Quantitative chest CT assessment of pulmonary alveolar proteinosis with deep learning: a real-world longitudinal study
Introduction
Pulmonary alveolar proteinosis (PAP) is a rare disorder characterized by the accumulation of surfactant lipids and proteins and periodic acid-Schiff (PAS)-positive materials in endoalveolar space (1). The radiologic features characteristic of PAP on high-resolution computed tomography (HRCT) are the presence of a diffuse, patchy, “geographic” pattern of ground-glass opacification superimposed on interlobular septal thickening in multiple lobes (2). It is common practice for radiologists to evaluate the extent of lung involvement according to the visual scoring methods proposed by Lee et al. (3) and eventually predict the therapeutic effects before and after the treatment for PAP. However, visual evaluation of changes between two HRCT scans is subjective, and its validity may depend on the radiologists’ experience. Therefore, developing a tool that can provide an automatic and objective estimation of the disease burden of PAP is urgently needed.
Reports have shown that quantitative analysis of the HRCT scans can be used in lung diseases, including idiopathic pulmonary fibrosis and the 2019 coronavirus disease (COVID-19) (4,5). Oral statin therapy, which reduces cholesterol accumulation and ameliorates PAP, emerged in 2018 as a novel pharmacotherapy for PAP (6). However, whether the quantitative analysis of the HRCT scans can evaluate the severity of PAP and predict the response to statin therapy is unclear. Thus, in the present study, we assessed quantitative parameters, including the percentage of ground-glass opacity distribution and that of different Hounsfield unit (HU) values of ground-glass opacities before and after statin therapy. We present the following article in accordance with the STROBE reporting checklist (available at https://qims.amegroups.com/article/view/10.21037/qims-22-205/rc).
Methods
Study population
In a prospective, real-world observational study, patients with PAP aged between 18 and 70 years were enrolled at the Affiliated Drum Tower Hospital of Nanjing University Medical School in China. Patients were included if they had a HRCT-based diagnosis of PAP that was further pathologically confirmed by testing for amorphous PAS-positive granules. Patients were ineligible if they were pregnant or breastfeeding, had chronic lung diseases or any other serious medical conditions, or had been treated with whole lung lavage (WLL) or granulocyte-macrophage colony-stimulating factor (GM-CSF). The study was conducted according to the Declaration of Helsinki (as revised in 2013) and approved by the Ethics Committee of Nanjing Drum Tower Hospital, the Affiliated Hospital of Nanjing University Medical School. All patients gave informed written consent to participate.
Demographic and clinical data
The period of recruitment and follow-up was from November 28, 2018, to April 12, 2021, after the last enrolled patient completed the 12-month follow-up. All baseline and follow-up clinical data, including demographic information, symptoms, and a full blood examination—consisting of plasma lipids, serum lactate dehydrogenase (LDH), carcinoembryonic antigen (CEA), and cytokeratin 21-1 (CYFRA 21-1) tests—were collected. The GM-CSF antibody test was performed according to the method established by Uchida et al. (7,8). According to the literature, the critical threshold of serum GM-CSF antibody was set at 2.39 g/mL (9). The pulmonary function tests (PFTs) were conducted at the initial diagnosis of PAP and at the 12-month follow-up. The percentage of predicted forced vital capacity (FVC), the percentage of predicted forced expiratory volume in 1 second (FEV1), and the percentage of predicted diffusion capacity for carbon monoxide (DLCO) were included in the analysis.
Each patient was assigned a disease severity score (DSS) based on the presence or absence of symptoms and the degree of partial pressure of arterial oxygen (PaO2) at the initial diagnosis of PAP. The grades ranged from grade 1 to grade 5: grade 1, PaO2 ≥70 mmHg without respiratory symptoms; grade 2, PaO2 ≥70 mmHg with respiratory symptoms; grade 3, 70 mmHg > PaO2 ≥60 mmHg; grade 4, 60 mmHg ≥ PaO2 ≥50 mmHg; and grade 5, PaO2 <50 mmHg. PAP patients were further divided into 2 groups: the mild-moderate PAP group [patients with lower DSS (DSS 1–2)] and the severe PAP group [patients with higher DSS (DSS 3–5)].
HRCT image analysis
Chest HRCT examination was performed up to 3 days before or after the initial diagnosis of PAP. Both CT scans were completed with the same scanner under the following parameters: tube voltage 120 kV and tube current 350 mAs. Reconstruction was performed with a slice thickness of 1.00–1.25 mm, a lung window with a width of 1,500 HU, and a level of −600 HU. The quantitative analysis of lung opacification was performed by a deep-learning algorithm. The algorithm consisted of 3 modules: the lung and lobes segmentation module, the lung opacity segmentation module, and the quantitative analysis module. This deep-learning algorithm used a well-established fully convolutional neural network architecture (10) and was trained on annotated data sets of PAP. All segmentation results derived from this deep-learning algorithm were visually evaluated by 2 radiologists (one with 5 years of experience in pulmonary imaging and the other with 10 years of experience in pulmonary imaging) who viewed the segmentation independently. Therefore, in this study, all HRCT images were uploaded and processed on the deep-learning algorithm and were eventually evaluated. with respect to the following: the percentage of lung ground-glass opacification within the entire lung volume (total opacification percentage of the whole lung, opacification percentage of the right upper lobe, opacification percentage of the right middle lobe, opacification percentage of the right lower lobe, opacification percentage of the left upper lobe, opacification percentage of the left lower lobe) and the percentage of different densities of ground glass (between −∞ and −750 HU, −750 and −300 HU, between −300 and 49 HU, and 50+ HU).
Statistical analysis
Continuous variables were tested for normal distribution by the Kolmogorov-Smirnov test. Continuous variables with a normal distribution are expressed as the mean ± SD or as median and interquartile range. Differences between 2 groups were analyzed using a t-test or the Mann-Whitney test. Categorical variables are expressed as percentages and were compared with the chi-squared test. The relationship between changes in total opacification of the whole lung and changes in PaO2, FVC%pred, FEV1%pred, and DLCO%pred was assessed using Pearson correlation analysis with GraphPad Prism (GraphPad Software Inc., La Jolla, CA, USA). Data were analyzed using SPSS 26.0 (IBM Corp, Armonk, NY, USA). P<0.05 (2-sided) was considered to indicate statistical significance.
Results
Baseline characteristics of the study participants
A total of 103 PAP patients who were diagnosed by transbronchial lung biopsy, clinical manifestations, and chest images from November 28, 2018, to April 12, 2021, were screened. Finally, a total of 50 patients with PAP were included. Figure 1 presents the flow diagram of the study population. Patients with PAP were further divided into 2 groups according to the DSS value: the mild-moderate PAP group (n=25) and the severe PAP group (n=25). Baseline clinical characteristics and comparisons of 50 PAP patients are summarized in Table 1. The average ages, gender, duration of the disease, and smoking history were similar. Progressive dyspnea and cough were more common in the severe PAP group (P=0.007 and P=0.015, respectively). Mean serum anti-GM-CSF antibody, LDH, and CYF21-1 levels also differed between the 2 groups (P=0.040, P=0.001, and P=0.002, respectively). In addition, lower levels of PaO2, alveolar-arterial oxygen gradient (A-aDO2), FVC%pred, FEV1%pred, and DLCO%pred were observed in patients with severe PAP (all P values <0.001).
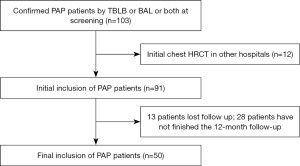
Table 1
Variables | Mild-moderate PAP group (n=25) | Severe PAP group (n=25) | P value |
---|---|---|---|
Age (years) | 48.60±12.09 | 47.32±12.68 | 0.717 |
Sex (female), n (%) | 11 (44.0) | 10 (40.0) | 0.774 |
Duration of the disease (months) | 6.0 (1.8, 12.0) | 6.0 (2.5, 36.0) | 0.197 |
Smoking history (yes), n (%) | 8 (32.0) | 9 (36.0) | 0.765 |
Symptoms, n (%) | |||
Asymptomatic | 6 (24.0) | 0 (0.0) | 0.030 |
Progressive dyspnea | 17 (68.0) | 25 (100.0) | 0.007 |
Cough | 13 (52.0) | 21 (84.0) | 0.015 |
Chest pain | 1 (4.0) | 0 (0.0) | 1.000 |
Laboratory findings | |||
Anti-GM-CSF antibody (μg/mL) | 29.52 (17.09, 44.03) | 10.66 (6.37, 25.73) | 0.040 |
PaO2 (mmHg) | 78.96±6.27 | 61.10±5.82 | <0.001 |
A-aDO2 (mmHg) | 23.33±8.15 | 43.96±7.81 | <0.001 |
LDH (U/L) | 218.44±35.84 | 300.72±99.72 | 0.001 |
CEA (ng/mL) | 2.81±2.61 | 5.00±4.91 | 0.054 |
CYF21-1 (ng/mL) | 5.74±3.50 | 12.13±8.82 | 0.002 |
TC (mmol/L) | 4.70±1.56 | 4.47±0.91 | 0.521 |
TG (mmol/L) | 2.13±1.69 | 1.57±0.78 | 0.140 |
HDL (mmol/L) | 1.21±0.43 | 1.17±0.43 | 0.739 |
LDL (mmol/L) | 2.79±1.17 | 2.71±0.72 | 0.767 |
TC/HDL | 4.13±1.31 | 4.19±1.36 | 0.880 |
PFT | |||
FVC%pred | 86.36±13.98 | 70.61±15.21 | <0.001 |
FEV1%pred | 89.28±12.99 | 71.48±16.89 | <0.001 |
DLCO%pred | 73.39±17.69 | 50.38±17.42 | <0.001 |
Continuous variables with a normal distribution are expressed as the mean ± SD or as median and interquartile range. PAP, pulmonary alveolar proteinosis; GM-CSF, granulocyte-macrophage colony-stimulating factor; PaO2, partial pressure of arterial oxygen; A-aDO2, alveolar-arterial oxygen gradient; LDH, lactate dehydrogenase; CEA, carcinoembryonic antigen; CYF21-1, cytokeratin 21-1; TC, total cholesterol; TG, triglycerides; HDL, high density lipoprotein; LDL, low density lipoprotein; PFT, pulmonary function test; FVC%pred, percentage of forced vital capacity predicted; FEV1%pred, percentage of forced expiratory volume in 1 second predicted; DLCO%pred, percentage of predicted diffusion capacity for carbon monoxide.
Significant differences of lung ground-glass opacification percentage of the whole lung and 5 lobes and the percentage of different densities of ground glass were found between the 2 different clinical types at the baseline (all P values <0.05; Table 2).
Table 2
Parameters | Mild-moderate PAP group (n=25) | Severe PAP group (n=25) | P value |
---|---|---|---|
Total opacification percentage of the whole lung (%) | 12.20 (5.75, 25.60) | 44.00 (23.80, 64.45) | <0.001 |
Opacification percentage of the right upper lobe (%) | 14.10 (5.10, 39.50) | 47.00 (20.55, 66.30) | 0.002 |
Opacification percentage of the right middle lobe (%) | 15.40 (4.00, 25.45) | 40.20 (26.15, 60.10) | <0.001 |
Opacification percentage of the right lower lobe (%) | 12.30 (3.65, 22.65) | 41.20 (22.05, 70.00) | <0.001 |
Opacification percentage of the left upper lobe (%) | 14.30 (4.90, 35.65) | 40.00 (23.35, 59.75) | 0.001 |
Opacification percentage of the left lower lobe (%) | 10.90 (1.40, 19.45) | 43.10 (16.75, 63.80) | <0.001 |
Percentage of HU value of (−∞, −750] (%) | 2.40 (1.20, 6.00) | 5.20 (3.00, 10.65) | 0.030 |
Percentage of HU value of (−750, −300] (%) | 6.40 (3.40, 11.90) | 23.10 (14.35, 32.65) | <0.001 |
Percentage of HU value of (−300, 50] (%) | 1.50 (0.85, 3.30) | 7.70 (3.10, 14.40) | 0.004 |
Percentage of HU value of 50+ (%) | 0.40 (0.10, 0.75) | 1.20 (0.60, 3.95) | 0.016 |
Data are presented as median and interquartile range. CT, computed tomography; PAP, pulmonary alveolar proteinosis; HU, Hounsfield unit.
Quantitative HRCT parameters at baseline and 12-month follow-up HRCT scans
Regardless of the specific form of PAP, oral atorvastatin was initiated as therapy for these enrolled PAP patients. A comparison of the quantitative HRCT analysis before and after 12 months of oral statin treatment is shown in Table 3. Overall, the percentage of whole-lung ground-glass opacification significantly decreased between the baseline HRCT and the 12-month follow-up HRCT (30.15±22.82 vs. 20.11±20.72; P=0.023; 95% CI: 1.384–18.684). As shown in Table 3, the opacification percentage of the right middle lobe and the left upper lobe decreased more frequently than in other lung lobes. In terms of changes in the density of ground glass, the percentage of HU value of (−300, 50] and the percentage of HU value of 50+ significantly decreased (P=0.013 and P=0.019, respectively).
Table 3
Parameters | At baseline | 12 months follow-up | P value |
---|---|---|---|
Total opacification percentage of the whole lung (%) | 24.25 (8.63, 48.68) | 13.55 (4.70, 27.88) | 0.023 |
Opacification percentage of the right upper lobe (%) | 24.85 (11.33, 59.73) | 17.95 (5.35, 42.60) | 0.094 |
Opacification percentage of the right middle lobe (%) | 25.55 (5.58, 48.35) | 10.95 (1.70, 35.40) | 0.018 |
Opacification percentage of the right lower lobe (%) | 22.65 (5.65, 47.38) | 10.70 (2.85, 28.33) | 0.101 |
Opacification percentage of the left upper lobe (%) | 27.50 (8.58, 48.43) | 16.50 (3.60, 35.68) | 0.040 |
Opacification percentage of the left lower lobe (%) | 18.75 (4.08, 51.55) | 7.55 (1.23, 31.68) | 0.079 |
Percentage of HU value of (−∞, −750] (%) | 4.25 (1.68, 7.95) | 3.35 (1.13, 7.33) | 0.454 |
Percentage of HU value of (−750, −300] (%) | 13.10 (5.83, 27.30) | 7.60 (2.38, 16.43) | 0.110 |
Percentage of HU value of (−300, 50] (%) | 3.10 (1.20, 9.90) | 1.15 (0.40, 3.83) | 0.013 |
Percentage of HU value of 50+ (%) | 0.70 (0.30, 2.10) | 0.20 (0.10, 0.68) | 0.019 |
Data are presented as median and interquartile range. CT, computed tomography; PAP, pulmonary alveolar proteinosis; HU, Hounsfield unit.
We further defined the increased PaO2, FVC%pred, FEV1%pred, and DLCO%pred from the baseline to 12 months of statin treatment as ΔPaO2, ΔFVC%pred, ΔFEV1%pred, and ΔDLCO%pred. Δtotal opacification of the whole lung (%) was measured by the decreased total opacification percentage of the whole lung from the baseline to the follow-up after 12 months of statin treatment. Correlation analyses were performed between Δtotal opacification of the whole lung (%) and ΔPaO2, ΔFVC%pred, ΔFEV1%pred, and ΔDLCO%pred in the 50 patients with PAP. As shown in Figure 2, Δtotal opacification of the whole lung (%; decreased total opacification percentage of whole lung from baseline to 12 months of statin treatment) was positively correlated with ΔPaO2 (increased PaO2) and ΔDLCO%pred (increased DLCO%pred) level (ΔPaO2: r=0.716, P<0.001; ΔDLCO%pred: r=0.664, P<0.001). However, no association was observed between Δtotal opacification of the whole lung (%) and ΔFVC%pred (increased FVC%pred; P=0.064) or ΔFEV1%pred (increased FEV1%pred; P=0.290).
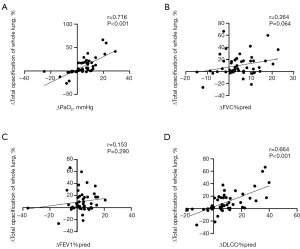
Quantitative HRCT opacification parameters in different clinical PAP patients
Compared to the baseline HRCT scan, the percentage of the HU value at 50+ decreased significantly in mild-moderate patients (P=0.034), while no remarkable difference was found in the percentage of whole-lung ground-glass opacification (Table 4). In severe patients, the opacification percentage of the whole lung, the right middle lobe, and the left upper lobe showed significant differences between the baseline and 12-month follow-up (all P values <0.05; Figure 3; Table 5).
Table 4
Parameters | Baseline CT | 12 months follow-up CT | P value |
---|---|---|---|
Total opacification percentage of the whole lung (%) | 12.20 (5.75, 25.60) | 10.65 (4.93, 19.70) | 0.197 |
Opacification percentage of the right upper lobe (%) | 14.10 (5.10, 39.50) | 11.90 (4.20, 21.55) | 0.208 |
Opacification percentage of the right middle lobe (%) | 15.40 (4.00, 25.45) | 10.95 (1.70, 24.20) | 0.304 |
Opacification percentage of the right lower lobe (%) | 12.30 (3.65, 22.65) | 10.45 (3.08, 19.65) | 0.247 |
Opacification percentage of the left upper lobe (%) | 14.30 (4.90, 35.65) | 11.90 (4.40, 27.73) | 0.325 |
Opacification percentage of the left lower lobe (%) | 10.90 (1.40, 19.45) | 6.90 (0.80, 16.48) | 0.373 |
Percentage of HU value of (−∞, −750] (%) | 2.40 (1.20, 6.00) | 2.20 (1.13, 6.40) | 0.749 |
Percentage of HU value of (−750, −300] (%) | 6.40 (3.40, 11.90) | 6.35 (2.65, 10.18) | 0.370 |
Percentage of HU value of (−300, 50] (%) | 1.50 (0.85, 3.30) | 1.10 (0.50, 2.55) | 0.074 |
Percentage of HU value of 50+ (%) | 0.40 (0.10, 0.75) | 0.25 (0.10, 0.53) | 0.034 |
Data are presented as median and interquartile range. CT, computed tomography; PAP, pulmonary alveolar proteinosis; HU, Hounsfield unit.
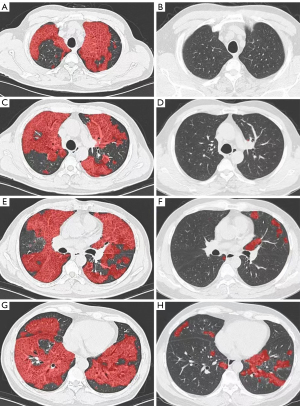
Table 5
Parameters | Baseline CT | 12 months follow-up CT | P value |
---|---|---|---|
Total opacification percentage of the whole lung (%) | 44.00 (23.80, 64.45) | 19.90 (5.35, 49.95) | 0.030 |
Opacification percentage of the right upper lobe (%) | 44.84±24.60 | 35.27±25.73 | 0.185 |
Opacification percentage of the right middle lobe (%) | 40.20 (26.15, 60.10) | 17.90 (2.30, 44.60) | 0.016 |
Opacification percentage of the right lower lobe (%) | 41.20 (22.05, 70.00) | 21.00 (5.30, 63.45) | 0.141 |
Opacification percentage of the left upper lobe (%) | 40.00 (23.35, 59.75) | 22.30 (5.00, 47.45) | 0.046 |
Opacification percentage of the left lower lobe (%) | 43.10 (16.75, 63.80) | 16.50 (1.50, 62.05) | 0.082 |
Percentage of HU value of (−∞, −750] (%) | 5.20 (3.00, 10.65) | 4.60 (1.60, 9.75) | 0.476 |
Percentage of HU value of (−750, −300] (%) | 23.10 (14.35, 32.65) | 10.10 (3.00, 32.25) | 0.124 |
Percentage of HU value of (−300, 50] (%) | 7.70 (3.10, 14.40) | 2.40 (0.65, 7.20) | 0.045 |
Percentage of HU value of 50+ (%) | 1.20 (0.60, 3.95) | 0.40 (0.15, 1.80) | 0.068 |
Data are presented as mean ± SD or median and interquartile range. CT, computed tomography; PAP, pulmonary alveolar proteinosis; HU, Hounsfield unit.
Discussion
In this study, we evaluated the longitudinal changes of severity in different clinical types of PAP before and after oral statin therapy. We used a quantitative imaging parameter that was automatically generated by a deep-learning tool from chest HRCT scans. Quantitative HRCT assessment provides automatic segmentation and almost instant results based on shadow distribution; therefore, it may be a more reliable way to evaluate the severity of PAP than a visual assessment by radiologists or respiratory physicians, reducing the influence of subjectivity. In this study, we found that (I) quantitative HRCT assessment could identify differences in the lung opacity burden from the different clinical severity of PAP; (II) the opacification percentage of the whole lung, right middle lobe, and left upper lobe significantly decreased after the 12 months of statin therapy; and (III) changes of total opacification of the whole lung were positively correlated with changes in PaO2 and DLCO%pred.
Patients in the severe PAP group had obvious hypoxemia with a lower level of PaO2 and A-aDO2, which has been described as a strong indicator of PAP severity and used as a main endpoint in several clinical trials (11,12). Patients with severe PAP also had worse pulmonary function, with the majority showing symptoms of cough and progressive dyspnea. Moreover, a higher level of lung opacification percentage in baseline HRCT was found in the severe PAP group compared with the mild-moderate PAP group. The opacification percentage of the whole lung, right middle lobe, and left upper lobe significantly decreased after 12 months of statin therapy. Previous studies reported that the opacification percentage is correlated significantly with the presence of a restrictive ventilator defect, reduced diffusing capacity, and hypoxemia (3,13). In a study by Tokura et al. (14), quantitative CT assessment of the extent and degree of opacification in CT scans (CT grading score) was identified to be valuable in assessing the therapeutic response of inhaled GM-CSF therapy among autoimmune PAP patients. The results of this study suggest that HRCT quantitative analysis could be used in the initial severity assessment of PAP and may predict the response to statin treatment of PAP. Moreover, several serum markers, including LDH and CYF21-1 levels, also differed between mild-moderate and severe types of PAP, which is consistent with findings of a previous study (15).
Moreover, changes in the quantitative HRCT analysis and DLCO%pred before and after statin therapy also appeared to be correlated. Classification of the severity of PAP is usually based on PFT results, such as DLCO (16). However, a PFT has high operating requirements from the doctor and demands close cooperation from the patient. In the future, the effectiveness of oral statin therapy for PAP may be monitored through detecting the quantitative image parameters in HRCT.
Some limitations of this study should be noted. First, we employed a prospective, longitudinal and observational design that lacked the comparison of a placebo-controlled study. Second, our sample size was small. However, considering the low frequency of PAP in the general population, the sample size in our study can be considered acceptable. Finally, all patients were recruited from Nanjing in the Jiangsu province of China. Therefore, it is uncertain whether these results are generalizable to other ethnic groups.
Conclusions
Applying deep-learning tools to quantitative HRCT can objectively and stably assess the severity of PAP and thus may possibly be used to evaluate the efficacy of oral statin therapy in treating PAP.
Acknowledgments
Funding: This study received financial support from the National Nature Science Foundation of China (No. 81570061), the National Key Research and Development Program of China (No. 2016YFC0901502), and the Chinese Academy of Medical Sciences (CAMS) Initiative for Innovative Medicine (No. 2017-12 M-2-001).
Footnote
Reporting Checklist: The authors have completed the STROBE reporting checklist. Available at https://qims.amegroups.com/article/view/10.21037/qims-22-205/rc
Conflicts of Interest: All authors have completed the ICMJE uniform disclosure form (available at https://qims.amegroups.com/article/view/10.21037/qims-22-205/coif). The authors have no conflicts of interest to declare.
Ethical Statement: The authors are accountable for all aspects of the work in ensuring that questions related to the accuracy or integrity of any part of the work are appropriately investigated and resolved. The study was conducted according to the Declaration of Helsinki (as revised in 2013) and approved by the Ethics Committee of Nanjing Drum Tower Hospital, the Affiliated Hospital of Nanjing University Medical School. All subjects gave informed written consent to participate.
Open Access Statement: This is an Open Access article distributed in accordance with the Creative Commons Attribution-NonCommercial-NoDerivs 4.0 International License (CC BY-NC-ND 4.0), which permits the non-commercial replication and distribution of the article with the strict proviso that no changes or edits are made and the original work is properly cited (including links to both the formal publication through the relevant DOI and the license). See: https://creativecommons.org/licenses/by-nc-nd/4.0/.
References
- Trapnell BC, Whitsett JA, Nakata K. Pulmonary alveolar proteinosis. N Engl J Med 2003;349:2527-39. [Crossref] [PubMed]
- Holbert JM, Costello P, Li W, Hoffman RM, Rogers RM. CT features of pulmonary alveolar proteinosis. AJR Am J Roentgenol 2001;176:1287-94. [Crossref] [PubMed]
- Lee KN, Levin DL, Webb WR, Chen D, Storto ML, Golden JA. Pulmonary alveolar proteinosis: high-resolution CT, chest radiographic, and functional correlations. Chest 1997;111:989-95. [Crossref] [PubMed]
- Best AC, Lynch AM, Bozic CM, Miller D, Grunwald GK, Lynch DA. Quantitative CT indexes in idiopathic pulmonary fibrosis: relationship with physiologic impairment. Radiology 2003;228:407-14. [Crossref] [PubMed]
- Huang L, Han R, Ai T, Yu P, Kang H, Tao Q, Xia L. Serial Quantitative Chest CT Assessment of COVID-19: A Deep Learning Approach. Radiol Cardiothorac Imaging 2020;2:e200075. [Crossref] [PubMed]
- McCarthy C, Lee E, Bridges JP, Sallese A, Suzuki T, Woods JC, Bartholmai BJ, Wang T, Chalk C, Carey BC, Arumugam P, Shima K, Tarling EJ, Trapnell BC. Statin as a novel pharmacotherapy of pulmonary alveolar proteinosis. Nat Commun 2018;9:3127. [Crossref] [PubMed]
- Uchida K, Nakata K, Carey B, Chalk C, Suzuki T, Sakagami T, Koch DE, Stevens C, Inoue Y, Yamada Y, Trapnell BC. Standardized serum GM-CSF autoantibody testing for the routine clinical diagnosis of autoimmune pulmonary alveolar proteinosis. J Immunol Methods 2014;402:57-70. [Crossref] [PubMed]
- Uchida K, Nakata K, Trapnell BC, Terakawa T, Hamano E, Mikami A, Matsushita I, Seymour JF, Oh-Eda M, Ishige I, Eishi Y, Kitamura T, Yamada Y, Hanaoka K, Keicho N. High-affinity autoantibodies specifically eliminate granulocyte-macrophage colony-stimulating factor activity in the lungs of patients with idiopathic pulmonary alveolar proteinosis. Blood 2004;103:1089-98. [Crossref] [PubMed]
- Li Y, Tian X, Gui Y, Ma A, Li X, Zeng N, Zhang P, Li G, Xu K. Serum markers in patients with idiopathic pulmonary alveolar proteinosis. Zhonghua Jie He He Hu Xi Za Zhi 2014;37:497-501. [PubMed]
- Ronneberger O, Fischer P, Brox T. U-Net: Convolutional Networks for Biomedical Image Segmentation. In: Navab N, Hornegger J, Wells W, Frangi A. editors. Medical Image Computing and Computer-Assisted Intervention MICCAI 2015. Cham: Springer, 2015:234-41.
- Trapnell BC, Nakata K, Bonella F, Campo I, Griese M, Hamilton J, Wang T, Morgan C, Cottin V, McCarthy C. Pulmonary alveolar proteinosis. Nat Rev Dis Primers 2019;5:16. [Crossref] [PubMed]
- Tazawa R, Ueda T, Abe M, Tatsumi K, Eda R, Kondoh S, et al. Inhaled GM-CSF for Pulmonary Alveolar Proteinosis. N Engl J Med 2019;381:923-32. [Crossref] [PubMed]
- Ramtohul T, Cabel L, Paoletti X, Chiche L, Moreau P, Noret A, Vuagnat P, Cherel P, Tardivon A, Cottu P, Bidard FC, Servois V, Quantitative CT. Extent of Lung Damage in COVID-19 Pneumonia Is an Independent Risk Factor for Inpatient Mortality in a Population of Cancer Patients: A Prospective Study. Front Oncol 2020;10:1560. [Crossref] [PubMed]
- Tokura S, Akira M, Okuma T, Tazawa R, Arai T, Sugimoto C, et al. A Semiquantitative Computed Tomographic Grading System for Evaluating Therapeutic Response in Pulmonary Alveolar Proteinosis. Ann Am Thorac Soc 2017;14:1403-11. [Crossref] [PubMed]
- Shi S, Chen L, Qiu X, Zhao Q, Xiao Y, Yan X. Valuable Serum Markers in Pulmonary Alveolar Proteinosis. Dis Markers 2019;2019:9709531. [Crossref] [PubMed]
- Seymour JF, Presneill JJ. Pulmonary alveolar proteinosis: progress in the first 44 years. Am J Respir Crit Care Med 2002;166:215-35. [Crossref] [PubMed]