.443 |
Echo pattern (parallel to ductal-like structures) | 0.051 | ||||
Non–ductal-like hypo-echoic area | 1.362 | 0.799 | 0.088 | 3.905 | 0.816–18.693 |
Architectural distortion | 1.408 | 0.578 | 0.015 | 4.089 | 1.318–12.688 |
Posterior features (n) | −0.028 | 0.211 | 0.894 | 0.972 | 0.643–1.469 |
Calcification (n) | 1.01 | 0.395 | 0.011 | 2.745 | 1.265–5.956 |
Other features* (n) | 0.122 | 0.664 | 0.855 | 1.129 | 0.307–4.153 |
Adler’s classification (Level 0) | 0.003 | ||||
Level 1 | −1.517 | 0.613 | 0.013 | 0.219 | 0.066–0.73 |
Level 2 | −1.018 | 0.652 | 0.118 | 0.361 | 0.101–1.295 |
Level 3 | 0.49 | 0.706 | 0.488 | 1.633 | 0.409–6.52 |
*, other features included skin thickening, skin contraction, and edema.
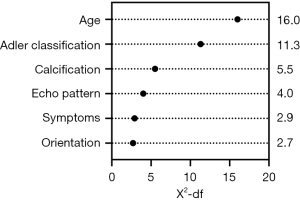
Development and internal validation of the nomogram
Figure 4A shows the static nomogram of our study. The results of the internal validation showed good calibration, with the bias-corrected C-index and AUC equaling 0.790 and 0.828, respectively (Figure 4B and Figure 5). The AUC analysis revealed the optimal cutoff value of our nomogram to be 0.366 for predicting malignant NML breast lesions, with a sensitivity, specificity, and Youden indexes of 0.704, 0.816, and 0.521, respectively (Figure 5).
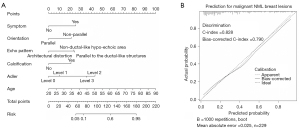
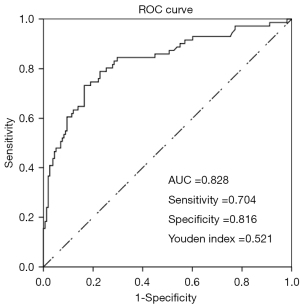
Comparison of the nomogram with BI-RADS in terms of clinical benefit
DCA analysis indicated that the nomogram had satisfactory clinical applicability in predicting malignant breast NML lesions. The nomogram was superior to the BI-RADS in our patient cohorts as evidenced by the nomogram’s higher net benefit across a wide range of threshold probabilities (0.2–0.7; Figure 6).
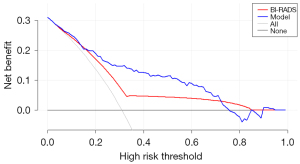
Discussion
In the present study, we developed a simplified nomogram for identifying malignant NML breast lesions, which was based on common clinical data and imaging characteristics accessed from conventional US measurements in primary screening. Nomograms have been widely used for enhancing the diagnostic efficiency of US in detecting malignant lesions, including thyroid carcinoma (15) and metastasis of sentinel lymph nodes in breast cancer (16). As far as we know, our study was the first to use a conventional US-based nomogram for predicting malignant NML breast lesions. As a pictorial representation of a complex mathematical formula that allows a graphical computation, the points at the horizontal axis in the nomogram represent the predictive values of the targeted variables (14). Clinicians could obtain a specific risk prediction for a given clinical outcome based on the total score according to a patients’ response for each variable in the nomogram. As the bias-corrected C-index and the AUC for predicting malignant NML breast lesions were both nearly 0.8 in our study, we proposed that the nomogram in the present study demonstrated satisfactory discrimination. By comparing predicted versus actual probabilities, our model also demonstrated reasonable calibration.
Several prediction models have been developed for the diagnosis of a malignant breast mass, with the BI-RADS being considered to have the best sensitivity and specificity (10). However, NML lesions are not included in the BI-RADS, and the diagnostic efficiency of the BI-RADS has not yet been validated in NML breast lesions. Furthermore, there is still room for improvement of the BI-RADS. For patients classified as BI-RADS 4, the risk of malignant breast mass was scored between 3% to 94% (10). These poor differentiating percentages were insufficient for identifying patients at high risk of developing malignant breast lesions. Additionally, patients who were classified as BI-RADS 4 but with a relatively low risk of breast cancer might have been overtreated, as a biopsy was recommended for all patients with BI-RADS 4.
In the present study, we also used DCA to compare the efficiency of the nomogram and BI-RADS in differentiating malignant and benign NML breast lesions. It has been proven that DCA can effectively overcome the limitations of discrimination and calibration, which are not directly informative to both clinical value or cost-effective analysis (17). It has also been suggested that DCA is an important supplement to AUC analysis. According to Vickers’s guideline (17), DCA calculates a clinical “net benefit” for the prediction models in comparison to the default strategies of treating all or no patients. Calculated across a range of threshold probabilities, the DCA presents the minimum probability of disease at which further intervention would be warranted. Subsequently, a clinical judgment of the relative value of benefits (treating a true-positive case) and harms (treating a false-positive case) associated with prediction models could be made (18). As suggested by the results of DCA, our nomogram was superior to the BI-RADS, with higher net benefit across a wide range of threshold probabilities.
Furthermore, most of the prediction models for malignant breast mass were developed and validated according to data from European and North American populations. The predictive efficiency of these models in Asian populations has not yet been fully evaluated. As differences of incidence and clinical manifestations in breast cancer have been found between Asian and White populations (19,20), we believed that the prediction model for malignant NML breast lesions in the Asian population of the present study was practical for the clinical scenarios.
According to the fifth edition of the BI-RADS, the US characteristics of shape, orientation, margin, echo pattern, posterior features, and calcifications should be employed for breast mass assessment (1). Our predictive model incorporated most of the US characteristics reported in the BI-RADS except for shape and margin, as both benign and malignant lesions have irregular shapes and non-circumscribed margins. Additionally, other features, such as vascularity, diameter, location, and number of lesions, which are reported to be predictive of malignant breast lesions, were included in the multivariable logistic regression analysis (21). In order to obtain the largest power of discrimination and calibration, clinical characteristics readily available for radiologists during US screening, such as age and clinical symptoms, were also collected.
Six variables, including age, clinical symptoms, echo pattern, calcification, orientation, and flow signals graded by Adler’s classification, were finally enrolled in the prediction model according to the results of multivariable logistic regression analysis. Although age showed the largest impact on the present model, we admit that the relationship between age and malignant breast cancer remains conflicting (22). Nevertheless, a recently published biomarker expression analysis revealed that age is a risk factor for breast cancer (23). It might be that lesions representing NML are more likely to be fibrocystic breast disease in younger people; however, further investigation is needed. A meta-analysis study showed that clinical symptoms, such as bloody discharge, were well-documented risk factors for breast cancer (24), which was consistent with our study.
In terms of US characteristics, our results showed that flow signals graded by Adler’s classification and calcification was the feature with most influence on the prediction of malignant NML breast lesions. By providing more detailed information about the flow signal of breast masses, Adler’s classification presented satisfied diagnostic efficiency for differentiating malignant breast neoplasms in previous studies (9). Although a higher grade of Adler’s classification was associated with a higher risk of malignant breast lesion (25), our study found that an Adler’s level 2 classification was more predictive than a level 3 classification. As chronic mastitis with a marked flow signal accounted for nearly one-fifth (17.9%) of all of the breast lesions in our study, we supposed that this might be a reasonable explanation for the inconsistent result between the previous study and ours. Calcification is also a well-documented risk factor for breast cancer (26). It has been reported that malignant breast lesions with calcifications are closely related with decreased survival, increased risk of recurrence, higher tumor grade, and increased likelihood of spread to the lymph nodes (27). As for echo patterns, the present study found that the NML breast lesions appeared parallel to the ductal-like structures and had a larger risk of malignancy. Consistent with this, a previous study also found a significantly higher incidence of malignancy in this type of echo pattern compared with other echo patterns (28). Our model is similar to the BI-RADS in those lesions with a nonparallel orientation showed significantly higher risks of malignancy. It has been proven that invasive cancers present more frequently with a nonparallel orientation (29).
Our study was subject to a few limitations. Firstly, a large-scale sample is needed in a future study for developing nomograms with higher discrimination and calibration although the sample size of the current study met statistical requirements. Secondly, selection bias might have been present due to the retrospective, single-center design of the present study. Furthermore, age was selected as the candidate predictor for our prediction model, but the interaction effect of this variable on the main outcome remains unknown, and further analysis is recommended according to Iasonos’s guideline (11). However, as the VIF of age is 1.044, we propose that the potential interaction effects of age might have been limited in our study. Moreover, the presence of lymph node involvement, family history, and history of known breast benign disease are risk factors for breast cancer. However, we were unable to obtain these data from all outpatients. As the building of the nomogram could not tolerate missing data of the enrolled variable, we did not include these variables in the current study, and the rate of malignancy in the selected patients was relatively high. Our department is one of the top 5 medical imaging centers in Guangdong and adjacent provinces. Patients primarily diagnosed with malignant NML breast lesions in the local hospital tend to be transferred to our center for further consultations and interventions. This might be a potential cause of the relatively higher rate of malignancy documented in our study. Nevertheless, our results (31.0%) are comparable and even lower to those of previous reports [53.8% in Wang et al. (4) and 59% in Qu et al. (2)]. We do, however, insist that external validation is strongly needed before the widespread application of this nomogram, especially for health screening. Lastly, the dependence of US performance on the operator should not be overlooked in this study.
Conclusions
We developed a prediction model using a combination of age, clinical symptoms, echo pattern, calcification, orientation, and Adler’s classification for the prediction of malignant NML breast lesions, and the model showed adequate discrimination and calibration. This model demonstrated an advantage in clinical utility compared with the BI-RADS. We believe that our prediction model could be a valuable reference for radiologists during primary NML breast lesion screening.
Acknowledgments
We sincerely thank Ms. Yunlian Xue, an experienced statistician, for her valuable advice on the building and validation of the nomogram.
Funding: This work was supported by the Department Development Foundation of Guangdong Province Hospital of Chinese Medicine (No. 2017-01).
Footnote
Reporting Checklist: The authors have completed the STARD reporting checklist. Available at https://qims.amegroups.com/article/view/10.21037/qims-22-378/rc
Conflicts of Interest: All authors have completed the ICMJE uniform disclosure form (available at https://qims.amegroups.com/article/view/10.21037/qims-22-378/coif). The authors have no conflicts of interest to declare.
Ethical Statement: The authors are accountable for all aspects of the work in ensuring that questions related to the accuracy or integrity of any part of the work are appropriately investigated and resolved. The present study was conducted in accordance with the Declaration of Helsinki (as revised in 2013). The study design was approved by the institutional review board of Guangdong Province Hospital of Chinese Medicine, and individual consent for this retrospective analysis was waived.
Open Access Statement: This is an Open Access article distributed in accordance with the Creative Commons Attribution-NonCommercial-NoDerivs 4.0 International License (CC BY-NC-ND 4.0), which permits the non-commercial replication and distribution of the article with the strict proviso that no changes or edits are made and the original work is properly cited (including links to both the formal publication through the relevant DOI and the license). See: https://creativecommons.org/licenses/by-nc-nd/4.0/.
References
- Rao AA, Feneis J, Lalonde C, Ojeda-Fournier H. A Pictorial Review of Changes in the BI-RADS Fifth Edition. Radiographics 2016;36:623-39.
- Qu XX, Song Y, Zhang YH, Qing HM. Value of Ultrasonic Elastography and Conventional Ultrasonography in the Differential Diagnosis of Non-Mass-like Breast Lesions. Ultrasound Med Biol 2019;45:1358-66. [Crossref] [PubMed]
- Yang Y, Hu Y, Shen S, Jiang X, Gu R, Wang H, Liu F, Mei J, Liang J, Jia H, Liu Q, Gong C. A new nomogram for predicting the malignant diagnosis of Breast Imaging Reporting and Data System (BI-RADS) ultrasonography category 4A lesions in women with dense breast tissue in the diagnostic setting. Quant Imaging Med Surg 2021;11:3005-17. [Crossref] [PubMed]
- Wang ZL, Li N, Li M, Wan WB. Non-mass-like lesions on breast ultrasound: classification and correlation with histology. Radiol Med 2015;120:905-10. [Crossref] [PubMed]
- Girometti R, Zanotel M, Londero V, Linda A, Lorenzon M, Zuiani C. Automated breast volume scanner (ABVS) in assessing breast cancer size: A comparison with conventional ultrasound and magnetic resonance imaging. Eur Radiol 2018;28:1000-8. [Crossref] [PubMed]
- Xu P, Yang M, Liu Y, Li YP, Zhang H, Shao GR. Breast non-mass-like lesions on contrast-enhanced ultrasonography: Feature analysis, breast image reporting and data system classification assessment. World J Clin Cases 2020;8:700-12. [Crossref] [PubMed]
- Xu W, Zheng B, Chen W, Wen C, Zeng H, He Z, Qin G, Li Y. Can the delayed phase of quantitative contrast-enhanced mammography improve the diagnostic performance on breast masses? Quant Imaging Med Surg 2021;11:3684-97. [Crossref] [PubMed]
- Bossuyt PM, Reitsma JB, Bruns DE, Gatsonis CA, Glasziou PP, Irwig L, Lijmer JG, Moher D, Rennie D, de Vet HC, Kressel HY, Rifai N, Golub RM, Altman DG, Hooft L, Korevaar DA, Cohen JF. STARD Group. STARD 2015: an updated list of essential items for reporting diagnostic accuracy studies. BMJ 2015;351:h5527. [PubMed]
- Zhu YC, Zhang Y, Deng SH, Jiang Q. Diagnostic Performance of Superb Microvascular Imaging (SMI) Combined with Shear-Wave Elastography in Evaluating Breast Lesions. Med Sci Monit 2018;24:5935-42. [Crossref] [PubMed]
- Spak DA, Plaxco JS, Santiago L, Dryden MJ, Dogan BE. BI-RADS® fifth edition: A summary of changes. Diagn Interv Imaging 2017;98:179-90.
- Iasonos A, Schrag D, Raj GV, Panageas KS. How to build and interpret a nomogram for cancer prognosis. J Clin Oncol 2008;26:1364-70. [Crossref] [PubMed]
- Fu G, Li M, Xue Y, Li Q, Deng Z, Ma Y, Zheng Q. Perioperative patient-specific factors-based nomograms predict short-term periprosthetic bone loss after total hip arthroplasty. J Orthop Surg Res 2020;15:503. [Crossref] [PubMed]
- Fu G, Li M, Xue Y, Wang H, Zhang R, Ma Y, Zheng Q. Rapid preoperative predicting tools for 1-year mortality and walking ability of Asian elderly femoral neck fracture patients who planned for hip arthroplasty. J Orthop Surg Res 2021;16:455. [Crossref] [PubMed]
- Wu R, Ma Y, Yang Y, Li M, Zheng Q, Fu G. A clinical model for predicting knee replacement in early-stage knee osteoarthritis: data from osteoarthritis initiative. Clin Rheumatol 2022;41:1199-210. [Crossref] [PubMed]
- Chen L, Zhang J, Meng L, Lai Y, Huang W. A new ultrasound nomogram for differentiating benign and malignant thyroid nodules. Clin Endocrinol (Oxf) 2019;90:351-9. [Crossref] [PubMed]
- Bae SJ, Youk JH, Yoon CI, Park S, Cha CH, Lee HW, Ahn SG, Lee SA, Son EJ, Jeong J. A nomogram constructed using intraoperative ex vivo shear-wave elastography precisely predicts metastasis of sentinel lymph nodes in breast cancer. Eur Radiol 2020;30:789-97. [Crossref] [PubMed]
- Vickers AJ, van Calster B, Steyerberg EW. A simple, step-by-step guide to interpreting decision curve analysis. Diagn Progn Res 2019;3:18. [Crossref] [PubMed]
- Zhang Z, Rousson V, Lee WC, Ferdynus C, Chen M, Qian X, Guo Y. written on behalf of AME Big-Data Clinical Trial Collaborative Group. Decision curve analysis: a technical note. Ann Transl Med 2018;6:308. [Crossref] [PubMed]
- Evans DG, Brentnall AR, Harvie M, Astley S, Harkness EF, Stavrinos P, Donnelly LS, Sampson S, Idries F, Watterson D, Cuzick J, Wilson M, Jain A, Harrison F, Maxwell AJ, Howell A. Breast cancer risk in a screening cohort of Asian and white British/Irish women from Manchester UK. BMC Public Health 2018;18:178. [Crossref] [PubMed]
- Pan JW, Zabidi MMA, Ng PS, Meng MY, Hasan SN, Sandey B, Sammut SJ, Yip CH, Rajadurai P, Rueda OM, Caldas C, Chin SF, Teo SH. The molecular landscape of Asian breast cancers reveals clinically relevant population-specific differences. Nat Commun 2020;11:6433. [Crossref] [PubMed]
- Lee EJ, Chang YW. Combination of Quantitative Parameters of Shear Wave Elastography and Superb Microvascular Imaging to Evaluate Breast Masses. Korean J Radiol 2020;21:1045-54. [Crossref] [PubMed]
- Coughlin SS. Epidemiology of Breast Cancer in Women. Adv Exp Med Biol 2019;1152:9-29. [Crossref] [PubMed]
- Ma X, Liu C, Xu X, Liu L, Gao C, Zhuang J, Li H, Feng F, Zhou C, Liu Z, Li J, Wei J, Wang L, Sun C. Biomarker expression analysis in different age groups revealed age was a risk factor for breast cancer. J Cell Physiol 2020;235:4268-78. [Crossref] [PubMed]
- Chen L, Zhou WB, Zhao Y, Liu XA, Ding Q, Zha XM, Wang S. Bloody nipple discharge is a predictor of breast cancer risk: a meta-analysis. Breast Cancer Res Treat 2012;132:9-14. [Crossref] [PubMed]
- Adler DD, Carson PL, Rubin JM, Quinn-Reid D. Doppler ultrasound color flow imaging in the study of breast cancer: preliminary findings. Ultrasound Med Biol 1990;16:553-9. [Crossref] [PubMed]
- Li Y, Cao J, Zhou Y, Mao F, Shen S, Sun Q. Mammographic casting-type calcification is an independent prognostic factor in invasive breast cancer. Sci Rep 2019;9:10544. [Crossref] [PubMed]
- O'Grady S, Morgan MP. Microcalcifications in breast cancer: From pathophysiology to diagnosis and prognosis. Biochim Biophys Acta Rev Cancer 2018;1869:310-20. [Crossref] [PubMed]
- Ko KH, Hsu HH, Yu JC, Peng YJ, Tung HJ, Chu CM, Chang TH, Chang WC, Wu YC, Lin YP, Hsu GC. Non-mass-like breast lesions at ultrasonography: feature analysis and BI-RADS assessment. Eur J Radiol 2015;84:77-85. [Crossref] [PubMed]
- Kim SH, Seo BK, Lee J, Kim SJ, Cho KR, Lee KY, Je BK, Kim HY, Kim YS, Lee JH. Correlation of ultrasound findings with histology, tumor grade, and biological markers in breast cancer. Acta Oncol 2008;47:1531-8. [Crossref] [PubMed]