Clinical studies of magnetic resonance elastography from 1995 to 2021: Scientometric and visualization analysis based on CiteSpace
Introduction
Viscoelasticity is a mechanical property of soft tissue. Its parameters are related to the structure (1) and physiological state of the tissue, such as muscle tension and compression (2), stiffening of the penis during erection (3), and small changes in brain perfusion due to activity (4). These changes are also related to numerous diseases and responses to treatment (5-10). Therefore, quantitative assessment of tissue viscoelasticity will further our understanding of the physiological condition of an organ, disease diagnosis, monitoring of treatment effects, and prognosis.
Tissue viscoelasticity comprises elasticity and viscosity and can be assessed non-invasively by elastography. Elasticity, representing tissue stiffness, is measured with a shear storage modulus, whereas viscosity, reflecting internal friction between fluid layers under shear stress, is evaluated with a shear loss modulus (11). Elastography is commonly performed using ultrasound (US) or magnetic resonance imaging (MRI) (12). Since Parker et al. first used US-based elastography to image the biomechanical properties of tissues over 30 years ago, the use of this technique has expanded rapidly (13). There are 2 major types of elastography: strain elastography and shear wave elastography (SWE). Strain elastography qualitatively measures the tissue deformation caused by compression, but its clinical use is limited due to difficulty assessing tissue deformation in response to an external mechanical force. Meanwhile, SWE quantitatively measures the velocity of propagation of a shear wave through a tissue. Currently available SWE methods include vibration-controlled transient elastography (VCTE), point SWE (pSWE), and 2-dimensional (2D) SWE. The VCTE method measures the unidimensional wave speed of a single mechanical pulse emitted by the probe through the target tissue without images. The A and TM mode maps are used to guide operators to find the ideal location within the tissue. The pSWE method uses acoustic radiation force to displace tissues at a single point within a tissue and measures shear wave speed within small regions of interest, whereas 2D-SWE uses sequential multiple points of displacement to produce a 2D map of stiffness measurement within a larger region of interest (ROI) (14,15). These methods have the advantages of being easily applied by operators, fast to perform, relatively inexpensive, and readily accepted by patients; therefore, they are widely used to evaluate lesions in various organs, such as the liver, breast, prostate, thyroid, spleen, and kidney (16-20). However, these methods are dependent on both the operator and recipient, and the diagnostic performance of US elastography is reduced in obese patients due to insufficient penetration (21).
The accuracy of results obtained via US elastography has been compared to that of magnetic resonance elastography (MRE) (22). In particular, time-harmonic US elastography, which has been used to reach deeper tissues, has been reported to have similar diagnostic accuracy to MRE (23). The MRE technique is a MRI method with a phase-contrast pulse sequence that yields phase difference images, from which the shear modulus based on the wave speed of propagating shear waves is measured and then used to calculate a tissue’s viscoelasticity (24,25). The MRE differs from US-based elastography, which assesses Young’s modulus (14). Compared with US-based elastography, MRE samples a much larger volume of tissue, is not affected by the mass index, and is not operator dependent (26-28). The most well-established clinical application of MRE is in the detection and quantification of hepatic fibrosis (29), with accurate and reliable results, as well as high intra- and inter-observer agreement (30). Recently, applications for MRE have been extended to the detection of stiffness in the brain, lung, heart, kidney, spleen, and prostate tissues (31-36). Many studies have examined the development of the MRE technique and its clinical application. However, there has been no scientometric analysis and visualization of research in this field.
The CiteSpace software developed by Chen et al. (37) is a powerful tool for scientometric and visualization analysis that focuses on exploring key research studies, institutions, and countries, as well as their cooperation. CiteSpace also identifies research hotspots, frontier areas, and research trends in a specified field based on data from the Web of Science database (38). CiteSpace has been widely used in the medical field (39-42).
In the present study, CiteSpace was used to perform a scientometric and visualization analysis of MRE from 1995 to 2021. The aims of this study were to construct an MRE knowledge framework and explore research hotspots, frontier areas, and emerging trends in MRE.
Methods
Data acquisition
To obtain MRE-related studies published between 1995 and 2021, the Science Citation Index Expanded of the Web of Science Core Collection (WoSCC) was searched on 22 October 2021 using the following terms: “magnetic resonance elastography” OR “MR elastography” OR “MRE” AND English. Retrieved articles were categorized as either “article” or “review” document types. Subsequently, the abstracts of each of the papers were screened by 2 reviewers (NC and YZ) jointly to identify studies on human participants. Studies performed on animals and those not related to MRE were excluded. Finally, the raw data for the identified papers were downloaded as full-text documents with references and in plain-text format, including the title, abstract, keywords, authors’ names, institutions, countries, year of publication, and references.
This study was designed as a literature review; neither ethics approval nor informed consent was applicable.
Analytical methods
Microsoft (Bellevue, WA, USA) Excel 2016 and CiteSpace V version 5.8.R3 (downloaded from http://cluster.cis.drexel.edu/~cchen/citespace/) were used to analyze the downloaded data. Excel 2016 was used to draw annual maps of published literature on MRE from 1995 to 2021, revealing trends in the number of articles published by year. CiteSpace V was used to analyze the author, institution, and country cooperative networks, to perform reference co-citation analysis to display the MRE knowledge framework, and to conduct keyword co-occurrence and burst keyword analyses to explore research trends, hotspots, and research frontiers in the field. The CiteSpace parameters were set as follows: the publication date of the articles (time slicing) ranging from January 1995 to October 2021, “years per slice” = 1; the term “source” included the title, abstract, author keywords, and keywords plus; Strength = “Cosine”, Scope = “Within slices”, and Top N=30; “Minimum Spanning Tree” and “Pruning Sliced networks” for the cooperation network, and “pathfinder” and “pruning the merged network” for the reference co-citation and keywords co-occurrence analyses; Visualization was set as “Cluster View Static” and “Show Merged Network”.
Networks are characterized by the central parameters of CiteSpace, including node, betweenness centrality, and burst detection. Nodes in the cooperation network maps represent authors, institutions, or countries, whereas in keyword networks they represent keywords. The size of a node is proportional to the number of articles published or the frequency of keyword occurrence, and the color of nodes indicates the years of occurrence or citation. Betweenness centrality, a quantitative indicator of the influence of a node in the network, is defined as the fraction of shortest paths between node pairs that pass through a given node of interest (43). The higher the betweenness centrality of the node, the greater its importance in the network, and these nodes appear with a purple rim in the output maps. The burst detection algorithm can be adapted to detect sharp increases in interest in a specialty (44). The degree of burst is represented by burst strength, and keywords with a higher strength are often identified as hotspots or turning points in the field.
Keywords are words or phrases that reflect the characteristics of a paper. A reference refers to the literature cited when writing papers or specific research works. It is the basis of research progress in a certain field. Therefore, the knowledge framework is derived from the reference co-citation network, keyword clusters of cited references, and the highly cited reference literature (45). Co-occurrence keywords analysis is commonly used to explore research hotspots and emerging trends, whereas keyword burst detection is used to discover frontier areas (46,47). In the present study, hotspots are represented by keywords with higher frequency and betweenness centrality, whereas emerging trends are represented by the evolution of keywords. Frontier areas are represented by keyword bursts with a higher strength.
Results
Article selection and publication year
In all, 2,825 studies were identified from the WoSCC search, with 2,353 studies included after refinement using the “article” and “review” keywords and the exclusion of meeting abstracts, early access papers, proceedings papers, editorial material, corrections, notes, letters, and data papers. After reading each abstract, a further 1,117 studies were excluded, of which 8 were associated with animal experiments and 1,109 were not related to MRE. Thus, 1,236 studies were subjected to CiteSpace analysis in the present study (Figure 1).
Three distinct time periods were demonstrated for publications on MRE (Figure 2). The first period was between 1995 and 1999, when 1 article on MRE was published per year. During the second period, from 2000 to 2010, the number of articles published increased steadily and slowly, and was maintained at 6–36 papers annually. During the third period [2011–2021], the number of articles published grew at a remarkable rate, increasing from 34 in 2011 to 140 in 2020. At the time of writing, 138 articles had been published in 2021.
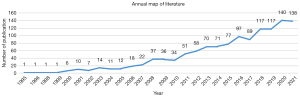
Collaboration network analysis
Analysis of author cooperation
The network map of coauthorship (Figure 3) shows that 1,236 articles were written by 726 authors; 675 authors published <10 articles, 32 published 10–20 articles, 12 published 21–50 articles, and 7 published >50 articles. The top 10 authors were Richard Ehman (n=185 articles), Ingolf Sack (n=130), Juergen Braun (n=109), Ralph Sinkus (n=82), Kevin Glaser (n=78), Armando Manduca (n=71), Meng Yin (n=54), Sudhakar Venkatesh (n=49), Jing Guo (n=38), and Rohit Loomba (n=37), accounting for 67.4% of all articles included in this analysis. Neil Roberts, John Huston III, Kevin Glaser, Dieter Klatt, Philippe Garteiser, and Jens Wuerfel played a positive role in authors’ cooperation because of their higher centrality (Table 1). Richard Ehman, Kevin Glaser, Meng Yin, Armando Manduca, Sudhakar Venkatesh, Rohit Loomba, John Huston III, and Dieter Klatt were from the US; Ingolf Sack, Juergen Braun, Jing Guo, and Jens Wuerfel were from Germany; Ralph Sinkus and Neil Roberts were from the UK; and Philippe Garteiser was from France.
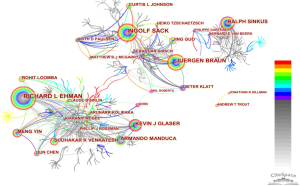
Table 1
Ranking | Author | No. of papers | Country | Author | Centrality | Country |
---|---|---|---|---|---|---|
1 | Richard Ehman | 185 | USA | Neil Roberts | 0.53 | UK |
2 | Ingolf Sack | 130 | Germany | John Huston III | 0.51 | US |
3 | Juergen Braun | 109 | Germany | Kevin J. Glaser | 0.50 | US |
4 | Ralph Sinkus | 82 | UK | Philippe Garteiser | 0.32 | France |
5 | Kevin Glaser | 78 | USA | Jens Wuerfel | 0.32 | Germany |
6 | Armando Manduca | 71 | USA | Dieter Klatt | 0.31 | USA |
7 | Meng Yin | 54 | USA | Ralph Sinkus | 0.28 | UK |
8 | Sudhakar Venkatesh | 49 | USA | Peter Martus | 0.26 | Germany |
9 | Jing Guo | 38 | Germany | Ingolf Sack | 0.19 | Germany |
10 | Rohit Loomba | 37 | USA | Keith Paulsen | 0.19 | USA |
MRE, magnetic resonance elastography.
Analysis of institutional cooperation
A total of 540 institutions had contributed to MRE research (Figure 4). The top 10 institutions published 679 articles (Table 2), accounting for 54.9% of all articles included in this study. The Mayo Clinic published 240 papers, and was ranked the publishing institution, followed by Charité, University of Illinois, Dartmouth College, and University of California San Diego. Each of these institutions published ≥50 papers. King’s College London, University of Delaware, Dartmouth Hitchcock Medical Center, Cincinnati Children’s Hospital Medical Center, and University of Ohio State each published 26–33 papers. In terms of centrality, Mayo Clinic (0.61), Charité (0.20), King’s College London (0.15), and University of California San Diego (0.14) played an important role in institutional cooperation.
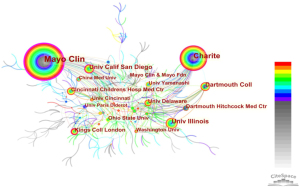
Table 2
Ranking | Country | No. of papers | Centrality | Institution | No. of papers | Centrality |
---|---|---|---|---|---|---|
1 | USA | 631 | 0.61 | Mayo Clinic | 240 | 0.61 |
2 | Germany | 202 | 0.24 | Charité | 131 | 0.20 |
3 | France | 134 | 0.40 | University of Illinois | 56 | 0.04 |
4 | China | 105 | 0.07 | Dartmouth College | 52 | 0.04 |
5 | UK | 96 | 0.06 | University of California San Diego | 50 | 0.13 |
6 | Japan | 91 | 0.20 | King’s College London | 33 | 0.15 |
7 | Canada | 73 | 0.13 | University of Delaware | 32 | 0.04 |
8 | South Korea | 57 | 0.00 | Dartmouth Hitchcock Medical Center | 31 | 0.01 |
9 | Australia | 30 | 0.06 | Cincinnati Children’s Hospital Medical Center | 28 | 0.02 |
10 | Switzerland | 29 | 0.12 | University of Ohio State | 26 | 0.03 |
MRE, magnetic resonance elastography.
Eight of the top 10 institutions are in the US (Mayo Clinic, University of Illinois, Dartmouth College, University of California San Diego, University of Delaware, Dartmouth Hitchcock Medical Center, Cincinnati Children’s Hospital Medical Center, and University of Ohio State); Charité is in Germany; and King’s College London is in the UK.
Analysis of country cooperation
The network map of country cooperation showed that 40 countries had participated in MRE research (Figure 5). As indicated in Table 2, the USA had contributed the highest number of articles (n=631), followed by Germany (n=202), France (n=134), China (n=105), UK (n=96), Japan (n=91), Canada (n=73), South Korea (n=57), Australia (n=30), and Switzerland (n=29). Of these countries, the USA, France, Germany, and Japan played important roles in this field, with centrality values of 0.61, 0.40, 0.24, and 0.20, respectively.

Analysis of co-citation references
The 24,347 distinct references formed a network map of co-citation references with 639 nodes, 2,100 links, and 48 clusters obtained via reasonable clustering using specific keywords (Figure 6). In the top 10 clusters (Table 3), “liver fibrosis” ranked first, with 86 members, followed by “springpot” (n=84), “brain” (n=62), “radiofrequency” (n=55), “myofascial pain” (n=46), “MR imaging” (n=42), “elasticity reconstruction” (n=40), “mechanical” (n=29), “elastic modulus” (n=25), and “contraction” (n=24).
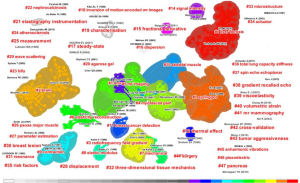
Table 3
Cluster ID | Size | Silhouette value | Mean year | Cluster label | Label (LLR) |
---|---|---|---|---|---|
0 | 86 | 0.94 | 2014 | Liver fibrosis | Liver fibrosis; brain; transient elastography; non-alcoholic fatty liver disease |
1 | 84 | 0.90 | 2006 | Springpot | Springpot; acoustic radiation force; brain viscoelasticity; dynamic contrast-enhanced MR imaging; normal pressure hydrocephalus |
2 | 62 | 0.95 | 2013 | Brain | Brain; hippocampus; viscoelasticity; liver fibrosis; aging |
3 | 55 | 0.95 | 2000 | Radiofrequency | Radiofrequency field gradient; vibrations; compression test; anharmonic vibrations; non-linear harmonics |
4 | 46 | 0.98 | 2001 | Myofascial pain | Myofascial pain; elastic properties; finite element modeling; skeletal muscle; shear stiffness |
5 | 42 | 0.93 | 2001 | MR imaging | MR imaging; prostate gland; inversion algorithms; plantar mechanical properties; breast cancer detection |
6 | 40 | 0.97 | 1995 | Elasticity reconstruction | Elasticity reconstruction; subzone technique; model-based imaging; regularized inversion techniques; finite element method |
7 | 29 | 0.92 | 1999 | Mechanical | Mechanical; arteriosclerosis; stress; ultrasonography; magnetic resonance elastography |
8 | 25 | 0.97 | 1997 | Elastic modulus | Elastic modulus; MR-elastography; tumor detection; tissue characterization; magnetic resonance elastography |
9 | 24 | 1 | 1997 | Contraction | Contraction; muscle; mechanical properties; Magnetic resonance imaging; elastography |
MRE, magnetic resonance elastography; LLR, log-likelihood ratio; MR, magnetic resonance.
The top 15 references with higher citations are presented in Table 4. The article published by Venkatesh et al. (48) was cited 94 times, whereas the other 14 articles (27,29,49-60) were cited between 52 and 85 times. Some 7 articles were from the cluster “liver fibrosis”, 7 were from the “springpot” cluster, and 1 was from the “brain” cluster.
Table 4
Ranking | No. of citations | Centrality | Year | Reference |
---|---|---|---|---|
1 | 94 | 0.01 | 2013 | Venkatesh et al. (48) |
2 | 85 | 0.02 | 2016 | Yin et al. (27) |
3 | 81 | 0.11 | 2007 | Yin et al. (51) |
4 | 81 | 0.05 | 2015 | Singh et al. (55) |
5 | 70 | 0.01 | 2008 | Sack et al. (59) |
6 | 69 | 0.02 | 2016 | Imajo et al. (54) |
7 | 65 | 0.01 | 2006 | Rouvière et al. (49) |
8 | 65 | 0.04 | 2014 | Loomba et al. (29) |
9 | 62 | 0.07 | 2011 | Chen et al. (53) |
10 | 62 | 0.02 | 2008 | Huwart et al. (50) |
11 | 60 | 0.04 | 2010 | Wuerfel et al. (58) |
12 | 59 | 0.05 | 2010 | Asbach et al. (52) |
13 | 55 | 0.14 | 2008 | Green et al. (56) |
14 | 54 | 0.08 | 2008 | Kruse et al. (57) |
15 | 52 | 0.19 | 2009 | Sack et al. (60) |
MRE, magnetic resonance elastography.
Analysis of keyword co-occurrence
The map of keyword co-occurrence, with 294 nodes and 1,259 links, was established from the qualified 1,195 records (Figure 7A). “Magnetic resonance elastography” had the highest frequency of use (n=843 times), followed by “hepatic fibrosis” (n=366), “stiffness” (n=239), “non-invasive assessment” (n=187), and “tissue” (n=167). Nodes representing important keywords were marked with a purple circle; “in vivo” and “skeletal muscle” were identified as the first keywords with the highest centrality (0.23), followed by “magnetic resonance elastography” (0.17), “acoustic strain wave” (0.13), and “stiffness” (0.12; Table 5).
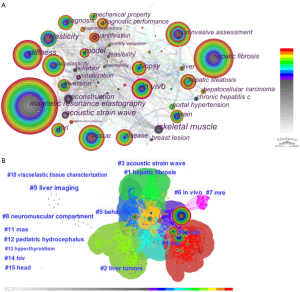
Table 5
Ranking | Keyword | Frequency | Ranking | Keyword | Centrality |
---|---|---|---|---|---|
1 | Magnetic resonance elastography | 843 | 1 | In vivo | 0.23 |
2 | Hepatic fibrosis | 366 | 2 | Skeletal muscle | 0.23 |
3 | Stiffness | 239 | 3 | Magnetic resonance elastography | 0.17 |
4 | Non-invasive assessment | 187 | 4 | Acoustic strain wave | 0.13 |
5 | Tissue | 167 | 5 | Stiffness | 0.12 |
6 | Viscoelasticity | 145 | 6 | Model | 0.11 |
7 | In vivo | 143 | 7 | Elasticity | 0.10 |
8 | Disease | 138 | 8 | Reconstruction | 0.10 |
9 | Hepatic steatosis | 122 | 9 | Disease | 0.09 |
10 | Elasticity | 112 | 10 | MRI | 0.09 |
MRE, magnetic resonance elastography; MRI, magnetic resonance imaging.
There were 16 clusters in the keyword co-occurrence clustering map (Figure 7B), which were summarized into 2 categories: (I) MRE technique (“acoustic strain wave”, “MRI”, “MRE” and “viscoelastic tissue characterization”); and (II) MRE clinical application (“brain”, “hepatic fibrosis”, “liver tumors”, “behavior”, “in vivo”, “neuromuscular compartment”, “liver imaging”, “MAS”, “pediatric hydrocephalus”, “hyperthyroidism”, “HIV”, and “head”; Table 6).
Table 6
Cluster ID | Size | Silhouette value | Mean year | Cluster label | Label (LLR) |
---|---|---|---|---|---|
0 | 62 | 0.81 | 2010 | Brain | Brain; liver fibrosis; transient elastography; biopsy; disease |
1 | 44 | 0.67 | 2003 | Hepatic fibrosis | Hepatic fibrosis; reconstruction; finite element modeling; liver fibrosis; strain imaging |
2 | 38 | 0.78 | 2008 | Liver tumor | Liver tumors; diffusion MRI; contrast agent; Diffusion-weighted imaging; prostate imaging |
3 | 36 | 0.81 | 2005 | Acoustic strain wave | Acoustic strain wave; radiofrequency field gradient; thigh muscle; actuator; contraction |
4 | 32 | 0.72 | 2008 | MRI | MRI; strain; myocardium; accurate; magnetic resonance elastography |
5 | 25 | 0.71 | 2009 | Behavior | Brain; behavior; MRI; viscoelasticity; disease |
6 | 21 | 0.89 | 2007 | In vivo | In vivo; viscoelastic parameters; transient elastography; feasibility; temperature |
7 | 10 | 0.95 | 2001 | MRE | MRE; meningioma; MR elastography; magnetic resonance elastography; complication |
8 | 9 | 0.96 | 2006 | Neuromuscular compartment | Neuromuscular compartment; human skeletal muscle; cross sectional area; spinal cord injury; relaxation time |
9 | 8 | 1 | 2010 | Liver image | Liver imaging; dynamic contrast enhanced MR imaging; MR spectroscopy; functional MR imaging; apparent diffusion coefficient |
LLR, log-likelihood ratio; MR, magnetic resonance; MRE, magnetic resonance elastography; MRI, magnetic resonance imaging.
The time zone view of the co-occurrence of keywords (Figure 8) shows the top 10 high-frequency keywords and the top 10 high-centrality keywords between 1995 and 2011. The earliest research direction was “tissue” in 1995, followed by “magnetic resonance elastography” and “acoustic strain wave” in 1998; “disease”, “elasticity”, and “reconstruction” in 2000; “in vivo”, “MRI”, “model”, “skeletal muscle”, and “visualization” in 2001; “stiffness” in 2002; “breast lesion”, “breast cancer”, and “cancer” in 2003; “viscoelasticity” and “behavior” in 2004; “hepatic fibrosis”, “non-invasive assessment”, and “biopsy” in 2006; “diagnosis” and “portal hypertension” in 2007; “inversion”, “brain”, and “quantification” in 2008; “hepatic steatosis” in 2009; “diagnostic performance”, “mechanical property”, and “hepatocellular carcinoma” in 2010; and “repeatability” in 2013. Since 2014, other keywords have appeared, such as “diagnostic accuracy” and “chronic hepatitis B”; however, these keywords have lower frequency and centrality.
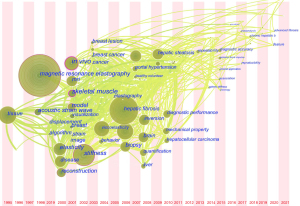
Analysis of the keyword burst
Figure 9 shows the top 25 keywords with the strongest citation bursts. The first keyword burst was “tissue”, which started in 1995 and ended in 2008. The second keyword burst was “acoustic strain wave”, with the highest burst strength (19.71), which appeared in 1998 and lasted until 2008. From 2000 to 2017, 15 keywords bursts disappeared consecutively. In the past 4 years, 8 keyword bursts have received significant attention: “diagnostic performance”, “diagnostic accuracy”, “hepatic steatosis”, “chronic hepatitis B”, “radiation force impulse”, “children”, “elastography”, and “echo”.
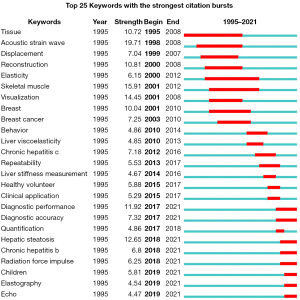
Discussion
General information
Since Muthupillai et al. (61) introduced MRE in 1995, this method has become a useful tool for studying a tissue’s physiological or pathological state. Technological improvements to the technique and its extended application in various organs have led to marked increases in the number of MRE-related articles published each year. This trend is consistent with the history of MRE (Appendix 1).
In this study, we identified articles published by 726 authors from 540 institutions in 40 countries. The USA, Germany, and France have made important contributions to research related to MRE and are central collaborators with other countries. Some 8 of the top 10 institutions and 8 of top 15 important authors (with higher number of papers or higher centrality) were from the USA; 1 institution and 4 authors were from Germany, and 1 author was from France.
Knowledge framework
The 639 keywords extracted from 24,347 cited references were grouped into 48 clusters, which, along with 15 highly frequently cited references, formed the knowledge framework for the MRE field. In the top 10 clusters, Cluster numbers 0, 2, and 3 indicated that MRE was mainly used in the case of liver fibrosis, brain, and myofascial pain, respectively; the other clusters were focused on MRE techniques.
MRE is a non-invasive MRI technique that reflects the complex interactions between cells and the extracellular matrix (62). An MRE consists of 3 components: a mechanical wave generator, a phase-contrast magnetic resonance (MR) sequence for acquiring wave propagation, and an inversion algorithm to process the effects of the shear waves on mechanical parameters.
The sources of the mechanical waves can be either external or internal. Most MRE methods use external sources of mechanical waves, such as electromagnetic coils, piezoelectric stacks, or pneumatic actuators (61,63-65). Both electromagnetic coils and piezoelectric stacks generate high-frequency vibrations and have been primarily used in animal research. The pneumatic actuator system consists of a loudspeaker placed outside the MR scanner room and a passive driver on the surface of the target tissue. This system is commonly used in clinical applications. Internal mechanical waves primarily originate from physiological motion, such as a beating heart (66).
Both longitudinal and shear waves exist simultaneously when a mechanical wave is applied to the tissue surface. Because the velocity of longitudinal wave propagation is very high compared with that of shear wave propagation, it does not vary significantly in different soft tissues. The MRE commonly measures the velocity of shear wave propagation to explore the mechanical properties of the tissues (22); therefore, longitudinal waves, which are confounding factors, must be removed by a spatial high-pass filter or the curl of the data (67). The MR pulse sequences including gradient-recalled echo or spin echo with/without echo-planar imaging (EPI), balanced steady-state free precession (SSFP), and spiral sequence (68-71) can capture the propagation velocities of shear waves, which are then transformed into biological properties via linear or non-linear inversion algorithms.
Monofrequency MRE uses a 20–100 Hz monofrequency wave (72) through target tissue. It assumes that the measured tissue is nearly incompressible and isotropic, and thereby shear wave velocity is the only parameter used to determine the stiffness of tissue linearly, without a specific rheological model to calculate storage and loss modulus. For traditional monofrequency MRE, the frequency of the motion-encoding gradient (MEG) is synchronized with monofrequency mechanical excitation, and the minimum echo time depends on the duration of the MEG. When low-vibration frequencies are used, the minimum echo time is increased and the images acquired can be prone to a low phase-to-noise ratio, especially in tissues with short T2 relaxation, such as the liver with iron overload. However, using novel EPI-based sequences to image quickly, the feasibility and clinical potential of cardiac monofrequency MRE (73) and functional (f)MRE (74) have been demonstrated. To evaluate tissue elasticity accurately, shear wave velocities in all 3 spatial directions are measured to acquire a complete data set. Since nearly all tissues/organs have different wave speeds at different frequencies, multifrequency MRE is often preferred to quantify the viscoelastic properties of these tissues/organs. Three models, namely Voigt, Maxwell, and springpot, are commonly used to analyze the data; the springpot model in particular better represents soft tissue behavior in the frequency range of MRE (75). However, in clinical practice, with limited scan time, monofrequency MRE has also been shown to be useful in characterizing tissue viscoelasticity (76).
With regard to MRE, the following should be noted:
- Achieving good-quality MRE requires the frequency and amplitude of the mechanical excitation to be in an appropriate range (22) and the wavelength/pixel ratio to be at 6–9 voxels per wavelength (77).
- In MRE, the density of the soft tissue is assumed to be equal to that of water (1.0 g/cm3); however, this assumption does not hold for lung MRE, where the tissue density is too low, and an exact density value is required (22).
- Following a meal, water intake, or activity, the biomechanical properties of the liver, spleen, kidney, and brain can change (78-80); therefore, patients should undergo MRE examinations during rest and in a fasting state.
The top 15 articles had the highest citation frequency. Venkatesh et al. (48) reviewed the technique, clinical application, and potential future application of liver MRE. Rouvière et al. (49) investigated the feasibility of MRE in volunteers and patients with chronic liver diseases. The success rate of liver MRE was estimated to be 94.4% (27,50). Others have studied values of conventional or multifrequency MRE to assess hepatic fibrosis (51,52), finding that the diagnostic performance of conventional or multifrequency MRE in determining the stage of liver fibrosis increased with the stage of fibrosis. Chen et al. (53) used MRE for the early detection of non-alcoholic steatohepatitis and reported that the mean hepatic stiffness for patients with inflammatory non-alcoholic fatty liver disease (NAFLD) was greater than that of patients with simple steatosis and lower than that of patients with fibrosis. Two studies (29,54) and a meta-analysis (55) have provided further evidence that MRE can be used to accurately diagnose advanced fibrosis in NAFLD patients.
In 2007, Green et al. (56) used MRE to measure the complex shear modulus in brain tissue in vivo and confirmed the validity of this technique. Concomitantly, Kruse et al. (57) obtained normative human cerebral data from different age groups using the shear modulus of MRE, with no age dependence found. However, the most acknowledged trend is brain softening with aging (81). Wuerfel et al. (58) demonstrated that brain parenchymal viscoelasticity was significantly reduced in patients with mild multiple sclerosis (MS) compared with healthy volunteers. In terms of a rheological model, Sack et al. (59) observed that MRE with Voigt’s model was inadequate for assessing the behavior of viscoelastic brain tissue, and that multifrequency MRE with the springpot model provided sensitive measurements (60).
Research hotspots, frontiers, and emerging trends
Research hotspots
Based on the results of CiteSpace, the 2 main research hotspots in the field of MRE were summarized as “MRE technique” and “clinical application”.
MRE technique
MRE is a non-invasive MRI method to quantitatively assess a tissue’s viscoelasticity or stiffness. Three technical components work together to complete this task: propagation of mechanical shear waves through the tissues of interest, MRE imaging sequences, and inversion algorithms. Shear waves produced by an actuator and their frequency have important implications for the contrast and resolution of stiffness maps. Optimal frequency will differentiate small lesions from surrounding tissues and improve sensitivity (82). Many MRE imaging sequences and emerging MRI technologies (68-71,83-91) have been used to shorten acquisition times, increase the coverage volume of the ROI, and obtain good resolution images, especially MRI scanners with newer techniques. Post-processing of acquired images to create quantitative stiffness maps requires specialized inversion algorithms. However, there are no standardized methods reported in the literature that are used across vendors and groups. They are also optimized over time (Appendix 1). These components continue to evolve, leading to decreased scan times and improved spatial resolution that, in turn, increase the sensitivity and accuracy of diagnosis, such that MRE can be widely used in clinical practice.
Apart from “magnetic resonance elastography”, the main research hotspots associated with MRE techniques from 1995 to 2021 were “stiffness”, “non-invasive assessment”, “tissue”, “in vivo”, “acoustic strain wave”, “viscoelasticity”, “elasticity”, “model”, “reconstruction”, and “mri”.
Clinical application
MRE research hotspots in the liver are “hepatic fibrosis” and “hepatic steatosis”, categorized as Cluster 0. Liver elastography is the first clinical application of MRE for the detection and staging of liver fibrosis. A standardized MRE protocol and agreement for the staging of fibrosis worldwide has been established (92). Liver elastography is currently the most accurate non-invasive imaging method for the initial detection and quantitative staging of fibrosis (92). It can differentiate significant fibrosis (stage ≥2) from mild fibrosis (stage 0–1) with >92% accuracy and a positive predictive value of >93%, and diagnose cirrhosis (stage 4) with >95% accuracy and a negative predictive value of >98% (52). MRE can also be used to evaluate NAFLD, specifically to differentiate NAFLD from steatohepatitis. A streamlined protocol has been established to calculate a virtual NAFLD activity score to estimate steatosis, inflammation/ballooning, and fibrosis (93).
Research hotspots in the brain are found in Cluster 1 (springpot) and Cluster 2 (brain). Neurological disorders are one of the most important public health concerns worldwide. MRE, as a non-invasive measurement of brain mechanical properties, has been used to investigate various neurological disorders, including intracranial tumors, and diffuse diseases such as dementia and MS. Wuerfel et al. (58) first studied brain stiffness in patients with MS and showed that global stiffness was decreased in MS patients compared with control subjects. Similarly, decreased brain stiffness has been seen in most neurodegenerative diseases, such as Alzheimer’s disease (94). For intracranial tumors, MRE not only accurately predicts tumor stiffness and helps surgeons with preoperative planning, but also measures heterogeneity within the tumor (95). Except for meningioma, which exhibits increased stiffness, brain tumors are reported as a softening of the mechanical rigidity of tissue (96). Furthermore, MRE can measure tumor adherence to surrounding tissues (97,98). More recently, 3-dimensional MRE has enabled evaluation of specific neuroanatomical regions, such as the corpus callosum, hippocampus, and corticospinal tract (99-101). However, there is still much work needed in this area, especially in establishing a brain MRE protocol for clinical application.
The research hotspot in “skeletal muscle” is categorized as Cluster 4. The US-based elastography has been widely used to investigate changes in muscle stiffness because it non-invasively provides information on muscle functional status, which is helpful in rehabilitation medicine for the designing assistive technologies. The MRE can also encode muscle function via the measurement of viscoelastic properties, but can only be used in a relaxed state (102). Recently, Schrank et al. (103) introduced real-time MRE to measure changes in viscoelastic parameters induced in different groups of skeletal muscle of the lower extremities during dynamic exercises. This method provides valuable information for the study of physiological processes and the diagnosis of diseases.
Emerging trends
The evolution of keywords reflects the emerging trends in MRE-associated research. From 1995 to 2000, studies focused on the development of MRE technology and preliminary experiences in ex vivo tissues, with keywords including “tissue”, “magnetic resonance elastography”, “acoustic strain wave”, “elasticity”, “disease”, and “reconstruction”. From 2001 to 2010, MRE was initially applied to various parts of the body, notably the liver, brain, breast, and skeletal muscle. A high number of research hotspots emerged, with keywords including “in vivo”, “MRI”, “model”, “skeletal muscle”, “visualization”, “stiffness”, “breast lesion”, “behavior”, “hepatic fibrosis”, “noninvasive assessment”, “biopsy”, “portal hypertension”, “brain”, “hepatic steatosis”, and “hepatocellular carcinoma”. Since 2011, the MRE technique has been optimized and is widely used clinically, and research on MRE has focused on “diagnostic performance”, “accuracy”, and “repeatability”.
Research frontiers
From the burst analysis of keywords, the research frontiers between 1995 and 2000 were “tissue”, “acoustic strain wave”, “displacement”, “reconstruction”, and “elasticity”. These topics were mainly associated with the MRE technique. Between 2001 and 2017, the research frontiers were “skeletal muscle”, “visualization”, “breast”, “breast cancer”, “behavior”, “liver viscoelasticity”, “chronic hepatitis C”, “repeatability”, “liver stiffness measurement”, “healthy volunteer”, and “clinical application”. These topics focused on the clinical application of MRE in various organs. In recent years, the research frontiers have included “diagnostic performance”, “diagnostic accuracy”, “hepatic steatosis”, “chronic hepatitis B”, “radiation force impulse”, “children”, “elastography”, and “echo”.
Many aspects of MRE have yet to be studied. First, unlike the case of liver MRE and the staging of fibrosis, many organs lack a standardized MRE protocol and agreement regarding baseline values in healthy individuals. Second, most MRE applications have focused on disease diagnosis, and recent studies have shifted towards monitoring treatment with neoadjuvant chemotherapy, predicting the risk of malignancy, and even disease prevention. Studies have shown that tumor stromal pressure and collagen changes may reflect the response to therapy (104,105), and more advanced MRE methods are needed to evaluate these microscopic changes. Third, the use of MRE in children is challenging (106). Therefore, it is critical to further develop MRE techniques to measure the mechanical properties of smaller structures more accurately. Current research focused on MRE technology has indicated the need for further improvement with regard to the MR sequence and the mechanical wave generator. These techniques, described by the keywords “echo” and “radiation force impulse”, are optimized to increase the “diagnostic performance” and “diagnostic accuracy” of MRE, so that it can be better used in “hepatic steatosis”, “chronic hepatitis B”, and “children”.
Limitations
This study had several limitations. First, only the WoSCC database was searched for research data. Certain literature studies were not included, which could have resulted in a significant sample size error. Second, only studies published in English were included, and those published in other languages were excluded, which may have caused language bias. Third, we focused mainly on MRE in humans and not animal experiments, so most studies involving animals were filtered out. A further limitation of this study is that authorship in relation to commercial contracts was not considered.
Conclusions
Scientometric and visualization analysis in MRE can provide information regarding the knowledge framework, research hotspots, frontier areas, and emerging trends in this field.
Acknowledgments
The authors express their gratitude to Wei Wang, Mingyong Gao (both from the Research Institute for Translational Medicine on Molecular Function and Artificial Intelligence Imaging and Department of Radiology, The First People’s Hospital of Foshan), and Zhichao Zhou (Director of Consulting Department, Health Science Library of Peking University) for their advice and careful modification of the manuscript.
Funding: This work was supported by the Summit Program of Foshan (Nos. 2019B008 and 2019C016).
Footnote
Conflicts of Interest: All authors have completed the ICMJE uniform disclosure form (available at https://qims.amegroups.com/article/view/10.21037/qims-22-207/coif). The authors have no conflicts of interest to declare.
Ethical Statement: The authors are accountable for all aspects of the work in ensuring that questions related to the accuracy or integrity of any part of the work are appropriately investigated and resolved. Due to the literature review design of the present study, neither ethics approval nor informed consent was applicable.
Open Access Statement: This is an Open Access article distributed in accordance with the Creative Commons Attribution-NonCommercial-NoDerivs 4.0 International License (CC BY-NC-ND 4.0), which permits the non-commercial replication and distribution of the article with the strict proviso that no changes or edits are made and the original work is properly cited (including links to both the formal publication through the relevant DOI and the license). See: https://creativecommons.org/licenses/by-nc-nd/4.0/.
References
- Ozkaya E, Fabris G, Macruz F, Suar ZM, Abderezaei J, Su B, Laksari K, Wu L, Camarillo DB, Pauly KB, Wintermark M, Kurt M. Viscoelasticity of children and adolescent brains through MR elastography. J Mech Behav Biomed Mater 2021;115:104229. [Crossref] [PubMed]
- Herbert RD, Clarke J, Kwah LK, Diong J, Martin J, Clarke EC, Bilston LE, Gandevia SC. In vivo passive mechanical behaviour of muscle fascicles and tendons in human gastrocnemius muscle-tendon units. J Physiol 2011;589:5257-67. [Crossref] [PubMed]
- Kelly DA. Penises as variable-volume hydrostatic skeletons. Ann N Y Acad Sci 2007;1101:453-63. [Crossref] [PubMed]
- Hetzer S, Birr P, Fehlner A, Hirsch S, Dittmann F, Barnhill E, Braun J, Sack I. Perfusion alters stiffness of deep gray matter. J Cereb Blood Flow Metab 2018;38:116-25. [Crossref] [PubMed]
- Darweesh SK, Zayed N, Atef M, Ramzy E, Yousry A, Musa S. Increased liver stiffness by transient elastography and acoustic radiation force impulse imaging in patients with extrahepatic cholestasis. Eur J Gastroenterol Hepatol 2021;33:1097-103. [Crossref] [PubMed]
- Zhou X, Rao J, Wu X, Deng R, Ma Y. Comparison of 2-D Shear Wave Elastography and Point Shear Wave Elastography for Assessing Liver Fibrosis. Ultrasound Med Biol 2021;47:408-27. [Crossref] [PubMed]
- Charette JH, Burak KW, Coffin CS, Congly SE, Lee SS, Israelson H, Williams S, Sadler M, Borman MA, Aspinall AI, Swain MG, Shaheen AA. Evaluating the role of transient elastography post chronic hepatitis C treatment to predict hepatocellular carcinoma. J Hepatol 2020;73:1287-9. [Crossref] [PubMed]
- Wu M, Ren A, Xu D, Peng X, Ye X, Li A. Diagnostic Performance of Elastography in Malignant Soft Tissue Tumors: A Systematic Review and Meta-analysis. Ultrasound Med Biol 2021;47:855-68. [Crossref] [PubMed]
- Hu B, Deng Y, Chen J, Kuang S, Tang W, He B, Zhang L, Xiao Y, Chen J, Rossman P, Arani A, Yin Z, Glaser KJ, Yin M, Venkatesh SK, Ehman RL, Wang J. Evaluation of MR elastography for prediction of lymph node metastasis in prostate cancer. Abdom Radiol (NY) 2021;46:3387-400. [Crossref] [PubMed]
- Katyan A, Mittal MK, Mani C, Mandal AK. Strain wave elastography in response assessment to neo-adjuvant chemotherapy in patients with locally advanced breast cancer. Br J Radiol 2019;92:20180515. [Crossref] [PubMed]
- Zhu Y, Dong C, Yin Y, Chen X, Guo Y, Zheng Y, Shen Y, Wang T, Zhang X, Chen S. The role of viscosity estimation for oil-in-gelatin phantom in shear wave based ultrasound elastography. Ultrasound Med Biol 2015;41:601-9. [Crossref] [PubMed]
- Yuan S, Magarik M, Lex AM, Fleischer AC. Clinical applications of sonoelastography. Expert Rev Med Devices 2016;13:1107-17. [Crossref] [PubMed]
- Parker KJ, Huang SR, Musulin RA, Lerner RM. Tissue response to mechanical vibrations for "sonoelasticity imaging". Ultrasound Med Biol 1990;16:241-6. [Crossref] [PubMed]
- Yin M, Venkatesh SK. Ultrasound or MR elastography of liver: which one shall I use? Abdom Radiol (NY) 2018;43:1546-51. [Crossref] [PubMed]
- Ozturk A, Olson MC, Samir AE, Venkatesh SK. Liver fibrosis assessment: MR and US elastography. Abdom Radiol (NY) 2022;47:3037-50. [Crossref] [PubMed]
- Ferraioli G, Barr RG, Farrokh A, Radzina M, Cui XW, Dong Y, Rocher L, Cantisani V, Polito E, D'Onofrio M, Roccarina D, Yamashita Y, Dighe MK, Dietrich CF. How to perform shear wave elastography. Part I. Med Ultrason 2022;24:95-106. [Crossref] [PubMed]
- Ferraioli G, Barr RG, Farrokh A, Radzina M, Cui XW, Dong Y, Rocher L, Cantisani V, Polito E, D'Onofrio M, Roccarina D, Yamashita Y, Dighe MK, Fodor D, Dietrich CF. How to perform shear wave elastography. Part II. Med Ultrason 2022;24:196-210. [Crossref] [PubMed]
- Tyloch DJ, Tyloch JF, Adamowicz J, Juszczak K, Ostrowski A, Warsiński P, Wilamowski J, Ludwikowska J, Drewa T. Elastography in prostate gland imaging and prostate cancer detection. Med Ultrason 2018;20:515-23. [Crossref] [PubMed]
- Lim WTH, Ooi EH, Foo JJ, Ng KH, Wong JHD, Leong SS. Shear Wave Elastography: A Review on the Confounding Factors and Their Potential Mitigation in Detecting Chronic Kidney Disease. Ultrasound Med Biol 2021;47:2033-47. [Crossref] [PubMed]
- Li B, Zhao X, Wang Q, Jing H, Shao H, Zhang L, Cheng W. Prediction of high nodal burden in invasive breast cancer by quantitative shear wave elastography. Quant Imaging Med Surg 2022;12:1336-47. [Crossref] [PubMed]
- Nadebaum DP, Nicoll AJ, Sood S, Gorelik A, Gibson RN. Variability of Liver Shear Wave Measurements Using a New Ultrasound Elastographic Technique. J Ultrasound Med 2018;37:647-56. [Crossref] [PubMed]
- Manduca A, Bayly PJ, Ehman RL, Kolipaka A, Royston TJ, Sack I, Sinkus R, Van Beers BE. MR elastography: Principles, guidelines, and terminology. Magn Reson Med 2021;85:2377-90. [Crossref] [PubMed]
- Hudert CA, Tzschätzsch H, Guo J, Rudolph B, Bläker H, Loddenkemper C, Luck W, Müller HP, Baumgart DC, Hamm B, Braun J, Holzhütter HG, Wiegand S, Sack I US. Time-Harmonic Elastography: Detection of Liver Fibrosis in Adolescents with Extreme Obesity with Nonalcoholic Fatty Liver Disease. Radiology 2018;288:99-106. [Crossref] [PubMed]
- Serai SD, Yin M. MR Elastography of the Abdomen: Basic Concepts. Methods Mol Biol 2021;2216:301-23. [Crossref] [PubMed]
- Venkatesh SK, Ehman RL. Magnetic resonance elastography of abdomen. Abdom Imaging 2015;40:745-59. [Crossref] [PubMed]
- Chen J, Yin M, Talwalkar JA, Oudry J, Glaser KJ, Smyrk TC, Miette V, Sandrin L, Ehman RL. Diagnostic Performance of MR Elastography and Vibration-controlled Transient Elastography in the Detection of Hepatic Fibrosis in Patients with Severe to Morbid Obesity. Radiology 2017;283:418-28. [Crossref] [PubMed]
- Yin M, Glaser KJ, Talwalkar JA, Chen J, Manduca A, Ehman RL, Hepatic MR. Elastography: Clinical Performance in a Series of 1377 Consecutive Examinations. Radiology 2016;278:114-24. [Crossref] [PubMed]
- Shire NJ, Yin M, Chen J, Railkar RA, Fox-Bosetti S, Johnson SM, Beals CR, Dardzinski BJ, Sanderson SO, Talwalkar JA, Ehman RL. Test-retest repeatability of MR elastography for noninvasive liver fibrosis assessment in hepatitis C. J Magn Reson Imaging 2011;34:947-55. [Crossref] [PubMed]
- Loomba R, Wolfson T, Ang B, Hooker J, Behling C, Peterson M, Valasek M, Lin G, Brenner D, Gamst A, Ehman R, Sirlin C. Magnetic resonance elastography predicts advanced fibrosis in patients with nonalcoholic fatty liver disease: a prospective study. Hepatology 2014;60:1920-8. [Crossref] [PubMed]
- Trout AT, Serai S, Mahley AD, Wang H, Zhang Y, Zhang B, Dillman JR. Liver Stiffness Measurements with MR Elastography: Agreement and Repeatability across Imaging Systems, Field Strengths, and Pulse Sequences. Radiology 2016;281:793-804. [Crossref] [PubMed]
- Bunevicius A, Schregel K, Sinkus R, Golby A, Patz S. REVIEW: MR elastography of brain tumors. Neuroimage Clin 2020;25:102109. [Crossref] [PubMed]
- Marinelli JP, Levin DL, Vassallo R, Carter RE, Hubmayr RD, Ehman RL, McGee KP. Quantitative assessment of lung stiffness in patients with interstitial lung disease using MR elastography. J Magn Reson Imaging 2017;46:365-74. [Crossref] [PubMed]
- Sui Y, Arunachalam SP, Arani A, Trzasko JD, Young PM, Glockner JF, Glaser KJ, Lake DS, McGee KP, Manduca A, Rossman PJ, Ehman RL, Araoz PA. Cardiac MR elastography using reduced-FOV, single-shot, spin-echo EPI. Magn Reson Med 2018;80:231-8. [Crossref] [PubMed]
- Han JH, Ahn JH, Kim JS. Magnetic resonance elastography for evaluation of renal parenchyma in chronic kidney disease: a pilot study. Radiol Med 2020;125:1209-15. [Crossref] [PubMed]
- Wang XP, Wang Y, Ma H, Wang H, Yang DW, Zhao XY, Jin EH, Yang ZH. Assessment of liver fibrosis with liver and spleen magnetic resonance elastography, serum markers in chronic liver disease. Quant Imaging Med Surg 2020;10:1208-22. [Crossref] [PubMed]
- Dittmann F, Reiter R, Guo J, Haas M, Asbach P, Fischer T, Braun J, Sack I. Tomoelastography of the prostate using multifrequency MR elastography and externally placed pressurized-air drivers. Magn Reson Med 2018;79:1325-33. [Crossref] [PubMed]
- Chen C, Hu Z, Liu S, Tseng H. Emerging trends in regenerative medicine: a scientometric analysis in CiteSpace. Expert Opin Biol Ther 2012;12:593-608. [Crossref] [PubMed]
- Chen D, Liu Z, Luo Z, Webber M, Chen J. Bibliometric and visualized analysis of emergy research. Ecol Eng 2016;90:285-93. [Crossref]
- Han X, Wei D. Advances in Research on Abused Women in China: Bibliometric Analysis by CiteSpace. Trauma Violence Abuse 2021; Epub ahead of print. [Crossref] [PubMed]
- Ma D, Yang B, Guan B, Song L, Liu Q, Fan Y, Zhao L, Wang T, Zhang Z, Gao Z, Li S, Xu H. A Bibliometric Analysis of Pyroptosis From 2001 to 2021. Front Immunol 2021;12:731933. [Crossref] [PubMed]
- Wu H, Wang Y, Tong L, Yan H, Sun Z. Global Research Trends of Ferroptosis: A Rapidly Evolving Field With Enormous Potential. Front Cell Dev Biol 2021;9:646311. [Crossref] [PubMed]
- Li Y, Fang R, Liu Z, Jiang L, Zhang J, Li H, Liu C, Li F. The association between toxic pesticide environmental exposure and Alzheimer's disease: A scientometric and visualization analysis. Chemosphere 2021;263:128238. [Crossref] [PubMed]
- Barthélemy M. Betweenness centrality in large complex networks. Eur Phys J B 2004;38:163-8. [Crossref]
- Kleinberg J. Bursty and hierarchical structure in streams. Proceedings of the 8th ACM SIGKDD Conference on knowledge Discovery and Data Mining. Edmonton, Alberta, Canada: ACM Press, 2002:91-101.
- Chen C, Ibekwe-SanJuan F, Hou J. The structure and dynamics of cocitation clusters: a multiple-perspective cocitation analysis. J Am Soc Inf Sci 2010;61:1386-409. [Crossref]
- OCLC. Achieving Web-scale for libraries [EB/OL]. [2012–11–13]. Available online: http://www.oclc.org/multimedia/2010/files/Achieving_Web_Scale_With_Libraries.pdf
- OCLC. OCLC Annual Report 2009/2010 [EB/OL]. [2012–11–13]. Available online: http://www.oclc.org/uk/en/news/publications/annualreports/2011/214629usb_Annual_Report.pdf
- Venkatesh SK, Yin M, Ehman RL. Magnetic resonance elastography of liver: technique, analysis, and clinical applications. J Magn Reson Imaging 2013;37:544-55. [Crossref] [PubMed]
- Rouvière O, Yin M, Dresner MA, Rossman PJ, Burgart LJ, Fidler JL, Ehman RL. MR elastography of the liver: preliminary results. Radiology 2006;240:440-8. [Crossref] [PubMed]
- Huwart L, Sempoux C, Vicaut E, Salameh N, Annet L, Danse E, Peeters F, ter Beek LC, Rahier J, Sinkus R, Horsmans Y, Van Beers BE. Magnetic resonance elastography for the noninvasive staging of liver fibrosis. Gastroenterology 2008;135:32-40. [Crossref] [PubMed]
- Yin M, Talwalkar JA, Glaser KJ, Manduca A, Grimm RC, Rossman PJ, Fidler JL, Ehman RL. Assessment of hepatic fibrosis with magnetic resonance elastography. Clin Gastroenterol Hepatol 2007;5:1207-1213.e2. [Crossref] [PubMed]
- Asbach P, Klatt D, Schlosser B, Biermer M, Muche M, Rieger A, Loddenkemper C, Somasundaram R, Berg T, Hamm B, Braun J, Sack I. Viscoelasticity-based staging of hepatic fibrosis with multifrequency MR elastography. Radiology 2010;257:80-6. [Crossref] [PubMed]
- Chen J, Talwalkar JA, Yin M, Glaser KJ, Sanderson SO, Ehman RL. Early detection of nonalcoholic steatohepatitis in patients with nonalcoholic fatty liver disease by using MR elastography. Radiology 2011;259:749-56. [Crossref] [PubMed]
- Imajo K, Kessoku T, Honda Y, Tomeno W, Ogawa Y, Mawatari H, Fujita K, Yoneda M, Taguri M, Hyogo H, Sumida Y, Ono M, Eguchi Y, Inoue T, Yamanaka T, Wada K, Saito S, Nakajima A. Magnetic Resonance Imaging More Accurately Classifies Steatosis and Fibrosis in Patients With Nonalcoholic Fatty Liver Disease Than Transient Elastography. Gastroenterology 2016;150:626-637.e7. [Crossref] [PubMed]
- Singh S, Venkatesh SK, Wang Z, Miller FH, Motosugi U, Low RN, Hassanein T, Asbach P, Godfrey EM, Yin M, Chen J, Keaveny AP, Bridges M, Bohte A, Murad MH, Lomas DJ, Talwalkar JA, Ehman RL. Diagnostic performance of magnetic resonance elastography in staging liver fibrosis: a systematic review and meta-analysis of individual participant data. Clin Gastroenterol Hepatol 2015;13:440-451.e6. [Crossref] [PubMed]
- Green MA, Bilston LE, Sinkus R. In vivo brain viscoelastic properties measured by magnetic resonance elastography. NMR Biomed 2008;21:755-64. [Crossref] [PubMed]
- Kruse SA, Rose GH, Glaser KJ, Manduca A, Felmlee JP, Jack CR Jr, Ehman RL. Magnetic resonance elastography of the brain. Neuroimage 2008;39:231-7. [Crossref] [PubMed]
- Wuerfel J, Paul F, Beierbach B, Hamhaber U, Klatt D, Papazoglou S, Zipp F, Martus P, Braun J, Sack I. MR-elastography reveals degradation of tissue integrity in multiple sclerosis. Neuroimage 2010;49:2520-5. [Crossref] [PubMed]
- Sack I, Beierbach B, Hamhaber U, Klatt D, Braun J. Non-invasive measurement of brain viscoelasticity using magnetic resonance elastography. NMR Biomed 2008;21:265-71. [Crossref] [PubMed]
- Sack I, Beierbach B, Wuerfel J, Klatt D, Hamhaber U, Papazoglou S, Martus P, Braun J. The impact of aging and gender on brain viscoelasticity. Neuroimage 2009;46:652-7. [Crossref] [PubMed]
- Muthupillai R, Lomas DJ, Rossman PJ, Greenleaf JF, Manduca A, Ehman RL. Magnetic resonance elastography by direct visualization of propagating acoustic strain waves. Science 1995;269:1854-7. [Crossref] [PubMed]
- Kostic A, Lynch CD, Sheetz MP. Differential matrix rigidity response in breast cancer cell lines correlates with the tissue tropism. PLoS One 2009;4:e6361. [Crossref] [PubMed]
- Doyley MM, Weaver JB, Van Houten EE, Kennedy FE, Paulsen KD. Thresholds for detecting and characterizing focal lesions using steady-state MR elastography. Med Phys 2003;30:495-504. [Crossref] [PubMed]
- Clayton EH, Garbow JR, Bayly PV. Frequency-dependent viscoelastic parameters of mouse brain tissue estimated by MR elastography. Phys Med Biol 2011;56:2391-406. [Crossref] [PubMed]
- Guertler CA, Okamoto RJ, Schmidt JL, Badachhape AA, Johnson CL, Bayly PV. Mechanical properties of porcine brain tissue in vivo and ex vivo estimated by MR elastography. J Biomech 2018;69:10-8. [Crossref] [PubMed]
- Keijzer LBH, Caenen A, Voorneveld J, Strachinaru M, Bowen DJ, van de Wouw J, Sorop O, Merkus D, Duncker DJ, van der Steen AFW, de Jong N, Bosch JG, Vos HJ. A direct comparison of natural and acoustic-radiation-force-induced cardiac mechanical waves. Sci Rep 2020;10:18431. [Crossref] [PubMed]
- Manduca A, Rossman TL, Lake DS, Glaser KJ, Arani A, Arunachalam SP, Rossman PJ, Trzasko JD, Ehman RL, Dragomir-Daescu D, Araoz PA. Waveguide effects and implications for cardiac magnetic resonance elastography: A finite element study. NMR Biomed 2018; Epub ahead of print. [Crossref] [PubMed]
- Braun J, Sack I. Phase cycling in dynamic magnetic resonance elastography by variation of the phase angle of the motion-encoding gradients. Proceedings of the 10th Annual Meeting of ISMRM. Honolulu, HI, USA, 2002:Abstract 40.
- Kruse SA, Grimm RC, Lake DS, Manduca A, Ehman RL. Fast EPI based 3D MR elastography of the brain. In: Proceedings of the International Society for Magnetic Resonance in Medicine. Seattle, Washington. 2006:3385.
- Klatt D, Asbach P, Rump J, Papazoglou S, Somasundaram R, Modrow J, Braun J, Sack I. In vivo determination of hepatic stiffness using steady-state free precession magnetic resonance elastography. Invest Radiol 2006;41:841-8. [Crossref] [PubMed]
- Peng X, Sui Y, Trzasko JD, Glaser KJ, Huston J 3rd, Ehman RL, Pipe JG. Fast 3D MR elastography of the whole brain using spiral staircase: Data acquisition, image reconstruction, and joint deblurring. Magn Reson Med 2021;86:2011-24. [Crossref] [PubMed]
- Glaser KJ, Manduca A, Ehman RL. Review of MR elastography applications and recent developments. J Magn Reson Imaging 2012;36:757-74. [Crossref] [PubMed]
- Arani A, Arunachalam SP, Chang ICY, Baffour F, Rossman PJ, Glaser KJ, Trzasko JD, McGee KP, Manduca A, Grogan M, Dispenzieri A, Ehman RL, Araoz PA. Cardiac MR elastography for quantitative assessment of elevated myocardial stiffness in cardiac amyloidosis. J Magn Reson Imaging 2017;46:1361-7. [Crossref] [PubMed]
- Lan PS, Glaser KJ, Ehman RL, Glover GH. Imaging brain function with simultaneous BOLD and viscoelasticity contrast: fMRI/fMRE. Neuroimage 2020;211:116592. [Crossref] [PubMed]
- Hirsch S, Braun J, Sack I. editors. Magnetic Resonance Elastography: Physical Background and Medical Applications. Weinheim, Germany: Wiley, 2017.
- Murphy MC, Cogswell PM, Trzasko JD, Manduca A, Senjem ML, Meyer FB, Ehman RL, Huston J 3rd. Identification of Normal Pressure Hydrocephalus by Disease-Specific Patterns of Brain Stiffness and Damping Ratio. Invest Radiol 2020;55:200-8. [Crossref] [PubMed]
- Mura J, Schrank F, Sack I. An analytical solution to the dispersion-by-inversion problem in magnetic resonance elastography. Magn Reson Med 2020;84:61-71. [Crossref] [PubMed]
- Dittmann F, Tzschätzsch H, Hirsch S, Barnhill E, Braun J, Sack I, Guo J. Tomoelastography of the abdomen: Tissue mechanical properties of the liver, spleen, kidney, and pancreas from single MR elastography scans at different hydration states. Magn Reson Med 2017;78:976-83. [Crossref] [PubMed]
- Jajamovich GH, Dyvorne H, Donnerhack C, Taouli B. Quantitative liver MRI combining phase contrast imaging, elastography, and DWI: assessment of reproducibility and postprandial effect at 3.0 T. PLoS One 2014;9:e97355. [Crossref] [PubMed]
- Yin Z, Romano AJ, Manduca A, Ehman RL, Huston J 3rd. Stiffness and Beyond: What MR Elastography Can Tell Us About Brain Structure and Function Under Physiologic and Pathologic Conditions. Top Magn Reson Imaging 2018;27:305-18. [Crossref] [PubMed]
- Hiscox LV, Schwarb H, McGarry MDJ, Johnson CL. Aging brain mechanics: Progress and promise of magnetic resonance elastography. Neuroimage 2021;232:117889. [Crossref] [PubMed]
- Siegmann KC, Xydeas T, Sinkus R, Kraemer B, Vogel U, Claussen CD. Diagnostic value of MR elastography in addition to contrast-enhanced MR imaging of the breast-initial clinical results. Eur Radiol 2010;20:318-25. [Crossref] [PubMed]
- Maderwald S, Uffmann K, Galbán CJ, de Greiff A, Ladd ME. Accelerating MR elastography: a multiecho phase-contrast gradient-echo sequence. J Magn Reson Imaging 2006;23:774-80. [Crossref] [PubMed]
- Rump J, Klatt D, Braun J, Warmuth C, Sack I. Fractional encoding of harmonic motions in MR elastography. Magn Reson Med 2007;57:388-95. [Crossref] [PubMed]
- Klatt D, Hamhaber U, Asbach P, Braun J, Sack I. Noninvasive assessment of the rheological behavior of human organs using multifrequency MR elastography: a study of brain and liver viscoelasticity. Phys Med Biol 2007;52:7281-94. [Crossref] [PubMed]
- Klatt D, Yasar TK, Royston TJ, Magin RL. Sample interval modulation for the simultaneous acquisition of displacement vector data in magnetic resonance elastography: theory and application. Phys Med Biol 2013;58:8663-75. [Crossref] [PubMed]
- Yin Z, Magin RL, Klatt D. Simultaneous MR elastography and diffusion acquisitions: diffusion-MRE (dMRE). Magn Reson Med 2014;71:1682-8. [Crossref] [PubMed]
- Yin Z, Kearney SP, Magin RL, Klatt D. Concurrent 3D acquisition of diffusion tensor imaging and magnetic resonance elastography displacement data (DTI-MRE): Theory and in vivo application. Magn Reson Med 2017;77:273-84. [Crossref] [PubMed]
- Ahmad R, Schroeder S, White RD, Kolipaka A. Accelerated MR Elastography for Quantitative Measurement of Myocardial Stiffness. 24th Sci Meet Int Soc Magn Reson Med 2016.
- Guenthner C, Runge JH, Sinkus R, Kozerke S. Simultaneous multislice acquisition for magnetic resonance elastography. Proc Intl Soc Magn Reson Med 2017;25:1132.
- Tzschätzsch H, Guo J, Dittmann F, Hirsch S, Barnhill E, Jöhrens K, Braun J, Sack I. Tomoelastography by multifrequency wave number recovery from time-harmonic propagating shear waves. Med Image Anal 2016;30:1-10. [Crossref] [PubMed]
- Hoodeshenas S, Yin M, Venkatesh SK. Magnetic Resonance Elastography of Liver: Current Update. Top Magn Reson Imaging 2018;27:319-33. [Crossref] [PubMed]
- Allen AM, Shah VH, Therneau TM, Venkatesh SK, Mounajjed T, Larson JJ, Mara KC, Schulte PJ, Kellogg TA, Kendrick ML, McKenzie TJ, Greiner SM, Li J, Glaser KJ, Wells ML, Chen J, Ehman RL, Yin M. The Role of Three-Dimensional Magnetic Resonance Elastography in the Diagnosis of Nonalcoholic Steatohepatitis in Obese Patients Undergoing Bariatric Surgery. Hepatology 2020;71:510-21. [Crossref] [PubMed]
- Murphy MC, Huston J 3rd, Jack CR Jr, Glaser KJ, Manduca A, Felmlee JP, Ehman RL. Decreased brain stiffness in Alzheimer's disease determined by magnetic resonance elastography. J Magn Reson Imaging 2011;34:494-8. [Crossref] [PubMed]
- Hughes JD, Fattahi N, Van Gompel J, Arani A, Meyer F, Lanzino G, Link MJ, Ehman R, Huston J. Higher-Resolution Magnetic Resonance Elastography in Meningiomas to Determine Intratumoral Consistency. Neurosurgery 2015;77:653-8; discussion 658-9. [Crossref] [PubMed]
- Sack I, Johrens K, Wurfel J, Braun J. Structure-sensitive elastography: on the viscoelastic power-law behavior of in vivo human tissue in health and disease. Soft Matter 2013;9:5672-80. [Crossref]
- Yin Z, Glaser KJ, Manduca A, Van Gompel JJ, Link MJ, Hughes JD, Romano A, Ehman RL, Huston J 3rd. Slip Interface Imaging Predicts Tumor-Brain Adhesion in Vestibular Schwannomas. Radiology 2015;277:507-17. [Crossref] [PubMed]
- Yin Z, Hughes JD, Trzasko JD, Glaser KJ, Manduca A, Van Gompel J, Link MJ, Romano A, Ehman RL, Huston J 3rd. Slip interface imaging based on MR-elastography preoperatively predicts meningioma-brain adhesion. J Magn Reson Imaging 2017;46:1007-16. [Crossref] [PubMed]
- Johnson CL, Schwarb H, D J, McGarry M, Anderson AT, Huesmann GR, Sutton BP, Cohen NJ. Viscoelasticity of subcortical gray matter structures. Hum Brain Mapp 2016;37:4221-33. [Crossref] [PubMed]
- Johnson CL, McGarry MD, Gharibans AA, Weaver JB, Paulsen KD, Wang H, Olivero WC, Sutton BP, Georgiadis JG. Local mechanical properties of white matter structures in the human brain. Neuroimage 2013;79:145-52. [Crossref] [PubMed]
- Romano A, Scheel M, Hirsch S, Braun J, Sack I. In vivo waveguide elastography of white matter tracts in the human brain. Magn Reson Med 2012;68:1410-22. [Crossref] [PubMed]
- Barnhill E, Kennedy P, Hammer S, van Beek EJ, Brown C, Roberts N. Statistical mapping of the effect of knee extension on thigh muscle viscoelastic properties using magnetic resonance elastography. Physiol Meas 2013;34:1675-98. [Crossref] [PubMed]
- Schrank F, Warmuth C, Görner S, Meyer T, Tzschätzsch H, Guo J, Uca YO, Elgeti T, Braun J, Sack I. Real-time MR elastography for viscoelasticity quantification in skeletal muscle during dynamic exercises. Magn Reson Med 2020;84:103-14. [Crossref] [PubMed]
- Januchowski R, Świerczewska M, Sterzyńska K, Wojtowicz K, Nowicki M, Zabel M. Increased Expression of Several Collagen Genes is Associated with Drug Resistance in Ovarian Cancer Cell Lines. J Cancer 2016;7:1295-310. [Crossref] [PubMed]
- Heldin CH, Rubin K, Pietras K, Ostman A. High interstitial fluid pressure - an obstacle in cancer therapy. Nat Rev Cancer 2004;4:806-13. [Crossref] [PubMed]
- Dillman JR, Tkach JA, Pedneker A, Trout AT. Quantitative abdominal magnetic resonance imaging in children-special considerations. Abdom Radiol (NY) 2022;47:3069-77. [Crossref] [PubMed]